How Robotics AI Developers Handle Bias
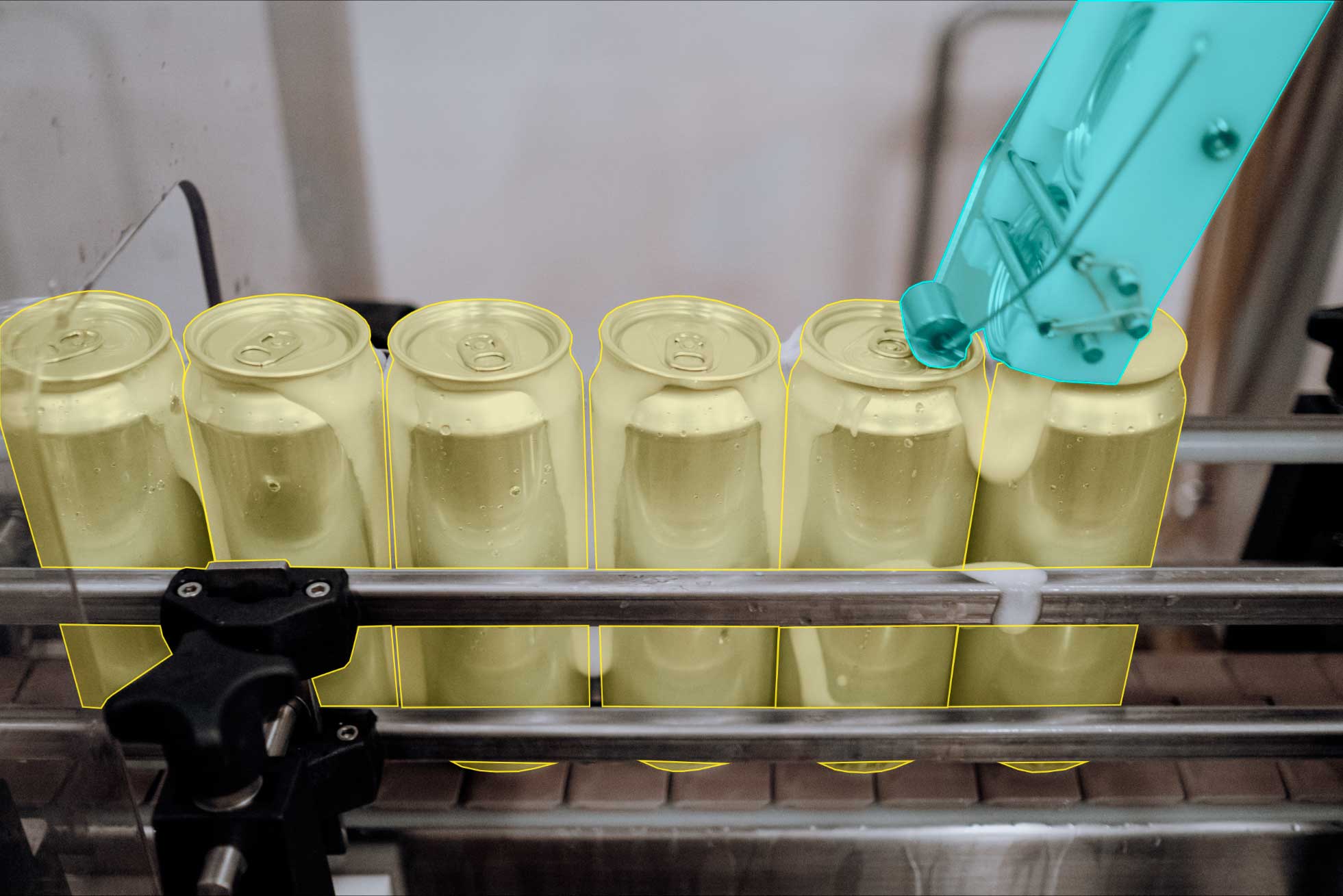
Robots are deployed at every point of the modern manufacturing process. Robots help ensure that products are put together correctly and that the assembly line is safe and efficient. However, further improvements are possible with the help of AI. Automated robotic systems have the capacity to act intelligently in a dynamic factory environment.
As a result a variety of AI use cases are being developed in this industry. Start data annotation is key to the continued success of these applications. Data annotation providers, like Keymakr, can help robotics AI developers to overcome the problem of biased training data.
This blog will first look at the promising potential use cases for AI in this sector. Secondly we will address some specific bias challenges. And finally got we show how AI innovators can access high quality, varied training data.
Exciting potential
AI powered robots can operate autonomously in factories and on assembly lines. This ability to act independently opens up a range of possible use cases:
- Environmental sensing: It is important that AI robot systems can navigate safely in fast moving factory settings. Autonomous machines need to be able to plan a route and avoid obstacles. Image and video annotation allows robots to identify objects and move around them. As a result the assembly line is kept safe and efficient.
- Inventory and logistics: Smart robots can monitor inventories and sort products to optimise storage space. AI also has the potential to revolutionise logistics. For example robots can sort packages for delivery and separate products and other materials.
- Quality control: When examining products for quality control it can sometimes be hard for humans to spot small defects. AI systems can be trained to identify tiny errors that could have a big impact on overall product quality. To achieve this capability AI models are trained with thousands of annotated training images.
- Predictive maintenance: AI systems are capable of spotting tiny flaws. This ability is extremely useful for equipment maintenance. AI models are trained with images of machinery. This allows them to identify wear and tear, and alert engineers who can act to protect valuable machinery.
Complex Environments
Factories and assembly lines are dynamic and chaotic environments. Poorly functioning AI models could be a risk to human safety and operational efficiency in these settings. It is therefore essential that computer vision based AI systems are trained with accurate, unbiased image and video data
Specific bias challenges
Developers in this sector face a number of bias difficulties when constructing training datasets:
- Image and video quality: Poor quality images and video can impair the performance of AI models. AI developers can overcome this by collecting and creating high quality training data that reflects real world conditions.
- Diverse manufacturing locations: Manufacturing conditions can vary substantially, even within the same country. AI models trained to recognise a particular set of signs or assembly line layouts may not function as well in a different setting. Diverse training data can help to overcome this.
- Cultural differences: Manufacturing standards and practices can also be very different in different countries. AI systems must be able to work effectively in different manufacturing cultures.
Find the right data annotation provider
Overcoming these bias issues can be difficult for any AI company. Keymakr is an annotation provider with experience collecting varied datasets and creating new images and video for AI training.
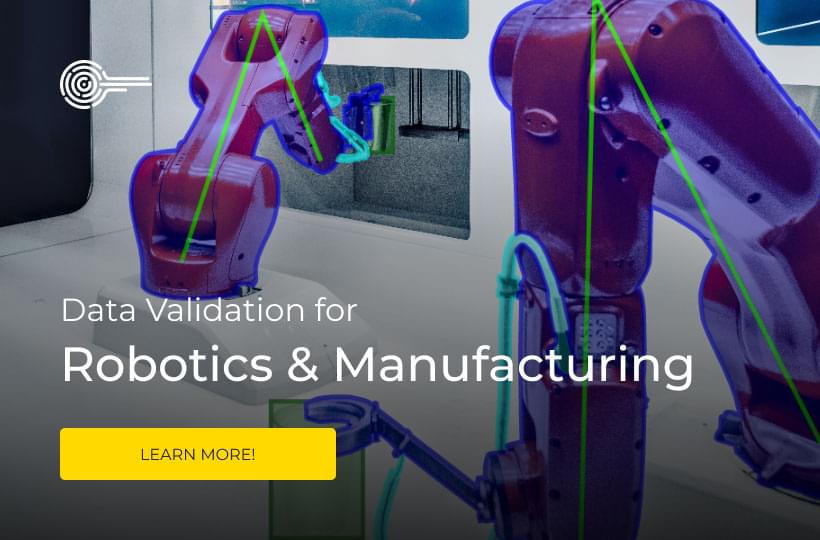
Contact a team member to book your personalized demo today.