Vertical farming - a checklist to help you start a successful project
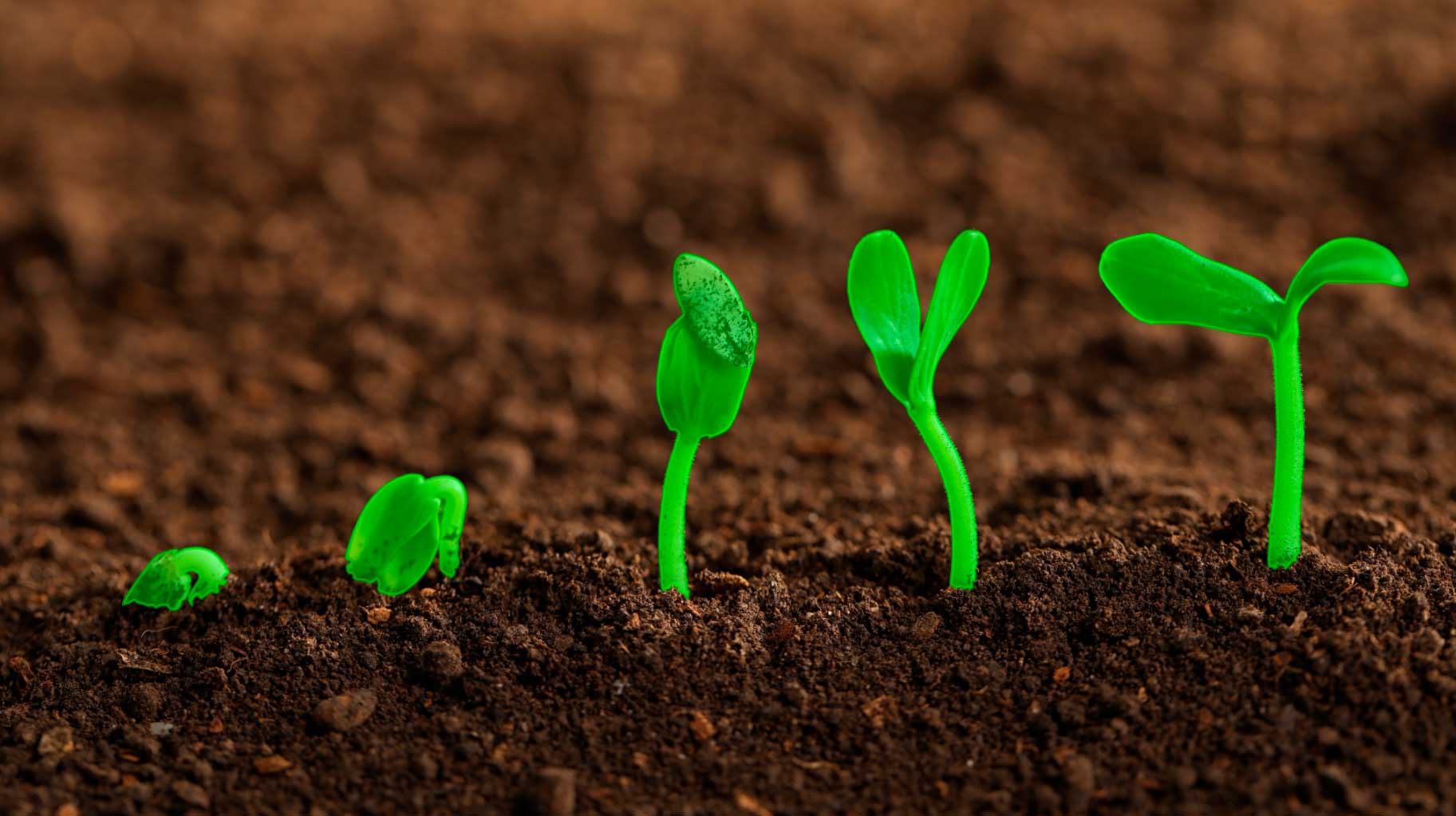
Vertical farming has the potential to transform the efficiency and sustainability of agriculture. Vertical farming is the practice of growing crops vertically in stacked layers, indoors. This approach has a number of advantages. Vertical farms have increased crop yields with a far smaller unit area of land requirement. Indoor crops are also protected from weather conditions in a stable, controlled environment.
And, due to its limited land usage, vertical farming is less disruptive to local ecosystems and native plants and animals. This form of agriculture has always been at the forefront of innovation, making use of soilless farming techniques such as hydroponics and aeroponics. And now leaders in this sector are using computer vision AI models to further automate and optimize vertical farming.
Today's blog will look at some of the key factors that vertical farming AI teams need to consider, including how to access high quality annotated training data.
Growth monitoring
Assessing plant growth is an essential part of increasing vertical farm efficiency and improving yields. By continually assessing the rate of plant growth farmers are able to intervene when crops are developing sub-optimally. AI systems are capable of monitoring plant growth autonomously.
Round the crop surveillance of crop development means more data for growers and more chances to protect yields. Polygon image annotation captures the irregular shape of plants in training images and is a vital part of creating training data for growth monitoring models.
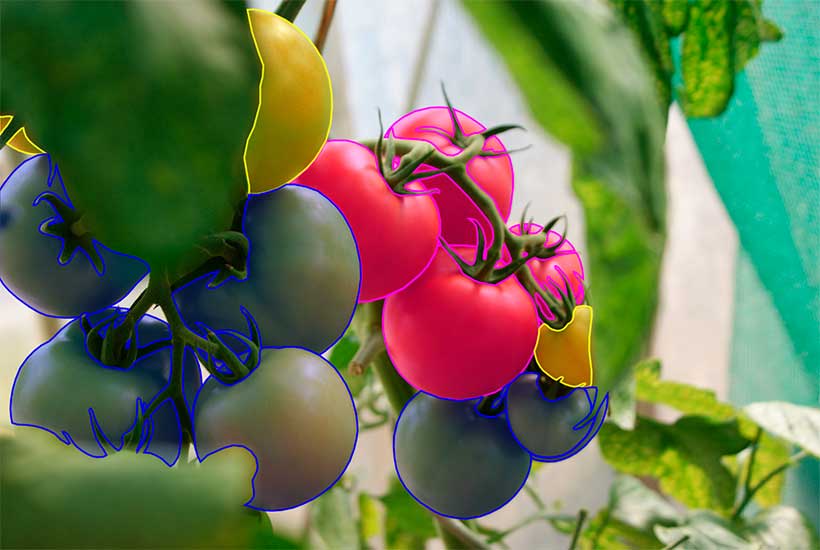
Ripeness detection
It is important that fruits and vegetables are picked at the optimal level of ripeness. Picking too early can lead to poor flavor and picking too late can reduce the shelf life of produce. AI models can analyze the color of fruiting plants and provide an assessment of ripeness levels and classify growth stages.
Growers can use this information to harvest produce at the right time which in turn helps to improve product and profitability. Semantic segmentation annotation allows AI developers to precisely locate fruits and vegetables in images. This data can be used by ripeness detection AIs to accurately monitor growing plants.
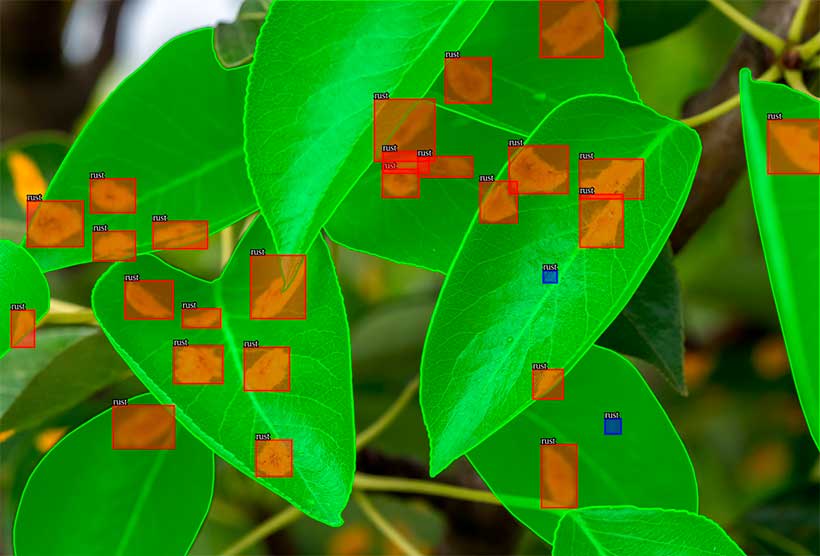
Automated planting, seeding and harvesting
Vertical farms are ideally suited to make use of automated farming systems. AI models, working in tandem with sophisticated robotics, can plant and seed vertical farm terraces throughout the year, increasing the efficiency of vertical farms and allowing them to feed more people in the future. Annotated video data may help to improve the performance of automated harvesting systems.
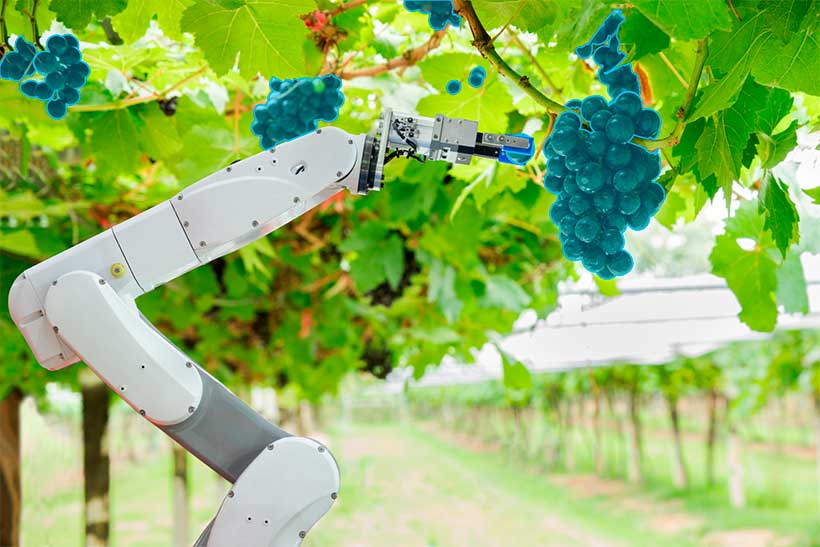
Crowdsourced vs in-house annotation teams
High-quality image and video annotation can help vertical farming AI projects to reach their potential. However, it can be difficult to find reliable data annotation services that scale. Crowdsourced annotations can be a cheap option but they can also be more prone to errors and a lack of precision.
Keymakr is a data annotation provider with a track record of producing reliable datasets. Keymakr uses an in-house team of experienced annotators to create labeled images and video for AI training. This team is led by experienced managers who can respond quickly to troubleshooting and changes in data needs.
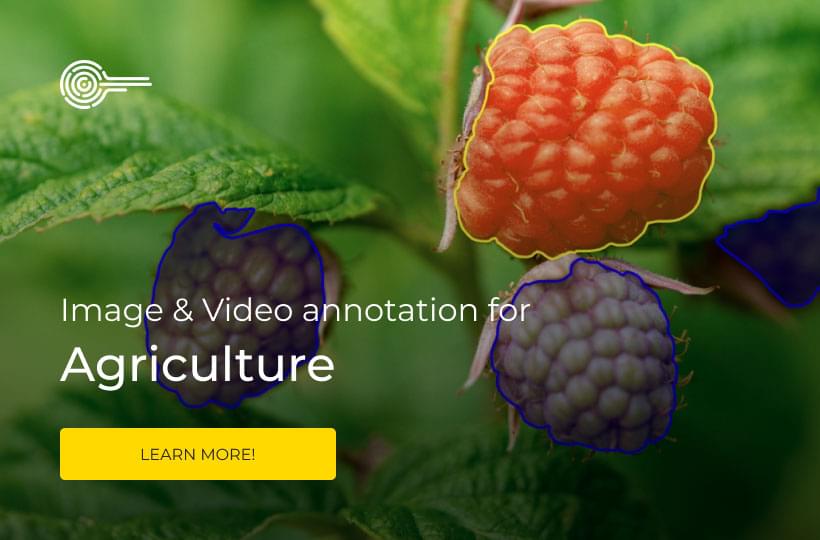
Effective annotation tools
The right annotation tool can transform a difficult labeling project. Vertical farming AI teams can benefit from innovative annotation platforms that streamline labeling. Keymakr’s tool is designed to produce an efficient annotation workflow. By linking annotators and verifiers Keymak’s tool ensures that projects are finished on time and with a high level of accuracy.
Contact a team member to book your personalized demo today.