Video Annotation in Focus
What is video annotation
Many computer vision models are designed to interpret and operate within chaotic, moving, real-world environments. Video annotation helps AI to function effectively in these spaces by applying information to video footage.
This information is generally added to videos by human annotators who apply outlines and labels to video frames in line with the specific requirements of each machine learning model.
AI developers can make use of video training data creation by focusing on labeling best practice, as well as how to cope with obscure or unclear objects. This process of refinement can help AI companies to produce the highest functioning models possible.
The practice of video annotation
In most cases video annotation means teams of annotators locating relevant objects in each frame of video data. Most commonly annotators use bounding boxes to pinpoint objects that machine learning engineers have designated as important to label. In video footage of vehicle traffic this means annotators combing through each individual frame and placing a box around each car.
These boxes will then be assigned a colour and a label. Different machine learning projects require different ranges of objects to be labeled, in different ways. Annotation methods and techniques can be combined to create bespoke datasets, with specific goals in mind.
The core video annotation methods
The simplest form of video annotation is image classification. This means using one label to describe the contents of a frame of video. For example, in a video of a cat each frame would simply be labeled as “cat”, until the cat has left the video frame.
However, many times AI developers will need additional levels of detail and information in their annotated video data. This enhanced detail is made possible by the following annotation methods:
- Object detection: Machine learning engineers often require objects to be located in video training data. This is termed object detection and requires human annotators to place a box around each object and label them according to the class they belong to. If a piece of video footage contains cats and dogs, both would need to be boxed and labeled accurately in each frame.
- Semantic segmentation: This annotation method divides every pixel in each video frame into relevant classes. Initially annotators (using annotation platforms and labeling tools) outline the precise shape of target objects. For video training data for autonomous driving, this might mean cars, vehicles and pedestrians. Annotators will then assign classes to the remaining pixels in the image. In the traffic example this would of course mean the road itself, buildings, the sky etc. Semantic segmentation annotation is more time consuming than object detection but it creates video data that is richer in information for machine learning models.
- Instance segmentation: This method adds granularity to semantic segmentation by labeling each instance of a class of object. In a video of cats, for example, each cat would be outlined and highlighted with a different colour and labeled “cat 1”, “cat 2”, etc. This allows machine learning models to recognize the presence of multiple objects of the same class.

Choosing the right video annotation technique
There are a number of techniques that can be deployed to annotate video data. Annotation platforms allow machine learning developers to pick the techniques that are most appropriate for their computer vision model:
- Skeletal annotation: Video data is particularly useful when it comes to training AIs how to interpret movement. Skeletal annotation is often used to clearly show the movement of the human body in videos. In each frame annotators draw lines along human limbs, connected at points of articulation, e.g. shoulders and hips. The movement of these lines between frames provides a useful abstraction of a moving human body.
- Polygon annotation: Segmentation methods require a wide variety of shapes in each video frame to be precisely outlined. This technique enables annotators to capture these complex and irregular shapes. It works by connecting together many small lines around the edge of the target object.
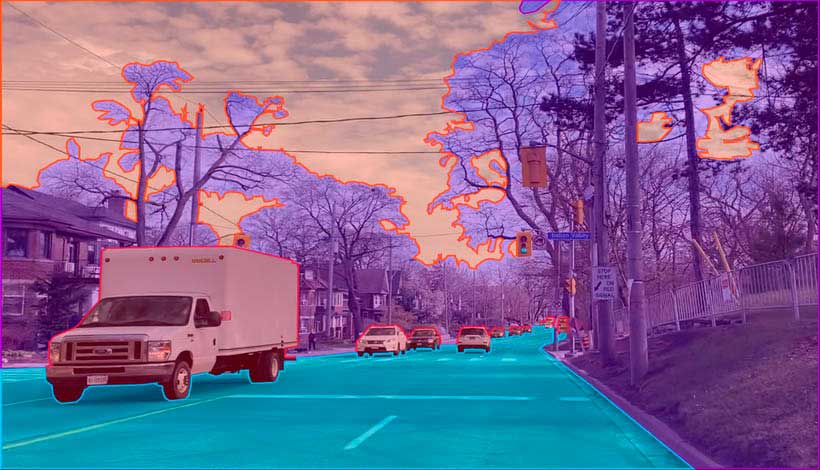
- Key point annotation: Video training data can be used to make facial recognition models for security or retail applications. This technology is enabled by key point annotation. This technique involves annotators marking key facial features (mouth, nose, eyes) as they appear in each frame of video.
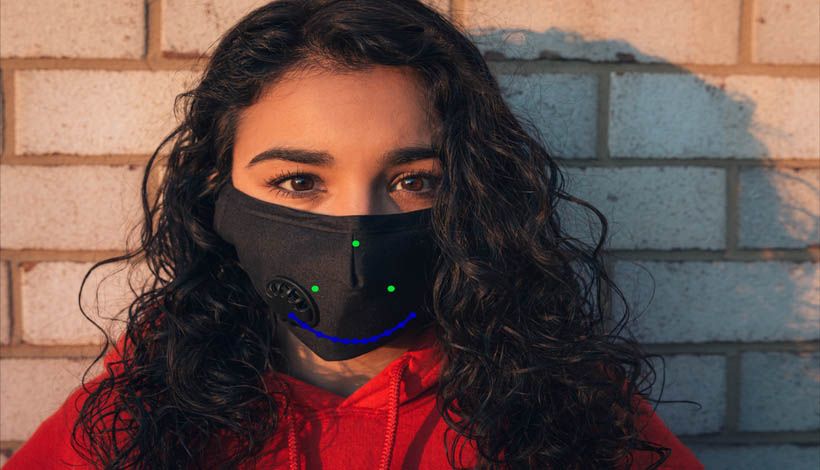
- Bounding boxes: This is the most straightforward annotation technique. Annotators move from frame to frame dragging boxes around objects of relevance. The advantage of this technique is its speed and simplicity, which can prove vital across thousands of video frames.
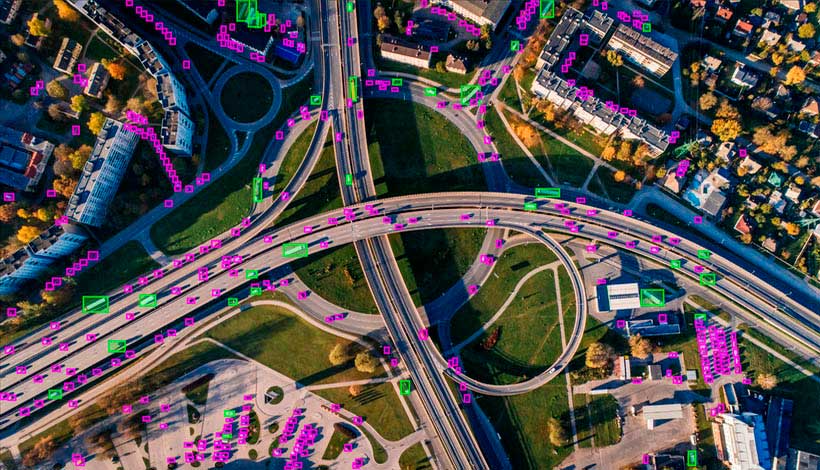
- Lane annotation: This is particularly useful for defining linear shapes, such as roads and train lines. Dashed or solid lines can be used to delineate these features, which helps contextualise other labeled objects.
How the right platform can optimize video annotation
Video annotation is substantially more time consuming than image annotation. Even short pieces of training footage can contain thousands of individual frames, all of which need to be conscientiously labeled. The right annotation platform can make a huge difference when it comes to accelerating video annotation without sacrificing quality. Keymakr is a professional annotation service that has developed a platform with features designed for video annotation:
- Object interpolation: This feature tracks objects through consecutive frames of video. Initially annotators locate the object with a bounding box in the first key frame and then again in a later key frame. An algorithm then places a box over the object in the intervening frames. This means that annotators do not have to find a specific object in each frame of a video and can instead simply verify the results of the interpolation.
- Metrics and analytics: Detailed analytics allow managers to assess performance and control for annotation errors. Managers can see every action taken by an individual annotator. This information can then be used for the purposes of training or improving productivity.
- Task assignment: Analytics also allow managers to assign tasks to annotators who have performed well, or are best suited to a particular project. Keymakr’s platform makes distributing work efficient and adaptive.
- Video annotation workflows: It can be a daunting task to annotate each frame of a longer piece of video training data. Keymark’s platform includes features that allow for multiple annotators to work on one video. Their annotations can then be seamlessly integrated at whichever point their work overlaps.
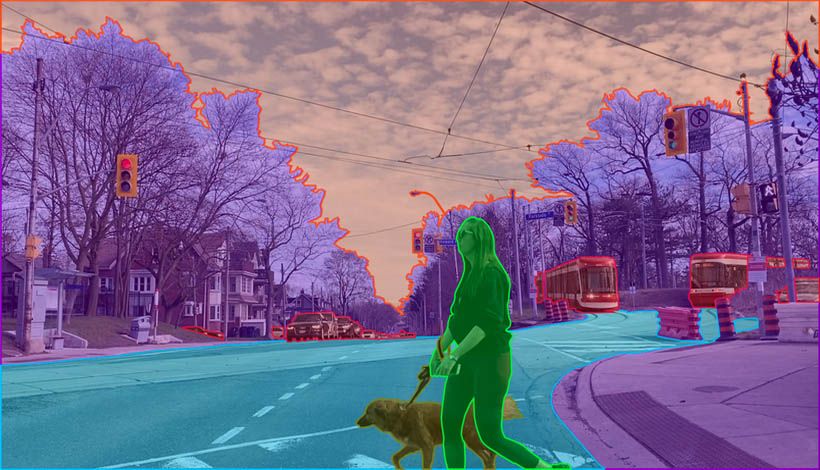
Video annotation services support innovation
Video annotation enables a vast array of exciting, transformative use cases. However, it is also an extremely time consuming and labour intensive task. Assembling a video annotation operation, including contracting and management, can be a debilitating distraction for many AI companies. Outsourcing to specialists in video annotation, like Keymakr, can provide advantages in a key areas:
- Annotation speed: Video annotation takes more time to get right. Annotation providers like Keymakr can leverage experience across many projects, as well as proprietary annotation software, to ensure that video annotation tasks are completed to schedule.
- Controlling costs: Outsourcing video annotation means that costs can be scaled up and down as data needs change. Video annotation can be delivered on demand by experienced providers, without committing to large scale labeling projects.
- Guaranteeing precision: Crowdsourced annotations can be cost effective but can also contain significant errors that impede development. Keymakr’s annotation teams work together, on-site, and are led by experienced team leaders and managers. This allows for far greater communication and troubleshooting capabilities, and ensures that video annotation quality remains at a high level.
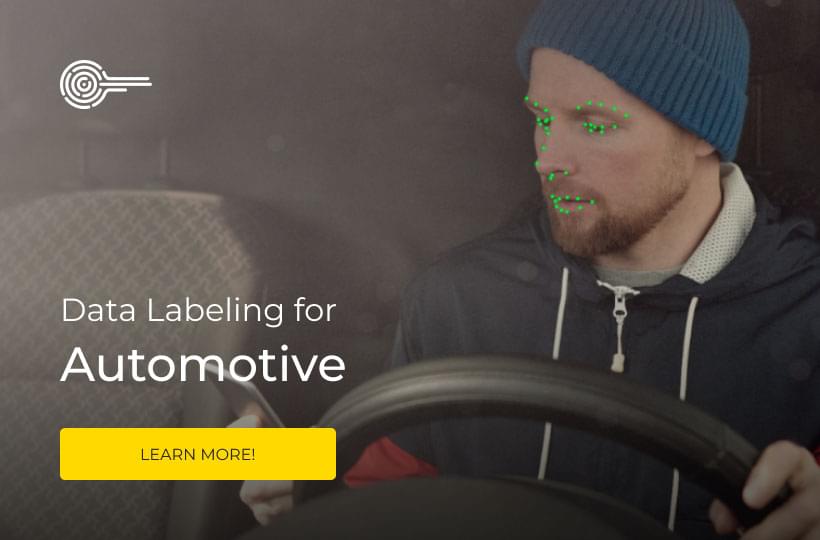
Collaboration for exceptional video annotation
Keymakr supports innovation with pixel perfect video annotation. Take advantage of our proprietary technology and our quality control expertise to create exceptional training datasets.
Contact a team member to book your personalized demo today.