Legal AI Concepts in 2024: Navigating the Future of Law and Technology
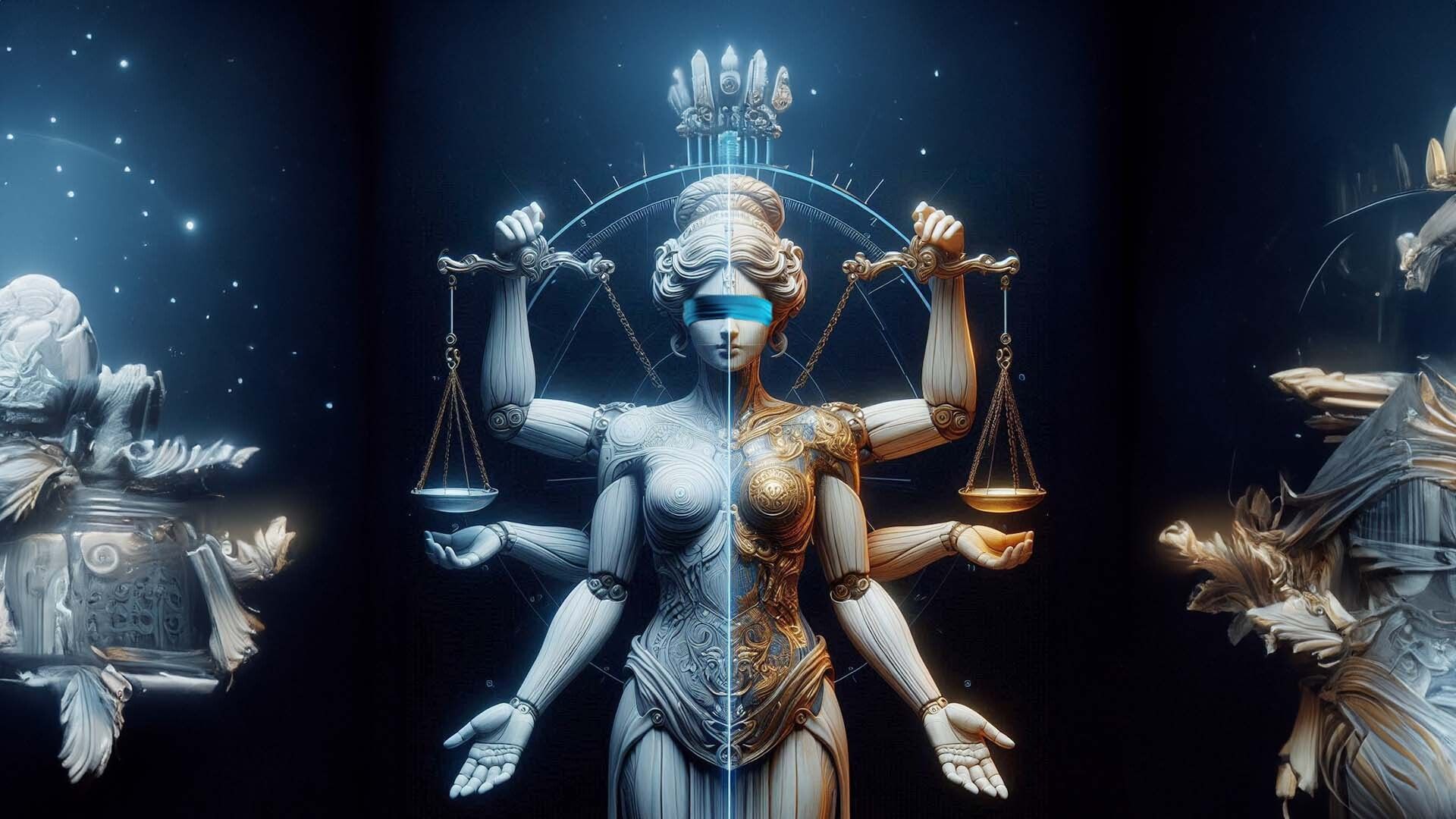
Despite AI's quick evolution, the laws guiding it lag behind. Compliance and oversight are tricky with it due to conflicting interests, tangled regulations, and legal gaps. To cope, most countries in the world are scrambling to propose principles and legal AI concept frameworks on how to handle legislating models, in particular generative models that took the world by storm.
We hear ideas and proposals coming from the White House, the European Union, the United Nations, G20, and pretty much any governmental or global body of any size. This field's created countless workshops, journals, and cooperative efforts. Everyone seems to agree that Ethical AI is the way forward, but there’s no consensus on what that means or actually looks like.
Let’s explore the confusing world of legal AI concepts and unpack some general trends:
Key Takeaways
- The U.S. currently relies on a sectoral, self-regulatory approach to AI, while efforts to develop a comprehensive federal framework have fallen short. The EU has taken a more centralized risk-based approach.
- The AI & Law sector is thriving academically, with increased research, conferences, and collaborations focusing on the intersection of law and technology.
- Despite the explosion of interest, there’s no consensus on the implications of AI - it presents provocative concepts that can’t be easily defined.
- Balancing innovation with regulatory safeguards is crucial in AI development, as emphasized by legal experts and governmental bodies.

Understanding AI Ethics
Software development discourse has been all about dividing ownership and responsibility since the very start of computer science. We had debates about whether software is a service or a good, copyright implications of various platforms, and countless lawsuits about determining responsibility between users, developers, and service providers.
But what happens when our software is capable of doing things we thought were uniquely human, with minimal instruction?
This isn’t a hypothetical question anymore. AI-generated art is winning prizes, and chatbots are passing legal exams in top schools. There are fewer and fewer tasks that humans beat AI on. Here is an illustration:
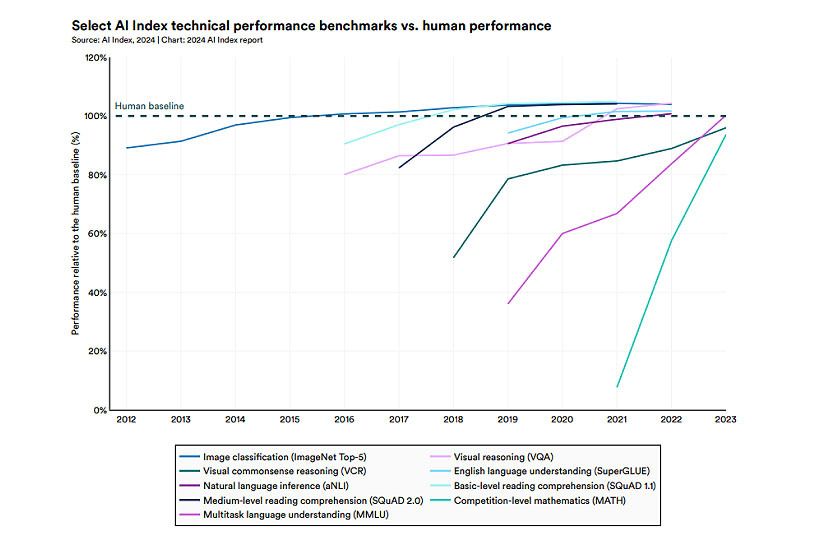
AI Index Report - Human vs AI Benchmarks
Unsurprisingly, the debates that we thought were more or less solved came back with a vengeance, more complex than ever before.
Early Foundations
Science fiction has been portraying robots and AI since the last century, from popular authors like Isaac Asimov to TV shows like Star Trek. Turns out, all the moral and ethical dilemmas raised by the genre weren’t just fantastical in nature.
Asimov’s laws of robotics were even brought up while discussing European legal norms for AI. While these don’t provide a comprehensive definition, they’re a good place to start:
- A robot may not injure a human being or, through inaction, allow a human being to come to harm.
- A robot must obey orders given it by human beings except where such orders would conflict with the First Law.
- A robot must protect its own existence as long as such protection does not conflict with the First or Second Law.
More recently, The National Science and Technology Council published a forward-looking report about preparing for the future of AI in 2016. In it, they specifically emphasized the importance of ‘ethical training’ and the involvement of ethicists alongside scientists, developers, and other domain experts.
So What Are AI Ethics Today?
AI ethics is an entire field of study concerned with understanding the ethical implications of artificial agents in different fields. Broadly, it focuses on developing and using AI technologies in a responsible way that benefits society.
This field is concerned with harm avoidance, fairness, privacy, accountability, and transparency. Its objectives are to:
- Minimize the risks and potential negative consequences of AI.
- Maximize the positive contributions AI can make to individuals and society at large.
- Incorporate human judgment and accountability into the use of AI as appropriate.
The Intelligence Community's introduction of AI Ethics Principles expands on this by talking about ethical development and the use of machine learning. These principles underscore the necessity of upholding human rights, and legal and policy compliance while doubling down on the importance of transparency in AI applications.
Why AI Ethics Are Tough to Agree On
As any large societal problem, AI ethics span a large number of stakeholders:
- Private companies, such as Google, Meta, and others - developing models and promoting them, all motivated by different economic and societal factors. The largest players set standards for the market.
- Investors and consumers vote with their time and wallets for a range of different AI applications and models, which steers their development in specific directions.
- Governments, with many agencies and committees debating this problem in every nation. Each would have its own approach and ideas about what AI should and shouldn’t be like. The largest regulators like the US and the EU set the tone for the entire world.
- Intergovernmental entities like the United Nations, UNESCO, the World Bank, and others, all come with different specialized perspectives. Regulators in different countries pay close attention to their work.
- Academic institutions, interested in researching the field and its impact. All researchers come from diverse backgrounds and traditions, so their ideas support both governments and companies in their endeavors.
This isn’t even an exhaustive list, because all of these stakeholders influence each other, and are driven by a wide range of motivations. So, there is broad agreement on principles, such as ‘do no harm’ and ‘maximize positive contributions,’ but the moment we start talking about specific judgments and what they mean, every institution or even individual will have their own approach.
Key topics in AI ethics discussions span from model foundations and data privacy to job impact, bias, and fairness. Of all these issues, Algorithmic Bias is among the most studied:
Algorithmic Bias
Algorithmic Bias is a technical term for what happens when an algorithm is making unbalanced or downright unfair calculations or decisions. Put simply, biased data creates biased algorithms, which then create uneven outcomes and turn all that bias into real-world impact.
When AI discriminates based on things like physical characteristics, race, or gender, it's called algorithmic bias. This impacts various fields, from education to healthcare, by leading to unjust outcomes.
For example, Gideon Christian’s research points out that facial recognition technology shows failure rates as high as 35% when dealing with the faces of people of color, especially black women. Moreover, certain self-driving cars are worse at detecting children and darker-skinned pedestrians, which poses bigger risks to them. In the finance sector, algorithms have charged higher interest rates to certain minority groups, illustrating a clear need to address fairness issues.
How to Reduce Algorithmic Bias?
To reduce bias, experts suggest several key steps:
- Increasing the amount of data input. Using diverse high-quality data helps models make better decisions.
- Setting higher standards for accuracy. In other words, rigorous testing and data validation.
- Retaining human oversight for models. Using human-in-the-loop techniques for model training.
- Continuously auditing for biases. Involve individuals from diverse backgrounds and fields of expertise for perspective.
More than acting, it’s about focusing on fairness, an area where many companies admit they have not yet taken action due to the complexity involved.
Research shows that biases in AI can be surprisingly subtle. For instance, a Princeton study found that machine learning identified European names as more pleasant and associated 'woman' and 'girl' more with arts than with science and math
It's essential to combat algorithmic bias to build trust in AI systems. AI auditing and regulation primarily aim to mitigate AI bias and make models more transparent. Unfortunately, as algorithms become more complex, transparency is even harder to come by - making biases much more difficult to detect due to ‘black box’ decision-making of most models.
This kickstarted conversations about Explainable AI:
Explainable AI (XAI)
Explainable AI (XAI) focuses on making AI's decision-making process clear and understandable. In traditional AI, even engineers often don’t know how a model reached a specific result due to the existence of thousands upon thousands of variables. For reference, model complexity has been growing at an enormous rate over the past decade:
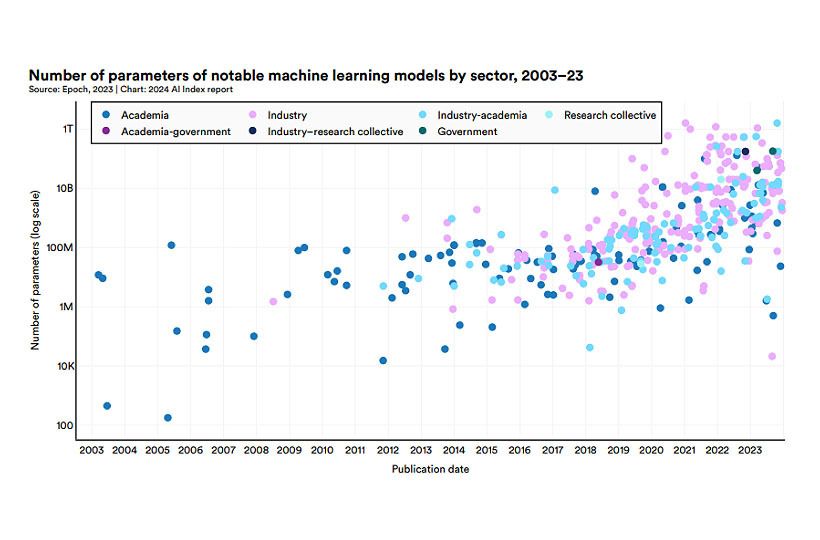
AI Index Report - Parameters of ML Models
With this amount of information, tracking down why a model made a specific call becomes an enormous task. So, key techniques in XAI, such as LIME and DeepLIFT, aim for predictive accuracy while ensuring that decisions are understandable. These strategies shed light on how AI models arrive at their predictions and reveal the connections between various model components. This significantly boosts the comprehensibility of AI technologies.
XAI includes continuous model evaluation, fairness checks, combating biases, model drift monitoring, and ensuring model safety. All these elements are vital for ensuring AI's reliability and longevity while minimizing harmful biases.
The European Union and the US FDA are leading efforts to bring XAI to the forefront through regulatory means. The EU's recent AI Act referenced earlier, places AI into different risk categories, requiring strict regulations for high-risk applications. XAI plays a pivotal role in meeting these regulatory standards, especially for high-risk AI systems that deal with personal data, such as facial recognition technologies.
The main goals of XAI are to:
- Increase trust and confidence. This includes both engineers and end users of models because traceable decisions lead to higher trustworthiness.
- Friction and speed. You can more easily evaluate the speed and performance of models when they are understandable - finding and solving specific problems is easier.
- Risk mitigation. Transparent models are compliant and easier to audit, which means they don’t make nearly as many mistakes.
In the evolving AI landscape, the significance of AI transparency and AI accountability cannot be overlooked. By adopting XAI and adhering to sound AI governance, organizations can cultivate trust with their stakeholders, meet regulatory standards, and utilize AI's benefits for societal development.
Unless, of course, you were looking for X AI, Elon Musk’s company focused on creating the next big AI product. Will it also be explainable in nature? That partly depends on how it uses data:
Data Privacy and AI
By its nature, AI requires lots and lots of personal data for executing tasks. This has raised concerns about gathering, saving, and using this information. There are many laws around the world regulating and limiting the use of personal data, from GDPR in Europe to HIPAA in the US regulating access to personal data. Here is a reference for how strict data protection laws are around the world:
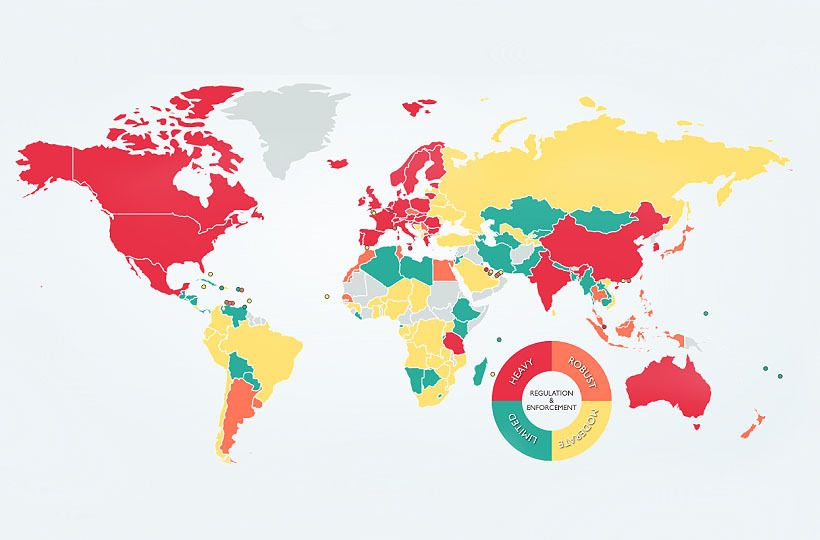
DLA Piper - Data Protection Laws
In the U.S, there isn't a large federal AI law currently, but some states have made their own rules. In the last five years, 17 states have passed 29 laws about how AI is made and used. States like California, Colorado, and Virginia are at the forefront. In 2023, seven new state laws about privacy and AI were made, expecting big impacts.
These state laws look at different sides of AI privacy. For example, they aim to:
- Make sure data is private and companies are held accountable
- Stop bad data practices and let individuals control how AI uses their data
- Make AI easy to understand and control
- Prevent unfair treatment and encourage fair AI design
Worldwide, there's a push to sync up privacy and AI laws. The White House’s “Executive Order on the Safe, Secure, and Trustworthy Development and Use of Artificial Intelligence” is considered the most comprehensive guideline in the US that exists today.
In general, respecting Personal Identifiable Information (PII) is even more important in the age of AI because its use tends to be difficult to predict, unlike with more traditional software.
Google's Checks platform and other AI solutions are coming out to assist companies with compliance and rules-following. They simplify keeping up with changing laws and promote clear communication. As AI grows, firms need to keep up with new privacy rules, which also affects how models make decisions in the first place.
AI Governance
AI governance consists of rules, processes, and norms that aim to ensure that AI technologies are developed and used in a safe and ethical way. It’s a legislative extension of AI ethics that tries to put comprehensive guardrails on model development. They ensure AI systems are created, used, and distributed in a responsible and ethical way.
This is mostly a response to public mistrust - as the negative impacts of AI, such as copyright infringement, privacy concerns, and so on become widespread, legislators take action. This, in turn, leads to companies and developers developing models that account for these challenges and make better decisions.
AI governance directly comes out of other concepts explained in this article and consists of:
- Ethical decision making
- Mitigation of biases
- Transparency and accountability
- Responsible use of data
The goal is to create a system and continually monitor AI development in accordance with a chosen framework.
How do AI governance frameworks work?
Worldwide, governments and regulatory bodies are working on numerous AI governance frameworks. For example, the European Union has proposed penalties up to 7 percent of global annual revenue for those not meeting the standards. In comparison, the United States unveiled the Blueprint for an AI Bill, outlining key principles for AI system design and deployment, but no ‘stick’ for compliance quite yet.
Companies like IBM are proposing different structures for responsible development, which include the CEO and senior executives being ultimately responsible for implementing responsible governance into AI development lifecycles.
They propose general principles of empathy, accountability, transparency, and bias control to ensure good governance. They suggest that you could implement:
- Informal governance, based on the culture and values of the organization.
- Ad hoc governance, with specific AI policies that relate to certain situations.
- Formal governance, which provides comprehensive rules and an oversight process.
Much like the rest of this ever-changing field, specific guidelines will vary between countries and organizations - there’s still no consensus on what an ideal governance system should look like.
Where the World of AI Regulation is Headed
AI's progress is making the future of AI regulation very significant. World entities are crafting frameworks to manage AI's impacts and benefits. The EU has taken a big step by passing the AI Act, the first comprehensive AI law. It restricts some uses of AI and introduces a risk grading system.
Many places are advancing in AI regulation beyond the EU. 31 nations have enacted AI laws, and 13 more are discussing them. For instance, China has had companies registering their AI models since 2023. Meanwhile, African nations like Rwanda and Nigeria are preparing strategies for AI development and protection. As the world looks to regulate AI, aspects such as bias, transparent AI, data protection, and proper AI oversight take center stage.
FAQ
What is the current state of AI regulation in the United States?
In the United States, AI regulation is not yet comprehensive. It's managed by a combination of national and state laws, self-regulation by the industry, and decisions made in the court. These approaches face difficulties though. There are conflicts due to different rules, and legal systems can struggle to address AI disputes effectively.
How is the European Union addressing AI regulation?
The European Union is leading the way in AI regulation through the EU Artificial Intelligence Act, which was passed in March 2024. This act categorizes AI by the risks they pose. It also sets forth duties for AI providers, including the need to comply with copyright rules, reveal data used for AI learning, and mark content changed by AI.
What are the key ethical considerations in AI development and regulation?
Ethical concerns are central in developing and regulating AI. Fast-evolving generative AI is raising red flags because of its misuse potential. Governments are starting to question if they should impose tougher controls. It's crucial to use AI responsibly and ethically to safeguard important data without hindering innovation.
How can businesses address algorithmic bias in AI systems?
To combat algorithmic bias, teams should conduct risk evaluations focusing on AI. They should ensure that content coming from AI is checked and adjusted by people to avoid spreading bias. Leaders in digital advertising need to be on the lookout for issues caused by generative AI, like bias continuation.
What is explainable AI (XAI), and why is it important?
Explainable AI (XAI) concentrates on clarifying AI processes. As AI becomes advanced, understanding its decisions becomes much harder, so we need specific tools to do it. XAI offers a window into how AI models draw their conclusions. This transparency is key for making AI trustworthy and dependable.
How do data privacy regulations impact AI development and use?
Data privacy is a key issue in AI because its training extensively uses personal information. The impact of the GDPR from the European Union is felt globally to this day. Its privacy principles are being weaved into AI laws around the world. As AI grows, companies must prioritize data protection and compliance with privacy laws to maintain customer trust.
What is the role of AI governance in ensuring responsible AI use?
AI governance includes the rules, steps, and structures guiding AI development and use. Strong AI governance ensures responsible and lawful AI use. This involves setting clear rules for AI growth, adopting risk management, and establishing accountability procedures.
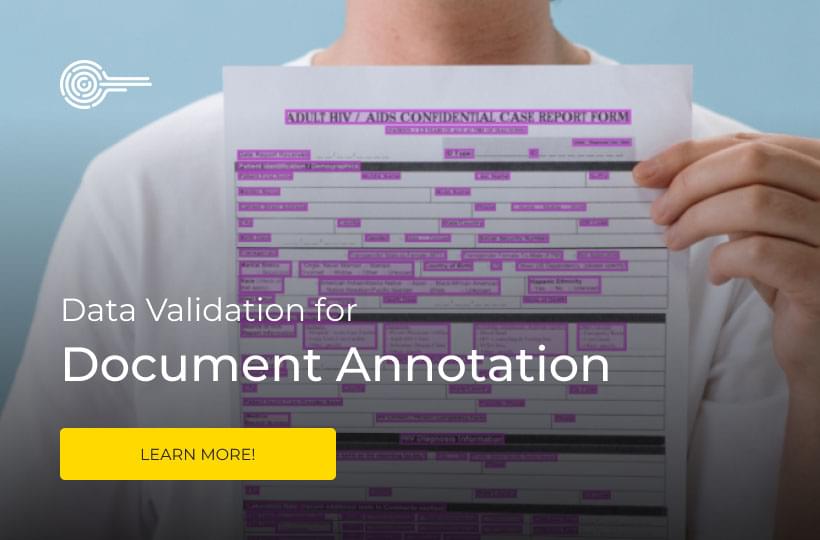