Image and Video Annotation for Agriculture and Livestock Management
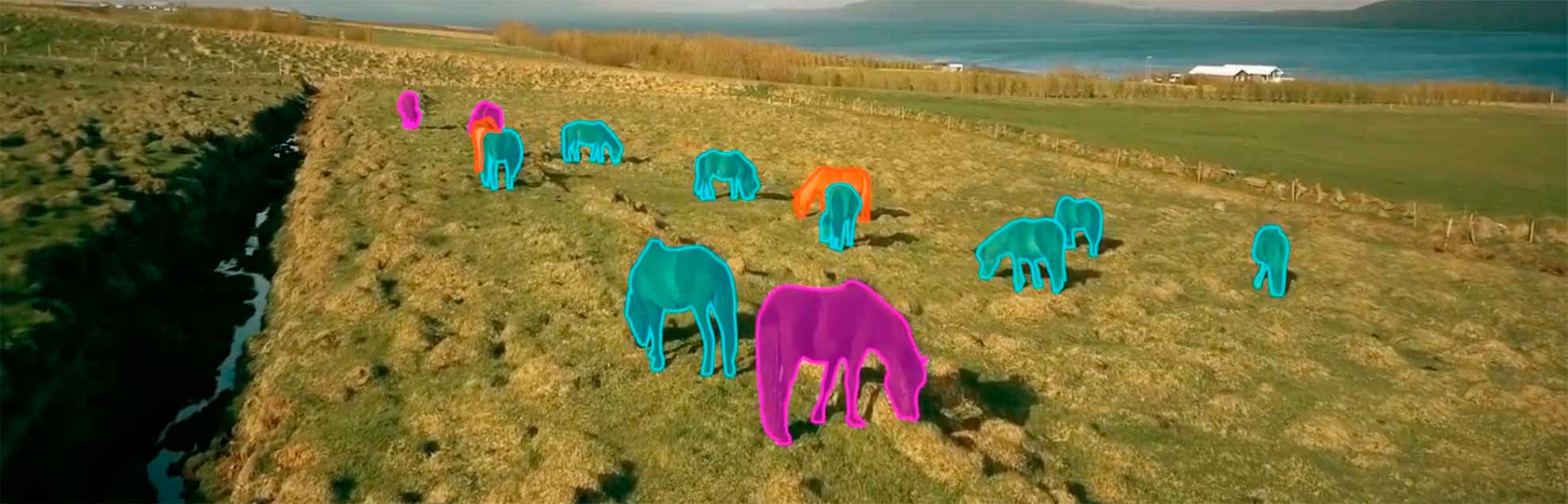
Artificial intelligence has transformed industries. From retail to agriculture, high-precision algorithms drive productivity and better decision-making. But how does it work?
What enables deep learning algorithms to understand our day-to-day business operations better than we do? To answer this question, we need to talk about a technique known as data annotation.
But first, how exactly have farmers joined forces with AI?
Machine Learning in Agriculture: Smart Farming Technologies
The use of AI in agriculture has exploded. Smart agriculture, otherwise known as smart farming, now deals with the complete cycle of growing and harvesting. From the moment a seed is planted in the soil, computer vision is already involved.
Here are four different ways in which AI now powers agriculture and livestock management.
- Selective Breeding
When it comes to selective breeding, even the most experienced farmers pale in comparison to deep learning algorithms. Trained algorithms make predictions based on decades of field data, including crop performance in various climates and plant characteristics that have emerged over time.
The result? A probability model that determines which genes are most likely to confer beneficial traits.
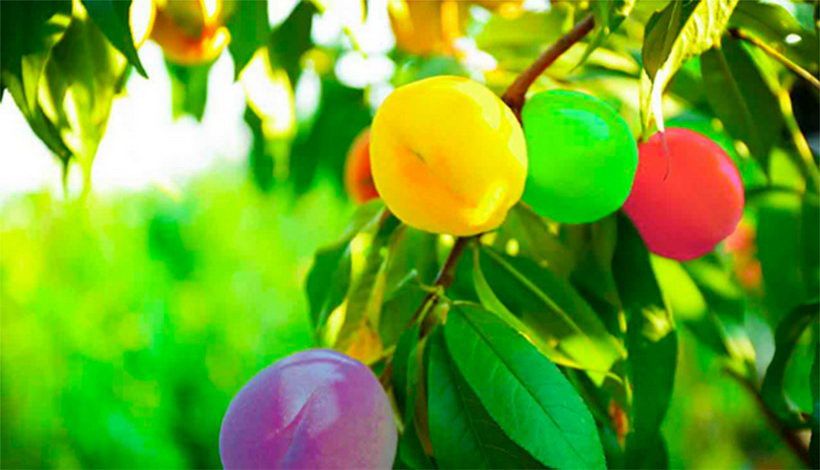
2. Soil and Water Management
Perhaps the most useful machine learning algorithm in smart farming is the one responsible for monitoring field conditions. Evaporation and evapotranspiration processes are difficult to predict — that is, until deep learning.
Machine learning algorithms study daily dew point temperatures, soil moisture recordings, temperature fluctuations, and other factors. Their predictions allow farmers to make the best use of irrigation systems, prepare for unexpected weather conditions, and optimize soil conditions year-round.
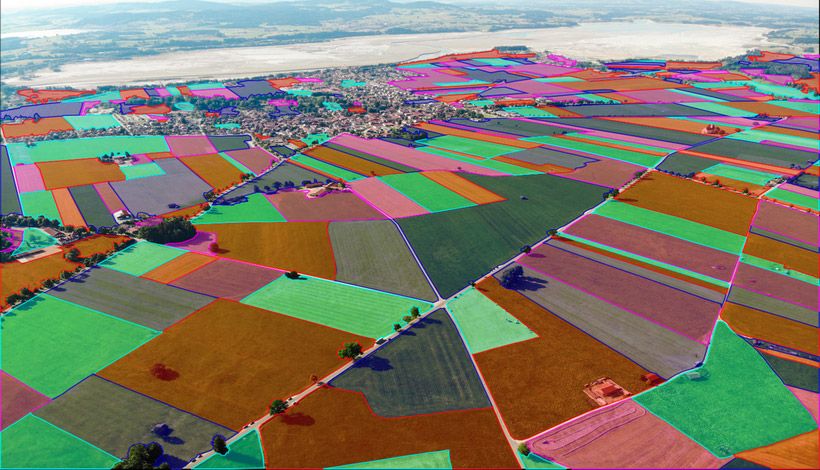
3. Yield Prediction
Computer vision keeps an eye on just about everything farmers usually watch over: crops, livestock, weather patterns — even economic conditions. Real-time monitoring combined with data-driven analytics generates yield predictions that go far beyond any analysis a human could perform.
On a related note, machine learning algorithms are always watching for weeds, maximizing crop yields. In the future, weed removal is likely to join forces with robotic process automation, minimizing the harmful side effects of herbicides.
4. Livestock Management
Machine learning has evolved to monitor livestock production systems, optimizing their efficiency wherever possible. For example, weight predicting algorithms can predict an animal’s weight 150 days from now, allowing farmers to make diet adjustments far ahead of time.
Classifiers for animal behavior monitor their movement patterns to determine how much stress an animal may be experiencing. Stress predictions guide further predictions regarding disease susceptibility, weight loss, and other related effects.
Agriculture Datasets for Machine Learning
Machine learning powers smart agriculture. But what powers machine learning? That’s where image and video annotation come into play.
Without data annotation, computer vision would still be out of reach. Regardless of whether we’re talking about healthcare, retail or agriculture, deep learning algorithms require vast amounts of labeled images and videos in order to interpret the world around them.
So, how is data annotation used in smart agriculture?
- Crop detection. Annotated training datasets teach machines to recognize and distinguish between endless variations of fruits and vegetables.
- Crop maturity. Labeled images and videos of plants at different stages of maturity teach machines to identify and alert farmers when it’s time to harvest.
- Weed removal. Weeds monopolize minerals and nutrients, damaging crops and decreasing yield. Annotation helps machines flag and remove unwanted plants.
- Health monitoring. Training datasets involving plants and livestock in various states and conditions allows machines to determine disease probability, stress levels, and more.
In a nutshell, image and video annotation make objects recognizable to machines. Insufficient training data, or data that has been improperly labeled, throw a wrench in any machine learning model — regardless of how robust its algorithms are.
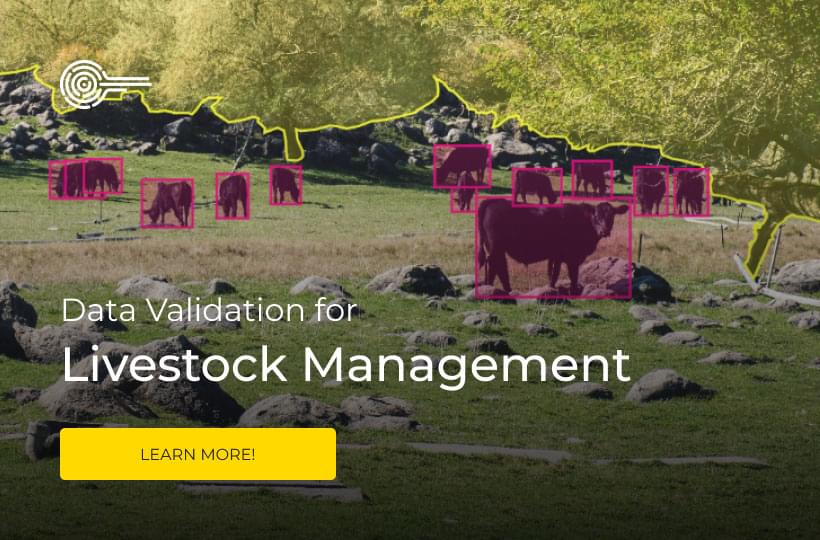
Outsource High-Quality Image and Video Annotation
Computer vision has taken root across industries, empowering better decision-making and maximizing productivity. But the business potential of any machine learning model relies on its ability to make accurate predictions.
Without high-quality data annotation, accuracy levels suffer. That’s why AI companies rely on professional data annotation services like Keymakr.
Delivering the pixel-perfect training dataset your machine learning model craves. Get in touch with a member of our team to schedule your personalized demo today.