Personalization Powerhouse: Leveraging AI and Data Training for Product Recommendations
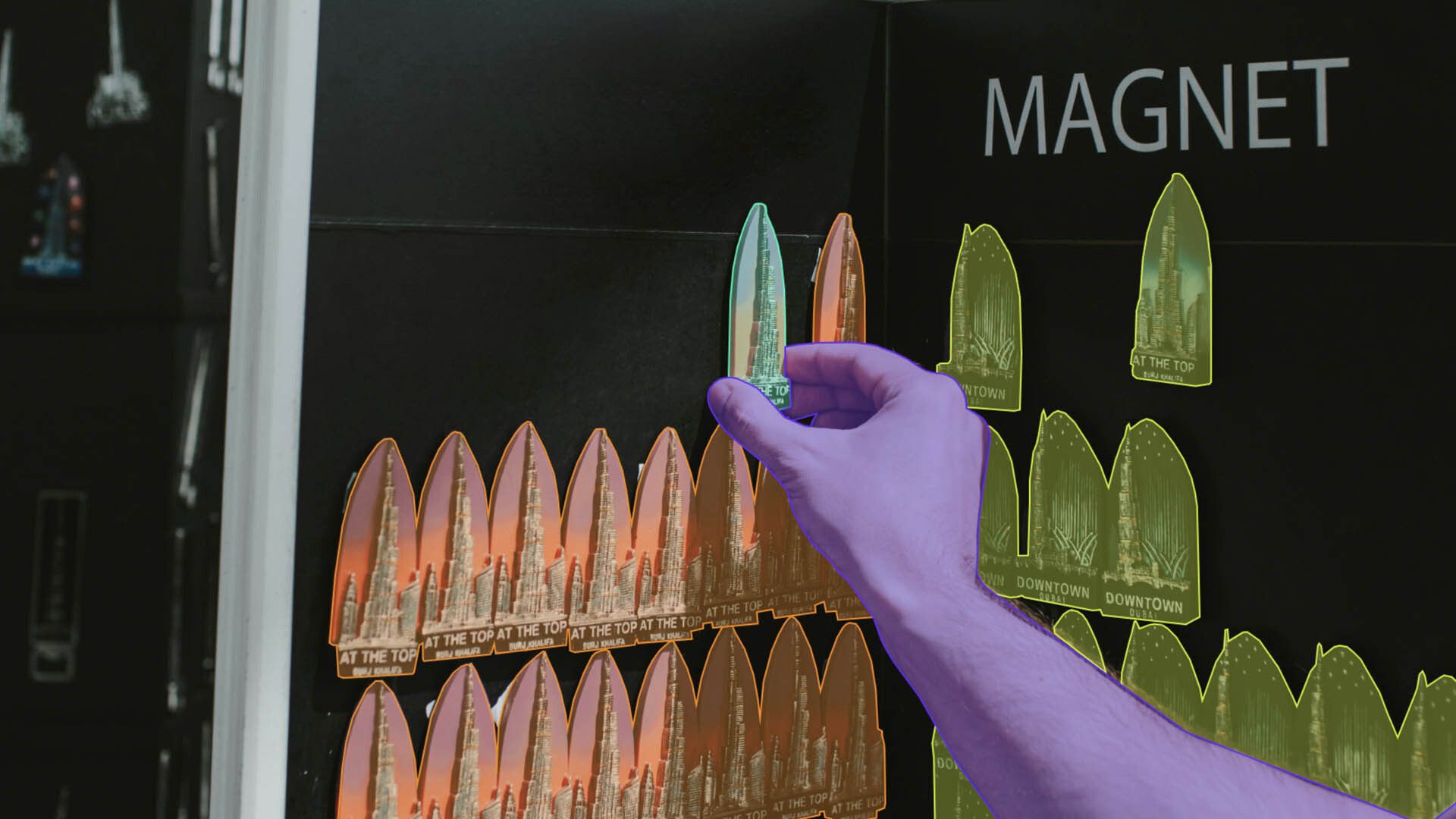
91% of consumers are more likely to shop with brands that provide personalized recommendations. This statistic highlights the critical role of AI for product recommendations in today's e-commerce world for more data-driven enterprises. As we move into 2024, AI is transforming industries and pushing businesses forward at an unprecedented rate.
AI-powered websites lead this revolution, offering dynamic content, smart recommendations, and adaptive interfaces. These personalized experiences are not just nice-to-have features; they're key drivers of increased engagement, higher conversions, and improved customer satisfaction.
By analyzing vast amounts of customer data, AI can offer relevant product suggestions that streamline your shopping experience. This level of personalization not only makes shopping easier but also strengthens brand loyalty, trust, and your value as a customer over time.
Key Takeaways
- 91% of consumers prefer brands offering personalized recommendations
- AI-powered websites deliver dynamic, tailored content
- Personalized experiences increase engagement and conversions
- AI analyzes customer data for relevant product suggestions
- E-commerce personalization builds brand loyalty and trust

Understanding Personalized User Experiences
Today's consumers demand relevant interactions. AI responds by tailoring experiences for each user. A remarkable 82% of companies now leverage AI for personalization to boost online customer experiences. This strategy enhances satisfaction and fosters loyalty.
AI's Role in Transforming Online Shopping
AI is transforming online shopping with intelligent recommendations and customized search results. Machine learning in customer data analysis underpins these features, making the shopping journey more engaging. Interestingly, 67% of first-time buyers rely on AI for product recommendations when deciding to purchase.
Benefits for Businesses and Customers
AI-driven personalization brings numerous benefits:
- Increased customer satisfaction
- Boosted sales
- Improved customer loyalty
- Reduced cart abandonment
For businesses, AI simplifies operations and boosts efficiency. Customers benefit from a more personalized shopping experience, with 78% more likely to return when they encounter tailored website content. This integration of AI technology and e-commerce is defining the future of online retail.
Fundamentals of AI for Product Recommendations
AI algorithms transform product recommendations by delving into customer data and behavior patterns. They gather insights from numerous touchpoints, such as past purchases, browsing history, and demographic information. Through sophisticated customer data analysis, AI segments customers dynamically and uses predictive analytics to elevate lifetime value.
For effective operation, recommendation systems need large datasets. AI-driven personalization typically demands hundreds or thousands of items and at least 30,000 users with interaction data. This setup ensures precise predictions and pertinent suggestions.
AI algorithms employ various techniques to produce recommendations:
- Collaborative filtering suggests products based on similar user profiles
- Content-based algorithms focus on item attributes and user interests
- Hybrid models combine both approaches for improved accuracy
- Matrix factorization breaks down user-item interactions into latent factors
These strategies allow AI to adapt recommendations in real-time as customers browse online stores. For businesses with limited historical data, fallback models ensure consistent recommendations across different widgets.
"AI-powered recommendations lead to higher conversion rates and larger average order values."
The impact of AI on e-commerce is profound. Companies leveraging AI for product recommendations see a rise in conversions and revenue.
Data Collection and Preprocessing for AI Models
Effective data training for personalization begins with collecting and preprocessing customer data. This step is crucial for the success of AI-driven product recommendations.
Types of Data Used in Recommendation Systems
Recommendation systems use a variety of data types for personalized experiences. These include:
- Past purchase history
- Browsing behavior
- Product views
- Demographic details
Customer Data Platforms (CDPs) provide numerous attributes for AI algorithms to identify user segments. These segments change in real-time, reflecting shifts in customer behaviors and preferences.
Techniques for Data Cleaning and Normalization
Data preprocessing is essential for preparing raw data for AI algorithms. It often makes up to 80% of the total effort in implementing AI systems. Key techniques include:
- Handling missing values
- Converting categorical features to integer form
- Scaling and normalizing datasets
- Dealing with outliers
Tools like Pandas and Scikit-learn offer functionalities for data manipulation, cleansing, and visualization.
Ensuring Data Quality and Relevance
High-quality, relevant data is crucial for accurate recommendations. Steps to ensure data quality include:
- Consistent data storage
- Smooth data export from various sources
- Data augmentation
- Proper data splitting (typically 80:20 train-test ratio)
By focusing on these aspects, you can establish a solid foundation for your AI-powered recommendation system. This enhances personalization and drives customer engagement.
Data Preprocessing Step | Purpose | Common Techniques |
---|---|---|
Data Cleaning | Remove errors and inconsistencies | Handling missing values, outlier detection |
Data Transformation | Convert data into suitable format | Standardization, normalization |
Feature Selection | Choose relevant attributes | Correlation analysis, principal component analysis |
Data Splitting | Prepare data for model training | Train-test split, cross-validation |
Machine Learning Algorithms for Product Recommendations
ML algorithms are crucial for modern AI-powered recommendation engines. They delve into vast customer data to find patterns and predict user behavior. This allows businesses to offer product suggestions that match each customer's preferences and actions.
The effect of these AI-driven suggestions is profound. According to McKinsey, algorithm-based recommendations make up 35% of Amazon's sales and influence 75% of Netflix content watched. This personalized strategy has greatly benefited businesses:
- Increased sales and revenue growth
- Enhanced customer engagement
- Improved product discovery
- Higher average order values
- Strengthened customer loyalty
Popular ML algorithms for recommendation engines include collaborative filtering, content-based filtering, and matrix factorization. These methods help businesses offer timely, scalable suggestions. They introduce customers to products they might not have found on their own.
As the field advances, deep learning and reinforcement learning are becoming key for better recommendations. These advanced techniques learn from user interactions. They promise to provide more personalized and engaging shopping experiences in the future.
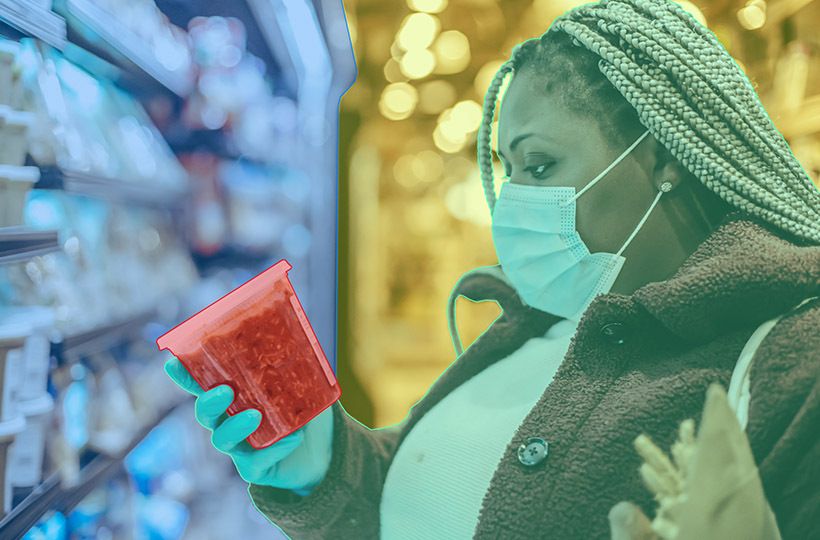
AI for Product Recommendations: Implementation Strategies
With 76% of shoppers finding current experiences not personalized enough, AI offers a solution. Let's delve into key strategies for successful integration.
Selecting the Right AI Algorithms
It's crucial to choose AI algorithms that match your business objectives. Consider your product variety, customer demographics, and data availability. Popular choices include collaborative filtering, content-based filtering, and hybrid models.
E-commerce Integration
For AI-powered recommendations to thrive, seamless e-commerce integration is essential. Utilize a Customer Data Platform to consolidate data sources and ensure data flows smoothly. This strategy enhances engagement and increases conversion rates.
Model Optimization
Continuous learning and model optimization are crucial for sustained success. Monitor key metrics like click-through rates and average order values. Use these insights to refine your AI models, thereby improving recommendation accuracy.
Strategy | Benefit | Impact |
---|---|---|
AI Algorithm Selection | Tailored Recommendations | 56% increase in customer return rate |
E-commerce Integration | Unified Data Flow | Higher engagement and conversion rates |
Model Optimization | Improved Accuracy | Increased revenue per visitor |
By adopting these strategies, you can leverage AI to create personalized shopping experiences. This approach not only meets customer expectations but also fuels business growth in the competitive e-commerce arena.
Enhancing Customer Experience through AI-Driven Personalization
AI-driven personalization is transforming how e-commerce interacts with customers. With data volumes soaring to 79 zettabytes in 2021 and expected to reach 181 zettabytes by 2025, companies now have the tools to craft highly personalized shopping experiences.
Reebok and Pandora are at the forefront, using AI to send highly targeted messages and offers. They analyze customer data, shopping history, and real-time behavior to suggest content across various platforms. This ensures a cohesive experience, with consistent messaging across all touchpoints.
AI-powered chatbots are essential for engaging with customers. These virtual assistants offer support around the clock, in multiple languages, and efficiently handle basic queries. This frees up human agents to tackle more complex issues, enhancing service quality.
AI Personalization Benefits | Impact |
---|---|
Customer Satisfaction | Improved engagement and loyalty |
Revenue | Increased sales through upselling and cross-selling |
Marketing Effectiveness | Higher conversion rates and average order values |
Customer Retention | Optimized interactions through tailored content |
Investing in ethical AI personalization allows businesses to craft valuable, relevant experiences while safeguarding customer privacy. This strategy boosts customer satisfaction and drives growth and efficiency in e-commerce.
Measuring the Impact of AI-Powered Product Recommendations
AI-powered product recommendations have transformed e-commerce. To assess their impact, businesses must concentrate on specific KPIs for your recommendation system. These metrics are vital for monitoring performance and enhancing strategies.
Key Performance Indicators
Key metrics include conversion rates, customer engagement, and average order value. Companies leveraging AI for recommendations have witnessed sales surges of up to 300%. Tailored suggestions can elevate conversion rates by about 50%.
A/B Testing for Continuous Improvement
A/B testing is pivotal for enhancing recommendation systems. It allows for the comparison of different versions to pinpoint the most effective strategies. This method ensures the AI model remains up-to-date and impactful.
Ethical Considerations and Privacy in AI-Driven Personalization
It is vital to ensure transparent AI decisions to build trust. Companies must clearly explain how they handle customer data. This transparency is not only ethical but also beneficial for business.
Dealing with bias in AI algorithms is crucial for ethical AI. Businesses should use diverse training data and bias detection tools to ensure experiences are fair and inclusive for all. This approach not only meets ethical standards but can also increase revenue by 9%.
As AI influences the future of e-commerce personalization, focusing on AI ethics, data privacy, and transparent AI decisions is crucial. This approach will be key to long-term success and maintaining customer trust.
The Future of AI in E-commerce Personalization
The future of AI in e-commerce is promising, with a focus on personalization technology. As AI advances, e-commerce trends suggest more refined and personalized shopping experiences. Your online store will become more intelligent, anticipating customer needs and offering customized interactions.
AI-driven personalization is transforming the e-commerce industry. Recommendation engines, similar to those at Amazon and Netflix, are becoming more sophisticated. They analyze user data to forecast future purchases, boosting sales and enhancing customer loyalty. Virtual shopping assistants are poised to change online shopping, offering unmatched convenience and personalization.
AI's influence goes beyond customer-facing tools. It's transforming inventory management, optimizing supply chains, and improving fraud detection. AI-powered search and discovery technologies are enhancing user experience and efficiency. As you adopt these technologies, it's crucial to prioritize ethical considerations to keep customer trust.
FAQ
What is AI-driven personalization, and why is it important in e-commerce?
AI-driven personalization uses artificial intelligence and machine learning to analyze customer data. It provides tailored product recommendations and customized shopping experiences. This approach is vital in e-commerce as it meets consumers' expectations for relevant interactions. It enhances engagement, conversions, and customer satisfaction.
What are the benefits of AI-driven personalization for businesses and customers?
For businesses, AI-driven personalization boosts brand loyalty and trust, increasing customer lifetime value. It saves time, improves efficiency, and offers relevant product suggestions. For customers, it makes shopping easier, improves product discovery, and strengthens brand connections.
What types of data are used in recommendation systems?
Recommendation systems use customer data like past purchases, browsing behavior, and demographic details. Data cleaning and normalization are key to preparing this data for AI analysis.
How do machine learning algorithms work for product recommendations?
Machine learning algorithms analyze customer data to identify patterns and predict preferences. They provide tailored product suggestions based on past purchases, browsing history, and predicted interests. AI-powered engines introduce customers to new products, enhancing discovery and sales.
What are some key implementation strategies for AI-driven product recommendations?
Businesses should evaluate recommendation algorithms and datasets carefully. Integrating data with AI through a Customer Data Platform ensures unified data flow. Continuous learning and model optimization are vital. Tracking metrics like click-through rates and conversion rates helps evaluate performance.
How can AI-driven personalization enhance customer experience?
AI-powered personalization offers tailored product suggestions and personalized content. It improves customer service through AI chatbots. Recommendation engines create unique shopping experiences, fostering brand loyalty and boosting sales.
How can businesses measure the impact of AI-powered product recommendations?
Businesses track key metrics like click-through rates, conversion rates, and average order values to measure impact. A/B testing is crucial for improving recommendation systems. Monitoring long-term effects on customer loyalty and lifetime value is also important.
What are the ethical considerations and privacy concerns related to AI-driven personalization?
Businesses must follow ethical principles like fairness, transparency, and accountability when using AI-driven personalization. They should address data biases and clearly communicate data policies to customers. Balancing personalization with privacy is key to maintaining trust and complying with regulations.
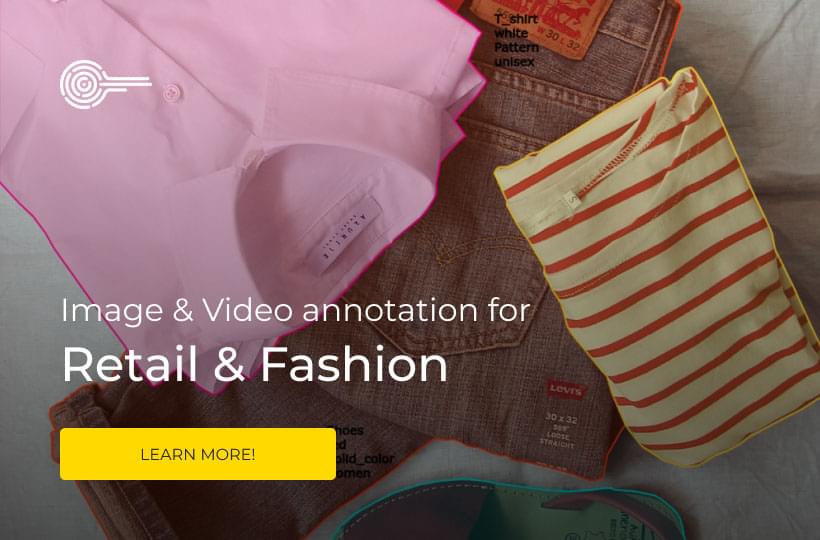