Calculating ROI for Data Annotation: Metrics That Matter
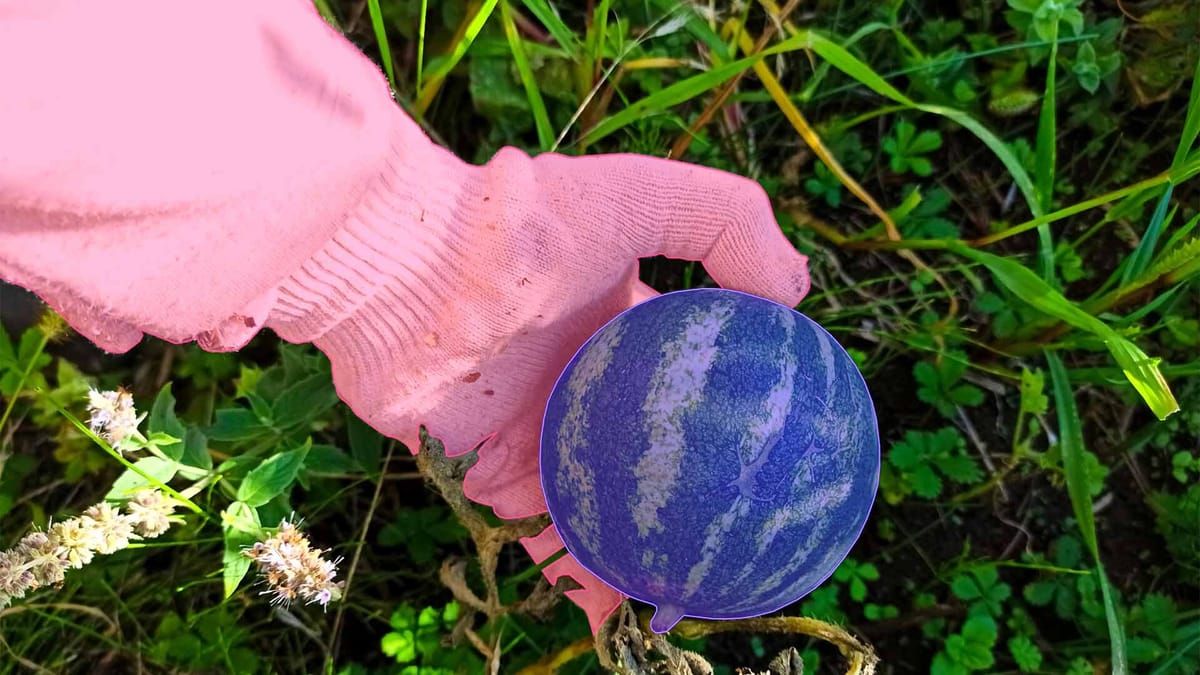
ROI (Return on Investment) in the context of data labeling is an indicator that helps to assess how much the cost of labeling pays off and affects the efficiency of AI and business in general. To estimate ROI correctly, you need to consider the cost and quality of the labeled data.
ROI is calculated as the ratio of benefits to costs. In the case of data markup, costs include the labor of markup engineers and the use of platforms and software. The benefits can come from improved model accuracy, reduced error correction costs, and faster AI training processes.
- Quality of labeling - accurate and relevant labels increase machine learning algorithms' efficiency, reducing the need for additional training iterations.
- Labeling costs - outsourcing to experienced labelers or automated tools can optimize costs and improve results.
- Speed of execution - fast data markup allows companies to implement model updates quickly and reduce time to market.
One of the main problems is the difficulty of assessing the long-term effect of markup. For example, the cost of markup may look high, but if it significantly improves the accuracy of the model, the business will avoid mistakes that could be more costly.
If a company invests $10,000 in data markup and the improved model accuracy reduces recognition errors by 20%, it could result in $50,000 in savings due to reduced data correction costs and improved customer service. The ROI would be positive in this case, as the benefits outweigh the costs.
Key Takeaways
- Tracking and analyzing ROI is essential for confirming the success of data annotation investments.
- AI-driven insights, centralized data, and visualization capabilities are critical solutions to modern challenges.
- High-quality data annotation directly influences the performance of machine learning models and business profitability.
- Quantifying data annotation success ensures alignment with broader business goals and optimal resource allocation.

Understanding ROI in Data Annotation Projects
Return on investment is a financial measure used to evaluate the return on an investment compared to its cost. It is determined by the ratio of costs to efficiency and can be calculated by the formula: (Net income / Total investment) x 100%.
If the ROI is positive, the investment has generated a profit; if it is negative, it has resulted in a loss.
The main costs include annotators' salaries, software costs, and process management. If you need to annotate a large amount of data in a short time, automation and the use of artificial intelligence can help to significantly reduce costs. For example, instead of hundreds of annotators manually annotating material for a month, AI algorithms can do it a hundred times faster. But it's worth remembering that manual annotation is much more accurate and high-quality, so if time is not a critical factor, it's better to go with this approach for complex projects.
By investing in high-quality annotations early on, businesses avoid the cost of correcting errors in the future. This not only reduces model training time, but also increases model accuracy, which ultimately leads to increased efficiency and profitability.
Key Metrics for Evaluating Data Annotation ROI
These metrics help in annotation cost analysis and gauge the overall effectiveness of annotation quality and productivity metrics.
Cost per Annotated Data Point
To find training data, you can find a service provider or use free open source datasets. Open-source datasets are great for small projects or research, but for commercial products, you usually need to create your own.
The price of annotating a single data item depends on its type and complexity. The use of automated tools can reduce costs, and the involvement of experts improves quality but increases cost.
If you need a small amount of data, you can do your own annotation. However, if your data requires more than a few part-time employees to work with, outsourcing is often more efficient. Outsourcing is suitable for projects of all sizes, as setting up complex processes and managing teams can quickly become cumbersome.
Time Efficiency in Annotation
The speed of data annotation depends on a combination of manual work and automated solutions. The use of AI tools can speed up the process by up to 70%, while maintaining accuracy at 85-95%. For example, automatic object recognition in images reduces the markup time from 5-10 minutes to a few seconds. Companies that combine machine learning with human verification can significantly increase productivity while reducing time and resources.
A professional company can achieve 10x the output of in-house annotation without established processes. Factors contributing to ROI include:
- Access to the best tools and expertise
- Lower labor costs in certain regions
- Time savings through assisted annotation and automation
- Scalability and faster team building for large projects
You don’t need to look for tools or train your team on using those tools. So it’s already there, reducing onboarding time.
Another thing to note is that the right tools save you even more. One big example is assisted annotation. We would do this evaluation for you: check where automatic annotation or partly automatic annotation is applicable. Instead of spending hours on a video, you can bring it down to minutes and focus on validating results instead.
Potential Revenue Gains from High-Quality Data
High-quality data has a direct impact on AI’s performance and, as a result, on business results. Correct data partitioning improves model accuracy, reduces errors, and increases trust in technology. As a result, companies gain a competitive advantage, increase revenue, and optimize the cost of developing and implementing AI solutions.
- Improving model accuracy. The more accurate the data partitioning, the better the model recognizes and analyzes information. In autonomous transportation systems, for example, correctly identifying traffic signs and pedestrians reduces the likelihood of accidents, making the technology safer and more in demand. In healthcare, accurate data allows models to identify diseases more accurately, speeding diagnosis and improving patient care.
- Reduced error correction costs. Data errors lead to incorrect predictions and the need to refine models, increasing training algorithms' time and expense. Using high-quality markup tools early in the development process avoids unnecessary costs and speeds up technology adoption in the real world.
- Minimize bias in models. Inadequate or lopsided data can lead to incorrect results. Investing in quality data partitioning helps create fairer and more accurate algorithms, increasing technology confidence and expanding the potential market.
- Increased speed of product development. Well-partitioned data allows models to be trained faster, shortening the development cycle for new solutions.
- Optimize data monetization. Companies with clean and structured data can effectively sell or use it in strategic partnerships. For example, in retail, quality data on shopping behavior allows for more accurate demand forecasting and personalized recommendations, which increases sales.
Financial metrics such as Net Present Value (NPV) are used to evaluate the effectiveness of data processing investments. These metrics help to determine whether the investment in data partitioning is worthwhile and how quickly it will pay for itself.
NPV calculates the difference between the present value of future cash flows generated from quality data and the cost of processing it.
Companies that use these indicators can more accurately forecast financial results, reduce risks, and make better investment decisions.
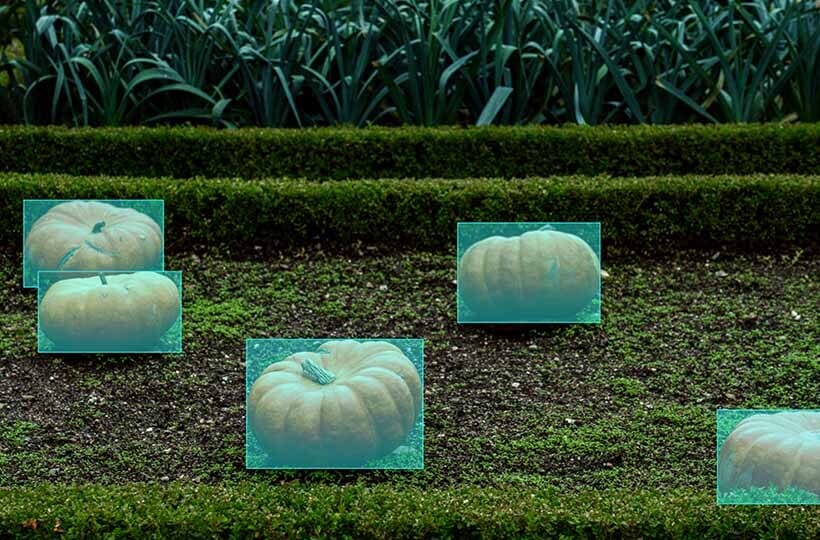
Evaluating Human vs. Automated Annotation
When choosing an approach to data partitioning, companies consider two main options: manual partitioning and automated tools. Both methods have their financial pros and cons that affect the final return on investment.
Pros and Cons of Human Annotation
Manual marking involves specialists who analyze and mark the data manually. This method provides a high level of accuracy but is time-consuming and costly. Advantages:
- High accuracy and contextualized understanding of data.
- Ability to handle complex and ambiguous data.
- Flexibility to adapt to specific business challenges.
Disadvantages:
- High labor costs for specialists.
- Long turnaround time for annotations.
- Possibility of human errors requiring additional verification.
From a financial point of view, manual markup is justified in cases where high accuracy is required, such as in medical diagnostics or legal analysis. However, this method becomes less efficient as processes scale.
Automated Processes
Automated systems use machine learning and AI algorithms to annotate data. They speed up the annotation process and reduce costs but do not always provide the necessary accuracy. Benefits:
- Significant reduction in time and cost.
- Ability to process large amounts of data in short time frames.
- Ease of scaling up as the workload increases.
Disadvantages:
- Possible errors are due to the insufficient accuracy of the algorithms.
- Limited ability to interpret complex and ambiguous data.
- Requires additional validation of results by experts.
Automated partitioning is beneficial when working with large amounts of data, such as in user behavior analysis or image processing for neural networks. However, the need for subsequent validation and possible adjustments must be considered when used.
When to Use Each Approach
The choice between manual and automated annotation depends on the company's specific tasks and financial capabilities. Manual annotation provides high accuracy but requires significant investment, while automated systems reduce costs and speed up the process but may require additional adjustments. In most cases, the best solution is a combined approach that combines the advantages of both methods and strikes a balance between quality and cost.
The Impact of Annotation Quality on ROI
In AI and machine learning, annotation quality is critical for ROI. High annotation data quality ensures models perform well, leading to better results. We'll explore how to measure and boost annotation quality metrics to enhance ROI.
Measuring Data Quality
To ensure the accuracy of annotated data, it is necessary to check its quality regularly. This includes checks and special tools to detect errors and inconsistencies. Clear instructions and controls allow you to quickly find and correct inaccuracies, which improves the entire annotation process.
The involvement of specialists in certain areas and the training of analysts helps improve data labeling accuracy. This allows for more reliable results and reduces the likelihood of errors in future analyses.
FAQ
What metrics are essential for calculating ROI in data annotation?
The cost, time efficiency, and quality assurance metrics provide detailed insight into investment efficiency and profitability.
Why is ROI analysis critical in data annotation projects?
It ensures costs match expected AI efficacy and operational efficiency gains and quantifies success, aligning investments with broader business goals.
How do we measure the cost per annotated data point?
To measure, divide total annotation costs by the number of data points annotated. This metric reveals the process's cost efficiency.
What are the benefits of high-quality annotated data?
High-quality data significantly boosts revenue by improving AI effectiveness and reducing correction costs. It ensures AI models are trained on accurate, reliable data.
How can we optimize the annotation process to improve ROI?
Optimize by choosing the right tools, training workers, and implementing continuous improvement. These strategies enhance cost efficiency and quality.
What are the pros and cons of human annotation versus automated annotation?
Human annotation offers high accuracy and complex data understanding but is time-consuming and costly. Automated annotation is faster and cheaper but may struggle with nuanced data. The choice depends on project needs.
How can budgeting and expense analysis improve the financial perspective of annotation ROI?
Detailed budgeting and expense tracking are essential. They ensure expenditures are justified and aligned with financial goals, boosting ROI.
What should we consider when evaluating data annotation tools?
Evaluate tools based on performance, user experience, and integration capabilities. The right tools significantly impact process efficiency and cost-effectiveness, affecting ROI.
How do we measure the quality of annotated data?
Quality is measured through accuracy, consistency, and completeness metrics. Regularly assessing these ensures high-quality AI models.
What are some examples of successful data annotation implementations?
Case studies showcase best practices and successes across industries. They offer valuable insights and benchmarks for improving strategies and ROI.
How can we leverage emerging technologies to enhance ROI in data annotation?
Staying updated with AI and data annotation tech advancements, like predictive analytics and automation, offers competitive advantages. These technologies redefine cost and quality benchmarks, improving ROI.
What continuous assessment practices help sustain improvements in annotation ROI?
Continuous improvement, regular quality assessments, and technology adaptation are key. These practices ensure ongoing optimization, reducing corrections, and sustaining ROI improvements.
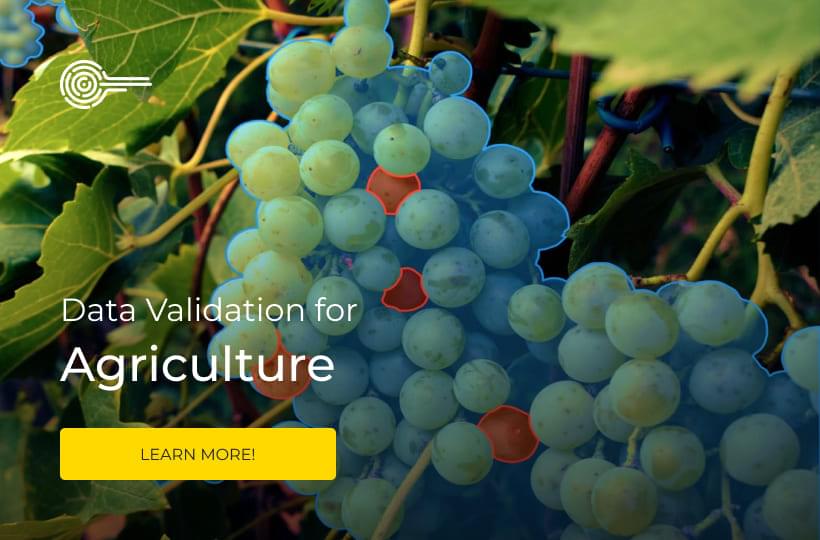