Polyline Annotation and Automated Vehicles
Automated vehicles promise to transform the way we travel in the coming years. This technology is already present in numerous products and is increasingly being adopted by large corporations. However, substantial challenges still remain before automated vehicles become a mainstay for consumers across the globe.
Machine learning algorithms that drive automated vehicles are able to interpret and navigate their environment because they are trained with annotated video and image data. Human operators add information to images, these labels enable computer vision AI to contextualise the roads and traffic in real time.
This blog will focus on one particular annotation technique that is essential for automated vehicles: polyline annotation. We will define it and reveal the exciting capabilities that it enables. Achieving high quality annotation can be a challenge for developers in this industry. We will show how annotation experts, like Keymakr, can give innovators an edge.
What is polyline annotation?
This technique is used to define linear structures in images and video. It uses small lines, connected at vertices, to trace the shape of structures like roads, rail tracks, and pipelines. Annotators apply these lines to images using annotation platforms and labeling tools. For video training data, these lines must be picked out in each individual frame.
How polyline annotation supports automated vehicles
Polyline annotation allows machine learning models for automated vehicles to locate themselves within the large context of the road system. This technique enables the following critical functionalities:
- Lane detection: This is a core capability for all autonomous vehicles because it keeps them centred in the correct part of the lane. It also gives computer vision models information on where to move next when navigating across multi-lane highways. Lane detection is reliant on polyline annotation to accurately delineate the road markings that are relevant to AI models.
- Navigating traffic laws: Different road markings indicate different traffic laws. It is vital for automated vehicles to distinguish between accessible lanes and emergency stopping lanes for example. Polyline annotation can create training data that highlights appropriate road markings with critical information.
- Avoiding obstacles: Lane annotation is crucial for vehicle safety. It can help autonomous vehicles to avoid collisions and obstacles by keeping them safe in designated lanes. Deviating from properly marked areas of the road runs the risk of collisions with median strips or vehicles in stopping lanes.
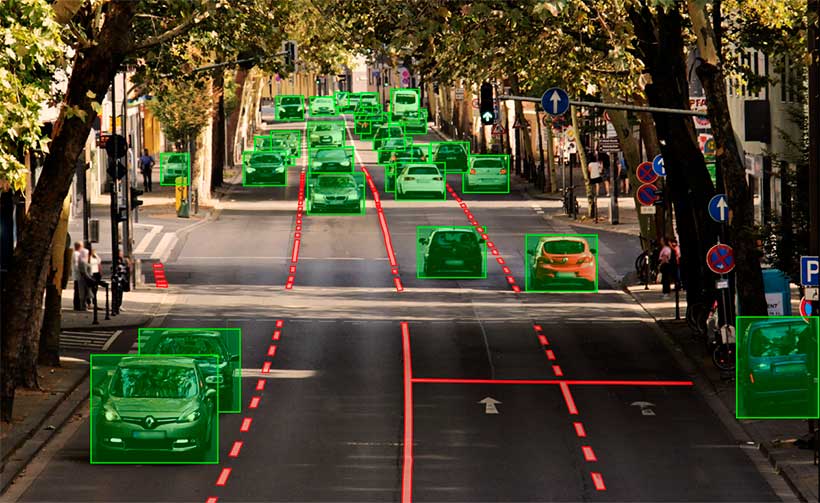
How to access the best polyline annotation
Polyline annotation is supporting one of the fastest growing sectors of the digital economy. This time consuming and detail oriented work can be difficult for technology companies to manage in-house. Conversely crowd sourcing solutions can result in error-strewn datasets. Keymakr is a professional annotation provider that can offer key advantages to autonomous car developers:
- Overcoming bias: Bias in training data can lead to significant problems when computer vision models operate in the real world. Images for machine learning need to reflect a variety of locations, weather conditions, and light levels. Models designed to work with one country's road conditions and lane markings might not function as well in other environments. Keymakr has experience creating varied datasets that help mitigate bias. Data creation facilities allow developers to craft bespoke annotated images that fit niche requirements.
- Annotation tools: Outsourcing to an annotation service like Keymakr means that AI companies can take advantage of proprietary annotation technology. Keymakr’s platform has a number of unique features that make project management and verification much more straightforward for busy developers.
- Video annotation: Adding polyline annotation to video is a significant challenge due to the increased workload created by multiple frames. Keymakr’s workflow and job allocation procedures allow multiple annotators to label video at the same time, whilst maintaining consistency.
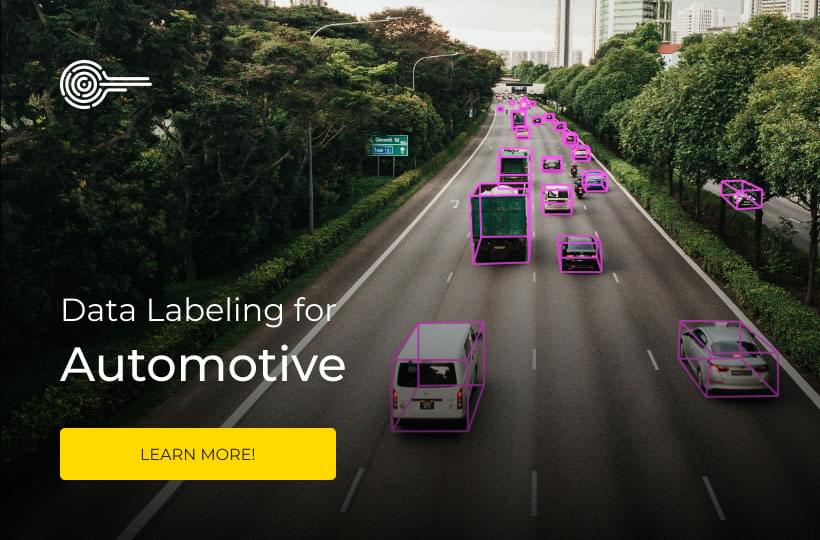
Keymakr is providing precise annotation at the scales you need.