Medical Imaging Annotation: A Guide for Precision and Care
Today, millions of images are used in AI for precise diagnosis. These images are stored digitally but need careful labeling. The goal is to help healthcare AI work better. As a doctor or medical professional, you're at the forefront of using these new technologies. You know that tools for analyzing healthcare images are more than gadgets. They are bridges to achieve better results for patients, thanks to insights from machine learning.
The healthcare field is flooded with data from various devices and apps. But turning this data into a diagnosis requires precise annotation. The quality of the software used for labeling and the tool's skill to pick out details in each scan are crucial. The more detailed the annotations, the more accurate the AI models become. By creating large and diverse datasets, the medical field aims to push AI to new levels. This effort is key for better clinical diagnoses and research.
Following strict rules such as HIPAA, FDA, and CE guidelines is a must in this field. These rules make sure AI in healthcare is advanced and ethical. They align with the main goal of precise healthcare. So, maintaining high annotation standards is not just important but required by law.
Key Takeaways
- Medical image datasets need large-scale, diverse images for effective AI modeling.
- Annotation in medical imaging with help, such as from Keymakr, enhances AI accuracy for diagnostics and treatment planning.
- Medical image labeling software provides the necessary precision for early disease detection and research development.
- Adhering to privacy and regulatory standards is critical for the viability of AI tools in healthcare.
- Annotation quality directly influences the performance and reliability of healthcare AI applications.
- Choosing a thorough radiology image annotation tool is essential for addressing data privacy and domain-specific challenges.
- Annotated medical data plays a pivotal role in surgical accuracy and can significantly improve patient outcomes.

Understanding the Essentials of Medical Image Annotation and Labeling
AI in medical imaging is changing patient care. Having a strong base in medical image annotation and labeling is crucial. This boosts the performance of AI models and is key for success in real health settings.
The Definition and Purpose of Medical Image Annotation
Medical image annotation means adding info to pictures for computers to understand. This guide is vital for those who build AI and doctors. They need the detailed data to make algorithms spot diseases accurately.
Importance of Labeled Medical Data in AI Developments
Labeled images help diagnose and treat diseases better. A good annotation tool is key to make health AI better. It helps handle big datasets for smarter health plans.
With the right annotations, AI improves how we care for patients. AI learns to spot issues in images, making diagnoses more accurate. This leads to treatment that works faster.
Learning about medical image annotation is crucial for advancing AI in health. It's about making care better by using the latest tech and accurate data.
Effective Strategies for Medical Data Preparation
Working on image analysis for radiology shows how important preparing data sets is. Make sure your data is diverse and high quality. This is key for machine learning models in the medical imaging annotation tool to work well. A good dataset boosts model trustworthiness and avoids outcome biases.
Start by getting data from many places. This helps algorithms work in different radiology cases. Also, carefully check for errors to keep data quality high.
Data should be divided into training, validation, and testing sets properly. Sticking with an 80-10-10 split for training, validation, and testing, respectively, is a good rule of thumb. This setup leads to better machine learning model testing and refining as you go.
Having high-quality data is more crucial than having a lot of data. A smaller, well-kept dataset is often more useful than a big, messy one. So, pay close attention to the correctness of every data point.
Here's a quick look at important steps for getting your medical data ready:
Data Aspect | Description |
---|---|
Variety in Data Sources | Get data from many medical sites to prevent bias. |
Data Quality Assurance | Check data carefully for mistakes and consistency problems. |
Dataset Distribution | Use 80% for training, then split the rest for validation and testing. |
When dealing with medical images, think about formats like DICOM and TIFF. DICOM stands out for radiology image analysis. It's crucial that your medical imaging annotation tools work well with this format to keep things running smoothly.
Dealing with medical imaging data is complex and critical. It calls for special tools and know-how. Pick an annotation tool that handles DICOM and TIFF well, and understands the depth of medical data. This choice helps ensure the accuracy required to improve radiology diagnoses.
By using these methods, you make your training data better and AI in radiology more likely to succeed.
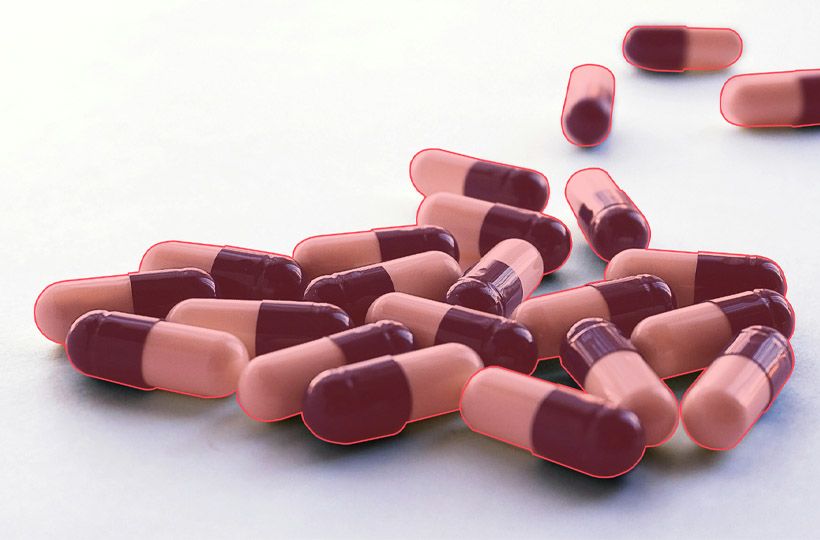
Differences in Data Availability and Types
Medical imaging tasks deal with very private and regulated data. This data is hard to get and can't be freely shared because of laws like HIPAA. On the other hand, general data is easier to access and has fewer restrictions.
The Technical Challenges Unique to Medical Images
Working with medical images is tough because they're more complex. They have more details and layers than images in other fields. This means we need advanced tools to read and use this data.
Special Requirements for Annotators' Expertise
Experts in medical image annotation need to know a lot about healthcare. They must understand complex medical terms and imaging standards. This knowledge helps make sure their work is accurate, which is crucial for patient care.
Insights into Different Types of Labeling Tasks
Labeling tasks vary widely in medical imaging, covering simple classes to complex segmentations. AI in medical imaging must be precise and flexible for these tasks. They are key for ensuring data's accuracy and usefulness. Now, let's dive into the main types of labeling jobs.
Exploring Object Detection and Segmentation
Object detection and segmentation are crucial for analyzing medical images. They help find structures like tumors or organs in scans. Object detection pinpoints these structures. Segmentation breaks the image into parts, making it easier to understand, needed for tasks like finding brain tumors.
Applications of Classification and Landmark Detection
In medical imaging, classification tags images by what's visible, like a cancer type or disease stage. Landmark detection finds specific points, aiding in studying anatomy or spotting disease areas. AI makes these tasks more precise, benefiting diagnosis and treatment outcomes.
Understanding the Nuances of Semantic and Instance Segmentation
Semantic and instance segmentation add more depth to image analysis. Semantic segmentation assigns classes to each image pixel, helping in detailed tissue analysis. Instance segmentation, on the other hand, identifies distinct objects of the same type. This is crucial for complex cases, e.g., multiple tumors in one scan.
Task | Utility in Medical Imaging | Percentage Use in Research Studies |
---|---|---|
Brain Tumor Detection | Locates and identifies tumors within brain scans. | 4.08% |
Liver Segmentation | Facilitates detailed analysis of liver morphology and pathology. | 3.06% |
Breast Cancer Classification | Helps in classifying histological images of breast tissue to detect malignancy. | 1.22% |
Superficial Lesion Diagnosis | Used in the evaluation and diagnosis of skin lesions using specific imaging techniques. | 2.04% |
Enhancing these tasks with AI and powerful labeling tools boosts healthcare quality. It makes diagnoses more accurate and treatments more targeted. As AI advances, it will introduce even more medical imaging breakthroughs.
Tackling the Challenges in Medical Data Annotation
Medical science is moving forward quickly. This makes high-quality data annotation in medical imaging very important. Key challenges include privacy concerns and dealing with the complex nature of medical data. Overcoming these is crucial for supporting healthcare experts and enhancing patient care.
Protecting private patient information is a top issue in medical data annotation. Tools must remove personal details to keep data safe. This ensures that while medical records go digital, they are still protected. It's about using strong security to follow data laws.
Medical data often comes in complicated formats, like DICOM files. Here, advanced radiology image annotation tools play a critical role. They must understand these formats well to be effective. Having experts in radiology and pathology helps a lot. Their knowledge, combined with technology, boosts the quality of annotations for better diagnoses and treatments.
Feature | Importance | Challenges |
---|---|---|
Data Privacy | Essential for patient confidentiality | Requires strong anonymization and security measures |
Domain Expertise | Crucial for accuracy | Dependent on available expert radiologists and pathologists |
Data Format Compatibility | Necessary for effective analysis | Complexities in handling DICOM and other medical-specific formats |
Tool Robustness | Impacts efficacy of diagnostic assessments | Integration of advanced features for detailed image analysis for radiology |
Using advanced radiology image annotation tools helps address these issues. It's about improving healthcare delivery standards. By continually enhancing these tools, doctors can make better choices. This leads to better outcomes for patients and more streamlined healthcare.
Navigating the Selection of an Annotation Partner
Choosing the right annotation partner is as important as the tool. Look for a partner with a deep expertise in medical image work and strong checks for quality, such as Keymakr. It's important that your partner understands how to keep data safe and be able to handle various datasets.
This partnership should focus on precise diagnoses and follow tight health data rules. It goes beyond business; it's a commitment to medical accuracy and patient health.
Integrating Annotation Tools with AI Technology for Healthcare
Mixing annotation tools with AI in health care needs a deep understanding of what clinicians need. The perfect healthcare image analysis platform should boost diagnoses without messing up daily clinical tasks. It aims to connect technology with health services to better patient outcomes through quick, precise diagnoses thanks to advanced AI models.
Deploying these advanced tools needs careful planning. It’s critical that they meet both technical data safety standards like HIPAA and fit into the real-life medical work setting.
- Support for Medical Data Formats (DICOM, TIFF): Key for meeting the medical field's needs.
- Data Privacy Measures: Vital to follow HIPAA and other privacy standards to protect patient information from being seen by unauthorized people.
- Scalability and Flexibility: Must-have for managing the large and ever-growing amount of medical images healthcare groups produce.
Navigating Privacy Concerns and Compliance in Medical Annotation
In healthcare, it's vital to keep patient info private while accurately tagging medical images. The healthcare image analysis platform you pick should help mark images correctly. It should also follow strict privacy laws like HIPAA and GDPR. These laws say you must hide patient details to safeguard private info.
You need a solid medical imaging annotation tool to meet these needs. This tool must have security to keep data safe. It should follow rules in the healthcare field. By doing this, you make sure patient data remains confidential. Plus, you keep your medical studies and diagnoses trustworthy.
Let’s look at what your platform should have to follow these tough rules:
- Encryption methods to protect data when it's stored or moved.
- Advanced user checks to stop people from viewing info they shouldn't.
- Record all data checks and changes for review.
- Follow strict rules on where data can be stored.
Adding these steps to your tagging tool isn’t just to meet laws. It’s also to earn trust from patients and those who use your data. They know their details are safe with you.
Remember, the strength of your data protection measures can significantly influence the credibility and reliability of your medical research outcomes.
As more focus is put on accurate and ethical image tags, the need for a solid healthcare image analysis platform increases. Look for a tool that makes patient privacy a top concern. This way, your imaging work will improve patient care, all while keeping their info private.
The Evolution and Impact of AI for Medical Imaging
Introducing artificial intelligence (AI) into medical imaging has changed the game for healthcare. AI has enhanced the way we diagnose and treat patients. It has changed how we handle medical images and how we use them in making medical decisions.
Medical Diagnosis and Predictive Models Enhanced by AI
AI makes it quicker and more accurate to find and treat illnesses. It uses deep learning to give more detailed views of medical images. This detail helps AI predict health issues earlier. Thus, doctors can offer treatments that fit each patient's needs better, improving their health.
From Early Detection to Surgical Assistance: AI's Broadening Role
Now, AI doesn't stop at diagnosis. It aids in surgeries and aftercare too. With advanced imaging tools, AI can show real-time pictures during operations. This makes surgeries safer and more precise. It hints at a future where AI will be key at every step of patient care, from finding an issue to treating it and recovery.
What's more, AI speeds up the healthcare process. It cuts down wait times, making treatments start sooner. This helps lower overall healthcare costs. Hospitals using AI in imaging report smoother processes and better use of resources. This leads to more efficient operations.
Application | Technology Used | Impact |
---|---|---|
Automated Treatment Planning | Deep Learning | Enhanced Radiotherapy Precision |
Disease Predictive Modeling | Convolutional Neural Networks | Better Treatment Outcomes |
Surgical Assistance | Computer Vision | Increased Surgical Safety and Accuracy |
Medical Data Analytics | Big Data Technologies | Optimized Treatment Plans |
AI is making diagnostic and treatment procedures better. It's also improving how we take care of patients. The evolving AI technology highlights its pivotal role in transforming healthcare. Medical imaging annotation tools are crucial in this transformation.
Summary
In the world of healthcare, AI is key. It's really important for AI to work well in healthcare. This happens when medical images are labeled correctly. You need to be careful and follow privacy rules when working with this data. Picking the right tool for labeling radiology images is crucial. It should be able to handle complex information and meet strict rules.
Preparing medical images for AI is a big deal. It's like building the basis for AI to become smart and helpful. With the help of experts and a good guide, we can create AI that saves lives. Every tiny detail matters, from marking cancer cells to finding problems in ultrasound images.
Your work in medical image labeling is part of a big dream. This dream is to use AI to make healthcare better, from X-rays to operations. By using labeled medical data, we can make big steps forward. It's not just about getting better results. It’s about creating a more precise healthcare future. This journey is all about discovering new ways to help the world stay healthy, using AI and our own intelligence.
FAQ
What is medical image annotation and what are its purposes?
Adding details to medical images is medical image annotation. It helps computers understand the images better. This is key for teaching AI in health, mainly for diagnosis and planning treatments.
Why is labeled medical data important for AI developments?
Labeled data is the foundation to train AI well. It's critical in research and real-world medical use. With this data, AI can help doctors spot diseases and choose treatments.
How do you effectively prepare medical data for annotation?
To prepare data, make sure it's varied and of good quality. Size and type of data sets are important too. Split data for training, validation, and testing. Quality is crucial for reliable AI.
What are the key differences between annotating medical images and general images?
Annotations for medical images need more technical know-how. This is because medical images are bigger and may need more detailed explanations. Annotators also need a background in healthcare.
What are some of the specific labeling tasks in healthcare imaging?
Tasks include object detection, classification, and segmentation. Detection and classification put labels on what’s in the image. Segmentation goes further by tagging every part of the image or finding specific objects.
What challenges are involved in medical data annotation?
Keeping patient data private is a big issue. Annotators need to know about medicine for accurate labeling. Also, dealing with medical formats like DICOM can be tricky.
What features should one look for in medical imaging annotation tools?
Look for tools that work with DICOM files and are easy to use. They should suit your AI needs and fit smoothly into your hospital's digital setup.
How do privacy concerns affect medical image annotation?
Annotation must follow strict privacy laws like HIPAA and GDPR. This means making the data anonymous without losing its meaning. Tools must keep data safe from breaches.
What is the role of AI in medical imaging?
AI impacts medical imaging from start to finish, making it faster and more accurate. It's used not only for diagnosis but also for guiding surgeries through computer vision.
What considerations should be made when choosing between open-source and proprietary annotation tools?
Deciding between tools involves looking at their features and ease of use. Open-source offers flexibility with community help. Proprietary may have unique tools and good support.
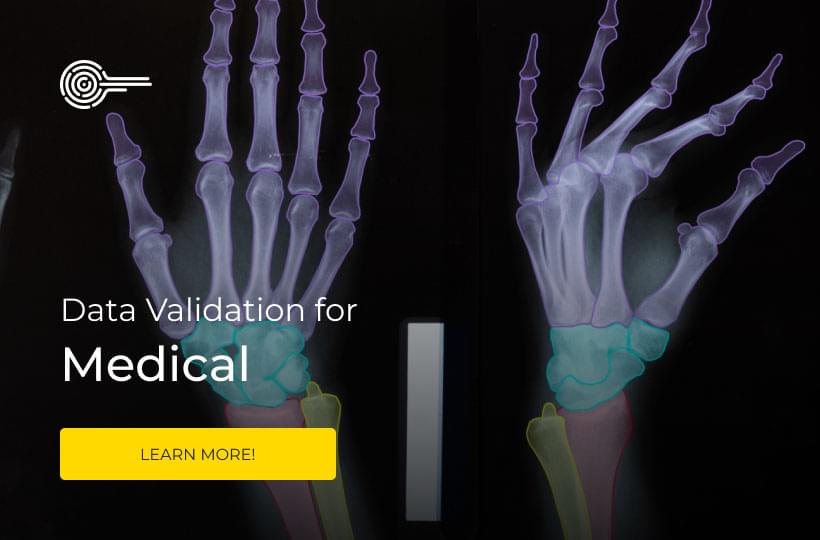