Image Annotation
Defining Image Annotation
Image annotation for AI projects
Image annotation is an essential but challenging process for many AI companies. Creating effective, efficient image training datasets for machine learning can be a drain on resources and focus for innovators. Management and training concerns can be a distraction for company leaders when they should be focusing on core development goals.
Outsourcing image annotation ensures that computer vision projects have access to precise training images whilst maintaining flexibility and oversight.
Image annotation types
We use a number of different techniques when applying information to AI training images. We can create training data that reflects the diversity of the real world by using these different options:
BOUNDING BOX ANNOTATION
ROTATED BOUNDING BOXES
CUBOID ANNOTATION
POLYGON ANNOTATION
SEMANTIC SEGMENTATION
SKELETAL ANNOTATION
KEY POINTS ANNOTATION
LANE ANNOTATION
INSTANCE SEGMENTATION
BITMASK ANNOTATION
CUSTOM ANNOTATION
3D POINT CLOUD ANNOTATION
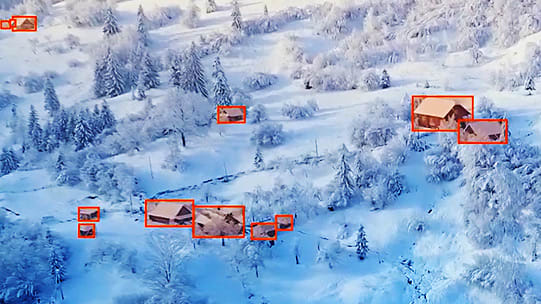
Keymakr’s advanced data annotation tools and our professional in-house annotation team ensure the best results for your computer vision training data needs.
Annotating videos while tracking objects through multiple frames. Each object on the video will be recognized and tracked even through different cameras or separate video segments.
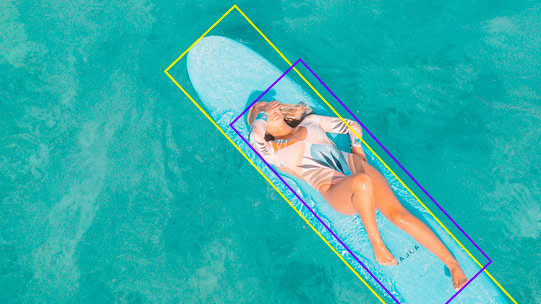
Keymakr’s advanced data annotation tools and our professional in-house annotation team ensure the best results for your computer vision training data needs.
Annotating videos while tracking objects through multiple frames. Each object on the video will be recognized and tracked even through different cameras or separate video segments.
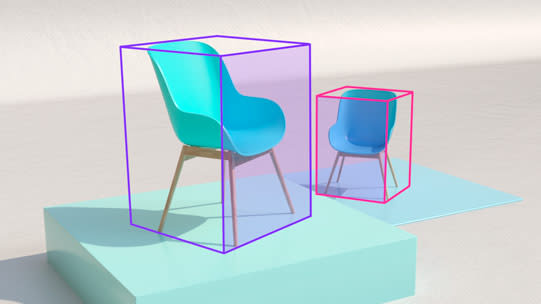
Keymakr’s advanced data annotation tools and our professional in-house annotation team ensure the best results for your computer vision training data needs.
Annotating videos while tracking objects through multiple frames. Each object on the video will be recognized and tracked even through different cameras or separate video segments.
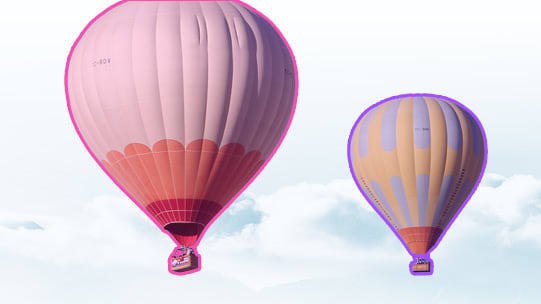
Keymakr’s advanced annotation tools and our professional in-house annotation team ensure the best results for your computer vision training data needs.
Annotating videos while tracking objects through multiple frames. Each object on the video will be recognized and tracked even through different cameras or separate video segments.
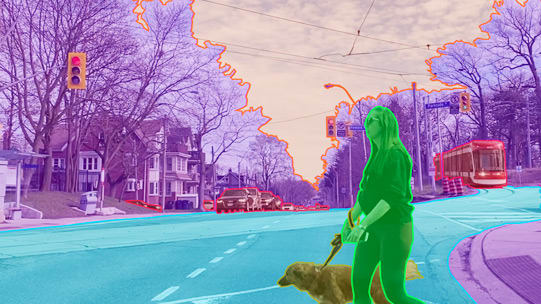
Keymakr’s advanced data annotation tools and our professional in-house annotation team ensure the best results for your computer vision training data needs.
Annotating videos while tracking objects through multiple frames. Each object on the video will be recognized and tracked even through different cameras or separate video segments.
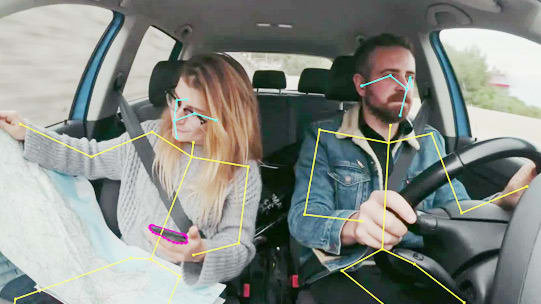
Keymakr’s advanced annotation tools and our professional in-house annotation team ensure the best results for your computer vision training data needs.
Annotating videos while tracking objects through multiple frames. Each object on the video will be recognized and tracked even through different cameras or separate video segments.
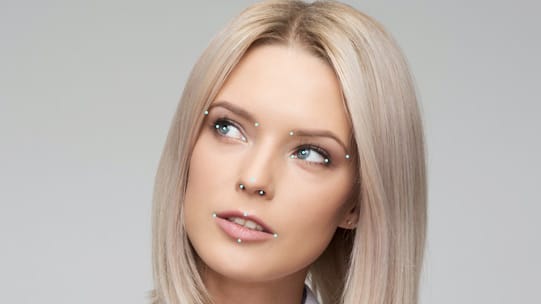
Keymakr’s advanced data annotation tools and our professional in-house annotation team ensure the best results for your computer vision training data needs.
Annotating videos while tracking objects through multiple frames. Each object on the video will be recognized and tracked even through different cameras or separate video segments.
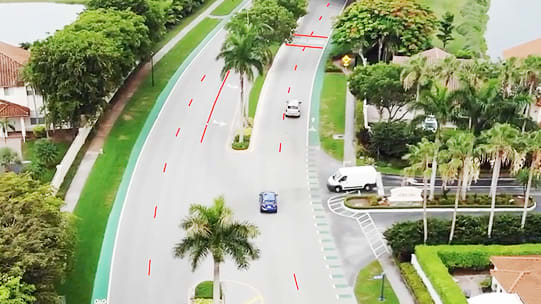
Keymakr’s advanced annotation tools and our professional in-house annotation team ensure the best results for your computer vision training data needs.
Annotating videos while tracking objects through multiple frames. Each object on the video will be recognized and tracked even through different cameras or separate video segments.
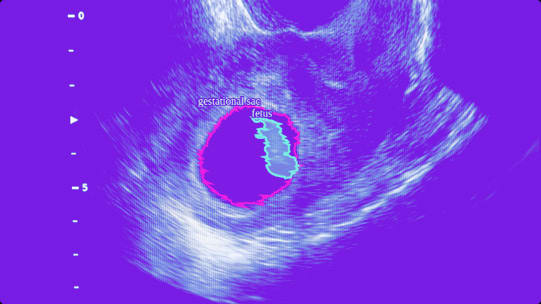
Keymakr’s advanced data annotation tools and our professional in-house annotation team ensure the best results for your computer vision training data needs.
Annotating videos while tracking objects through multiple frames. Each object on the video will be recognized and tracked even through different cameras or separate video segments.
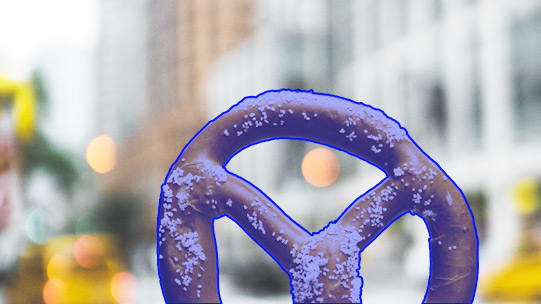
Keymakr’s advanced annotation tools and our professional in-house annotation team ensure the best results for your computer vision training data needs.
Annotating videos while tracking objects through multiple frames. Each object on the video will be recognized and tracked even through different cameras or separate video segments.
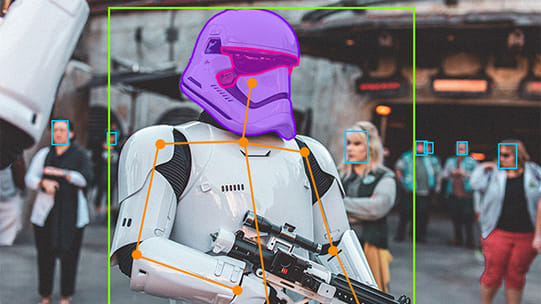
Keymakr’s advanced video annotation tools and our professional in-house annotation team ensure the best results for your computer vision training data needs.
Annotating videos while tracking objects through multiple frames. Each object on the video will be recognized and tracked even through different cameras or separate video segments.

3D point cloud annotation is a process of labeling and annotating the objects present in a 3D point cloud for use in AI projects such as computer vision and machine learning. Point clouds are sets of data points in a 3D coordinate system that represent the surface of an object or scene captured through sensors such as LiDAR or RGB-D cameras.
Annotating 3D point clouds is essential for object recognition, tracking, and scene understanding. The process involves labeling each point with attributes such as object class, size, shape, orientation, and position. This annotation process allows AI models to learn from the data and accurately detect and track objects in real-world scenarios.
Image annotation services and tools
There are lots of options for AI companies in search of image annotation. Companies can choose to use automated image labeling technology to produce datasets. Alternatively, they can choose to employ the services of data annotation providers, like Keymakr.
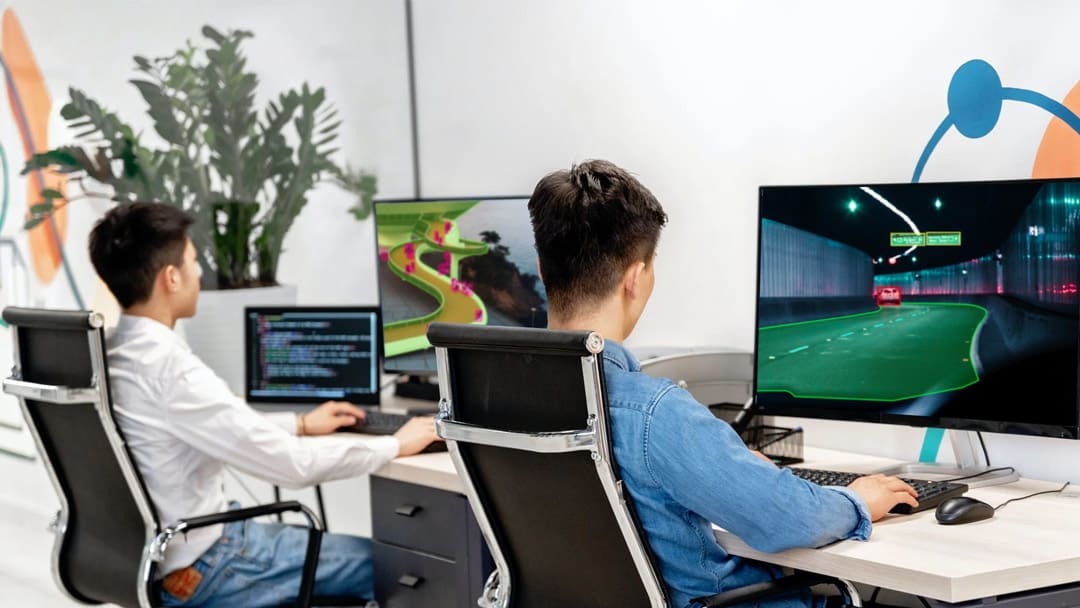
Image annotation services
Outsourcing data annotation to dedicated services, like Keymakr, saves computer vision developers time. It also guarantees high-quality and responsive image annotation. Human workers must locate, outline and label objects and people in order to annotate images. A wide variety of images require this careful annotation treatment.
Consequently Keymakr employs a large team of experienced annotators who construct exceptional datasets, according to the most demanding specifications.
Automated annotation
There are a number of AI assisted labeling tools that can speed up the image annotation process. This sometimes means producing automatic polygon outlines of objects for semantic segmentation, but it can also mean auto-labeling objects across thousands of images.
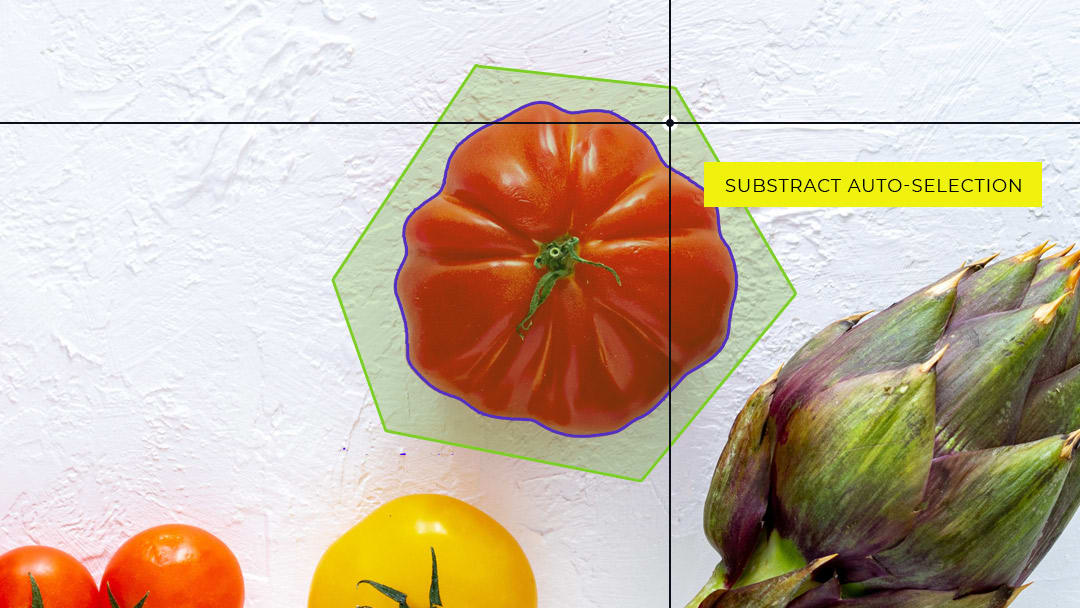
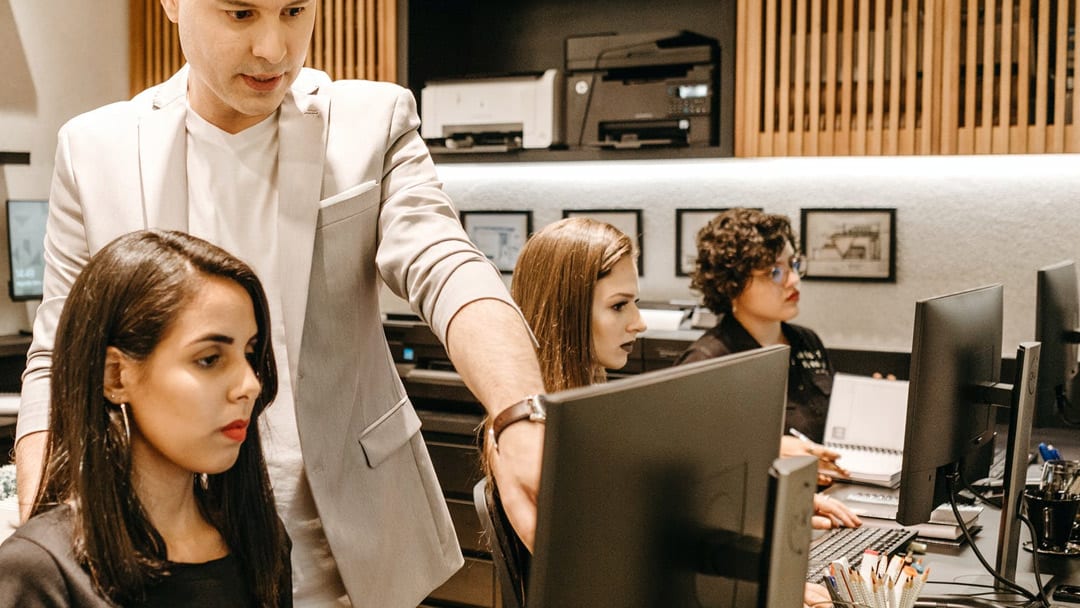
Annotation services vs automated tools
Auto-labeling platforms can create training datasets quickly. By bypassing many human performed labeling tasks this technology reduces labour time and labour costs. However, relying too heavily on automated annotation can have a negative impact on image data quality.
Keymakr’s in-house team is capable of working with a wide range of annotation methods and types. They are also led by experienced managers who know how to guide a large scale image annotation project.
In conclusion, outsourcing to annotation providers lifts the burden of hiring and training from AI innovators, whilst maintaining a high level of precision and quality control.
Smart task distribution system
Keymakr’s worker analytics capabilities make it easy to assess the skills and performance levels of individual annotators. With the help of this information Keymakr’s smart task distribution system assigns tasks to annotators according to their strengths and weaknesses. This means higher levels of precision and productivity.
24\7 monitoring and alerts
Keymakr’s proprietary platform also allows managers to see information about the progress of a project at any time. The platform also gives alerts when there are any problems with data quality, or if a labeling task is off schedule.
Vector or bitmask
Keymakr offers both bitmasks and vector graphics to suit the needs of any computer vision project. Keymakr can also easily convert between image types if necessary.
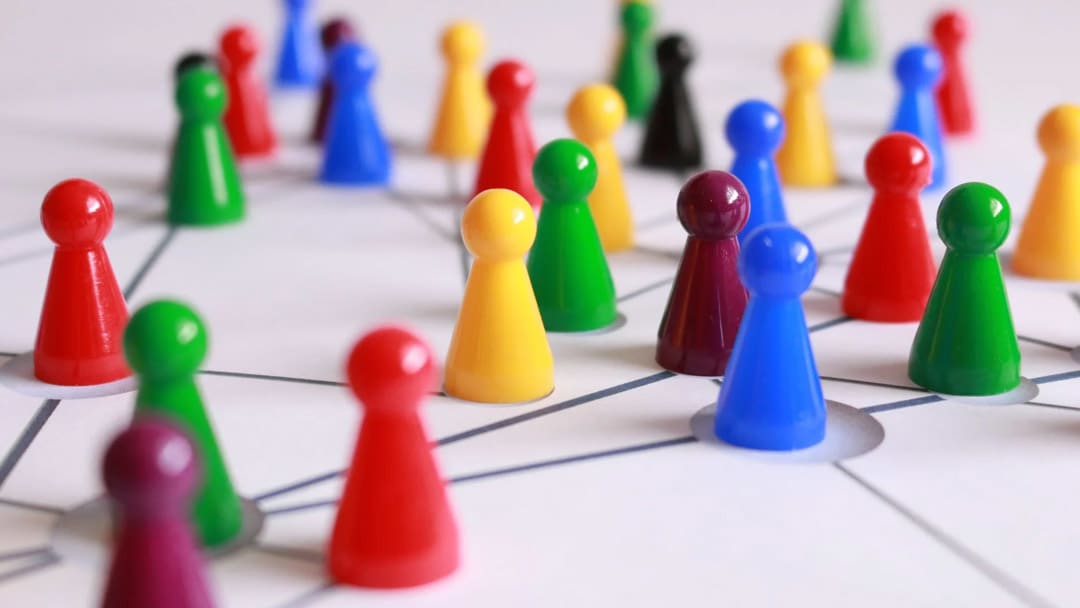
Image annotation use cases
Keymakr provides AI companies with accurate and flexible image annotation. As a result Keymakr has played a role in a number of exciting AI use cases:

Automotive industry
Keymakr annotated over 20,000 images of roads from Europe and North America. Annotators then segmented these images, and assigned each pixel to a particular object class (cars, trees, sky, road, signs).
These training images allow autonomous car models to reliably identify objects in their surroundings and navigate accordingly.
Semantic segmentation helps train autonomous vehicles or robots. For example, Autonomous cars need to be able to see the difference between a tree and a pedestrian. The images we annotate help train models capable of processing these differences. This is critical to guide safe driving.
Skeletal annotation for sports
Adding skeletal annotation to images of sports can help computer vision models to interpret human body positions. Skeletal annotation powers sports training and coaching AI applications.
For example, a sports brand could create an AI application to assess athletes. It could define how well they are doing in their regimen based on their skeletal movements. You can teach to predict how an athlete performs using skeletal annotations. Skeletal is ideal for human body shapes. Apply the skeletal tool to indicate joints and bones.
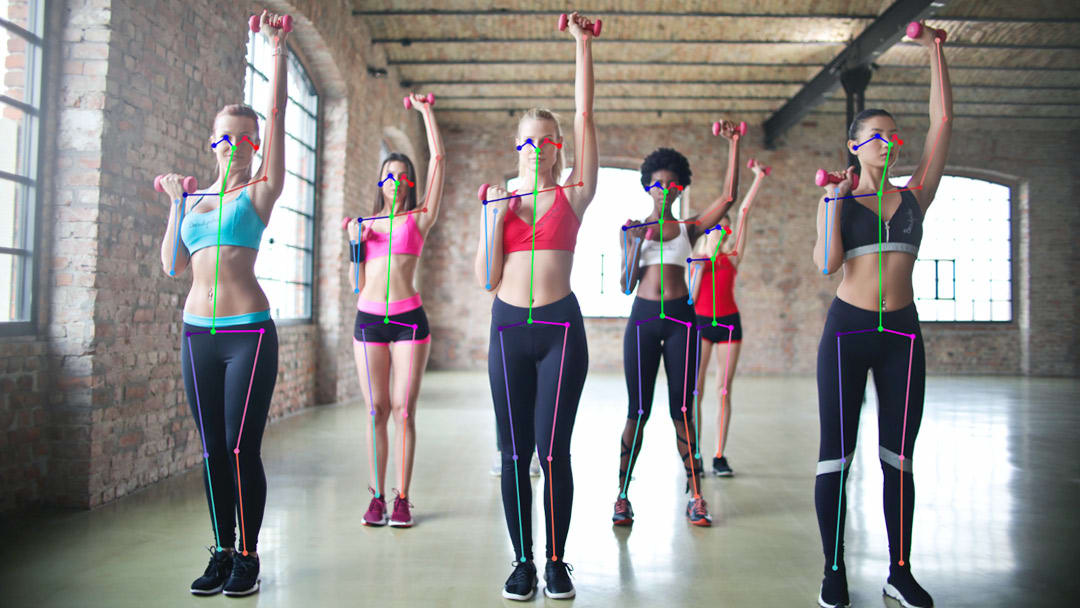
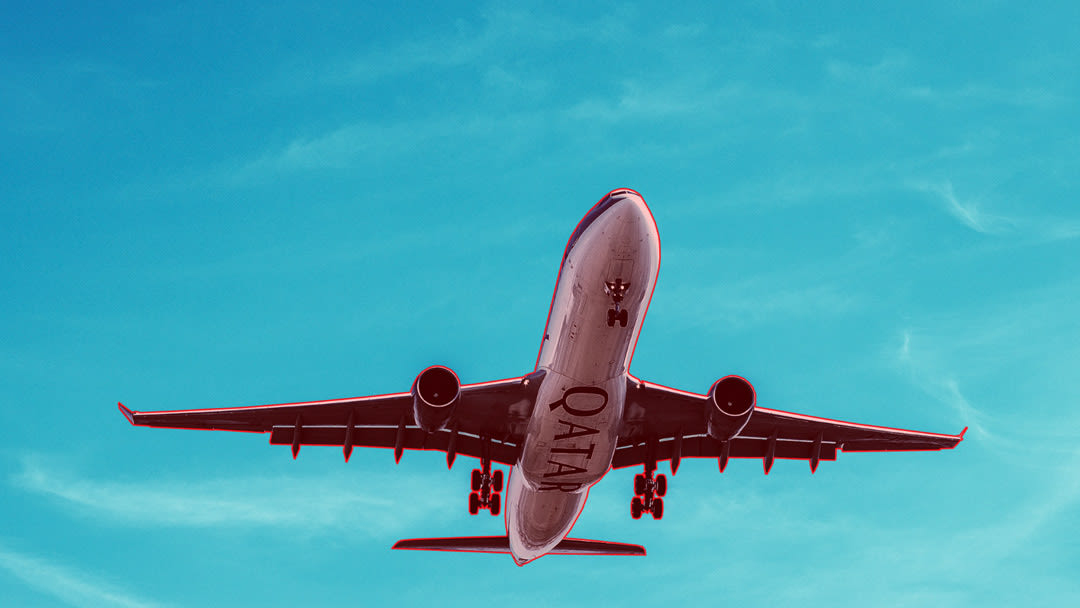
Polygon annotation for the insurance sector
By adding polygon annotation to images, annotators can help image data to capture complex and irregular shapes.
By outlining images of car parts with this technique, Keymakr’s team created a dataset for an insurance industry client.
The computer vision model trained with this data is capable of rapidly, accurately and autonomously processing insurance claims.
Facial recognition for security
Facial recognition is a valuable tool for security and law enforcement. These techniques can help determine suspects in police lineups. You can guide a computer vision model to recognize human faces. You can also search large databases to find individuals who have committed crimes.
Those interested in facial recognition for security should learn about face detection algorithms. There are many algorithms for processing human faces, but only some techniques work well on all faces.
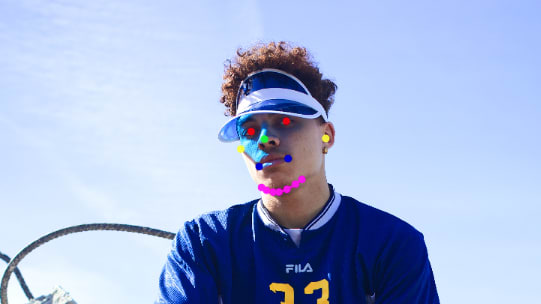
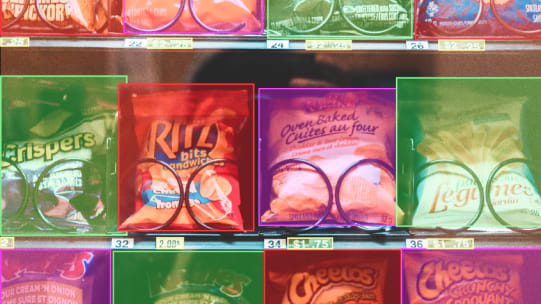
Bounding box for retail
The bounding box is straightforward, but it works well for many everyday things. You can use the bounding box annotation to recognize objects in retail shops. A 2D or 3D bounding box is a valuable tool for machine learning.
Train AI models to perform visual searches. Boxes can help you determine products quickly, and they are well-suited for application in retail environments. The semantic segmentation function determines and classifies objects in a series. You can use the bounding box annotation to guide a classification model. Or build object detection algorithms.
Cuboid annotation for real estate
When you're working in real estate, there are many types of objects to identify. The cuboid tool and segmentation annotation tool can help. You can use these shapes to accurately recognize 3D objects in photos to scale.
The cuboid tool lets you draw a three-dimensional shape around an object. This way, you can capture its depth and scale. For example, suppose you're working on a real estate project. You want to guide a model that can recognize buildings. The cuboid tool can draw a box around the building's features.
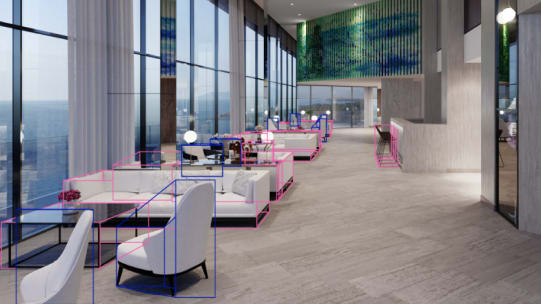
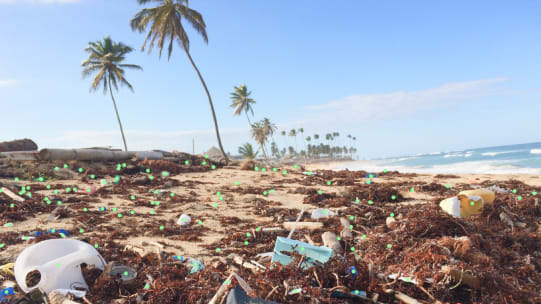
Points annotation for waste management
In waste management projects, you can train AI models to recognize trash in photos. The points tool lets you draw single points. The points tool is perfect when you have a dataset processing pictures of waste. You can employ points to point out where there is trash and where there isn't.
Train to recognize whether an item is plastic, metal, organic, or inorganic (like glass). Then, it uses semantic segmentation to recognize various types of waste and identify what to recycle. You can guide a computer vision model with a dataset with waste photos.
Image annotation for medicine
Image annotation is a great way to help doctors diagnose medical conditions. It can teach about patients with different diseases and symptoms. You can teach by showing it thousands of images annotated with the image labeling tool. Then you can teach it to check the condition automatically.
Doctors can engage machine learning to make diagnoses and recommend treatments. For example, radiologists may determine types of tumors in CT scans. Then they can refer them to specialists for further evaluation. Annotated images can recognize potentially cancerous cells or malformed blood vessels.
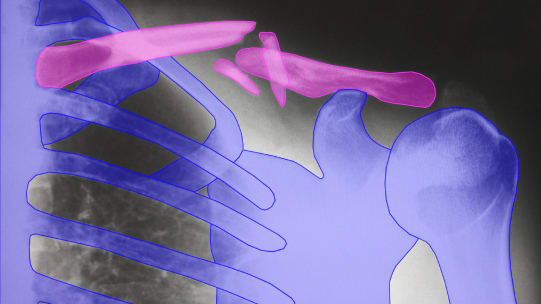
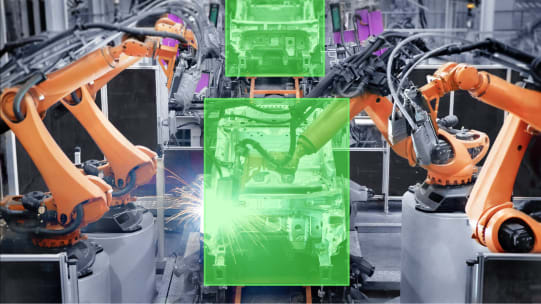
Bounding boxes for robotics and automation
They are used in robotics to determine where objects are located. The bounding box is also helpful for automation. You can have the device processing repetitive tasks without human intervention. Similarly, rotating bounding boxes can help create boxes around rotated shapes.
You can guide a model by processing thousands of images. Involve boxes to tell a robot where to place an object or how far it should move its arm. For example, suppose you want a drone to drop off a package in someone's hand. You'd create an image annotation of people holding objects of various sizes and shapes.
Polygon annotation for fashion
Polygons can capture the shape of clothes. These techniques work well for clothing. It's easy to label the location and size of each piece of apparel. It's useful for companies that want to monitor inventory.
The skeletal and polygon tools are also helpful for fashion designers and stylists. You can ensure clothes look good before sewing them. The polygon and skeletal tools can help define digital sketches of clothing before making it. So, you don't waste time sewing together pieces that don't fit well.
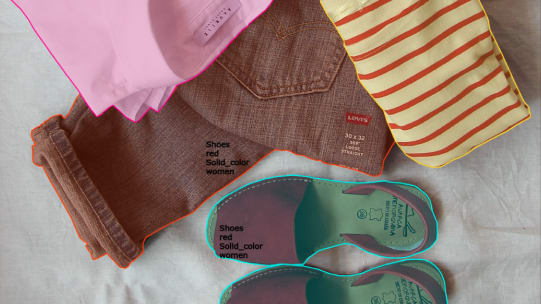
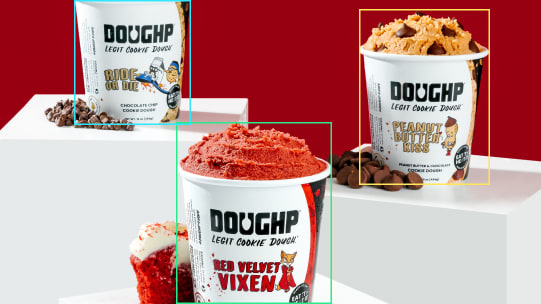
Image annotation for advertising and marketing
Annotation can help companies make better advertisements. Use the image annotation tool to label images of products to make it easier to find and define specific objects in ads. You can teach an ML model to find out a particular brand of coffee, for example.
Create product ads that are more effective, or find the best products to include in an ad. Then, decide which products to feature in future advertisements. You can also identify popular products and their location in a store.
Cuboid annotation for livestock
Companies that specialize in livestock can employ cuboid to identify specific animals. It helps farmers manage their herds more efficiently by finding out which animals are sick or injured. You can also exploit these tools to find the best breeding stock or locate missing animals.
First, you need to label images or videos of animals and their surroundings. Annotate photos of cows so you can define if they're in good health or need medical attention. Next, guide to identify specific ailments or injuries from shapes.
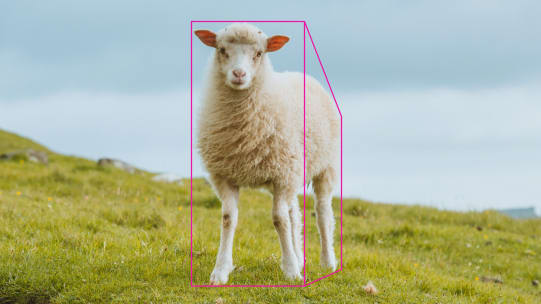
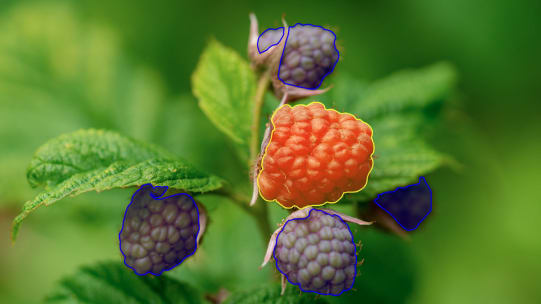
Image annotation in agriculture
Apply cuboids, bounding boxes, or another image annotation tool to identify specific areas of the field. Polygons help mark individual plants, like corn stalks or wheat. Involve polylines to create boundaries between different crops.
For example, you could apply polygon to help robots identify individual plants and determine their growth stage. This information can help farmers more efficiently manage their crops. Locate plants ready for harvesting or decide which ones need attention.
A Beginner's Complete Guide to Image Annotation for Machine Learning
Why does image annotation matter in the world of machine learning? The answer is simple. The images you use to train, validate, and test your algorithms will directly impact the performance of your AI project.
Every image in your datasets matters. The goal of a training dataset is to train your AI system to recognize and predict outcomes—the higher the quality of your annotations, the more accurate and precise your models are likely to be.
But image annotation isn’t always easy, especially if you’re dealing with large quantities of diverse data. Getting familiar with ML image labeling is one of the fastest ways to get to market with a high-performing, meaningful machine learning model.
Interested in boosting the performance of your next AI project? We’ve done the legwork and put together a comprehensive guide to image annotation types, tools, and techniques.
What is image annotation?
Image annotation is the process of labeling an image to show a machine learning model which features you want it to recognize. Annotating an image creates metadata through tagging, processing, or transcribing certain objects within the image.
Training a machine learning model to recognize desired features requires the principles of supervised learning. The goal is for your machine learning model to identify desired features in a real-world environment—and make a decision or take some action as a result.
Annotation is the process of labeling with additional information. It's most common in ML and computer vision applications. Examples include classification or object detection. Adding descriptive tags can guide a PC on what it looks at. And you can teach it to understand its environment.
Semantic segmentation is a process of segmenting pixels into meaningful regions such that each part can be attributed with a specific label, indicating what type of object it is. Semantic segmentation techniques define and classify object areas in elements, such as roads and sky.
Training to recognize desired characteristics requires a supervised process. The goal is to identify desired qualities in a real-world environment using the segmentation annotation tool. And after, decide or take action on the results.
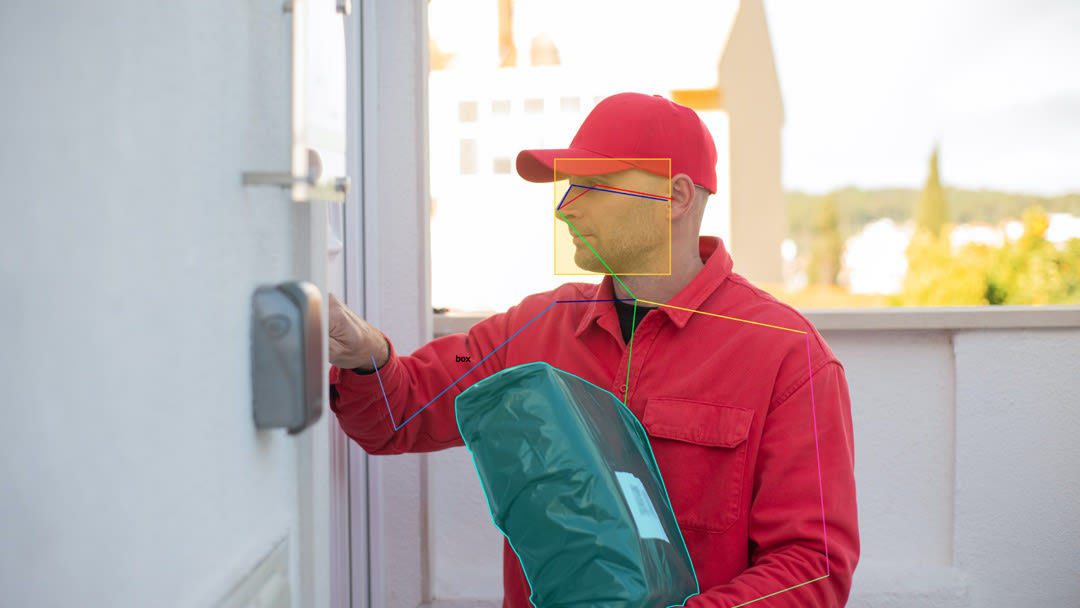

Image Annotation Process
Annotating is a labor-intensive process. The person who performs it is called an annotator. It requires knowing how to use image annotation software within the context of your industry. And then, you draw a skeletal box, polygon, and other shapes around the objects that meet your criteria.
Annotator must be familiar with the subject matter, have an eye for detail using the tools, and have a high level of accuracy. It is time-consuming and expensive. Most projects are limited in scale by the number of annotated data sets available.
Here's how it works. The annotator creates image training datasets by taking images or videos and drawing shapes around them. They then label each with one or more labels that describe what's in it.
Here's how it works. The annotator creates image training datasets by taking images or videos and drawing shapes around them. They then label each with one or more labels that describe what's in it.
Next, they exploit the set to guide an algorithm to perform the same task. The algorithm compares each in the test set and produces an output that indicates how well it's learned from its data.
Semantic segmentation is a type of segmentation that uses ML to help devices recognize, label, and segment objects. These techniques were initially developed for medical imaging, but it is quickly being adopted across many industries, including autonomous driving and aerial mapping. The method is also used to segment attributes.
Image Annotation Types and Techniques
There are many different types of image annotations. Each one is distinct in how it classifies particular features or areas of an image. Here are a few examples:
- Image classification: This form of annotation trains your model to recognize the presence of similar objects based on similar collections of objects that it’s seen before. For example, a data annotator using image classification could tag a kitchen scene as “kitchen.”
- Object detection: Otherwise known as object recognition, this type of image annotation detects the presence, location, and number of certain objects in an image. For example, a street scene can be separately annotated with bikes, pedestrians, vehicles, and other objects.
- Segmentation: There are two main types of image segmentation. Semantic segmentation outlines the boundaries between similar objects (e.g., stadium vs. crowd) while instance segmentation labeling marks the occurrence of every individual object within an object class (e.g., every person in the crowd).
In addition to tools, there are a variety of image annotation techniques. Commonly used methods include:
- Bounding box labeling: Annotators draw a box around target objects.
- Landmarking: Characteristics (such as facial features) within the image are “plotted.”
- Polygon labeling: Irregular objects are annotated by their edges.
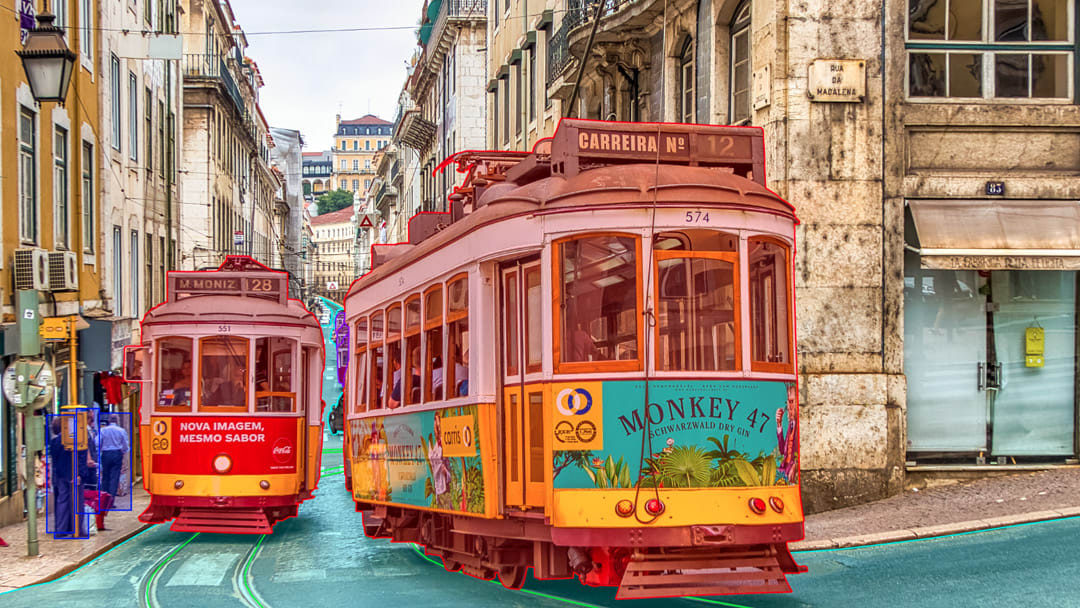
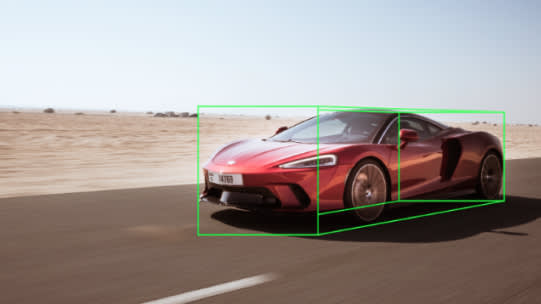
The right image annotation tool can help get the job done faster and with fewer errors using automatic image labeling. These are available on today’s market as open source or freeware image labeling tools.
If you’re working with an immense volume of data, you will need an experienced team of data annotators. Depending on the diversity of your datasets, more than one type of image annotation tool will be required.
Image annotation can often be a daunting task. Without the right tools, techniques, or workforce, you compromise on quality, precision, and the time it takes to get to market. That’s why AI companies often rely on professional data annotation services to label datasets for machine learning.
Popular algorithms for annotating images
Standard algorithms for image training datasets include neural networks, convolutional neural networks (CNNs), and recurrent neural networks (RNNs).
Neural networks
Neural network algorithms consist of connected nodes in layers. The first layer receives input and then passes it to the next layer. The next layer modifies the data in a particular way. Next, it passes through the third layer until it reaches the final output.
You can guide neural networks using backpropagation and gradient descent algorithms. The backpropagation algorithms calculate the gradient of a loss function. In other words, it can determine which variables affect your model's output and which do not.
The gradient descent algorithms update your neural network. These algorithms allow you to find the optimal values for each node in the network. Then, it can produce an output that best matches what you want.
Convolutional neural networks (CNNs)
CNNs are neural network algorithms that apply many layers of nonlinear processing units. They are helpful for recognition and segmentation tasks. Some examples include object detection, object tracking, and scene recognition.
These computer vision algorithms have multiple layers commonly used for classification tasks. For example, you can identify whether it contains a category or object.
Semantic segmentation is a type of computer vision problem used to detect and classify pixel-level regions into discrete categories. Semantic segmentation makes it possible for machines to understand what is happening.
The layers of a CNN include input, convolutional, pooling, and fully connected layers. The input layer receives the picture as an array of numbers. Then it passes it to the first convolutional layer for processing. Each convolutional layer uses many filters that extract features from the previous layer. Then they apply weights.
These weights pull elements from various parts, such as edges or corners from the software image. The pooling layers reduce the feature map's spatial size. And the fully connected layers combine these characteristics to classify.
Recurrent Neural Networks (RNNs)
RNNs are neural networks containing at least one feedback loop between nodes in the hidden layer. These algorithms are great at rich visual representations. And you can execute them for label accurately.
The difference between CNNs and RNNs is that CNNs classify images based on semantic segmentation. RNNs employ algorithms to predict the label given its features.
A recurrent neural network has two main components. They are a feed-forward layer and a feedback layer. The feed-forward layer receives input from an existing dataset. Next, it produces an output based on the amount of processing in each example.
CNNs are good at representation of the entire picture. RNNs are good at representations of specific parts. They're both useful algorithms for many tasks.
SIFT (Scale-Invariant Feature Transform)
SIFT is a feature detection algorithm. It's an essential component of Object Detection and Classification. Apply these algorithms for classification, object detection, and scene recognition.
The result is like the human visual system. It can identify distinctive regions invariant to scale, position, rotation, and illumination. It's also robust to small deformations in the shape of an object, such as warping or bending.
HOG (Histogram of Oriented Gradients)
HOG algorithms are for feature extraction for object detection and tracking. It's a simple but powerful feature processing for object detection and tracking. It's straightforward to implement and compute. These characteristics make it ideal for real-time applications. Examples include robotics, surveillance systems, and driver assistance devices.
Fisher Vectors
Fisher Vectors are dimensionality reduction techniques that can reduce dimensions. Using this method, you can reduce the pixels by up to 80%. Simultaneously, you maintain its structural information.
Fisher vectors are a feature descriptor for classification and object detection. It's a compact representation of a patch for fast matching in large databases. It's invariant to translation, rotation, scaling, and aspect ratio changes.
Locally Aggregated Descriptors (LADs)
LADs are a general-purpose feature extraction method for object detection, recognition, and tracking. You can apply them as a stand-alone descriptor or in conjunction with other characteristics. LADs are robust, fast, and easy to compute. They're helpful for object detection and recognition tasks. Engage them in urban surveillance and traffic monitoring applications.
They add local descriptors into a global classification descriptor. The algorithm assumes that objects have distinctive local features. These are consistent across different views of the same object.
ORB (Orientation-Based Recursive Best Matching)
ORB is a method for detecting objects and retrieval. The algorithm generates a set of feature vectors from the input. Then, it matches these vectors against features stored in a database.
It uses recursive best-matching to detect objects. A feature descriptor (e.g., SURF) helps calculate the instances in a patch.
The list is not exhaustive. You can employ many other methods for processing and feature extraction. But, it's essential to understand the available approaches to choose the right one.
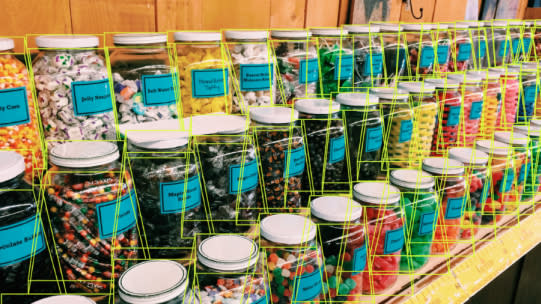
Professional Image Annotation Services
Machine learning models are only as good as the data that is used to train them. Keymakr has the skills, equipment, and expertise necessary to deliver pixel-perfect results that align with your timeframe and budget.
Are you interested in high-quality training datasets that have been labeled according to your standards and specifications? Get in touch with a team member to book your personalized demo today.
Distinct strategies for annotating images
There are many ways to annotate photos, depending on your need to scale and resources. Humans complete manual annotation, labeling, and objects with various attributes. Semantic segmentation also referred to as object segmentation, is a task in computer vision.
This process can be time-consuming and expensive, especially when processing large datasets. It may be the most effective method if you have a small one. But, as it grows and gets complex, it's essential to consider other options. Apply semantic segmentation for annotation too large to annotate manually.
Human-in-the-loop approach
Human-in-the-loop approach uses humans to train the ML model. This process improves the accuracy of the final product.
This approach to uses manual annotation to train an AI algorithm. Typically, you start with a small set of humans and expand to include more over time. You can apply this approach to large-scale automated annotation.
A human-in-the-loop approach labels with ML methods such as neural networks. This approach is suitable for small sets that don't require frequent changes. It can be more efficient than manual annotation. But you need to ensure accuracy before deploying.
Automated data annotation
This is a process used to label. It's typical for AI models but can also apply to other types. Annotation differs from human-in-the-loop (HIL) approaches. It doesn't involve direct human intervention.
It uses computer vision and ML methods to label images with attributes. It's suitable for large-scale, repetitive tasks and datasets with frequent changes. The most common methods for automatic are active in independent and semi-supervised studying.
You can also employ a hybrid approach. It combines the strengths of both manual and AI-automated. This method is more flexible than the manual alone. You can also use it to scale and improve the accuracy of ML algorithms.
HOG (Histogram of Oriented Gradients)
HOG algorithms are for feature extraction for object detection and tracking. It's a simple but powerful feature processing for object detection and tracking. It's straightforward to implement and compute. These characteristics make it ideal for real-time applications. Examples include robotics, surveillance systems, and driver assistance devices.
Active learning
In an active process, a human provides feedback to tell the system. A human labels a subset, and the PC exploits those labels to guide an AI model to label the remaining ones.
It is an efficient way to label because it only uses needed data. It can reduce bias and ensure that your training set includes diverse images. This method can also improve the quality.
Active learning is a good approach for tasks that require human feedback. Apply it to understand the problem domain better. It is beneficial when you have a large amount of unlabeled data.
For example, you can use active learning to label the images when building a classifier or object detector. This way, humans provide labels for only the examples that are difficult to recognize.
Semi-supervised learning
A semi-supervised approach uses an existing set. Then, it uses algorithms to learn how to label new images. The semi-supervised process uses labeled and unlabeled data to guide a model. Humans provide labels for some subset of your AI training set but not all.
Suppose you want to train a model that can identify animals in photos. In that case, you'll need labeled images of animals. Additionally, you need unlabeled ones of those same animals.
An iterative process is the best way to ensure a high level. In each round, the human labels a subset. Next, the PC uses those labels to guide an AI model to label the remaining ones. This approach allows you to focus on more challenging examples for your algorithm. It's also less time-consuming for humans.
How to find quality data
Before you can even think about your model, you need clean data to input into it. The more it relates to the problem you are trying to solve, the better. Suppose your dataset is not representative of your problem space. It will be difficult for you (and anyone else using it) to get meaningful results.
There are several ways to get data for your model, but there is no single best way. Your options will depend on the types of problems you want to solve, your resources, tools, and your expertise.
It is best to start with publicly available sets if you are a novice. You might also be able to find data from existing clients or customers that can be used for training and testing. Otherwise, consider partnering with another organization with access to the data you need.
Where to get the best training data
There are many sources that you can exploit to guide your model. Examples include the following:
- Internal
- Public sources, such as the US Census Bureau and the World Bank
- Academic datasets from universities around the world
- Private companies that collect information about their customers
- Government
- Publicly available (such as those from Kaggle)
- User-generated (such as social media posts or user reviews)
- Open datasets (census data, social media posts from websites and platforms
(e.g., Twitter), or even news stories from specific sources (e.g., CNN or BBC) - Publicly available (such as Freebase)
- Free APIs
Before using it, you should check if restrictions apply. To access these sets, you need to write code to read them. Then, you need to convert them into the correct format.
There are many ways to access data for ML. If you have a specific task involving public data, contact the owners and ask permission to access it. Alternatively, you can choose a third-party service that provides access to various APIs. Find out which API offers this kind of data and try to get API keys from them.
Creating training data
This process allows you to control the information in each record. You can see how many records are available for each input variable. Also, you can create data by training on existing sets. Then, employ it to predict missing values. A common way of doing this is by using transfer learning. Another option is to generate synthetic AI data sets.
The second option allows you to create data that matches your model's encounters when deployed. You can also opt for this approach to build and scale models more representative of the real world. For example, you can include aspects of human-curated datasets. You can also remove irrelevant features or add missing values.
The biggest drawback of creating it is that it can be a lot of work. The process can be time-consuming and requires domain expertise. The second issue is if you want to guide a model on sensitive information. Examples include medical records and personal information. It's critical to take steps to protect people's privacy. You must ensure you don't reuse datasets for other purposes.
Formats that produce the best results
Data is often available in various formats and sources. For example, social media is usually JSON, while government datasets may be in CSV or XML. As you're scoping out formats and transforms, it pays to keep a few things in mind.
For instance, suppose you have big images (such as photos) and many samples per image. If each sample is a pixel, a compressed format like TIFF might be better than JPEG or PNG. It takes less space while retaining quality.
But suppose your data contains many small ones like illustrations or diagrams. These may not require high resolution for small-scale projects. You could choose JPEG as the format with little loss in quality or file size reduction.
How to check data for quality and accuracy
It is essential. It will be harder to train your AI model if you have missing values, corrupted entries, or incorrect labels.
It can be inaccurate in many ways. It may have been corrupted during transmission. You should check the accuracy of your dataset. These include checking for:
- Missing values (for example, if someone forgot to record particular information)
- Outliers (values that are much higher or lower than others)
- Normal distribution
The accuracy of your data is a key factor in success. No matter how well you guide, the models cannot function if your information is inaccurate. To check the accuracy, look at a sample. Next, compare it with other known information about that subject. Examples include historical records and others. It would help if you used tools like Pandas or Numpy.
Be aware of data with a bias
Just because you've found high-quality data doesn't mean it's unbiased. Even if your dataset is large and diverse, there could still be bias lurking within. Bias can be insidious. It may not be evident at first glance, but it can greatly impact your data.
Bias can happen when researchers select data based on demographics for one project. But they don't necessarily apply to others. For example, researchers may ask a question only relevant to specific groups. Or they might employ a sample size that doesn't represent the population as a whole. In these cases, your data could have bias.
Your Workforce for Image Annotation
Humans are a crucial part of building AI. Suppose you want to identify objects or people. You need a workforce who can manage software to label and annotate photos. But how do you find the right workforce?
If you've decided to hire an internal workforce, it's essential to know when to bring in the pros. If you're new to AI, it can be tempting to take on the task yourself. The proper workforce can help you build an accurate dataset. They ensure that your model doesn't mislabel images.
Inhouse Workforce
When you assign annotation to an inhouse AI workforce, it's crucial to understand some practices. First, how long it takes your team to annotate? Remember that your inhouse workforce has extra responsibilities besides annotating.
The time can vary based on the complexity of the task. It also depends on whether the workforce is trained specialists. It could be problematic if your inhouse employees have never worked with similar data.
Inhouse teams can work on small projects. But this workforce usually isn’t equipped to handle large ones. Suppose your internal workforce has no experience with tagging tools or machines. It might take longer to learn how to do it well than if you hired an external workforce. A company specializing in annotation may be better for a large dataset.
Creating training data
This process allows you to control the information in each record. You can see how many records are available for each input variable. Also, you can create data by training on existing sets. Then, employ it to predict missing values. A common way of doing this is by using transfer learning. Another option is to generate synthetic AI data sets.
The second option allows you to create data that matches your model's encounters when deployed. You can also opt for this approach to build and scale models more representative of the real world. For example, you can include aspects of human-curated datasets. You can also remove irrelevant features or add missing values.
The biggest drawback of creating it is that it can be a lot of work. The process can be time-consuming and requires domain expertise. The second issue is if you want to guide a model on sensitive information. Examples include medical records and personal information. It's critical to take steps to protect people's privacy. You must ensure you don't reuse datasets for other purposes.
Third-party services
When you outsource your data projects, it can save your workforce time and resources. You can handle the job yourself when your company is first starting and the volume of images to annotate is low. But, once the number of images increases, it's time to get external workforce services.
Another reason to consider image annotation service professionals is if images require higher expertise from the workforce. For example, you are working with medical imagery. You may want an expert workforce familiar with these.
How to set up your internal workforce
If you're setting up your internal workforce, there are a few practices to consider. First, ensure that the workforce team has experience.
Second, ensure your workforce has training how to exploit each label. If possible, provide them with examples of sets labeled externally. Ask them to mimic those practices as closely as possible.
Here are some tips for setting up an internal team:
- Choose a leader who can manage the workforce and tasks.
- Guide your team and provide the workforce with a style guide.
Include examples of good and bad annotations in your materials.
This way, everyone knows what you want them to do when labeling data. - Centralize access to data for the workforce.
- Set up a regular workforce schedule for your team to meet.
This way, the workforce can work together to discuss each dataset
and give feedback on how it should be labeled. - Set clear expectations about the quality
expected from your team workforce. - Ensure each is labeled consistently and correctly
before moving on to the next dataset.
To set up your internal workforce, you'll need to ensure they have the image labeling tool, machines and training. The first step is determining what software and machines are necessary for the project. KeyLabs tool helps you annotate quickly and efficiently for your workforce.
When to Hire Professionals
You're working on an AI project with thousands of images. Each one needs to be annotated, and it takes your workforce an average of two minutes per image. That's over 33 hours to annotate the photos.
Suppose you have an extensive database you need to annotate. Also, there is not enough time for your workforce employees to complete them. In that case, you can outsource to a professional annotation service team.
Outsource your needs to us. Keymakr’s workforce and AI-powered tools can help you build solid datasets. Use them for your ML projects, including those for deep learning. Allow your data science team to focus on creating better models.
Questions to ask your image annotation provider
When searching for the right professionals, asking the right questions is essential. You ensure you get a clear idea of what your service provider can offer and how to help you achieve your goals. Here are some questions to ask:
Q: What is the turnaround time for getting annotated images?
A: The turnaround time is how long it takes to get your annotated images back after you submit them. Some companies offer same-day or next-day turnaround, while others take longer.
You can ask for an estimate based on your needs. But keep in mind that prices may change. Depending on how many images need annotating, the turnaround time can vary. The time also depends on how complex the task is and any other special requests.
Q: How do you ensure that your annotations are 100% accurate?
A: Annotations that are 100% accurate need strict control practices. We use a combination of human professionals and ML technology. This process ensures that each is reviewed multiple times by trained experts.
Regular audits of the database ensure that data meets the highest industry standards. Many companies also offer free revisions. So you can request changes if something isn't right.
Q: What type of clients have you worked with before?
A: We work with clients in the automotive, aerial, security, robotics, waste management, medical, retail, fashion, sport, agriculture industries, and many more.
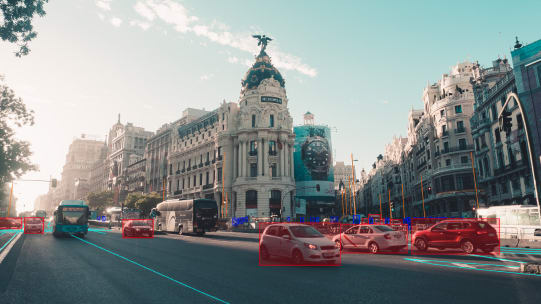
Conclusion and links to the relevant materials
Machines learn more and deliver better results with high-quality, diverse datasets. It's not easy to build them from scratch. We help you generate them. With the help of an AI-powered service like ours, you can annotate images for your ML projects.
To learn more about this critical area of computer vision, consider checking out the following resources:
- How Image Annotation Can Support Emotion Detection AI
- Image Annotation for Virtual Fitting Rooms
- How Image Annotation Enables Facial Recognition in a World of Masks
- How Image Annotation is Pushing Forward Object Detection in AI
- How Image Annotation Projects Transform Waste Management
- 3 Industries That Can Be Improved With Image Annotation
- The 4 Most Common Types of Image Annotation for Computer Vision AI
- Top 6 Qualities To Consider When Choosing Image Annotation Services