Data Governance and Its Role in Data Quality
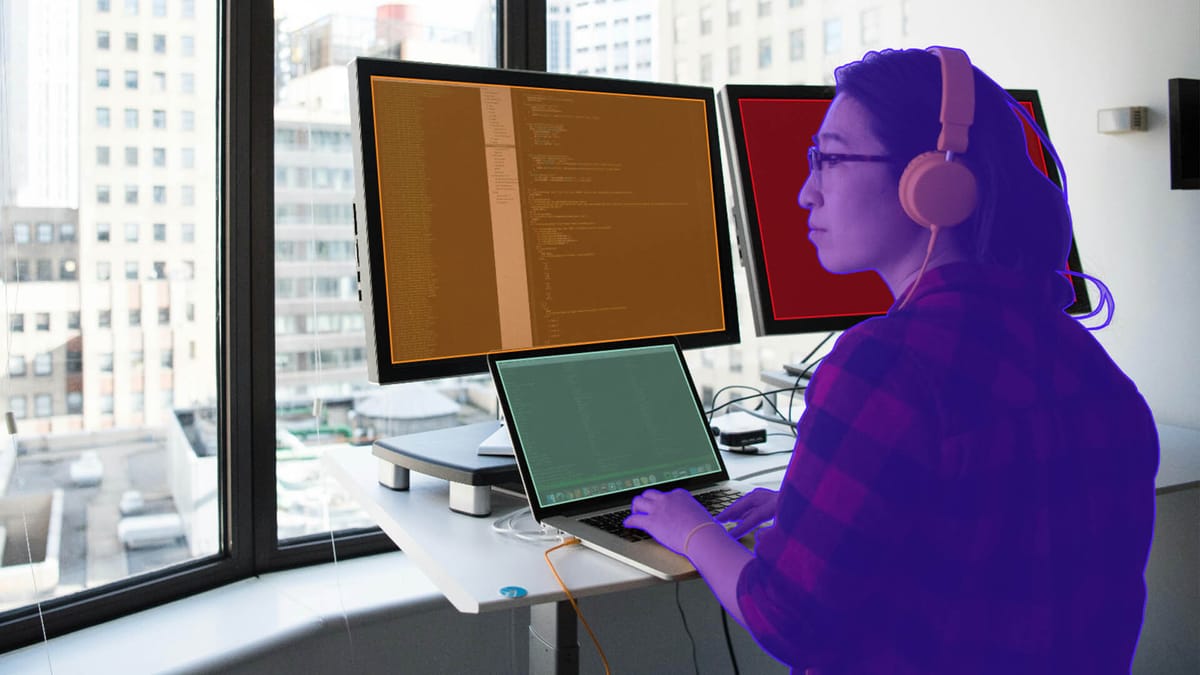
83% of companies face challenges in creating comprehensive user records from scattered data. As companies amass vast amounts of information, the significance of sustaining data quality through effective governance is more evident than ever.
Data governance frameworks are essential for organizations aiming to leverage their data assets effectively. They offer a structured method for managing data, ensuring its quality, and aligning it with business goals. By adopting a well-structured framework, companies can turn raw data into insightful information that supports informed decision-making.
The connection between data governance and data quality is crucial. Governance establishes the rules and processes, while quality assesses the data's accuracy and reliability. This partnership enables organizations to rely on their data and apply it with confidence across different applications.
As you explore the intricate world of data management, grasping the importance of governance frameworks in sustaining data quality is vital. These frameworks not only standardize data practices but also ensure adherence to stringent privacy laws and regulations.
Key Takeaways
- 83% of companies struggle with creating comprehensive user records from fragmented data.
- Data governance frameworks are crucial for managing and improving data quality.
- Effective governance helps organizations comply with privacy laws and regulations.
- Various frameworks exist, including models from McKinsey, PwC, and DAMA.
- Implementing a governance framework can lead to significant improvements in data accessibility and integration.

Understanding Data Governance and Data Quality
Data quality governance is vital in today's organizations. It ensures data's accuracy, consistency, and reliability by effectively managing data assets. Let's delve into the core elements of data governance and quality.
Defining Data Governance
Data governance establishes a system of authority over an organization's data. It fosters collaboration and enhances data management. Its importance is evident in its ability to standardize practices, boost security, and adhere to regulations.
Exploring Data Quality Dimensions
Data quality dimensions are critical for assessing data reliability. They encompass:
- Accuracy: Ensuring data is precise and free from errors
- Completeness: Ensuring all required information is present
- Consistency: Maintaining uniformity across systems
- Timeliness: Providing current information
- Reliability: Data's trustworthiness for decision-making
- Relevance: Data's applicability to business needs
The Interplay Between Governance and Quality
Data governance and quality are interdependent. A robust governance framework aids in enhancing and sustaining data quality. Through effective governance policies, companies can efficiently address quality concerns.
Data Governance Component | Impact on Data Quality |
---|---|
Policies and Procedures | Establishes standards for data management |
Data Stewards | Ensures accountability for data quality |
Data Lineage | Tracks data sources and transformations |
Data Security | Protects data integrity and confidentiality |
By concentrating on these areas, organizations can establish a strong data quality governance framework. This framework supports informed decision-making and propels business success.
The Importance of Data Governance Frameworks
Data governance frameworks are essential for managing and optimizing your organization's data assets. They are the foundation of a strong data management strategy, especially in large companies. A robust framework standardizes data operations, boosts accuracy, increases security, and ensures compliance with regulations.
Implementing data governance offers more than just organization. It directly influences your capacity to make decisions based on data and innovate. Let's delve into some key benefits:
- Improved data quality and consistency
- Enhanced data security and compliance
- Increased operational efficiency
- Better risk management
- Greater return on investment
One of the most notable advantages of data governance is the enhancement in data quality. This results in more accurate, consistent, and dependable data throughout your organization. It builds trust in insights and analytics.
Setting up a data governance framework demands a strategic approach and an awareness of potential obstacles. These obstacles include cultural, organizational, technical, and security challenges. Despite these hurdles, the long-term advantages make it a valuable investment for any data-driven entity.
Pillar | Description |
---|---|
Data Quality | Ensures accuracy and reliability of data |
Data Stewardship | Manages data ownership and accountability |
Data Protection and Compliance | Safeguards data and ensures regulatory adherence |
Data Management | Oversees data lifecycle and processes |
By adopting data governance, you prepare your organization for success in the digital era. It enables informed, data-driven decision making, driving innovation and growth.
Key Components of Effective Data Governance
Effective data governance hinges on several essential components that collectively ensure data quality and reliability. Organizations that adopt comprehensive data governance frameworks see marked improvements in data accuracy and decision-making processes.
Policies and Procedures
Data governance policies and procedures are fundamental to a successful data management strategy. They outline the handling, protection, and maintenance of data throughout its lifecycle. By setting clear rules, organizations can cut data security incidents by up to 30% and enhance regulatory compliance by 15%.
Roles and Responsibilities
Data stewardship is crucial for effective governance. Data stewards enforce policies and ensure data quality within specific domains. With the right data governance components in place, organizations can see a 25% increase in data accuracy.
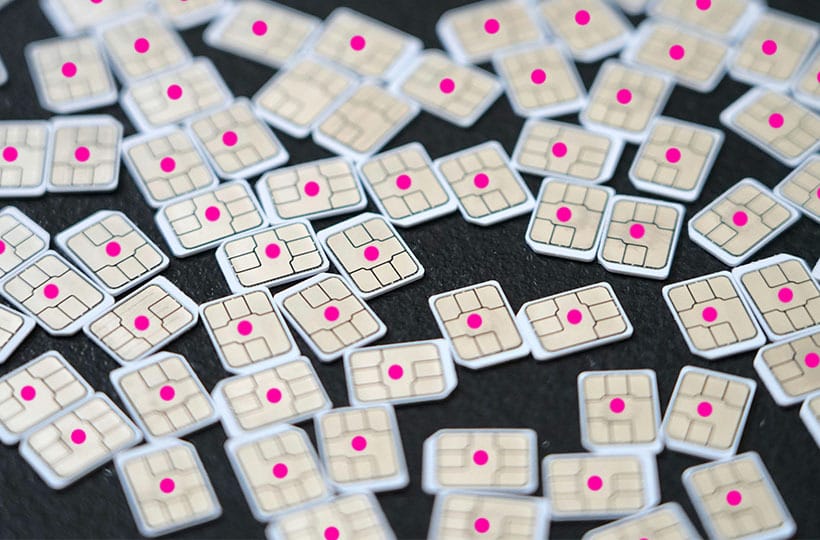
Data Standards and Metrics
Setting clear data standards and quality metrics is vital for maintaining high-quality, accurate, and relevant data. For instance, an e-commerce company might establish data quality metrics like the percentage of missing product descriptions or duplicate customer records. These metrics aid in tracking progress and pinpointing areas for enhancement.
Adopting data governance frameworks can be daunting, with about 40% of organizations initially facing hurdles. It generally takes 6-12 months to build a solid framework. Regular assessments of your data governance program's effectiveness are key. Evaluate its impact on data quality, compliance, and decision-making to foster ongoing improvement.
Data Governance Impact | Improvement Percentage |
---|---|
Data Accuracy Increase | 25% |
Data Security Incident Reduction | 30% |
Decision-Making Process Speed | 20% |
Data Handling Practices | 35% |
Data Quality: The Foundation of Trustworthy Analytics
Data quality management is crucial for trustworthy analytics. Your organization's success depends on the accuracy and reliability of your data. Without strong data quality, your insights may mislead you, leading to poor decisions and significant errors.
Data analytics needs high-quality information to deliver valuable outcomes. By prioritizing data quality, you're investing in your business's future. Companies that use data-driven decisions outperform others, achieving remarkable results and true digital transformation.
Bad data costs companies 12% of their revenue, according to Experian. This fact highlights the need for robust data quality practices. By focusing on accuracy, completeness, consistency, and timeliness, you ensure your data meets its purpose.
Effective data quality management involves:
- Data cleansing and enrichment
- Validation processes
- Regular auditing
- Implementing quality metrics
These practices enhance trust in using data within your organization, from business intelligence to artificial intelligence applications. They also ensure compliance with regulations and protect data privacy, preventing reputational damage and financial penalties.
Data governance and quality management are not just IT concerns - they impact every area of your organization, including legal, risk management, compliance, revenue generation, and security.
By treating data governance as an ongoing, evolving methodology, you establish a refined framework. This framework guides both strategic and technical data questions. It allows IT resources to shift from damage control to strategic initiatives, propelling your business forward.
How Data Governance Enables Data Quality Improvement
Data governance is essential for enhancing data quality. By adopting a comprehensive data governance framework, organizations can notably elevate their data quality. This, in turn, leads to superior business outcomes.
Establishing Quality Standards
Setting clear data quality standards is a cornerstone of data governance. It entails defining what high-quality data looks like for your organization. Metrics such as accuracy, completeness, and consistency are often included in these standards. These benchmarks lay the groundwork for data quality improvement.
Implementing Quality Control Processes
After setting standards, the next step is to put in place data quality control processes. These processes verify that data adheres to the quality criteria before it's utilized for analysis or decision-making. Effective data governance steers the creation and application of these controls.
Continuous Monitoring and Improvement
Data governance facilitates continuous data quality monitoring. This ongoing surveillance enables organizations to swiftly address issues and apply corrective measures. By promoting a culture of continuous improvement, companies can progressively refine their data quality.
Data Governance Impact | Percentage Improvement |
---|---|
Data Quality Confidence | 31% |
Data Analysis Time | 2% |
The statistics underscore the concrete advantages of data governance programs. A 31% increase in data quality confidence and a 2% rise in data analysis time empower organizations to make more informed decisions. This leads to more efficient operations.
"Data governance is the compass that guides organizations towards data quality excellence, ensuring that every piece of information is a valuable asset for decision-making."
Common Challenges in Implementing Data Governance Frameworks
Organizations encounter numerous data governance challenges when setting up frameworks. A survey shows that 54% of respondents have created a data governance framework. However, many still face various data management hurdles.
Poor data quality is a major issue. For instance, customer databases often have 75% blank fields. Vendor tables can accumulate thousands of duplicates, and test applications frequently include hundreds of dummy records.
Siloed data is another significant obstacle. Different approaches to data operations and technology versions often require hybrid databases. These databases combine structured and unstructured data to avoid new data silos.
Talent management is a major concern, with 62% of leaders seeing it as a top challenge in setting up a data governance strategy. Business analysts and BI specialists are often considered for data steward roles. This is because they are familiar with data and interact with technical teams.
Challenge | Percentage |
---|---|
Establishing data management best practices | 58% |
Understanding third-party compliance | 44% |
Dissatisfaction with team skills | 14% |
Dissatisfaction with data governance budget | 13% |
Despite the challenges, organizations see the value in data governance. 66% note improved data security as a benefit, and 39% have seen a decrease in financial risk. To address these issues, 78% plan to increase their data governance investment next year.
Best Practices for Integrating Data Quality into Governance
Data quality integration is vital for effective data governance. By adopting data governance best practices, you can ensure your data remains trustworthy and valuable. Let's delve into key strategies to boost your data management strategy.
Prioritizing Critical Data Assets
Start by focusing on your most crucial data. Pinpoint mission-critical information and shared master data. This focused strategy enables you to leverage your quality efforts effectively.
Automating Quality Checks
Automated data quality tools are vital for maintaining consistency across large datasets. Establish rules to automatically verify data accuracy, completeness, and validity. This method detects issues promptly, thus saving time and resources.
Creating Feedback Loops
Set up channels for data users to report any quality concerns. Regular feedback is crucial for identifying resolving problems, promoting ongoing enhancement in your data environment.
Best Practice | Benefits |
---|---|
Prioritize critical data | Focused improvement, efficient resource use |
Automate quality checks | Consistent monitoring, early issue detection |
Create feedback loops | Continuous improvement, user engagement |
By integrating these practices, you'll establish a strong framework that supports superior data quality throughout your organization. Remember, effective data governance hinges on a balanced approach to quality management and stakeholder alignment.
The Impact of Data Governance on Business Decision-Making
Data governance is crucial for enhancing business intelligence and making data-driven decisions. It sets up clear policies and procedures. This way, organizations can use high-quality data to support their strategic plans.
Good data governance makes sure information is precise, consistent, and easy to get across the company. This helps in making better decisions. It lets businesses quickly adapt to market changes and customer needs.
Data governance's effects on business operations are wide-ranging:
- Improved data quality leads to more reliable analytics and insights
- Streamlined processes reduce time spent on data preparation
- Enhanced compliance minimizes regulatory risks
- Increased data literacy empowers employees at all levels
By setting up strong data governance frameworks, companies turn raw data into valuable assets. This supports current business needs and prepares for future success in a data-driven world.
Aspect | Without Data Governance | With Data Governance |
---|---|---|
Decision Quality | Based on incomplete or inaccurate data | Informed by high-quality, reliable information |
Operational Efficiency | Hampered by data silos and inconsistencies | Improved through streamlined data management |
Compliance | Increased risk of violations | Enhanced ability to meet regulatory requirements |
Innovation | Limited by lack of trustworthy data | Accelerated by accessible, high-quality insights |
As businesses see the value of data-driven decisions, the role of strong data governance will become even more important. By focusing on this, organizations can open up new opportunities and stay ahead in their markets.
Popular Models and Approaches
Data governance models are essential for managing information assets. The Data Management Association (DAMA) framework highlights 10 key functions, with data governance central to them. This framework aids in organizing data management strategies effectively.
The Data Governance Institute (DGI) model is another prominent framework. It focuses on setting clear value statements, goals, and metrics to measure success. This emphasis on measurable outcomes is in line with the SAS Data Governance Framework, which underscores the need to align with business objectives in data governance strategies.
The Boston Consulting Group (BCG) Data Governance Framework presents a distinct viewpoint. It underlines the importance of establishing clear data structures, policies, and tools. BCG's framework also advocates for a detailed Target Operating Model (TOM) for structured governance. This model is beneficial for organizations aiming to overhaul their data management frameworks.
Choosing an appropriate data governance model is vital. Gartner's findings indicate that poor data quality incurs a $12.9 million annual cost for organizations. Therefore, investing in strong data governance strategies is crucial. By adopting these frameworks, you can enhance data quality, minimize risks, and foster better business decisions.
FAQ
What is data governance?
Data governance is a system that controls and manages an organization's data assets. It ensures a unified approach to data management. This system includes practices and processes aimed at standardizing and automating data management.
What are the key dimensions of data quality?
Key dimensions of data quality include accuracy, completeness, consistency, validity, uniqueness, and integrity. These aspects collectively determine the overall quality of data.
Why is the interplay between data governance and data quality important?
The interplay between data governance and quality is vital. Data governance enhances data quality by establishing a framework for collaboration. It clarifies roles and responsibilities, crucial for maintaining data quality.
What are the main benefits of data governance frameworks?
Data governance frameworks boost transparency in data use, availability, and management. They standardize data systems and policies, resolve data issues, and ensure compliance with regulations and organizational standards.
What are the key components of effective data governance?
Effective data governance includes policies and procedures, defined roles and responsibilities, data standards, metrics, lineage tracking, and security measures. These components ensure data is managed efficiently and securely.
Why is high-quality data crucial for organizations?
High-quality data is essential for reliable analytics and informed decision-making. Without it, decisions may be misguided, leading to inefficiencies, reduced trust, and financial losses.
How does data governance enable data quality improvement?
Data governance improves data quality by setting standards, implementing quality control, and ensuring continuous improvement. It fosters a collaborative environment for managing policies and assets, thus maintaining data quality control.
What are some common challenges in implementing data governance frameworks?
Challenges include prioritizing data elements, estimating risk from poor quality, ensuring consistency across systems, maintaining data lineage, and balancing security with accessibility.
What are some best practices for integrating data quality into governance?
Best practices include focusing on critical data assets, automating quality checks, and creating feedback loops for continuous improvement. It's also important to update policies, integrate quality checks into processes, and communicate policies to stakeholders.
How does data governance impact business decision-making?
Data governance significantly influences business decisions by ensuring data accuracy and reliability. It supports strategic choices through trustworthy analytics, increases efficiency by reducing data preparation time, and aids in regulatory compliance.
What are some popular data governance frameworks and approaches?
Popular frameworks include the Data Management Association (DAMA) framework and the Data Governance Institute (DGI) framework. Other approaches involve data governance councils, stewardship programs, and defining roles and responsibilities. Policies, procedures, and data quality metrics are also crucial.
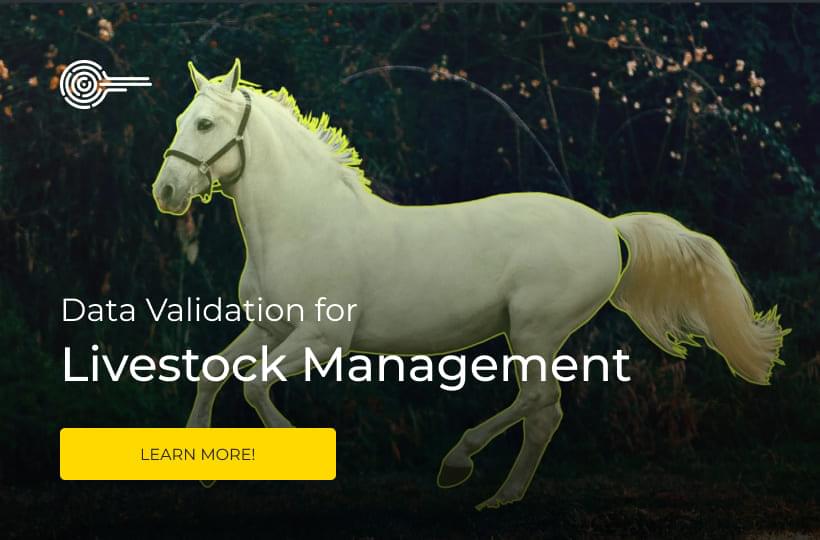