Polygon Image Annotation for Computer Vision
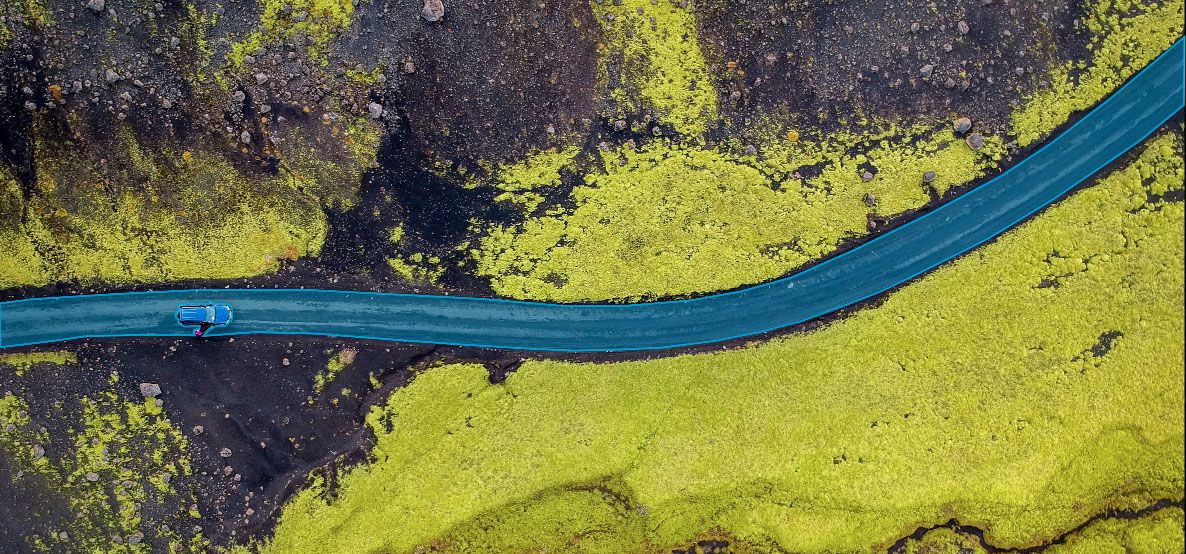
In the field of artificial intelligence, machine learning images and computer vision are becoming one of the hottest subfields at the moment. There are many applications of artificial intelligence and machine learning beyond the development of driverless cars and unmanned drones.
In the near future, computer vision and image annotation tools could change many fields. Among them are medical diagnosis and catching fraud in the retail or financial industries. What exactly is computer vision, and how does it work?
It all begins with the annotation of the image.
A computer needs training data in order to be able to perceive, process, and respond to its surroundings in a relevant manner. It is at this point that computer vision annotation tools come into play. These image annotation tools convert raw image data into data labeled images.
Then they will be used to train machine learning models. It could be a bounding box tool or a polygon annotation tool. But the purpose is common: high level image detection, high level image segmentation and finally convertion of the raw image data into data labeled images.
Computer vision annotation tools enable self-driving cars to detect and avoid people, traffic cones, and other vehicles on the road. Annotation tools are useful in many ways, but not all of them can be used to their full potential. In this article, we will discuss why polygon annotation is vitality neccessary for your next computer vision project.
How Does Polygon Annotation for Computer Vision AI Image Recognition Work?
Bounding boxes are quick and easy type of image annotation — but they fall short when it comes to target objects with irregular shapes. And when we’re talking about real time environments, irregular shapes are much more common than their regular counterparts.
Unlike bounding boxes, which are restricted to rectangles and squares, polygon annotation captures more lines and more angles. In practice, this means clicking at specific points to plot vertices. With polygon annotation, annotators have the freedom to change direction whenever necessary to best represent an object’s true shape. Image segmentation in image processing becomes easier.
Once an object is mapped with a polygon annotation tool, the annotator tags it with a descriptive label. This machine learning image processing is essential as it tells the machine what is found within the polygon annotation tool. If an image or video isn’t labeled properly, it often leads to inaccurate data.
Polygon Annotation in Action: Use Cases
Annotating data gives warehouse robots the ability to distinguish between different types of merchandise. At the same time data annotation gives delivery drones the ability to locate specific addresses.
Therefore, what function does the annotation of polygons serve to fulfill in the framework of computer vision? What is the main role of image processing techniques.
In the field of autonomous driving, semantic segmentation techniques of asymmetrical objects is essential.
- Image annotation projects using polygons is frequently employed in the process of semantic and instance segmentation. Bounding boxes are not very accurate for irregular shapes like people or bikes in a street scene. Polygons help self-driving cars identify road boundaries, walkways, and other features that may be difficult to see. This allows humans to provide guidance to the cars and helps improve safety.
When it comes to annotating data, precision is really necessary to ensure that your autonomous machine is functioning correctly. In many circumstances, the only option to guarantee pixel-perfect high level precision is to use datasets that include polygon segmentation algorithm into projects on image processing.
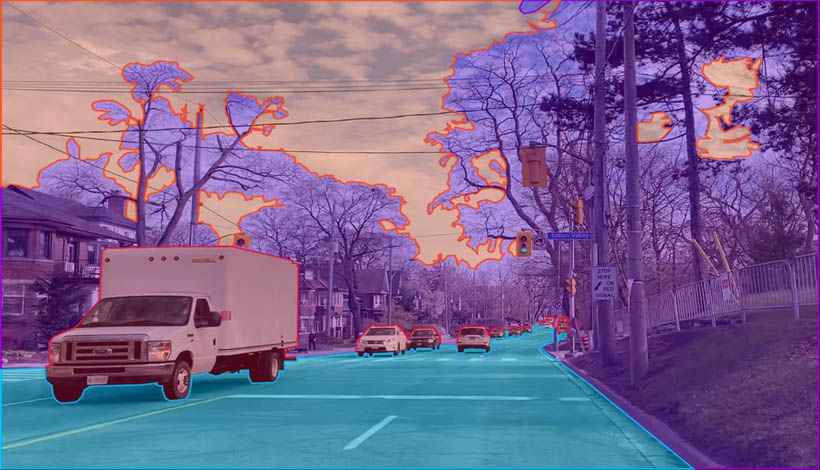
2. Drones and Satellites: Aerial Object Localization
Drones and satellites must recognize irregular shapes from above, from rooftops and chimneys to trees and swimming pools.
Aerial view imagery relies on contours. High level precision becomes extra important. That’s where polygon annotation tool comes in.
Object detection | Keymakr
3. Agriculture: Using Computer Vision to Detect Patterns
Annotating polygons enables computer vision to be used in its many different applications. Polygonal shapes can be found virtually everywhere; but, in order to capture them accurately, the suitable tool must be used.
In the field of agriculture, the use of polygon annotation is a useful tool for identifying essential features. Included in this category are crop rows, tracking the positions of insect legs. At the same time there are various other features that cannot be captured using bounding boxes.
It is important to have the right tools and trained staff when annotating polygons for computer vision. This will ensure that your training datasets are of good quality. Annotation tools for polygons ought to offer functions such as zooming and panning, in addition to the capacity to add remarks.
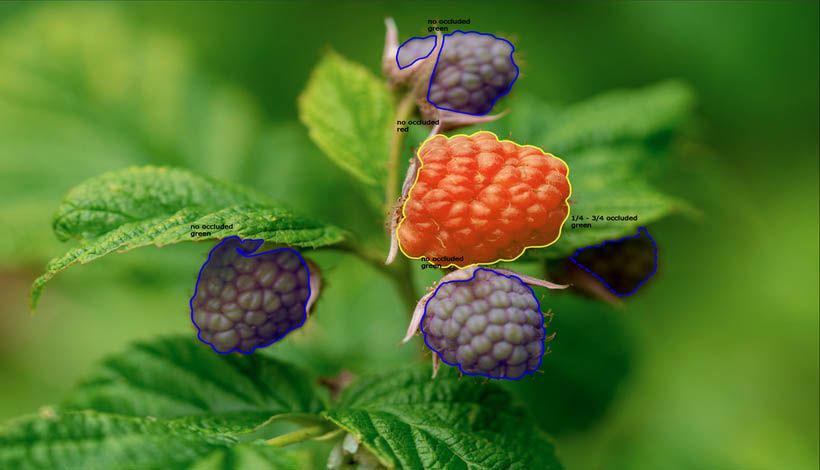
Professional Data Image and Video Annotation Services for Computer Vision Projects
Even though self-driving cars, medical imaging, and face recognition all have to do with computer vision, they are all very different. It’s not surprising that each of these machine learning models require different data annotation techniques.
Computer vision has limitless potential. But the performance of your AI hinges on its training data. Make sure your training datasets are up to par by partnering with a professional data annotation company that specializes in machine learning.
Keymakr offers affordable image- and video-based training datasets created by our team of expert data annotators.
Do you want to increase the quality of the datasets that you use to train your algorithms? Contact a member of our team now to book your unique demo.
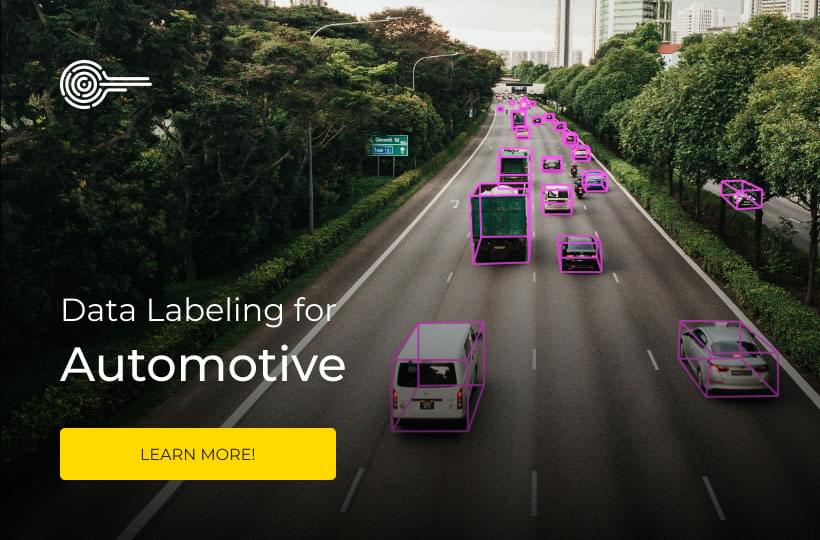