From Speech to Text: Top for Annotating Audio Transcriptions
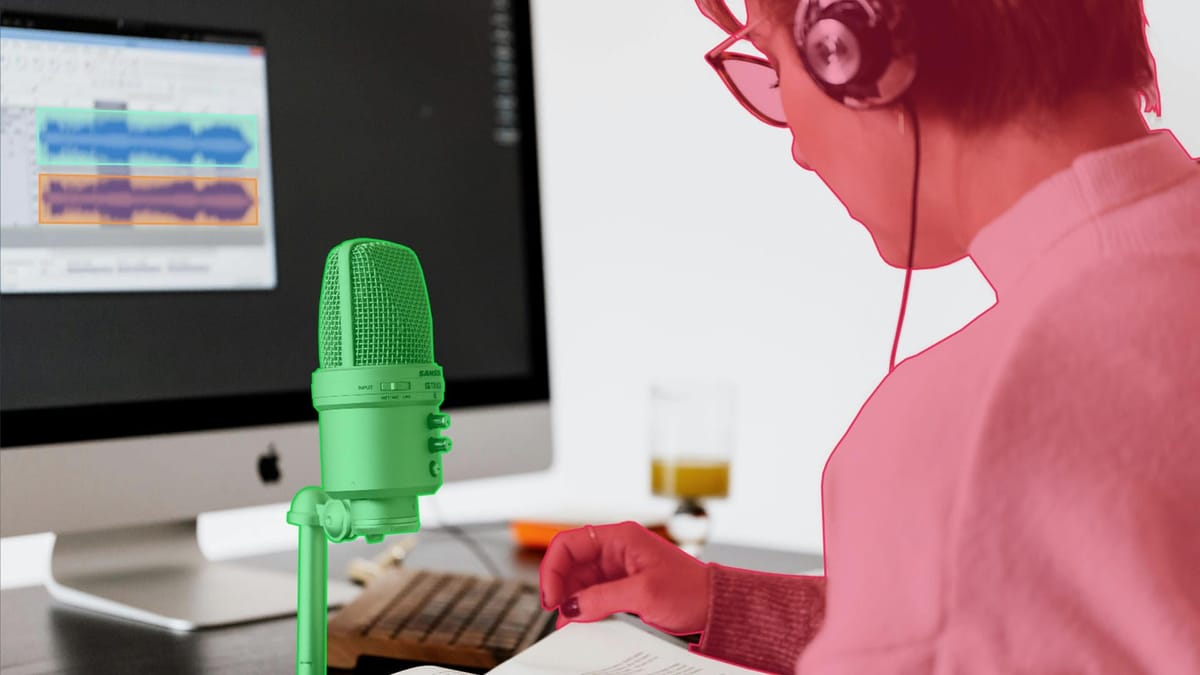
With the rise of artificial intelligence, audio transcription annotation is the key to better communication. We use automatic speech recognition (ASR) technology to convert spoken words into written text. This technology offers real-time transcription and automates tasks, improving productivity and making content more accessible to people with hearing loss.
ASR technology combines computer science, digital signal processing, and advanced artificial intelligence. It accurately converts speech into text. Sound quality, speaker diversity, and deep learning models are essential for high-quality work. Systems like Siri and Google Assistant can recognize over 40 and 35 languages respectfully.
Key Takeaways
- Audio transcription labeling increases communication with real-time transcription.
- The process boosts productivity by automating transcriptions.
- ASR technology ensures accessibility for those with hearing impairments.
- For accurate transcription, various speaker profiles and qualitative audio are most important.
- Google's Assistant and Siri systems demonstrate the multilingual ability of ASR systems.

More about Audio Transcription Labeling
Audio transcription labeling is a key to increasing the accuracy and organization of language programs. This process annotates audio with text, essential for training AI to grasp natural language. It benefits various industries through transcription services and automatic speech recognition. Automated tools can hit accuracy for high-quality audio with non-accented American English, highlighting the critical role of precise audio transcription labeling.
Importance and Clarity
Audio transcription labeling annotates audio files with text. It's vital for creating algorithms that increase conversational AI and speech recognition. This process trains AI models to make audio content accessible for SEO demands. Search engines don't index video or audio, so transcription makes audio content visible online.
Applications in Various Fields
Here is a small list of areas of AI transcription.
- Medicine. Document flow that allows you to reduce paperwork.
- Service department. Converts voice requests to text, for better and more transparent communication.
- Legal field. Recording court proceedings simplifies the search for information.
- Education. Recording lectures, making them accessible to the majority of people.
- Media and entertainment. Improving captions provides accurate and understandable text.
- Law enforcement. Interrogation recordings guarantee transparency.
It works with accurate language data, highlighting the importance of high-quality audio transcription.
Understanding Speech Recognition Technology
Speech recognition technology has developed rapidly. In 1987, IBM's Tangora voice system could understand up to 20,000 words, a significant advancement from earlier systems that could only recognize single digits.
How Speech Recognition Works
Modern speech Advanced Speech Recognition (ASR) uses acoustic models, such as recurrent neural networks (RNNs) or deep neural networks (DNNs). These increase the accuracy of the processed material, especially in noisy environments. Google Voice Search works in over 30 languages, demonstrating its broad reach and complexity.
Here’s how it works:
- Acoustic Modeling transforms audio signals into linguistic units.
- Language Modeling forecasts the next word in a sequence to enhance precision.
- Decoding deciphers the most likely word sequence from the audio input.
You can use this technology in voice transcription, smart home automation, and medical Documentation.
Limitations of Current Technology
Despite significant advancements, ASR technology faces several hurdles. Heavy accents and noisy settings can lower accuracy. For instance, IBM's VoiceType Simply Speaking application recognized 42,000 words in 1996, but today's systems continue to grapple with diverse accents and background noise.
Wayform of speech analysis also affected speech patterns or colloquial expressions. In 2017, Microsoft achieved a significant milestone in speech recognition by reducing the word error rate to 5.1%. Despite this progress, there is a need for ongoing algorithm refinement and model training.
In conclusion, further developments in speech recognition will help overcome current problems and achieve accuracy in transcription data.
Key Components of Audio Transcription
Each audio is subject to a careful methodology to ensure accurate and correct transcription. This is the process of collecting and identifying the speaker's speech.
Capturing Speech Data
Audio transcription tools are the basis of data collection. They reproduce audio in different formats, such as MP3, WAV, and M4a. We have an accurate and fast result at the end of the process. Verbatim transcription, capturing all sounds, is vital in legal or research settings.
Intelligent verbatim transcription, on the other hand, omits irrelevant elements. I produce a more concise transcript. Timestamps and inaudible labels enhance accuracy. Timestamps mark each utterance's timing, and inaudible labels note unclear speech.
Identifying Different Speakers
Another key aspect is differentiating speakers, known as speaker diarisation. This process labels speakers in recordings, which is vital in settings like meetings. Feature extraction techniques help attribute speech to individuals.
Edited transcription corrects errors for a formal look. Phonetic transcription represents sounds with symbols, useful for speech comparison. These methods together solve audio transcription challenges.
Using these techniques makes audio transcription reliable and essential in many fields. From accuracy to speaker identification, these elements are critical.
Use of Time Stamping
Timestamped transcription links specific text segments to exact moments in an audio file. It makes the transcription more accessible and easier to navigate, making it invaluable for automated subtitling and detailed qualitative analysis in research.
Consistency of Data Labeling
Consistent data labeling is the key to quality transcriptions. It improves data reliability and facilitates integration with ML models.
Annotation in healthcare ensures confidentiality and precision in medical transcription. Constant data collection and annotation updated speech models with new terms and conversational. Consistent labeling is vital for effective and responsible models.
Market trends note the importance of consistency. The global speech and voice recognition market is expected to grow significantly, as will the role of meticulous annotation practices. Learn how to Boost Your Annotation Accuracy with These Pro Tips.
Tools and Software for Transcription
The most effective transcription method involves using the right tools and software. The software enhances accuracy and efficiency in audio annotation. We'll consider popular transcription tools and how to select software.
Issues in Audio Transcription
In transcription, several problems include managing different accents and dialects, dealing with background noise, and maintaining high sound quality. Various methods and tools are used to solve these problems, achieving maximum accuracy and speed.
The Influence of Accents and Dialects
Accents and dialects complicate transcription significantly. Transcribers must excel in dialect-sensitive transcription when handling diverse audio sources. They face a dual challenge: grasping the content accurately despite regional variations and providing precise transcriptions. It often requires multiple listens and sometimes linguistic references to ensure accuracy.
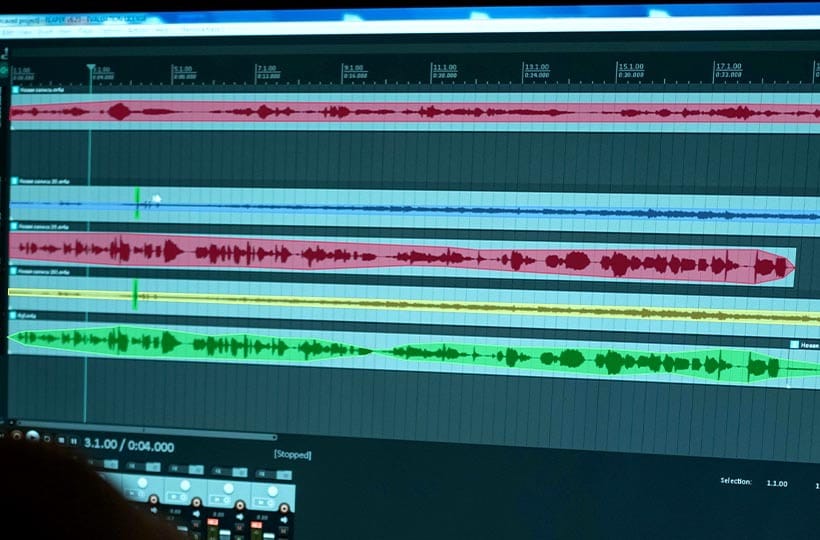
Methods for reducing Background noise
Background noise affects transcription quality. Ensuring sound quality is about separating the main speech from the surrounding noise. Techniques like noise-canceling headphones and audio editing software help achieve clear and accurate transcriptions.
Despite these hurdles, technological and methodological advancements are making progress. AI in speech recognition is continually updated to better handle varied accents. AI in speech recognition is continually updated to handle varied accents better. Enhancements in audio processing tools also offer significant audio quality enhancement and effective noise reduction solutions. These advancements enable more precise and reliable transcription services.
By employing these methods, transcribers can improve transcription quality. It has today's high standards for diverse and demanding users. Adopting advanced technology and skill development are essential to overcome audio transcription challenges.
Quality in Transcription Processes
Quality assurance is imperative due to the growing demand for accurate transcriptions. Transcription review and audio quality control processes ensure the accuracy and reliability of transcriptions. Next, you'll learn the main aspects of viewing and editing transcriptions. Also, the role of improving feedback loops should be considered.
Reviewing and Editing Transcriptions
Careful review and editing ensure accurate transcriptions. Transcribing one hour of audio or video without quality control can take about four hours. Detailed quality control checklists ensure document consistency. Professional human transcribers can type 80 to 100 words per minute, focusing on accuracy.
The review process involves several steps:
- Proofreading. Correcting linguistic errors or misinterpretations.
- Formatting Checks. Ensuring speaker labels, timestamps, and paragraphing are consistent.
- Final Review. A thorough review to ensure the final product is error-free and meets client needs.
Feedback Loops for Improvement
Iterative feedback is essential for improving the transcription process. Use collaborative platforms for better communication between transcription teams. This is necessary for improving the accuracy and quality of transcription in the future.
AI-annotated transcripts are not always accurate. Human verification is required to improve accuracy. The transcription process is guided by the experience and feedback of annotators and developers. Therefore, for high-quality transcription, monitor the quality of the audio data and provide a robust feedback system.
Consistent transcription quality is vital in legal proceedings, medical transcription, academic research, and business meetings. Precision is critical to conveying core ideas and information accurately.
The Role of Human Transcribers vs. AI
The combination of human transcribers and AI is helping the development of audio transcription. In a variety of industries, this method improves accuracy, reduces costs, and increases speed. But each method has its strengths and weaknesses.
Benefits of Human Transcription
A human's transcription is more accurate and better in complex scenarios. This is crucial in the legal and medical fields, where mistakes can have serious consequences.
High-quality speech recognition ensures accurate transcription. It reduces the risk of misinterpretation and aids in market research.
AI in Audio Transcription
Artificial intelligence quickly processes an hour's worth of material, significantly benefiting media industries.
Yet, AI transcription's accuracy falls short of human capabilities. The best AI platforms achieve up to 86% accuracy, sufficient for simple tasks but not complex scenarios. AI is best for recordings with a single speaker and minimal noise.
What should you choose if you need speed and accuracy in your projects? The best choice is a hybrid model that combines AI's speed with human verification accuracy.
The move towards hybrid models marks a significant growth in transcription technology. It will meet diverse transcription needs and boost productivity across various sectors. Learn more about when to use automatic vs manual annotation here.
Future Audio Labeling
What is the future of audio transcription? We predict that machine learning and AI will develop this industry. These technologies will increase accuracy, blurring the gap between human and machine annotation.
Progress in AI and Machine Learning
The development of synthetic voices similar to human speech has been a significant advance. This will benefit the healthcare and legal services sectors, where transcription accuracy is needed.
Eleven Labs and Replica Studios have high-quality natural language processing models. They simplify content creation and tagging, providing high-quality output. It extends content to diverse audiences and reduces the need for human resources.
New Tools and Methods
New tools are driving the industry forward. They help reduce the cost of replacing manual transcription. Text-to-speech (TTS) FakeYou gives you access to a library of voices. NaturalReader is an app that reads PDFs, online articles, cloud documents, and images.
Eliminating issues such as background noise and understanding accents will increase accuracy. This will allow us to transcribe large amounts of data in minutes. Using a hybrid type, we will achieve fast, error-free results.
In conclusion, we can note that continuous innovation and integration with AI are providing progress in audio labeling. Which is expected to affect accuracy, speed, and accessibility in various industries.
FAQ
What is audio transcription labeling?
Audio transcription labeling, or speech-to-text conversion. It is the conversion of spoken words into written text. This has the effect of teaching AI to understand natural language, which relies on automatic speech recognition (ASR) technology.
Why is audio transcription labeling important?
It's vital in healthcare to manage patient records and customer service for real-time text outputs. It also boosts accessibility for those with hearing impairments.
How does speech recognition technology work?
Speech recognition technology uses models like Deep Neural Networks (DNNs) and Convolutional Neural Networks (CNNs) to convert human speech into a machine-readable format. It enables real-time transcription, automating several tasks to boost productivity.
What are the limitations of current speech recognition technology?
Current ASR technology struggles with recognizing speech with heavy accents or noisy environments. It highlights the need for better recognition algorithms and model training to handle diverse and complex audio inputs.
What are the key components of accurate audio transcription?
High-quality audio data and speaker diarization are critical. These are essential in settings with multiple speakers, like meetings or interviews.
What are the correct practices in audio annotation?
Use time-stamping to link text to specific audio times. Maintain labeling consistency across datasets. These practices improve transcription reliability and usability.
What challenges does audio transcription face?
Challenges include recognizing various accents and dialects and managing background noise. Advanced audio processing and noise reduction technologies are needed to address these issues.
How is quality assurance achieved in transcription processes?
Quality assurance comes from thoroughly reviewing and editing transcriptions. A systematic feedback loop involving annotators and developers is also key. It helps refine transcription processes and improve AI model performance.
What role do human transcribers play in audio transcription?
Even with AI advancements, human transcribers are essential for high precision. Hybrid models combining AI efficiency with human oversight are becoming more common.
What are the future trends in audio transcription labeling?
AI and machine learning advancements drive future trends. These improvements will enhance the automatic transcription of challenging audio inputs. Emerging tools and practices will make transcription technology more sophisticated and widespread daily.
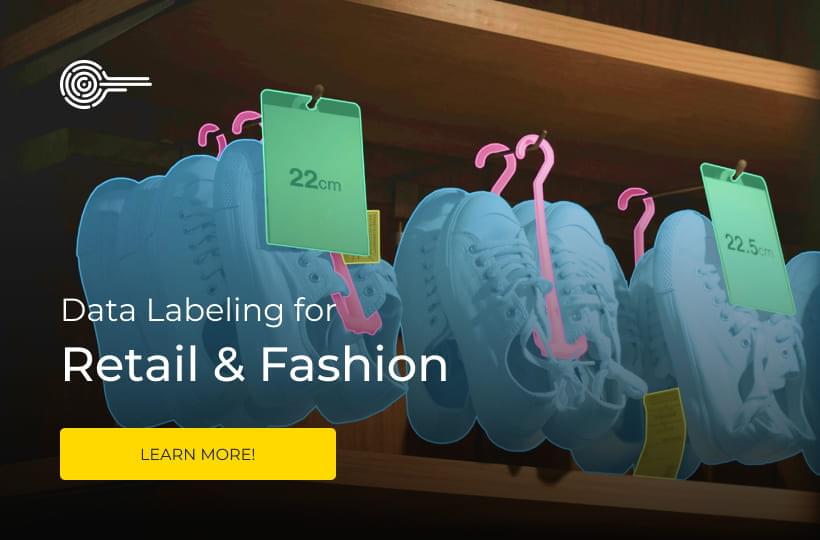