How A Bias was Discovered and Solved by Data Collection and Annotation
Computers and algorithms by themselves are not by their nature bigoted or biased. They are only tools. Bigotry is a failure of humans. Bias in an AI usually comes from using small datasets. It was discovered in the first facial recognition algorithms and solved with data innovation and using larger datasets. Famously early facial recognition technologies seemed bigoted because it was better at recognizing the faces of middle-aged white and Asian men like those who funded and created them.
Some larger and valid social criticisms about the causes of bias still exist. The reason was not that the companies were bigoted. The real reason was that the datasets used to train those early facial recognition systems’ AI were too small. There was insufficient data collection and annotation available to do a good job.
That also meant the data was not inclusive enough. There were also lighting problems and problems with some of the pictures. Finally, there were hardware problems with how early digital cameras worked. Those flaws simply were not noticed until those digital cameras made it to market.
The market exposed the early digital cameras to a larger dataset. Once the manufacturers recognized the challenges, they innovated solutions. However, those hardware challenges were still persistent enough to impact the dataset used to create the first facial recognition AI. That problem came before the first facial recognition AI was made. The perceived bias of the people who made it was not somehow coded into those algorithms.
We should all realize that everybody wants to be able to take good pictures of everybody and recognize everyone’s faces. Good companies want to be able to sell to everyone who can buy their products and services.
Technology can’t solve every large social problem. It is not always the right tool. The problem of digital cameras not taking the best pictures of everyone has been solved. The problem of facial recognition not working for everyone has also been solved. The accuracy rate for modern facial recognition AI is now 99.8%. The remaining .02% comes from injuries, aging, and other changes in appearance.
That was achieved by expanding the datasets used to train AI and machine learning algorithms.
Video data collection for machine learning has advanced significantly in the last 10+ years since that problem was known. The larger social problem of bigotry in society still exists. But, those larger social problems are different. The problems of bias in AI are technical challenges. Those problems can be solved much more easily. Technological advancements outpace social advancements. Humans and AI working together can mitigate the larger social problems.
Algorithms Are Made of Rules. They Can’t Bend or Break Rules!
An algorithm is a set of rules used by a computer to solve problems. Artificial Intelligence is a kind of algorithm. Good technology companies don’t just sell products and services. They sell solutions to problems. The best sell solutions to problems that you may not have known that you had. An AI can’t be bigoted. It also can’t bend or break the rules it is made from. That’s true even when it can learn and think in a way that is beyond how a human can think. So, we are unlikely to welcome any robot overlords.
This is also why humans won’t all be put out of work from an AI. An AI might not be bigoted and, for example, deny a loan application because of bigotry, but the same unfair results can occur. Image data collection services, better digital cameras, and other technology can’t solve that problem. So, good companies can do the right thing by having humans with decision-making power work with AI and make exceptions to rules.
The human and AI check each other’s work and learn from each other. When the same unjust outcome happens anyway, a human should still be able to make that right. If humans aren't doing the right thing due to unknown implicit biases, that doesn’t make them bad people.
There are many interesting use cases where an AI can reveal that implicit bias, and humans can learn from it and become better. Of course, technology can’t solve larger systemic social issues, but it can help.
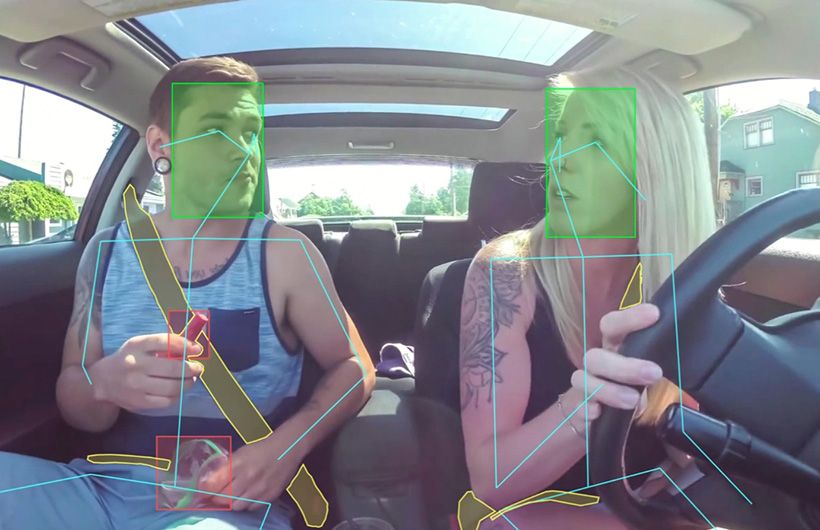
Kinds of Bias in AI
- Systemic bigotry and biases -The company doesn’t want to be bigoted. It is still built into society. So, the system still ends up biased and favors some over others.
- Automation bias -Over-reliance on AI causes a human to make the wrong decision.
- Selection bias -The data isn’t large enough or properly randomized.
- Overfitting and underfitting the data -Overfitting is when the algorithm learns from too much noise in the data, and underfitting is when it doesn’t learn from a trend in the data.
- Reporting Biases -That can be only looking at data in your native language, preferring only data used in citations by others, only choosing data with results that are “positive” and not negative, or making the data support the desired conclusion instead of the accurate result.
- Overgeneralization Bias - Applying something from one personal experience with a group member to all members of that group.
- Group Attribution Biases - They can look like preferring your own group or apply stereotypes to a group you are not a part of.
- Implicit Biases -Unconscious biases that humans are not aware of but affect our decisions. This doesn’t make anyone inherently bigoted or evil, and it can be uncomfortable to learn you have when it is revealed.
- Confirmation Bias - “When you told me about confirmation bias, I started seeing it everywhere.” is a funny example of confirmation bias.
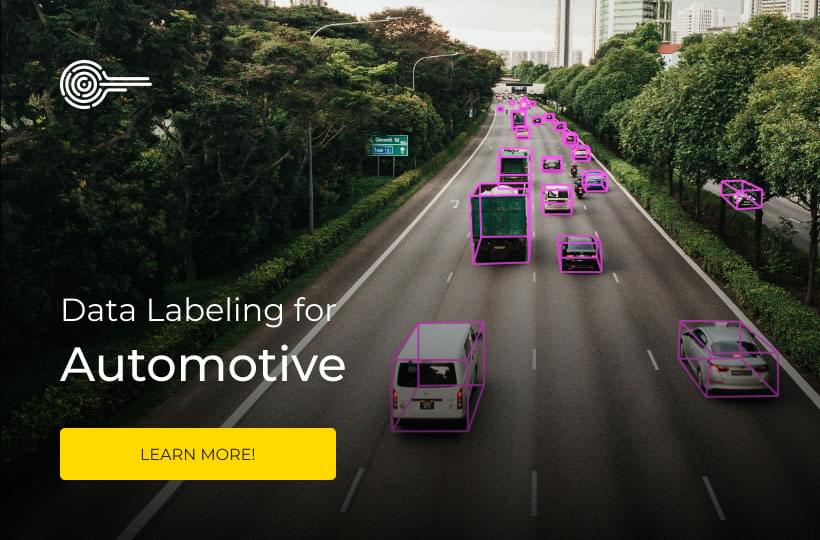
Key Takeaways
Bias in AI is just a technical problem that can be solved by science and engineering. Bias in humans and society is a larger social problem that is much harder to solve. Critics may say that AI bias brings a new meaning to phrases like “systemic racism.” But good companies don’t want to be bigoted. They only want to make all the money they can by making the best products and selling their products and services to everyone.
One of the best solutions to AI bias is exposing the algorithms to much larger, more diverse datasets. Companies should scale their data collection and annotation to be as inclusive as possible.