Handling Cultural and Language Nuances in Annotation Teams
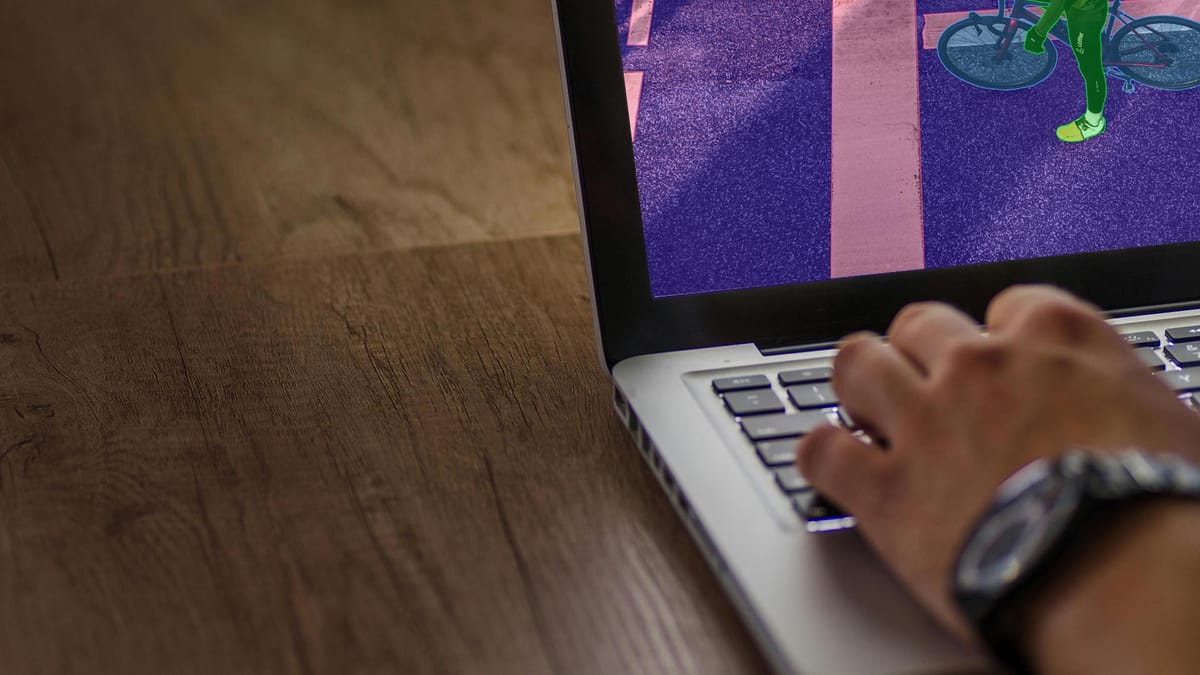
When building datasets, annotation teams often come from different cultural and linguistic backgrounds. This diversity can provide valuable perspectives but also leads to differences in how people understand the same task. Language is also closely related to the local context. Annotators may understand words but not their meanings if they come from a culture they don't know well. This is especially true for slang, humor, or references related to specific events or communities. Without a shared context, there is a risk of misunderstanding what the data is saying. This can lead to labels that seem accurate initially but miss the more profound meaning.
Dealing with this helps to build workflows that guide annotators while leaving room for discussion and clarification. Style guides, example-based training, and feedback loops can reduce confusion and bring people closer together in interpreting tasks. Some teams also utilize smaller regional teams or include cultural reviewers to identify issues early on. There is no perfect solution, but awareness of these gaps and building support around them makes the process more grounded and reliable.
Key Takeaways
- Cultural sensitivity is crucial for effective annotation teams.
- Language variations impact communication and understanding.
- Diverse teams lead to more accurate and inclusive annotations.
- Continuous learning is essential for cultural competency.
- Effective communication strategies bridge cultural gaps.
- Tools and technology can enhance cross-cultural collaboration.

Understanding Cultural Nuances in Annotation
Culture shapes how people interpret language, behavior, and intentions. This can be subtle when working with annotations. A sentence that seems polite or neutral in one culture may seem harsh or even disrespectful in another. This is important when annotators are asked to assess tone, emotion, or appropriateness. If their cultural perspective is different from the audience the model is supposed to serve, it can lead to discrepancies in the data.
Even when people speak the same language, cultural differences can affect how they read between the lines. Take sarcasm, for example. In some cultures, it's common and easy to recognize. In others, it may not be used, making it difficult for annotators to agree on whether a comment is serious or humorous. At first glance, these are small things, but they add up when you're annotating thousands of examples.
Realizing that perfect agreement isn't always the goal is helpful to avoid this. Instead, teams may want clear guidelines and an understanding of where variations occur. Including cultural context in task instructions or allowing annotators to explain their reasoning can help identify disagreements.
Why Cultural Sensitivity Matters
Cultural sensitivity in annotation is about avoiding mistakes and understanding the people behind the data. When a dataset reflects only one point of view, the models built on it may not take into account or misrepresent entire groups. This can manifest in small ways, such as clumsy wording, or in larger ways, such as labeling harmless content offensive because it does not meet the annotator's norms.
Personal and cultural perspectives shape how people react to the same input in tasks such as content moderation or emotion labeling. It is easy to mislabel content based on bias rather than intent without sensitivity to these differences. For example, a post written in African American Vernacular English (AAVE) or regional slang may be mislabeled if the annotator is unfamiliar with that style. Being culturally aware does not mean trying to know every experience; it simply means designing the process so that differences are noticed and handled with care. This may include checking for patterns in differences, creating space for feedback, or adjusting tasks to reduce gaps in interpretation.
The Role of Language in Annotation
Even small changes in word choice or phrasing can change the understanding of something in annotations. This becomes particularly challenging with tasks that involve feelings, intentions, or emotions, where meaning can be closely tied to tone and presentation. What sounds sarcastic, sincere, or angry in one language or dialect may be perceived quite differently in another.
Multilingual teams often face this challenge. Even when everyone uses the same language in a task, their interpretations may differ depending on what they are used to hearing or saying. Some expressions don't translate clearly, and direct literal meanings can lose their original meaning. To cope with this, it is helpful to approach the language flexibly and consciously. Providing examples, explaining tone, and using glossaries or translation notes help to clarify the task. It also helps to involve reviewers who know the local context if the data comes from a particular region or community. The language is complex, but annotation teams can handle it with the proper organization without getting bogged down in details.
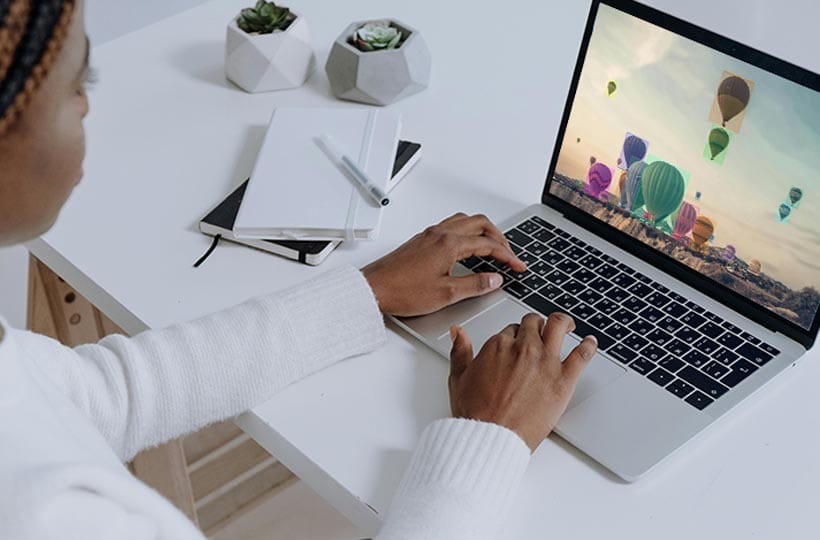
Building Diverse Annotation Teams
The diversity of the annotation team offers a broader range of perspectives, which can lead to better, more inclusive datasets. When people from different regions, cultures, and linguistic backgrounds are involved, it's easier to spot blind spots or assumptions that might otherwise slip through the cracks. This is especially useful when working with global data or user bases, where a single point of view is not enough to cover all the nuances.
But diversity alone is not a solution - it needs support. Teams work best with equal access to information, clear direction, and a voice. This may mean adapting communication styles, offering additional context for specific tasks, or using smaller groups when necessary. It's not about overcomplicating things; it's about ensuring everyone feels included.
Recruiting for diversity also means thinking beyond language skills. It's also helpful to look for cultural familiarity, curiosity, and openness to collaboration. The goal is not to get everyone to agree on every term but to create a space where different perspectives can be shared and worked through. This balance tends to strengthen both the team and the data.
Benefits of Diversity in Teams
- A broader view of language and meaning. Diverse teams are better able to identify nuances, idioms, and cultural references that a more homogeneous group might miss.
- Fewer blind spots in the data. With input from diverse backgrounds, it's easier to identify biases or mislabeling that could affect model performance later.
- Improved processing of subjective tasks. Tasks such as sentiment analysis or content moderation benefit from a combination of viewpoints, leading to more balanced decisions.
- More inclusive data sets. Diverse teams are more likely to represent diverse user groups, which helps create models that work well for a broader audience.
- Stronger collaboration and learning. Working across cultures encourages openness and clearer communication, often improving team dynamics.
Continuous Learning and Improvement
As teams process more data, they begin to recognize patterns in how cultural and language differences affect labeling. This insight can be used to clarify instructions, update training materials, or adjust workflows to make things easier. The better annotators understand these nuances, the better they can perform future tasks with consistency and accuracy.
Regular feedback is essential to support continuous improvement. Whether through team meetings, individual reviews, or joint training sessions, allowing annotators to discuss problems and solutions helps keep everyone on track.
Encouraging annotators to share what they have learned and remain open to feedback also helps improve the system as a whole. This constant exchange of information leads to a more adaptive approach where teams can remain flexible in the face of new cultural knowledge or language changes.
Adapting to Local Contexts
Data collected from different regions or cultures often contain unique expressions, references, and meanings that can be easily missed if not handled carefully. For example, a phrase considered formal in one place may seem casual or even too direct in another. Annotators must know these local differences to ensure the markups accurately reflect the intended message.
It is helpful to provide annotators with clear instructions that include local examples and contextual notes to address this issue. This may include cultural references or explaining regional slang in the training materials. Localizing instructions in this way makes it easier for teams to understand the nuances of the content they are tagging and ensures that the data reflects the true meaning of the words.
Another approach is hiring annotators familiar with the local culture or language, which helps bridge the gap between global and regional perspectives. When the team is well-armed with this knowledge, it is easier to identify potential misinterpretations before affecting data quality.
Language Translation in Annotation
Accurately translating content is critical to understanding its true meaning, but the translation itself can be challenging. Direct translations often fail to capture the nuance, tone, or intent of the original words, leading to misinterpretation of the data. For example, idiomatic expressions or regional phrases may not have an equivalent in another language, making it difficult to get the correct label.
To avoid these problems, it is essential to work with translators who are familiar with the content's language and cultural context. These translators can help adapt meanings, not just words, ensuring that annotations are consistent across languages. Additionally, using bilingual annotators can be helpful as they can identify potential translation issues or misunderstandings before they develop into more serious problems.
While translation tools such as machine translation can be helpful, they often require human oversight to ensure quality. Combining human expertise and technology helps create more accurate and culturally sensitive annotations. This process also requires constant communication between translators and annotators to clarify doubts and ensure language consistency.
Feedback Loops and Their Importance
When annotators receive regular feedback, they can quickly learn from mistakes or misinterpretations and apply this knowledge to future tasks. This continuous exchange helps ensure that everyone is on the same page and that annotations align with the intended recommendations. Without feedback, errors can go unnoticed, leading to inconsistencies that affect the overall quality of the data.
Teams can set up regular meetings where annotators discuss issues, ask questions, and share insights from their work. This helps identify any misunderstandings early and allows for adjustments to the recommendations as needed. The more feedback is built into the workflow, the more it encourages continuous learning and improvement within the team.
Summary
Understanding cultural and linguistic nuances in annotation teams is crucial to creating more accurate, equitable, and inclusive datasets. By understanding and accommodating differences in language, cultural context, and communication styles, teams can avoid biases and misinterpretations affecting data quality. Building diverse teams, promoting continuous learning, and implementing feedback loops are key strategies to ensure consistency and clarity in annotation.
FAQ
Why is cultural sensitivity important in data annotation?
Cultural sensitivity is vital in data annotation because it ensures that annotations are accurate and contextually relevant. It captures the nuances in communication, behavior, and interpretation across cultures.
How do language variations impact the annotation process?
Language variations and dialects significantly affect the annotation process. They influence communication and comprehension within teams.
What are the benefits of building diverse annotation teams?
Diverse annotation teams enhance cultural understanding and language coverage. They navigate complex artistic and linguistic landscapes better.
How vital are feedback loops in managing cultural and language nuances?
Feedback loops are crucial for continuously improving the handling of cultural and language nuances. They encourage open and honest communication.
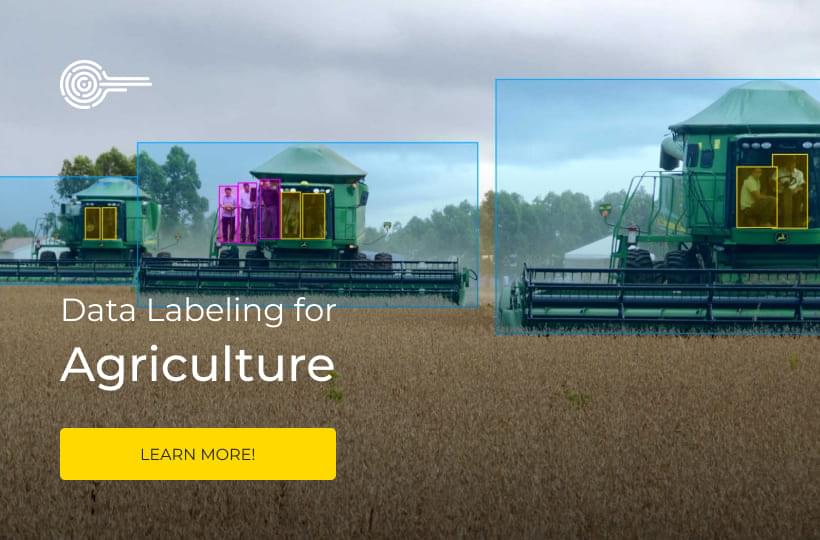