Privacy and Security Benefits of Synthetic Data
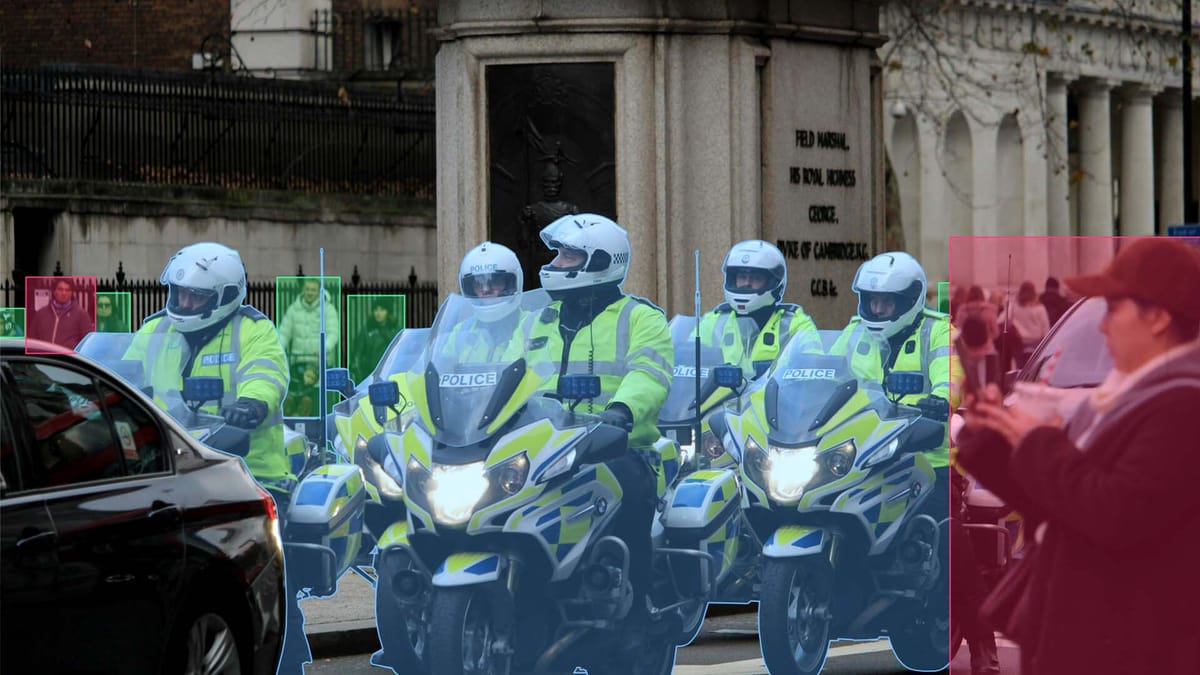
In a world where 85% of customers are unwilling to engage with companies that neglect data privacy, synthetic data presents a significant advantage. This artificially created data mimics real-world data, removing personally identifiable information and boosting anonymization techniques. It's not merely about safeguarding data; it's about fostering trust in a digital era where 75% of consumers will abandon a brand following a cybersecurity breach.
Synthetic data privacy is transforming how businesses manage sensitive data. By generating artificial datasets that replicate real data patterns, companies can test and develop systems without exposing actual customer data. This method not only strengthens data privacy but also unlocks new opportunities for innovation and expansion across sectors.
Exploring synthetic data further, you'll see how it's poised to redefine data management. It offers a secure, compliant, and efficient solution for numerous applications. From healthcare to finance, the scope of its use is extensive and expanding.
Key Takeaways
- Synthetic data eliminates personally identifiable information, boosting privacy.
- 85% of customers prioritize businesses that value data privacy.
- Annual data breach costs are projected to surpass $5 trillion by 2024.
- Synthetic data helps companies comply with data protection regulations.
- This technology offers a balance between data utility and privacy protection.
- Synthetic data is applicable across various industries, from healthcare to finance.

Understanding Synthetic Data in the Modern Digital Landscape
Definition and Characteristics of Synthetic Data
Synthetic data is artificially created, mirroring real-world data. It's crafted through sophisticated algorithms, devoid of personal details.
How Synthetic Data Differs from Real Data
Synthetic data stands out because it allows for full control over its characteristics. You can tweak everything from event frequency to noise levels. This adaptability makes it perfect for a wide range of uses, from software testing to financial modeling.
Aspect | Real Data | Synthetic Data |
---|---|---|
Privacy Risk | High | Low |
Cost | Expensive | Cost-effective |
Scalability | Limited | Highly scalable |
Customization | Difficult | Easily customizable |
The Role of AI in Generating Synthetic Data
AI is essential in creating synthetic data. Tools like Generative Adversarial Networks (GANs) and statistical models generate realistic datasets. These AI-driven approaches ensure the synthetic data retains real data's statistical properties while protecting privacy.
It's forecasted that 60% of data for AI and analytics will be synthetically produced by 2028. This trend highlights synthetic data's growing role in safeguarding data while fostering innovation across sectors.
Benefits of Synthetic Data for Privacy and Security
Synthetic data brings significant benefits for privacy and secure data management. As more organizations adopt it, its role in protecting data becomes clearer.
Elimination of Personally Identifiable Information (PII)
Synthetic data generation removes PII from datasets. It creates data that's statistically similar but doesn't include real personal information.
Enhanced Data Anonymization Techniques
Synthetic data offers better privacy than traditional anonymization methods. It keeps data relationships intact without linking to individual identities. This makes it easier to share and collaborate on data while keeping it confidential.
Reduced Risk in Data Breaches
Using synthetic data greatly reduces the risk of data breaches. With no real personal information, the damage from unauthorized access is minimal. This is a key aspect of secure data practices for companies handling sensitive data.
Compliance with Data Protection Regulations
Synthetic data helps meet regulatory needs like GDPR. It lets organizations work with data that looks like real-world statistics without risking individual privacy. This benefit is vital for industries like healthcare, finance, and tech, allowing for innovation while following strict data laws.
Aspect | Benefit |
---|---|
PII Elimination | 100% removal of personal identifiers |
Data Sharing | Unrestricted, safe collaboration |
Breach Impact | Significantly reduced risk |
Regulatory Compliance | Easier adherence to GDPR, CCPA |
Synthetic Data as a Solution for Legal Compliance
Synthetic data stands out as a robust solution for GDPR compliance and data privacy. It enables organizations to navigate complex legal terrains while driving innovation.
GDPR, CCPA, and Other Data Protection Laws
Regulations like GDPR and CCPA are designed to protect personal data. Synthetic data meets these standards by removing the need for real personal data. The EU AI Act also highlights the critical role of privacy in AI systems.
Regulation | Max Fine for Non-Compliance | Key Requirement |
---|---|---|
GDPR | 4% of annual turnover | Data minimization |
EU AI Act | 7% of annual turnover | Strict data quality criteria |
CCPA | $7,500 per violation | Consumer right to opt-out |
Mitigating Legal Risks through Synthetic Data Use
Synthetic data provides a secure option for handling sensitive data. It enables the development of AI models without infringing on individual privacy. This method greatly diminishes the risk of data breaches and the legal repercussions that follow.
Employing synthetic data allows companies to innovate without breaching GDPR. It's a solution that benefits both consumer rights and business interests in the ever-changing digital world.
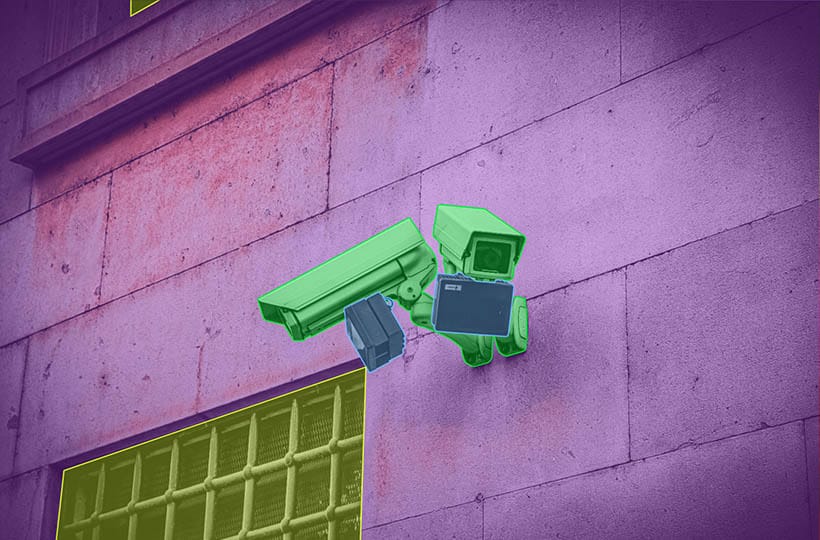
Building Consumer Trust with Synthetic Data Practices
Synthetic data lets businesses analyze trends and predict outcomes without needing specific customer details. This privacy-preserving data method is highly valuable in retail, finance, and insurance. It keeps customer trust high while allowing for performance measurement and prediction.
Here's how synthetic data builds consumer trust:
- Eliminates the need to use real customer data for analysis
- Reduces the risk of exposing sensitive information
- Enables compliance with strict data protection regulations
- Allows for innovative product development without privacy concerns.
This forecast shows synthetic data's growing role in building trust and driving innovation. As consumers grow more concerned about data security, companies using synthetic data will likely have a competitive advantage.
Industry | Synthetic Data Application | Trust-Building Benefit |
---|---|---|
Healthcare | Patient data analysis | Protects sensitive health information |
Finance | Fraud detection | Enhances security without exposing real transactions |
Insurance | Risk assessment | Maintains policyholder privacy |
By embracing synthetic data, businesses demonstrate their commitment to safeguarding customer information. This approach can greatly reduce the negative effects of data breaches on brand reputation and customer loyalty. It fosters long-term trust in our increasingly data-driven world.
Practical Applications of Synthetic Data in Various Industries
Synthetic data privacy and secure data practices are transforming industries across the board. Let's explore how different sectors are leveraging this technology to enhance their operations while safeguarding sensitive information.
Healthcare and Patient Data Protection
In healthcare, synthetic data is revolutionizing patient data protection. Hospitals and research institutions can now conduct studies without compromising individual privacy. For instance, synthetic patient records allow for in-depth analysis of treatment outcomes and disease patterns while keeping real patient information secure.
Financial Services and Sensitive Transaction Data
Banks and financial institutions are using synthetic data to bolster their security measures. By creating artificial transaction datasets, they can test fraud detection systems and develop new financial products without risking real customer data. JPMorgan's synthetic data sandbox exemplifies this approach, accelerating proofs of concept with third-party vendors.
Tech Industry and User Behavior Analysis
Tech companies are harnessing synthetic data for user behavior analysis and product testing. This allows them to refine features and improve user experience without infringing on privacy. Social networks use synthetic data to enhance content filtering systems and combat online threats, ensuring a safer digital environment.
- Autonomous vehicle development, enabling thousands of simulations
- Software testing, reducing wait times and increasing agility
- Quality assurance processes, improving anomaly detection
- Cloud machine learning pipelines, improving data security
As industries continue to adopt synthetic data, we're seeing a shift towards more secure, efficient, and innovative data practices across the board.
Overcoming Challenges in Synthetic Data Implementation
Implementing synthetic data presents unique hurdles in data privacy and accuracy. Businesses aim to safeguard sensitive information while ensuring synthetic dataset quality. This delicate balance is essential for data utility and privacy protection.
Ensuring Data Quality and Accuracy
Synthetic data privacy relies on datasets that accurately reflect real-world scenarios without revealing individual identities. Assessing synthetic data quality involves verifying its accuracy, consistency, and completeness. Tools like Cleanlab aid in data profiling, ensuring synthetic data retains the statistical properties of original data.
Balancing Privacy with Data Utility
The challenge is to create synthetic data that's both useful for analysis and protects privacy. Higher accuracy might inadvertently include personal attributes. Synthetic Data offers a solution by providing artificial, realistic data for AI model training without compromising individual privacy.
Addressing Potencial Biases
Bias in synthetic data can result in inaccurate models. It's vital to use diverse data sources to enhance diversity and coverage. Continuous monitoring of synthetic datasets is necessary to prevent drift from intended characteristics. Model audits are critical for uncovering biases, measuring accuracy, and error rates.
The synthetic data generation market is expanding rapidly, expected to hit $2.1 billion by 2028. This growth highlights the escalating demand for privacy-preserving data solutions across various sectors. As synthetic data adoption increases, addressing these challenges is vital for maintaining reliable and ethical data practices.
Future Trends in Synthetic Data and Privacy Protection
The future of data security and privacy is looking up with synthetic data leading the way. Industries are rapidly adopting this innovative method, seeing its benefits across various sectors. By 2030, synthetic data is expected to fully replace real data in machine learning, marking a significant shift in handling sensitive information.
The global synthetic data market is growing rapidly, projected to reach $2.1 billion by 2028 from $381.3 million in 2022. This growth rate of 33.1% shows the increasing need for privacy-focused data solutions.
Cloud-based synthetic data solutions are becoming more popular, with 89% of tech decision-makers seeing them as essential for staying competitive.
"Synthetic data will surpass real data in AI model usage by 2030, fostering innovation across industries."
Looking ahead, we can expect more advanced privacy-preserving methods, such as differential privacy, to improve synthetic data security. These advancements will be vital in overcoming data quality, bias, and practical limitations in synthetic data use.
Year | Projected Adoption | Key Focus Areas |
---|---|---|
2025 | 70% of enterprises | AI and analytics |
2028 | $2.1 billion market | Privacy and compliance |
2030 | 85+% in machine learning | Near-complete real data replacement |
The future of synthetic data holds promise for enhanced privacy, reduced bias, and cost savings across industries. As we move forward, finding the right balance between data utility and privacy protection will be essential. This balance will unlock synthetic data's full value in our increasingly digital world.
Summary
Synthetic data is revolutionizing data privacy and security. It offers companies advanced analytics without exposing user information. This is vital in sectors like finance and healthcare, where data protection is essential.
The benefits of synthetic data go beyond privacy. It accelerates insights generation, a process that often takes months with real data. Training machine learning models becomes more effective and cost-efficient. Synthetic data is also more affordable than real data, making it appealing to various industries.
Despite challenges in ensuring reliability and eliminating bias, efforts to develop standardized frameworks are underway. As these frameworks evolve, synthetic data adoption will likely increase across industries. The future of data privacy looks bright, with synthetic data leading the way in balancing innovation and protection in our digital world.
FAQ
What is synthetic data?
Synthetic data is artificially created data that mimics real-world data. It uses advanced algorithms and statistical models. This data doesn't contain information about real individuals.
How does synthetic data enhance data privacy and security?
Synthetic data removes personally identifiable information (PII) by generating datasets that mimic real data without personal details. This anonymizes data, making it impossible to trace back to individuals. It significantly reduces the risks of data breaches.
Why is data privacy and protection important?
Data privacy and protection are vital for legal compliance with regulations like GDPR, CCPA, HIPAA, and PDPC. Data breaches can lead to heavy fines, loss of consumer trust, and damage to brand reputation. In fact, 85% of customers are unwilling to do business with firms that don't prioritize data privacy.
How does synthetic data help with regulatory compliance?
Synthetic data meets strict privacy regulations like GDPR, CCPA, and HIPAA. It allows organizations to work with data that doesn't contain actual personal information. This significantly reduces legal risks associated with data handling and sharing.
Can synthetic data build consumer trust?
Yes, synthetic data can build consumer trust by showing a commitment to data privacy and protection. Consumers are more likely to trust brands that prioritize their data security and take proactive steps to protect customer information.
What are some practical applications of synthetic data?
Synthetic data has practical applications in healthcare for patient data protection, in financial services for sensitive transaction data, and in the tech industry for user behavior analysis and product testing. It can also be used to generate synthetic test data for application development and explore "what-if" scenarios.
What challenges are associated with synthetic data implementation?
Challenges include ensuring data quality and accuracy, balancing privacy with data utility, and addressing biases in synthetic data generation. This ensures fair and reliable outcomes in machine learning models and analytical insights.
What are the future trends in synthetic data and privacy protection?
Gartner predicts synthetic data will replace real data in machine learning by 2030. Advancements in AI and machine learning will improve synthetic data quality and utility. There's a growing focus on developing privacy-preserving techniques, such as differential privacy.
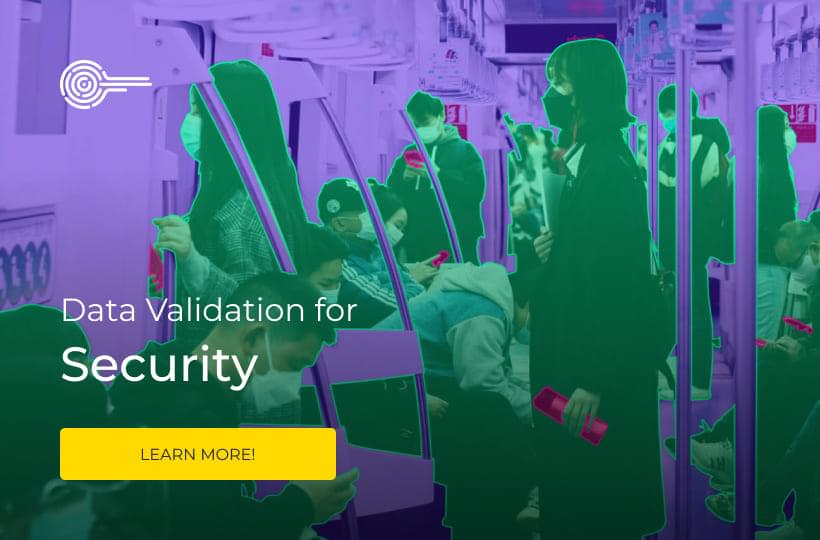