Image Annotation for Virtual Fitting Rooms
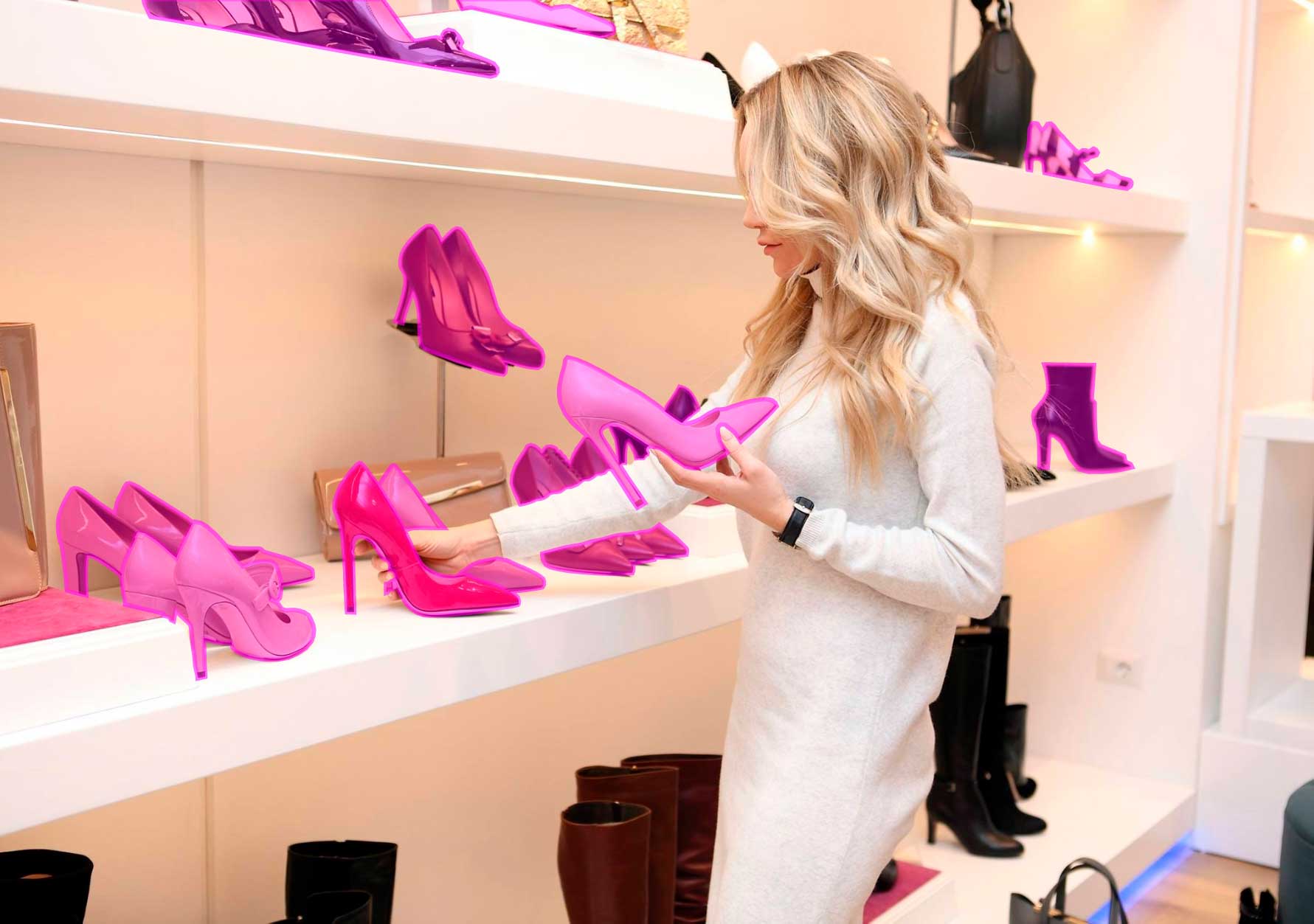
Covid-19 has hit the fashion industry hard, as of May 2020 sales of clothing and accessories were down 62.4% in the US alone. The restrictions placed on in store shopping have greatly impacted the sector and many brick and mortar operations are now struggling to survive.
However, the pandemic has also seen the rapid growth of online fashion retailers. Take Boohoo for example; the UK based, discount fashion brand has reported a 45% rise in online sales for the first half of the year. With this growing demand retailers are looking at ways to improve and streamline the online clothes shopping experience. One way to achieve this is through AI powered virtual fitting rooms.
Virtual fitting rooms allow customers to see what they would look like in any item of clothing. This technology is made possible by smart dataset annotation for machine learning. Keymakr is providing AI training data to support this emerging technology.
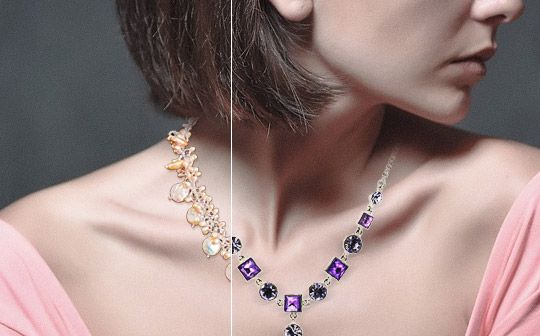
Meeting a Need
The interactive shopping startup Zeekit is at the forefront of virtual wardrobe innovation. In order to provide end users with a frictionless “trying on” experience a computer vision based machine learning model needs to overcome a number of challenges:
- Accurately mapping the body shape of each individual virtual shopper. A functional virtual wardrobe has to be able to accommodate a diverse range of body types.
- Recognising processing a range of clothing items and accessories. The AI model is required to identify a vast array of differently shaped objects and determine what they are and where they should be on the human body.
- These two processes need to work together so that any item of clothing or accessory can be accurately modeled on any individual body shape.
In order to achieve these complex objectives Zeekit engaged Keymakr to create bespoke training imagery.
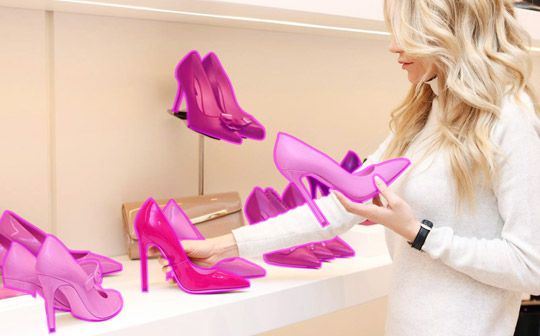
Creating the Dataset
In order to meet the client’s needs Keymakr’s experienced team of in-house annotators applied a range of annotation techniques to sets of raw image data. These carefully labeled images makeup the learning material that enables a virtual wardrobe AI model to recognise and process clothing:
- Polygon annotation: It is vital that training images accurately capture the shape of each clothing item. Polygon annotation allows annotators to plot points around an object and connect them with vertices. This gives annotators the freedom to precisely trace the outline of, for example, a leather jacket. This type annotation produces labeled items that adhere closely to their real world shape.
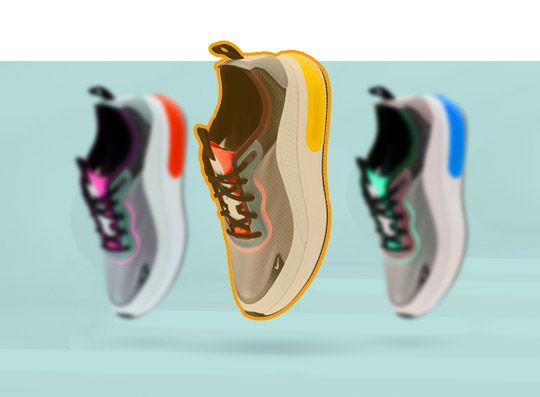
- Semantic Segmentation: In images that contain multiple clothing items the AI model must be able to identify and distinguish between different clothing types as well as non clothing objects. Semantic segmentation assigns each pixel in an image to a class e.g. dress, jacket, bracelet, background. This technique allows for a more granular understanding of a given image and improves the reliability of the virtual wardrobe.
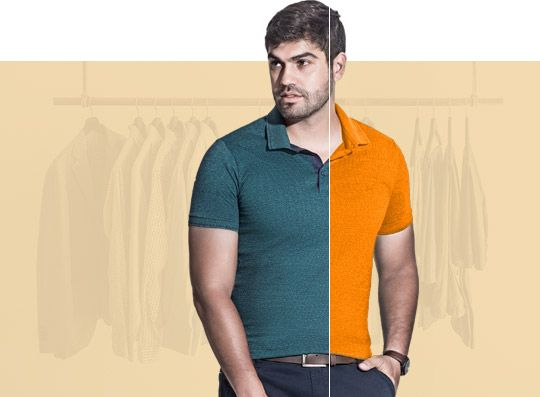
- Instance Segmentation: Instance segmentation provides an additional level of detail for training images. This technique identifies each instance of each object appearing in an image, for example, each individual shoe or individual sweaters worn by two people in an image. This enables the virtual wardrobe to locate specific clothing items and then map them to the dimensions of any shopper.
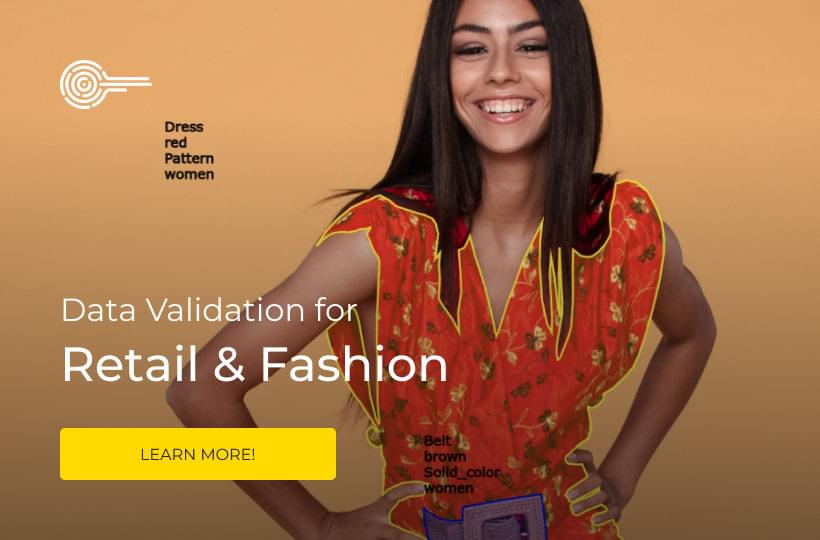
A Virtual Wardrobe
Supported by Keymakr’s precise annotation services Zeekit has been able to develop and bring to market the first dynamic virtual fitting room. This technology enables consumers to see themselves in any item of clothing that they can find online.
Keymakr combines professional, managed teams of annotators with bespoke annotation tools to meet all of your image and video labeling needs.
Contact a team member to book your personalized demo today.