Human-in-the-Loop Data Labeling for Machine Learning
We live in the era of big data. Every 18 to 24 months we generate as much data as has been generated in all prior human history. The exponential growth of digital data, primarily through the internet, has been of enormous benefit to the development of machine learning.
The variety and quantity of data that is now available for AI training is allowing innovators to refine Artificial Intelligence (AI) models to meet the demands of a myriad of industries and use cases.
However, this tremendous quantity of raw data presents some serious challenges. For example, accurate imagery data for AI development that require image annotations.. Manual image labeling is essential for dataset creation but can be costly and time consuming.
In order to correctly label the deluge of data now available for AI training, professional image annotation services are increasingly leveraging the power of human-in-the-loop (HITL) machine learning.
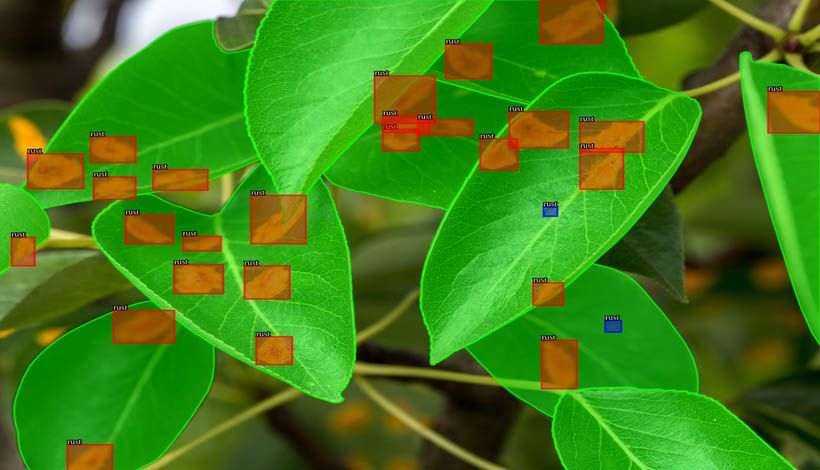
Reconciling Two Methods of Machine Learning
What is human-in-the-loop? At its most basic level HITL machine learning is a synthesis of the two main ways in which AI systems can be trained:
- Supervised learning is a process whereby AIs are trained using fully labelled data. A so-called “oracle”, usually a human annotator, will correctly annotate many thousands of pieces of data (e.g. images, video, sound) that can then be learned from by a machine learning algorithm.
- Unsupervised learning, in contrast, is where unlabelled datasets are fed into an AI. The AI then divides different ontologies (e.g. car, dogs, flowers) into categories following its own algorithmic processes. Different ontologies are separated into distinct groups but these objects or beings are not labeled.
Human in the loop AI seeks to combine these two approaches to machine learning and in so doing streamline the data labeling process, making development faster and more cost efficient.
The Loop – Reinforced learning or, simply, Data Verification:
The loop in HITL refers to the role of human feedback in the AI training process. The basic cycle of machine learning using HITL is shown below:
HITL machine learning aims to create a virtuous circle of training, where mistakes are learned from and humans contribute to the accuracy of an AI model. The advantages of this approach are significant. The HITL method combines the speed of automated data labelling with the accuracy and discernment of human intelligence. By utilising this powerful amalgamation it is possible to create large, precise training datasets quickly and cheaply.
Human-in-the-Loop Applications for Machine Learning Datasets
HITL training is central to the creation of many types of datasets in machine learning. The feedback loop allows for the speedy annotation of large quantities of images employing different labeling techniques including bounding box labeling and semantic segmentation annotation.
Facial recognition datasets can also be created that track the key features on countless faces. Text and speech and are also increasingly discernible by new generations of AI thanks to the training data that is being turbocharged by HITL processes.
Keymakr provides professional data annotation for computer vision, utilizing cutting edge techniques to ensure accuracy and scalability. Contact a team member to book your personalized demo today.
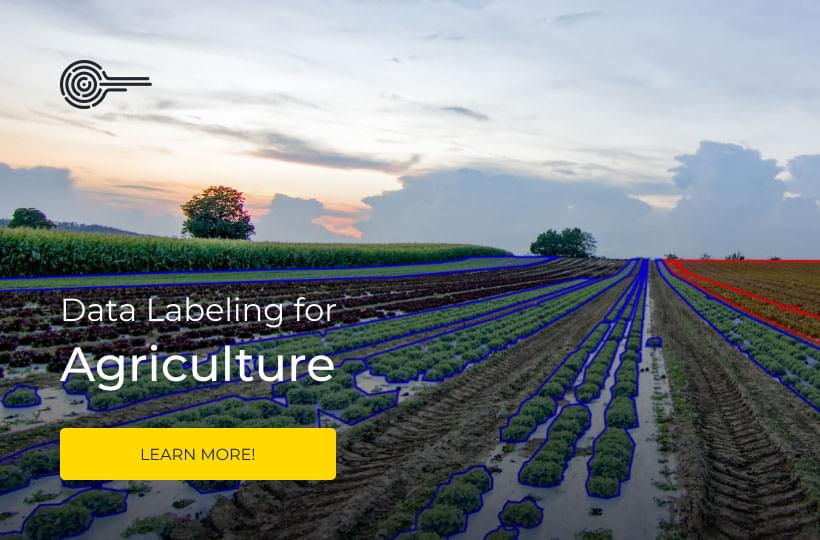