Intellectual Property Considerations in Data Annotation
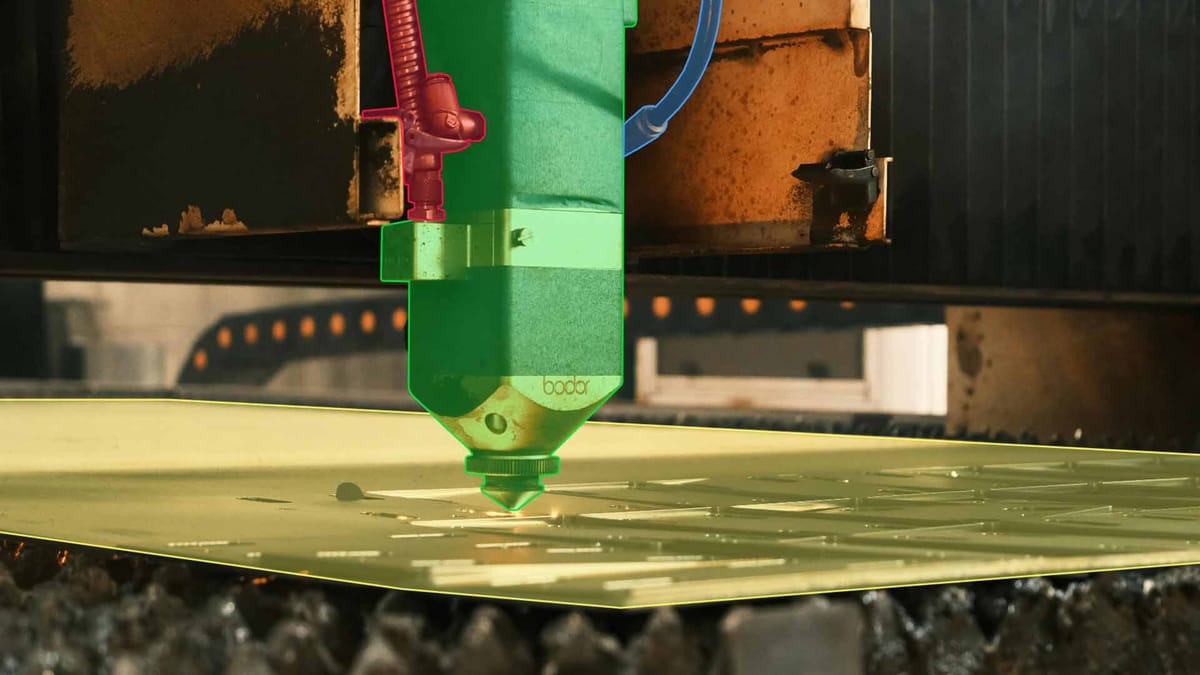
Intellectual property (IP) issues in data annotation are essential to address, especially as annotated datasets become central to the development of artificial intelligence systems. The first issue is who owns the original data. Whether it's text, images, video, or audio, this data often comes from sources with copyright protection or restrictions on use. Just because the data is publicly available does not mean that it can be used for free.
The annotations themselves, especially if they involve expert evaluation or a unique labeling structure, add value and can be seen as a new level of intellectual property. This can be the company that commissioned the work, the annotators who did the labeling, or the platform used to manage the process. Ideally, this should be made clear in the contracts from the outset. Without proper agreements, disputes can arise over how the data can be used, shared, or monetized.
Key Takeaways
- Data annotation is critical for building accurate machine-learning models but also requires strong IP protection.
- Understanding different types of IP, such as trademarks and patents, is essential for safeguarding your brand's assets.
- Legal complexities in the digital age require businesses to adopt robust IP strategies for data annotation.
- Advanced AI training data solutions can help maintain a competitive edge while ensuring IP protection.

The Role of IP in Data Annotation
The raw data itself may be protected by copyright or subject to license agreements, and its use without proper authorization may violate the rights of the original data owner. Companies should ensure they have the legal right to access and modify this data for annotation purposes.
After adding annotations, the resulting dataset may have its level of intellectual value. This raises the question of who owns the rights to the annotated version. Labeling, especially involving human judgment or expert knowledge, can create a new intellectual asset. In many cases, the rights to this asset are defined by agreements between the parties involved: the data owner, the company that funds the annotation work, and the individuals or companies that perform the labeling. Clear contracts are essential to define ownership and use rights, helping to avoid future disputes over how annotated data can be used or commercialized.
In addition to ownership, IP also plays a vital role in how annotated datasets are shared or licensed. Some datasets are made public for research, while others remain confidential for competitive reasons. Annotated datasets can be licensed, like software or other creative works, with terms and conditions that define how others can use, modify, or distribute them.
Why Effective Labeling Protects Your Assets
Adequate labeling protects your assets by transforming raw data into a structured, valuable resource that can be used securely and legally in machine learning and AI development. Without clear and accurate annotations, data lacks the precision needed to train high-performance models. More importantly, consistent labeling practices help establish an annotated dataset as a unique intellectual property asset. When labeling is done carefully, using well-documented methods and standards, it becomes easier to prove the originality and value of the dataset, which strengthens legal claims over its ownership and use.
Proper labeling also reduces the risk of legal and ethical problems arising from unclear data provenance or sloppy annotation methods. For example, if labels are applied inconsistently or without a consistent taxonomy, the dataset may be questioned regarding its validity, especially in regulated industries such as healthcare or finance. In more serious cases, improper annotation practices can violate someone's intellectual property or data use agreements.
Navigating IP Ownership and Confidentiality in Data Annotation
At the heart of the problem is determining who owns the various components of the annotation process: the source data, the annotations themselves, and the final labeled dataset. In many cases, the data may be owned by one party, the labeling work outsourced to another, and the final product used by another. Without explicit agreements, this can lead to confusion about who owns the rights to use, distribute, or profit from the annotated data. Contracts should define ownership of raw and annotated data from the outset, including work-for-hire or joint ownership provisions where applicable.
Confidentiality adds another layer of complexity, especially regarding sensitive or confidential data. Companies often rely on third-party annotators or crowdsourcing platforms to process data, meaning information may be exposed outside internal teams. Confidentiality agreements and strict data handling protocols are essential to protect trade secrets, personal data, or competitive knowledge. Annotators should only have access to the minimum amount of data required, and systems should be in place to prevent unauthorized sharing or reuse. For datasets containing personally identifiable information, legal compliance with privacy laws such as GDPR or HIPAA is also key to maintaining confidentiality and trust.
In addition to legal safeguards, companies must also think strategically about how intellectual property and privacy affect the long-term use of data. If ownership is unknown or data has been inappropriately disclosed, the dataset may lose value or become unusable for future projects. This not only affects compliance but also undermines the potential for licensing or reuse of the data at a later date. Establishing transparent workflows, documenting the entire annotation process, and creating a robust contractual framework are all part of ensuring that IP ownership and confidentiality are maintained. These steps help companies fully capture the value of their data while minimizing legal and operational risks.
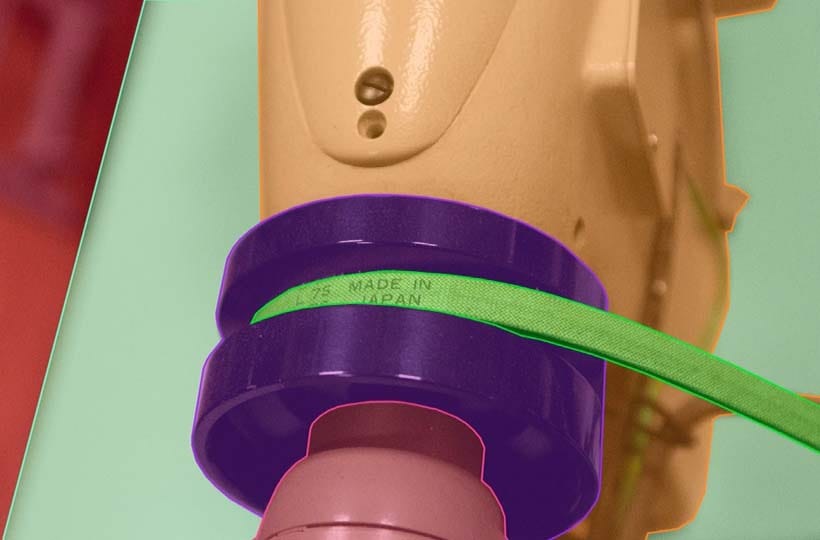
Key Clauses for Ownership Agreements
- Data ownership clause: This clause defines who owns both the original data and the annotated version. It should clarify whether the annotated dataset is a derivative work or a shared asset.
- Work for hire or transfer clause: When external contractors annotate data, this clause ensures that any intellectual property created belongs to the hiring company. Specifying whether the annotation work is considered work for hire or the rights must be formally assigned is essential.
- Confidentiality and Data Use Clause: This clause describes how data can be accessed, processed, and stored, ensuring the security of confidential information. It restricts the unauthorized use or dissemination of data and defines the conditions under which it can be shared. Privacy clauses protect sensitive data and ensure compliance with privacy laws.
- Rights and restrictions on use: This clause sets out the conditions for using, modifying, or licensing annotated data in the future. It helps to clarify whether the data can be shared, sublicensed, or used for other projects.
Quality Control in Annotation Processes
Given that machine learning models rely heavily on annotated data for training, the quality of this data directly affects the models' performance and efficiency. One of the first steps in implementing quality control is establishing clear guidelines and standards for the annotation task. These guidelines should define how to apply each label, the criteria for each category, and the overall structure of the data.
Another important aspect of quality control is regular monitoring and verification of annotations. This can be done through periodic spot checks or multiple annotators to annotate the same data and compare their results. Discrepancies can be flagged, and guidelines can be adjusted if necessary to ensure all annotators align with the same goals. Tools and software can also assist in this process by providing real-time feedback or using automated proofing checks to detect errors early. For example, machine learning models can identify potential mislabels by comparing annotated data to pre-existing models or datasets.
Annotators should receive constructive feedback on their work to improve accuracy over time. Regular communication between annotators and project managers can resolve any problems or ambiguities in the instructions. In addition, rewarding high-quality work and providing opportunities for retraining annotators can further improve the overall quality of annotations.
Monitoring, Compliance, and Strategic Legal Guidance
Monitoring, compliance, and strategic legal guidance are key elements of managing the risks and responsibilities associated with data annotation, especially in industries where intellectual property and data security are critical. Effective monitoring ensures that the entire annotation process complies with both internal standards and external regulations. This can include tracking the effectiveness of annotators, verifying that annotations follow guidelines, and identifying any inconsistencies or errors in the dataset at an early stage. Tools to automate this process, such as machine learning validation or quality assurance software, can significantly increase efficiency and help identify issues that might go unnoticed.
Compliance with data privacy laws and intellectual property rules is another important aspect of the annotation process. Compliance also extends to ensuring that the data used for annotations is lawfully obtained, has appropriate use permissions, and that the annotations themselves do not infringe on existing intellectual property rights.
Lawyers with experience in intellectual property, data privacy, and contract law can provide essential advice on drafting contracts that define ownership and use rights, entering into confidentiality agreements, and mitigating potential legal risks. They can also assist in negotiating third-party contracts with data providers or annotation vendors, ensuring that all parties understand their responsibilities and rights. Legal guidance is essential when entering new markets or scaling up data annotation operations, as laws and regulations can vary significantly from region to region. By involving legal experts at every stage, companies can better protect their assets and avoid costly legal challenges in the future.
Developing Effective Compliance Mechanisms
Developing effective compliance mechanisms ensures data annotation processes meet legal, ethical, and industry standards. A well-designed compliance framework helps companies mitigate data privacy, intellectual property, and regulatory risks. The first step in developing a compliance framework is clearly defining the legal requirements for the specific data being used.
Once the legal framework is in place, the next step is implementing policies and procedures that ensure data annotations comply with these regulations. This includes creating comprehensive guidelines for data processing and security and privacy measures.
Companies should implement ongoing monitoring mechanisms, such as regular audits or periodic reviews of their data processing and annotation practices, to ensure that they continue to meet legal and ethical standards. Automated tools can flag potential breaches or violations in real time, such as unauthorized access to data or inconsistencies in annotations that may violate data use agreements.
Summary
Intellectual property (IP) considerations in data annotation are crucial for protecting the ownership and use of raw and annotated datasets. The main objective is to ensure that the raw data is legally available and that its use for annotation does not violate copyright or license agreements. Once the annotations are made, questions of ownership arise, especially when external parties are involved in the labeling process. Clear contracts are needed to define who owns the annotated data and how it can be used, shared, or commercialized. In addition, intellectual property protection extends to ensuring that confidential information and proprietary labeling methods are protected throughout the process, reducing legal risks and preserving the data's value for future use.
FAQ
Who owns the data after it's been annotated?
Typically, the data owner retains ownership. However, contractual agreements should clearly outline this to avoid disputes and clarify usage rights.
What are the leading intellectual property concerns in data annotation?
The main concerns in data annotation involve determining ownership of both the original and annotated datasets.
How to protect annotated IP data?
Companies can protect the IP of annotated data by ensuring they have the proper licenses or ownership of the raw data used for annotation.
What role do contracts play in data annotation from an IP perspective?
Contracts define the rights and responsibilities of all parties involved in the annotation process, including ownership, confidentiality, and usage rights. Well-crafted contracts help clarify the scope of use and protect against the unauthorized reuse or distribution of the data.
Why is it essential to address IP concerns in data annotation at the beginning of a project?
Addressing IP concerns at the start of a project ensures that ownership and usage rights are clear, preventing conflicts later on.
What quality control measures ensure consistent data annotation?
Implement clear guidelines, conduct regular audits, and use experienced reviewers to maintain consistency and quality. This will help uphold your brand's standards and messaging.
Why is IP protection important in data annotation for AI training?
Protecting IP prevents unauthorized use and potential breaches. It safeguards your innovations and ensures the integrity of your data, which is vital for accurate AI model training and maintaining trust in your brand.
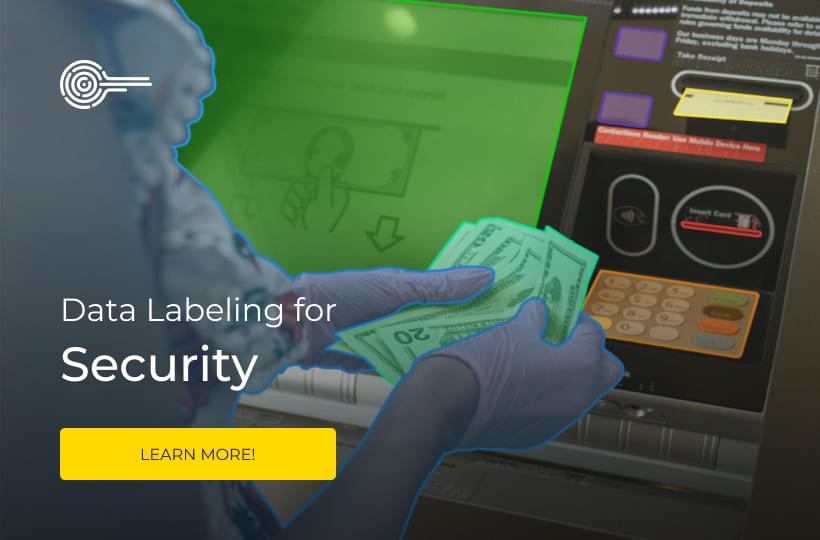