Semantic Segmentation: Uses and Applications
Computer vision models have exploded in recent years. From Google’s self-driving cars and Tesla’s autopilot mode to Amazon’s Virtual Mirror, computer vision has transformed the face of modern technology. But machine learning image processing isn’t as easy as it may seem.
Training and validating computer vision and machine learning algorithms as well as image processing techniques require massive amounts of annotated data and high quality image annotation tool. That’s where image and video segmentation comes in.
Segmentation allows us to organize the data contained within images and videos into meaningful categories. Image classification and object detection may tell us the presence and location of certain objects. Segmentation allows us to dive deeper even in a real time.
So, what exactly is semantic segmentation annotation? How is it used in the real projects on image processing? Let’s jump right in this type of data labeled.
What Is Image Segmentation?
Image segmentation is a type of image annotation projects. Image classification and object detection often rely on bounding boxes. However a semantic segmentation technique can yield pixel-perfect accuracy, revealing fine-grained information about an image’s contents.
Instead of drawing a box around a particular object, image segmentation in image processing delineates the object’s exact boundaries. But there’s more to the target object story.
What’s the difference between semantic segmentation vs. instance segmentation in image detection?
- Semantic segmentation. This type of segmentation involves grouping each pixel under a particular label. For example, any pixel belonging to a car would be assigned under the same “car” category.
- Instance segmentation. Instance segmentation takes semantic segmentation to the next level by distinguishing between distinct objects belonging to the same category. Each car in the previous example would still belong to the same label but would be given different colors.
The type of image segmentation you use drastically alters the amount of information you can obtain from the annotated image. While semantic segmentation tells you the presence and location of an object, instance segmentation adds quantity, size, and shape.
Semantic Segmentation in Action
Let’s take a look at a few examples of how a data annotator may use semantic segmentation.
- Facial Recognition
Semantic segmentation of faces involves categories such as eyes, nose, and mouth, in addition to skin, hair, and background. Detailed segmentation of facial features can be used to train computer vision applications to distinguish an individual’s ethnicity, age, and expression.
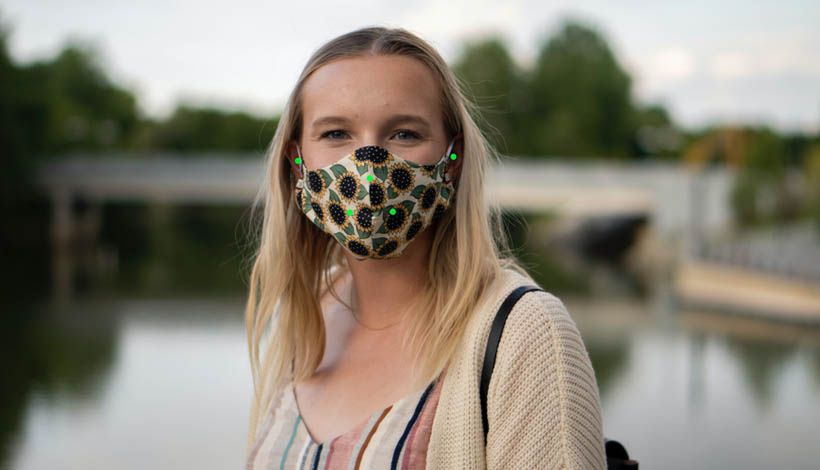
2. Self-Driving Cars
Autonomous vehicles are one of the most complex applications of computer vision to date. Poorly-trained algorithms don’t just decrease performance—they can endanger pedestrians and other vehicles on the road. That’s why artificial intelligence companies rely on semantic segmentation to maximize accuracy when it comes to detecting lanes and traffic signs.
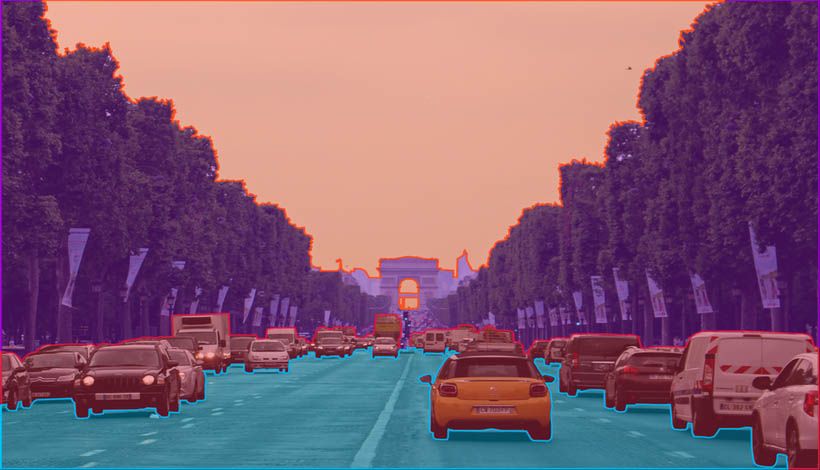
3. Virtual Fitting Rooms
Accurate clothing categorization can be a tremendously complex task due to the sheer variety of clothing articles. Semantic segmentation for clothing items has led to Amazon’s Virtual Mirror. It's a partially-reflective and partially-transmissive mirror that lets you try on clothes without ever getting changed.
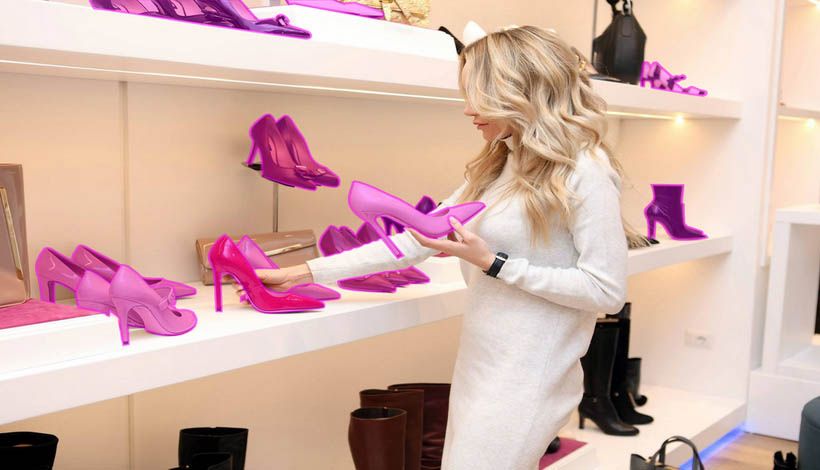
4. Medical Imaging and Diagnostics
Machine learning image segmentation plays a critical role in detecting medical abnormalities that appear in clinical scans, such as CT or MRI scans. Fast and accurate computer vision algorithms allow medical personnel to manage their time.
It's not neccesary to analyze each scan. Relying on computer vision doctors can streamline treatment and maximize the number of patients that can be examined.
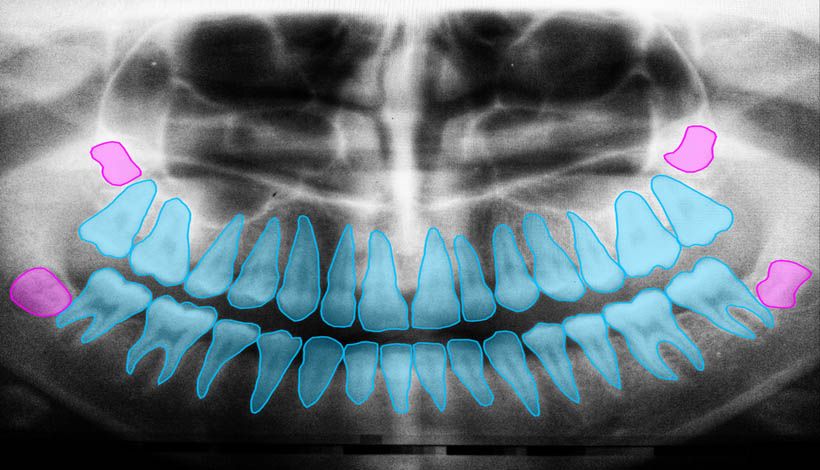
Professional Data Annotation Services for Computer Vision
Get to market faster with Keymakr. We provide pixel-perfect high level image and video annotations that meet your deadlines and suit your budget.
Keymakr relies on a combination of cutting-edge tools, verified workflows, and experienced annotators. That's why our main forces are to produce, structure, and label high volumes of training and testing data.
Are you interested in consistent, high-quality training data for your next computer vision project? Get in touch with a member of the Keymakr team to book your personalized demo today.
