Precision Medical Image Annotation: Ensuring Accurate Diagnostic Models
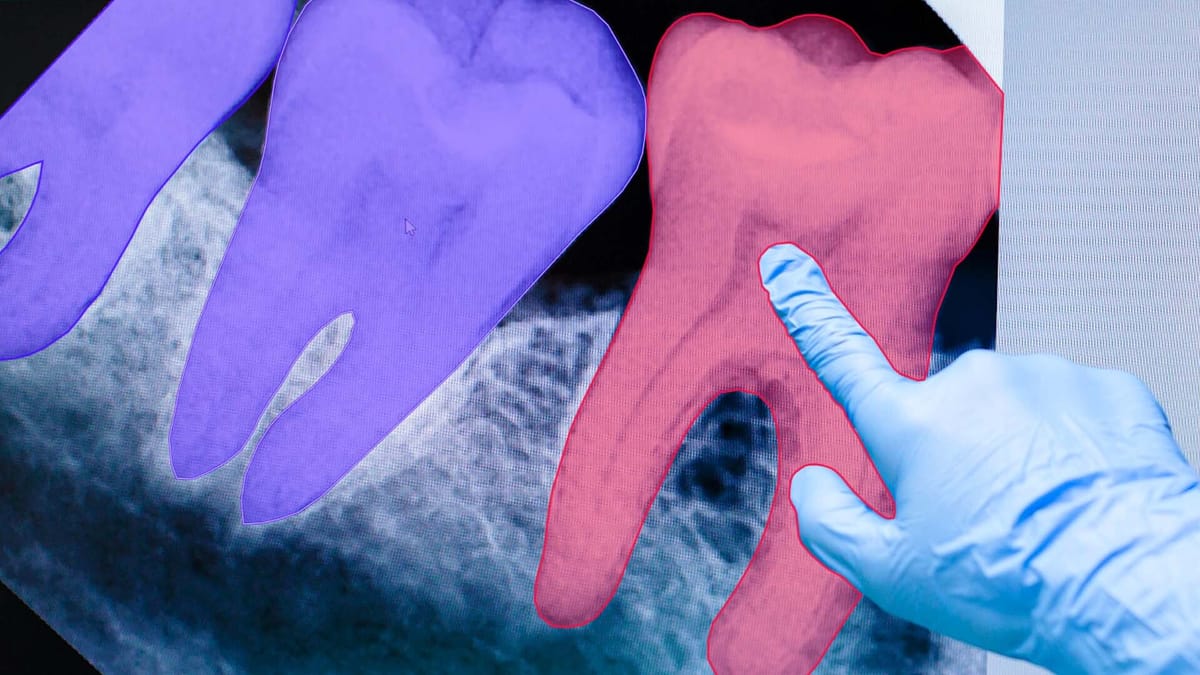
Artificial intelligence is now a leader in the development of healthcare technologies. With its help, medicine is developing very rapidly. Machine learning significantly increases the accuracy of diagnostics and automates most processes, optimizing human workflows.
Key Takeaways
- Medical image annotation is vital for precise diagnostics in healthcare.
- Detailed annotation enhances the accuracy of AI-driven diagnostics models.
- Advanced technologies and expert collaboration ensure high-quality datasets.
- NLP significantly contributes to tasks like Brain Tumor Detection and Liver Segmentation.

Introduction to Medical Image Labeling
These technologies are of great importance in the future. Still, it cannot be overestimated, as it directly affects the accuracy of medical conclusions with minimal risk of errors. The emergence of artificial intelligence technology helps radiologists and medical personnel accurately interpret diagnostic images and includes high-speed analysis and a virtually unlimited amount of knowledge.
Overview of Medical Imaging Techniques
MRI, CT, and X-ray provide essential information about health conditions and help plan treatment. The DICOM format is widely used for medical imaging, helping to manage complex data. Multi-layered TIF files are also used for microscopy, requiring continuous maintenance of data integrity in the format.
The precise medical imaging annotation process brings significant benefits, mainly when combined with radiology imaging.
CT Scans: Understanding Cross-Sectional Imaging
CT scan imaging uses X-rays and computers to create detailed cross-sections of the body. It's key for complex condition diagnosis, giving a full view of internal injuries, cancers, and heart diseases with high accuracy. For more context, you can learn how precise labeling boosts medical imaging AI's impact here.
Steps in Image Annotation
It all starts with collecting the necessary data (images, video frames, objects, etc). Then, this data is processed and prepared for the annotator to work with it. The last stage is verification, used to find and correct possible errors.
Quality Control Measures in Annotation
Stringent quality control in medical annotation ensures the reliability and accuracy of labeled data. It involves multiple verification levels and semi-automated annotation techniques, which are critical for AI model training and quality control in medical annotation.
Dealing with Noisy Data
An additional crucial issue in medical image annotation is handling noisy data. X-rays, MRIs, and CT scans are examples of medical imaging that frequently have artifacts, hazy regions, or extraneous information, which hides essential information. This noise can be eliminated with sophisticated algorithms and professional supervision, making annotations more accurate and precise.
Time and Resource Constraints
Medical image annotation requires a lot of time, resources, and specialized knowledge. To tackle these challenges, efficient resource management is essential in healthcare AI. Automation technologies and cloud platforms can significantly reduce costs and time while improving scalability.
Collaborative Approach in Annotation
Doctors concentrate on medical signs, such as tissue alterations or symptoms of diseases, while technical specialists ensure the quality of the images and the accuracy of the tools used for annotation. This combination of different skills leads to more precise outcomes. For instance, technical experts may spot details that are often overlooked. Every stage of this process requires focus and cooperation to achieve the best outcome. For instance, technical experts may spot details that are often overlooked. This process requires focus and cooperation to achieve the best result. Working together enhances annotation accuracy and builds a reliable foundation for medical decision-making.
Metrics for Measuring Accuracy
For models to work well, the annotations need to be accurate. We pay attention to a few key things to ensure everything is on track. First, we measure how accurately the elements in the images are annotated. But it's not just about accuracy; it's also about speed, so we also track the time spent on each stage.
There's also a system of checks that helps us detect any errors or inconsistencies and adds a level of confidence that the annotations are true as required. Automated tools speed up the process while still maintaining a high standard of quality. All these things together help ensure that our model training system works efficiently, enabling us to build precise and reliable models ready for real-world use.
Feedback Mechanisms for Continuous Improvement
When it involves optimizing algorithms, one of the key factors of fulfillment is non-stop remarks all through the process. In fields in which precision and accuracy are crucial, remarks perform a vital position in refining fashions and enhancing their performance. This is especially authentic in scientific photo annotation, For instance, in photo annotation, specialists or scientific experts might also additionally observe regions in which commands want to be extra distinctive or in which extra specs are required to appropriately label a photo. By addressing those points, annotation accuracy may be improved. Furthermore, by incorporating remarks at every degree of development, groups can reply quickly to troubles and solve them earlier than they amplify into large problems.
Ultimately, without regular checks and adjustments, feedback would be just another tool, but in fact, it becomes an essential part of the process. This, in turn, leads to more accurate diagnoses and, overall, a higher standard of healthcare services.
Ultimately, without regular checks and adjustments, feedback would be just another tool, but in fact, it becomes an essential part of the process. This, in turn, leads to more accurate diagnoses and, overall, a higher standard of healthcare services.
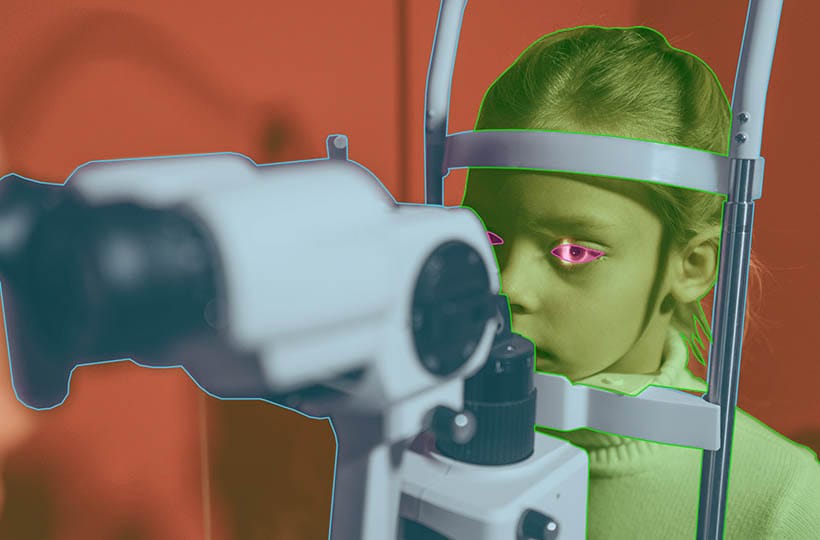
Emerging Trends in Medical Image Annotation
There are some cool new trends in medical image annotation that are changing the way we develop diagnostic models. For example, augmented reality is becoming more common. Also, cloud-based tools are now being used more to work with data. This makes it faster for medical professionals to collaborate, manage their time, and work more efficiently.
Use of Augmented Reality
New augmented reality technology offers medical professionals interesting opportunities to work with medical images. It allows doctors to see additional information as they work, which helps them to more accurately mark important details in images. For example, during operations, augmented reality allows doctors to better navigate in space, which reduces the risk of errors and improves overall treatment outcomes. Augmented reality assists in precisely identifying anatomical structures and is very useful for radiologists and clinicians. It plays a significant role in enhancing innovation in medical imaging and, thus, a new level in image analysis.
Advances in AI and Automation
Artificial intelligence in medicine is largely driven by deep learning, namely by neural networks, in particular convolutional networks (CNNs). Networks such as AlexNet, VGGNet, and ResNet allow for efficient learning from large amounts of data, which significantly improves disease diagnosis, image segmentation, and quantitative image analysis. These methods allow for efficient work with different types of data, both labeled and unlabeled, which helps to solve the problem of limited access to resources for model training.
One important trend is to make artificial intelligence models more understandable to users. This is of great importance as doctors and other medical professionals need to trust the results of such systems. Researchers are actively working to improve these aspects and ensure the accuracy and efficiency of models used in medicine.
Potential for Real-Time Annotation
Doctors can analyze visual data and work with additional information available directly during the procedure. This allows new solutions to be adapted to the clinic's actual needs. In addition, modern cloud technologies make these processes even more efficient by providing tools for storing large amounts of data and fast processing.
Case Studies on Successful Annotation Projects
Artificial intelligence in medicine has already become a reality, confirmed by numerous successes in clinical practice. These achievements demonstrate how carefully thought-out approaches to data annotation contribute to improved diagnosis and positively impact treatment outcomes.
Summary
Medical image annotation is key to reducing diagnostic errors and responding to a problem faster. Such images are used to train machine learning algorithms that help to recognize and predict various objects, which is essential for detection and classification in the medical field. The accuracy of the training data significantly affects the efficiency of the machine and deep learning models.
Automation and techniques such as transfer learning and synthetic data creation have greatly facilitated the process, reducing the time for manual annotation and increasing data accuracy. Infosys BPM combines human expertise with intelligent technology to deliver fast, high-quality training data.
FAQ
What is medical image annotation, and why is it important?
Medical image annotation labels and segments images from MRI, CT scans, and X-rays. It's key for creating precise AI models in healthcare. This boosts diagnostic accuracy and aids in treatment planning.
How does image annotation improve AI-driven diagnostics in healthcare?
Accurate image annotation feeds detailed data to AI models, which can read complex images. These models help detect diseases, automate diagnoses, and improve clinical decisions.
What are the main types of medical imaging techniques?
Main imaging strategies consist of X-rays for bones, MRI for smooth tissues, and CT scans for frame structures. Each gives precise insights into the frame's inner workings.
What are the steps involved in the medical image labeling process?
The process starts with selecting images and then annotating them accurately. Quality control is rigorous.
Why is quality control important in medical image annotation?
Quality control ensures label reliability, which is vital for AI diagnostic tools. Verification at multiple levels maintains high standards.
What challenges does medical image annotation face?
Challenges include variability in interpretation, noisy data, and the time-consuming nature of annotation. Advanced strategies and tools are needed to overcome these hurdles.
How are artificial intelligence and deep learning techniques used in medical image annotation?
AI and deep learning improve annotation accuracy and efficiency. They automate tasks and find patterns in large datasets, making these technologies essential for developing AI diagnostic tools.
What is the role of radiologists and data scientists in image annotation?
Radiologists offer insights into anatomy and pathology. Data scientists refine annotation tools and algorithms.
How is annotation quality evaluated in medical imaging?
Quality is evaluated through accuracy metrics, validation, and feedback. Regular updates ensure annotation practices stay current with medical and technological advancements.
What are the emerging trends in medical image annotation?
Trends include augmented reality for better visualization and cloud-based solutions for scalable annotation.
What are the future directions in medical image labeling?
Future directions include AI and automation for faster, more accurate annotation. Real-time annotation could provide immediate diagnostic insights during medical procedures.
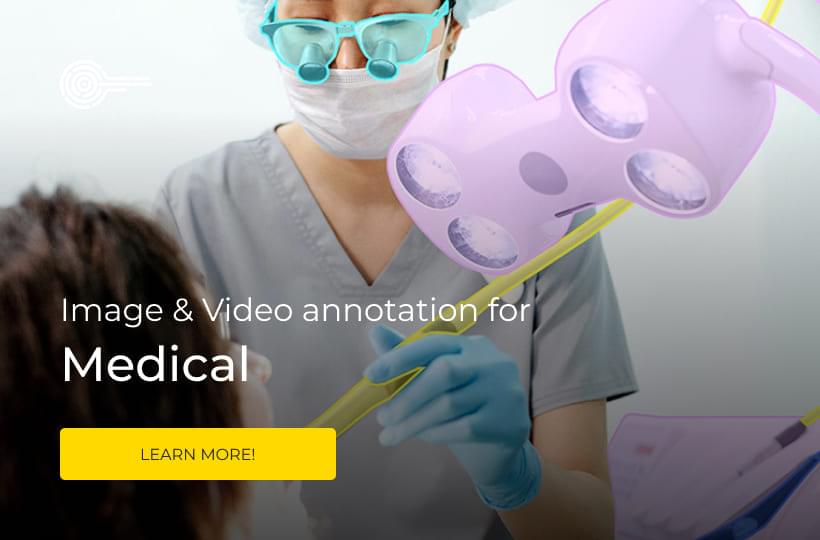