Automated Data Labeling vs Manual Data Labeling: Optimizing Annotation
Accurately labeled datasets are the raw material for the machine learning algorithms and deep learning revolution. Vast quantities of data are required to train new target object generations of Artificial Intelligence (AI). Correctly labeled images train computer vision systems to reliably distinguish between a stop sign and pedestrian. Or it can be a choice between a raised hand and a raised gun.
Demand for data labeling for vision-based machine learning is therefore growing rapidly. AI developers increasingly need larger training datasets that maintain the high level accuracy that is so vital for safety and reliability.
How do we go about creating the accurate, scalable datasets that industry needs? To begin answering this question we first need to consider automated data labeling vs manual data labeling. The differences between these approaches to data labeling point the way forward for smart dataset creation.
Automatic Data Labeling: Machines Training Machines
Automatic data labeling processes and image processing techniques have the potential to overcome some of the challenges presented by the laborious annotation cycle. After training from a labeled dataset, a machine learning model can be applied to a set of unlabeled data.
The computer vision models should then be able predict the appropriate labels for the new dataset during the image detection. Automated data labeling and image segmentation algorithms can be improved via human input. After the AI has labeled the raw data, a human annotator reviews and verifies the labels. Accurately labeled data can then take its place in the training dataset and projects on image processing.
If the annotator observes mistakes in the labeling they can then proceed to correct it. This corrected data can then also be used to train the labeling AI.
The Auto-label AI is capable of handling the majority of easily identified labels. This has the advantage of greatly speeding up the initial labeling stage. However, automated data labeling still produces a significant amount of errors. That could prove costly when fed through to an AI model.
Manual Data Labeling: The Human Touch
Manual data labeling generally means individual annotators identifying objects in images or video frames during image segmentation in image processing. These annotators comb through hundreds of thousands of images hoping to construct comprehensive, quality AI training data.
That is how machine learning image processing looks like. Specific labeling techniques and types of image annotation are applied to the raw data depending on the needs of each image annotation project. These techniques include:
- Bounding box annotation tool. A rectangle is drawn around the object in the image allowing an AI to recognise/avoid it. This technique is more common due to its relative simplicity and is therefore more cost effective in real time.
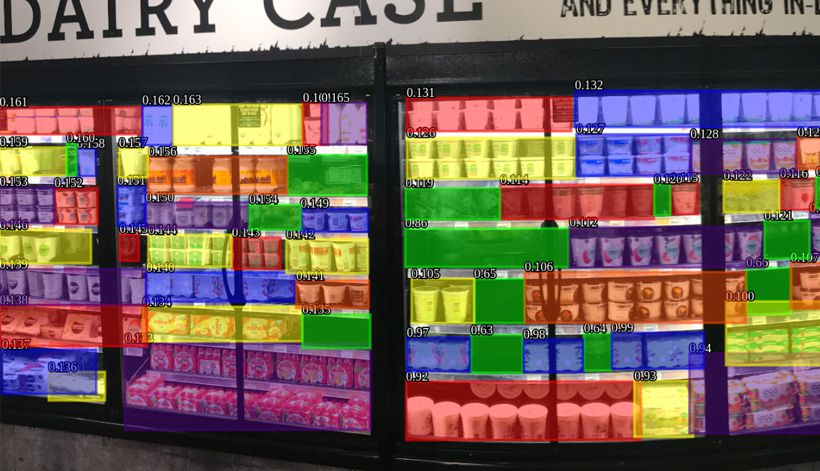
- Polygon annotation. In this case the annotator is required to plot vertices around an object in order to more accurately capture its shape.
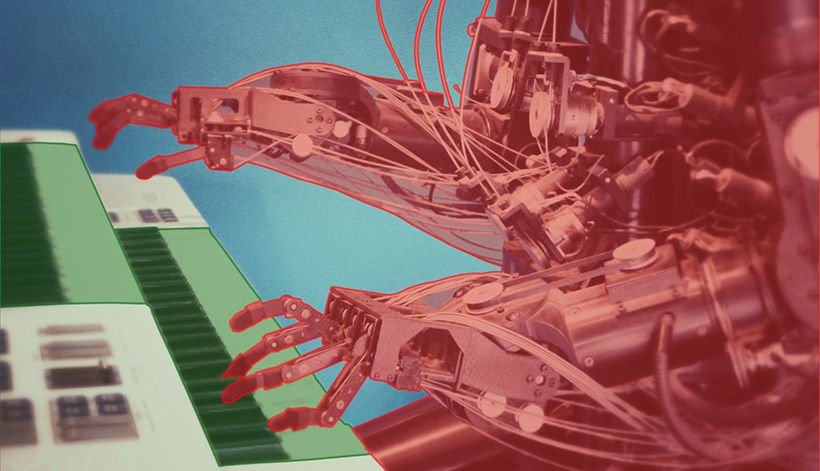
- Semantic segmentation. This is a technique used for grouping together objects in an image e.g. separating roads from buildings. This type of labeling is more precise and therefore more difficult.
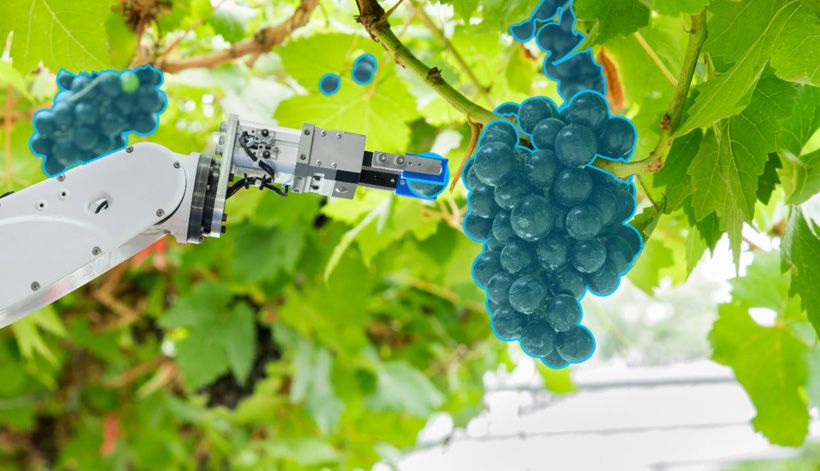
Manual data labeling has the potential to be somewhat labour intensive. Each instance of labeling may take seconds. The multiplicative effect of thousands of images could create a backlog and impede a project.
This is why many AI developers are opting to use professional data annotation services. For instance, Keymakr, to produce correct machine learning datasets.
A managed workforce of experienced annotators is able to scale manual data labeling to the demands of any project. Significant advancements have been made with automated labeling algorithms.
However, well-trained human annotators remain the go-to when it comes to precision and quality in training datasets. Manual labeling is able to capture the edge cases that automated systems continue to miss. Knowledgeable human managers are able to ensure quality across huge volumes of data.
Find the Right Data Labeling Tools for Your Project
Manual vs auto labeling is primarily a question of finding the right data labeling process for your machine learning project. The right labeling tools and a well-trained and professionally managed annotation workforce can be a powerful combination for today’s innovators.
Keymakr provides professional data labeling for machine learning services. That meets the need for pixel-perfect accuracy by utilizing proprietary annotation technology. Contact a team member to book your personalized demo today.
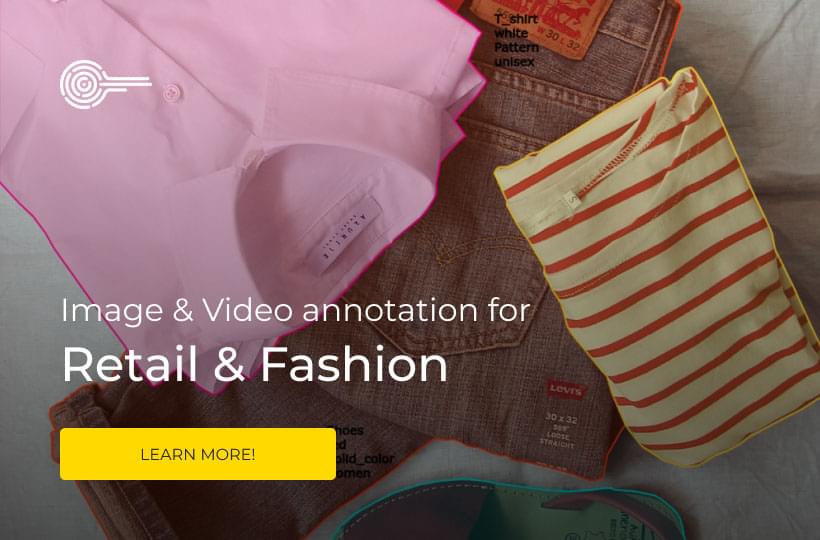