Improving Healthcare Outcomes with Medical Image and Video Annotation
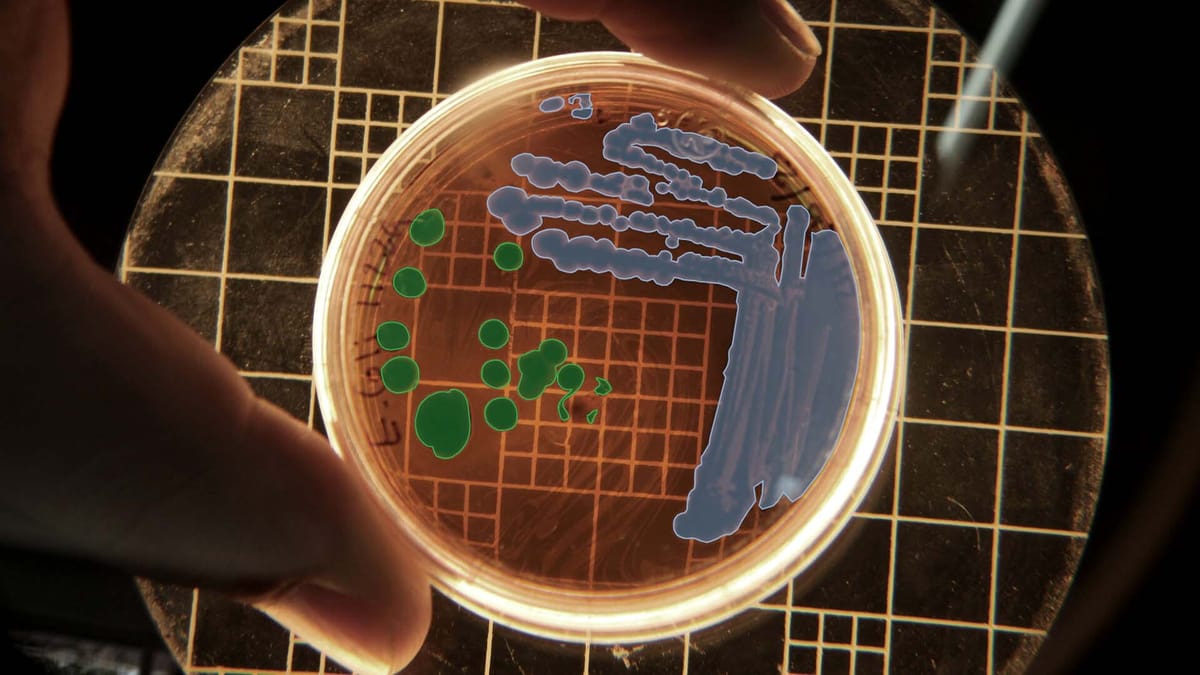
This article delves into the role of AI-driven medical image annotation services and video annotation. These practices enhance diagnostic accuracy and improve patient care. Advanced AI technologies, such as deep learning algorithms and neural networks, are key in medical analysis. They enable quicker and more precise identification of health issues like tumors or early disease signs in images.
Key Takeaways
- AI-based deep learning algorithms have significantly improved the accuracy and efficiency of medical image analysis.
- Medical video annotation contributes to speeding up the interpretation of complex medical images and enhances early disease detection.
- Distinct AI technologies enhance patient outcome by providing precise diagnostic improvement through AI.
- Medical image annotation services are vital in supporting radiology departments and assisting pathologists and oncologists.
- The need for rigorous data privacy and security measures is critical in the medical annotation landscape.

Introduction to Medical Image Annotation Services
Medical image annotation services are key to better diagnostics and patient care. This process labels medical images like MRIs, CT scans, and X-rays with important data. This lets AI models understand these images accurately.
What Is Medical Image Annotation?
For those in healthcare and AI, grasping the medical image annotation definition is vital. It's about carefully annotating medical images with the right data to train AI. This means highlighting specific features in images that might show health issues or abnormalities. Our annotations follow industry standards like DICOM, ensuring they work well with various imaging systems.
Medical images are different from regular pictures, with more layers and depth. So, our annotation work needs special tools and knowledge. These tools must support formats like DICOM and TIFF to fit smoothly into clinical settings.
Importance in Healthcare
The role of image annotation in healthcare is huge. Accurate annotation helps doctors spot important details in images, leading to better diagnoses. This boosts diagnostic accuracy and improves patient care.
Also, clinical data annotation is vital for getting medical data ready for machine learning (ML). About 80% of data is for training ML models, showing how critical good data is for AI. Keeping annotations consistent and standardized is also key for building strong AI systems.
Having experts from healthcare is essential for annotation work. It's also important that data labeling tools are easy to use. This lets medical professionals help with data preparation efficiently. By meeting these needs, we aim to make radiology workflows smoother and help develop reliable AI solutions.
Benefits of Medical Image Annotation Services
Medical image annotation services are essential in today's healthcare, bringing numerous benefits. They enhance diagnostic accuracy and streamline healthcare operations. These services are key for advanced machine learning, leading to better disease detection and treatment.
Enhanced Diagnostic Accuracy
The main benefits of medical image annotation are improved diagnostic accuracy. High-quality, labeled data helps AI algorithms spot patterns and diseases. This leads to early and accurate disease detection, vital for timely treatment.
Experts categorize images like X-rays and MRIs, aiding precise diagnostics. This supports better treatment planning, showing the importance of medical imaging AI in modern medicine.
Annotated medical images enhance the accuracy of diagnostic tools. They help detect fractures, tumors, and abnormalities with greater precision. This has a significant impact on healthcare, improving patient outcomes.
Streamlined Workflow for Healthcare Professionals
Medical image annotation services also boost efficiency in healthcare operations. They automate image analysis, freeing up medical professionals to focus on patient care. AI tools in annotation improve complex data interpretation, making workflows more efficient.
Companies like Keymakr lead with top-tier services, setting new standards. These services streamline workflows and support intelligent decision-making with precise insights from annotated data.
High-quality annotation services, backed by robust quality assurance, ensure accuracy and reliability. These advancements are key for future healthcare innovations. They lead to more efficient healthcare systems, benefiting both medical professionals and patients.
Different Types of Medical Imaging
Medical imaging is a vital part of modern healthcare, giving us deep insights into the body's inner workings. It's important to know the different imaging modalities to choose the right one for diagnosis and treatment. We'll explore X-rays, MRI, CT scans, and ultrasound technology, highlighting their unique benefits and uses.
X-rays and Their Uses
The use of X-rays is key in diagnostics. They work by passing a small dose of radiation through the body, creating images that show up dense structures like bones. This makes them essential for spotting fractures and infections. X-rays are also used in more complex procedures, like angiography and mammography, because they provide clear and quick images.
MRI and CT Scans
MRI and CT scans are invaluable in medical diagnostics. MRI uses magnetic fields and radio waves to create detailed images of soft tissues. This helps in diagnosing brain, spinal cord, and joint disorders. CT scans, on the other hand, combine X-ray measurements from different angles to create cross-sectional images. These images offer a detailed view of complex structures, aiding in the evaluation of trauma, cancer, and cardiovascular diseases.
Ultrasound Imaging
Ultrasound technology offers a non-invasive and real-time way to observe internal structures. It uses high-frequency sound waves, making it critical in prenatal care and guiding procedures like biopsies. Its versatility also extends to diagnosing conditions related to the heart, liver, kidneys, and other organs.
Our team ensures that each medical imaging type is annotated with the utmost precision. We use advanced tools and techniques from trusted sources like Keymakr to achieve accurate results. The process involves sourcing images from various partners worldwide, ensuring quality control through steps like error-free segmentation and collaboration with certified pathologists. This fosters reliable AI models in healthcare.
Medical Imaging Type | Key Benefits | Common Uses |
---|---|---|
X-rays | Quick and clear images of dense structures | Diagnosing fractures, infections, mammography |
MRI | Detailed images of soft tissues | Brain, spinal cord, and joint disorders |
CT Scans | Cross-sectional images of complex structures | Trauma, cancer, cardiovascular diseases |
Ultrasound | Non-invasive, real-time observation | Prenatal care, guiding biopsies, organ diagnostics |
Manual vs. Automated Annotation
The blend of manual and automated annotation techniques is key for top-notch results. Automation uses AI to speed up and scale annotation, but manual techniques are essential for accuracy. In complex or unclear cases, human annotators provide unmatched precision.
Radiology annotation technologies combine AI's speed with human annotators' accuracy. This ensures radiologists have detailed images for analysis, leading to better diagnostic decisions.
These advancements greatly improve workflow efficiency and healthcare outcomes. They provide a solid foundation for precise and reliable medical data.
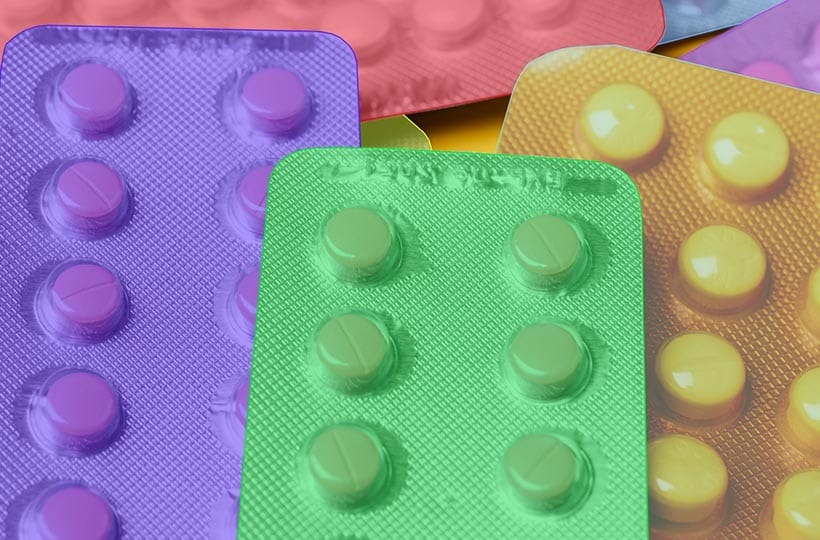
The Annotation Process
The medical annotation process is key to advancing AI-driven diagnostics. It provides robust and accurate annotated medical datasets. By systematically following key steps in medical image annotation, professionals ensure the reliability and quality of the derived insights.
Steps Involved in Medical Image Annotation
Successful medical image annotation involves several critical steps. Initially, annotated medical datasets are collected, encompassing diverse imaging modalities such as X-rays, MRIs, and CT scans. Expert annotators then use various annotation techniques like bounding boxes and semantic segmentation to label significant features within these images. Additional steps include:
- Bounding Boxes: This common method helps in identifying and labeling target objects effectively.
- Polygon Annotation: Allows for detailed mapping of irregular shapes, facilitating advanced computer vision applications.
- Key Point Annotation: Essential for detecting specific body points and facial expressions, often used in facial recognition technologies.
The quality and precision of these annotations are critical. They directly influence the AI model's performance in clinical applications.
Quality Assurance Measures
Ensuring high-quality annotations requires stringent quality assurance in annotation protocols. Implementing these measures guarantees that the annotated medical datasets maintain accuracy and consistency:
- Expert Review: Medical professionals and experienced annotators cross-verify the annotations to confirm their accuracy.
- Automated Validation: Advanced AI tools are used to check for discrepancies and ensure uniformity across the datasets.
- Continuous Feedback: An iterative feedback cycle allows for ongoing improvement and refinement of annotation standards.
Quality assurance in annotation is essential for developing reliable AI algorithms. These algorithms can significantly enhance diagnostic precision and patient outcomes.
Challenges in Medical Image Annotation
The field of medical image annotation has vast opportunities to enhance healthcare outcomes. Yet, it confronts critical challenges like securing medical data and the need for skilled annotators. Overcoming these hurdles is essential for the effective deployment and growth of healthcare AI solutions.
Data Privacy and Security Concerns
Data privacy in healthcare AI is a major challenge. Protecting sensitive patient data is critical, as breaches could reveal personal health details. HIPAA regulations enforce strict data protection, but vulnerabilities persist. It's vital to implement strong encryption and access controls to reduce these risks.
The Need for Skilled Annotators
Skilled annotators are also a critical factor. Accurate labeling of medical images demands specialized medical knowledge. This often relies on experts like physicians and radiologists, increasing costs and limiting scalability. The complexity and variability of medical images require extensive training and experience, straining the workforce. To tackle these challenges, targeted training and AI-assisted annotation tools are needed to boost efficiency and precision.
Questions to Ask Potencial Providers
When evaluating AI healthcare partners, it's important to ask the right questions. Consider these to ensure the provider meets your needs:
- What is your annotation process? Understanding the steps and methodologies used in the annotation process is essential. Providers like Keymakr outline their detailed process, providing insights into their efficiency and precision.
- How do you ensure data security? Verify the provider’s measures for safeguarding data. This includes encryption, access controls, and compliance with relevant privacy regulations.
- Can you provide examples of past projects? Reviewing previous successes can help assess the provider’s capability and experience. For instance, Keylabs' work with EHRs and telemedicine data showcases our versatility and impact in the healthcare sector.
- How scalable are your services? Scalability is critical for handling varying volumes of data. Alegion, known for its focus on flexibility and scalability, is a good example of a provider meeting this criterion.
Future Trends in Medical Image Annotation
The future of medical image annotation is set for a major leap forward, thanks to machine learning and artificial intelligence. These technologies will lead to more automated and real-time annotation processes. This change will speed up the workflow and improve data model accuracy and efficiency. Deep learning and real-time processing will make healthcare outcomes better and more scalable.
The Role of Machine Learning
Machine learning is changing how we annotate medical images by introducing advanced algorithms. These algorithms learn from large datasets, making the process more accurate and cost-effective. LiDAR technology is also being used for precise 3D image annotation in healthcare. It's essential for tasks like object detection and segmentation, vital for disease diagnosis and treatment planning.
Integrating Annotation with Telemedicine
Another key trend is combining annotation with telemedicine, making healthcare more accessible. AI-annotated images will improve remote diagnostics and consultations. This integration will make healthcare delivery more efficient and increase access for patients in remote or underserved areas. It will also support radiological departments by improving image classification and object detection results, leading to better patient care.
FAQ
What is medical image annotation?
Medical image annotation labels medical images like MRIs, CT scans, and X-rays. It's vital for AI models to understand these visuals. This process is key for training AI to spot patterns, predict outcomes, and provide accurate diagnoses.
Why is medical image annotation important in healthcare?
It boosts diagnostic accuracy and prepares for surgeries and treatments. AI can spot irregularities and patterns, leading to early diagnosis and better treatment. This improves patient outcomes and care quality.
How does medical image annotation improve diagnostic accuracy?
High-quality, annotated data enhances AI's pattern recognition and disease identification. This results in early and accurate disease detection. It's essential for effective treatment.
What are the benefits of medical image annotation services for healthcare professionals?
These services automate medical image analysis, streamlining healthcare workflows. They save time and effort, allowing professionals to focus on patient care.
What are the different types of medical imaging that require annotation?
Various techniques like X-rays, MRI, CT scans, and ultrasound imaging are used. Each requires specific annotation techniques for accurate diagnosis and patient care.
How is artificial intelligence utilized in medical image annotation?
AI, including machine learning and deep learning, interprets complex data and automates annotations. This reduces time and error. Yet, manual annotation is needed for precision in complex or ambiguous cases.
What steps are involved in the medical image annotation process?
It involves collecting datasets, annotating them using AI or manual methods, and quality assurance. This ensures accuracy and consistency.
How does medical image annotation support radiology departments?
It enhances image analysis accuracy and speed in radiology departments. This improves diagnostics and aids in precise medical issue identification.
What are the main challenges in medical image annotation?
Challenges include ensuring data privacy and security and meeting the demand for skilled annotators. They need to understand both technical and clinical aspects of medical data.
What factors should be considered when choosing a medical annotation service?
Look at the provider's technological capabilities, healthcare AI experience, and data privacy standards. Ask about the annotation process, data security, and past successes to make an informed choice.
What are the future trends in medical image annotation?
Future trends include more machine learning for better annotation precision and efficiency. Integration with telemedicine will expand healthcare access, enabling remote diagnostics and consultations.
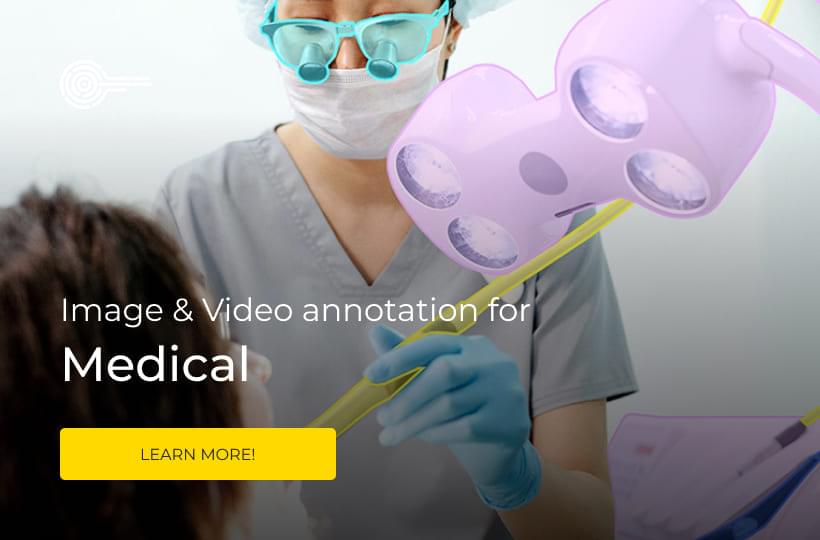