Fraud Fighters: How AI and Machine Learning Can Combat E-commerce Fraud
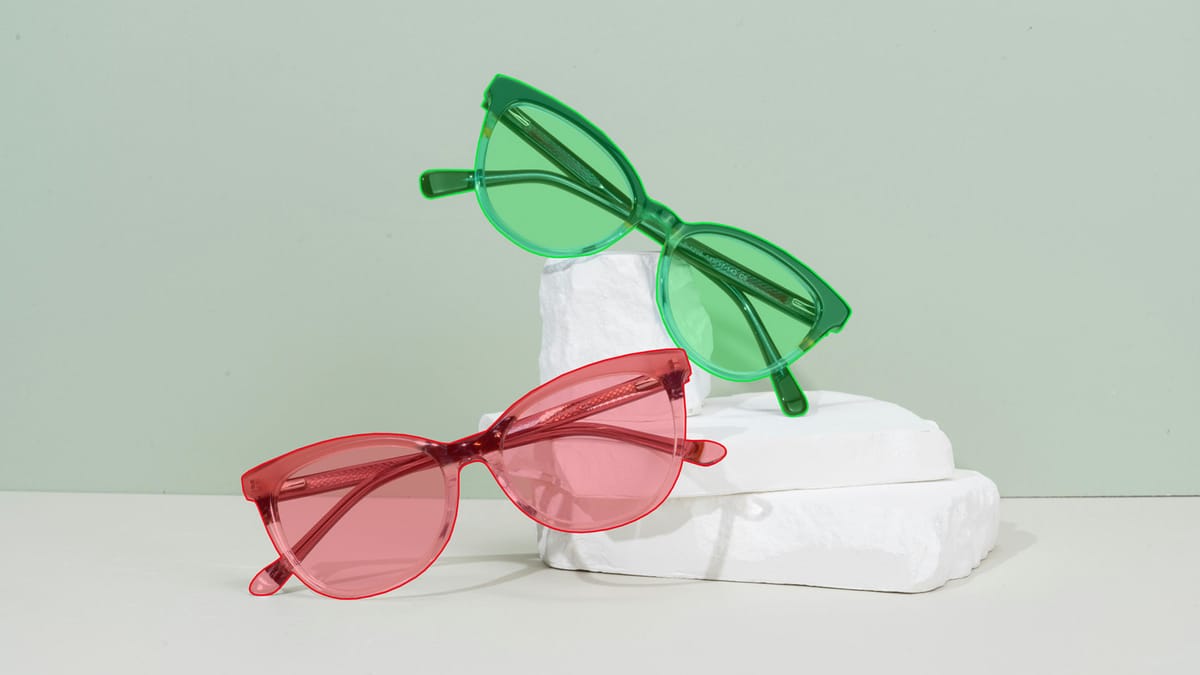
As online shopping grows, so does the complexity of fraud. E-commerce security is now a critical concern for businesses aiming to safeguard their profits and customer trust. AI for fraud detection in e-commerce emerges as a vital solution, tackling the escalating threats of scams effectively.
Machine learning fraud prevention systems are transforming how online retailers address fraud. These technologies can sift through vast data sets in real-time, pinpointing suspicious patterns and behaviors that elude human analysts. By harnessing AI and machine learning, businesses can notably decrease false declines, enhance customer satisfaction, and reduce the costs linked to manual fraud reviews.
The effects of these technologies go beyond mere financial protection. False declines due to suspected fraud often deter consumers from returning to a retailer. By adopting AI-powered fraud detection, e-commerce entities can ensure a smoother customer journey, expedite the checkout process, and foster enduring customer relationships.
Key Takeaways
- E-commerce fraud losses are expected to exceed $20 billion in 2021
- AI and machine learning play a crucial role in modern fraud prevention
- Advanced fraud detection systems can reduce false declines and improve customer experience
- Machine learning technology helps identify both fraudulent and legitimate transactions
- Implementing AI-powered fraud prevention can lead to significant cost savings for businesses

The Rising Threat of E-commerce Fraud
E-commerce fraud statistics reveal a concerning trend for online businesses. As digital shopping expands, so does the risk of financial losses from fraudulent activities. It's essential to delve into the current situation and understand why advanced fraud prevention is vital.
Current Statistics on E-commerce Fraud Losses
The numbers are alarming. In 2022, global e-commerce fraud losses hit $41 million, and this figure is expected to exceed $48 billion in 2023. North America bears over 42% of global e-commerce fraud by value this year. These statistics underscore the pressing need for effective fraud protection strategies.
Year | Global E-commerce Fraud Losses |
---|---|
2022 | $41 million |
2023 (Projected) | $48+ billion |
Impact on Businesses and Consumers
The effects of fraud on businesses go beyond just financial losses. In 2020, false declines led to a $600 billion loss in global e-commerce. This not only harms revenue but also damages customer relationships and brand reputation. Consumers risk identity theft and financial loss, undermining trust in online shopping.
The Need for Advanced Fraud Prevention Techniques
To counter these threats, advanced fraud prevention techniques are crucial. AI-driven models can analyze vast datasets, understand user behavior, and spot anomalies in real-time. This proactive strategy results in more precise fraud detection and prevention, safeguarding both businesses and consumers in the dynamic e-commerce environment.
Understanding AI and Machine Learning in Fraud Detection
AI in fraud detection has transformed how businesses safeguard themselves and their customers. With online scams increasing from 24% to 38% of all reported scams worldwide in 2020, the urgency for advanced fraud prevention technology is undeniable. Machine learning algorithms sift through vast data, uncovering patterns and anomalies that escape human detection.
Ecommerce businesses faced over $40 billion in losses due to online payment fraud in 2022. Traditional fraud prevention methods, such as Address Verification System (AVS) and Card Verification Value (CVV), fall short against sophisticated fraud strategies. AI, however, excels, processing thousands of transactions swiftly with unparalleled accuracy.
AI and machine learning in e-commerce fraud prevention stand out for their adaptability. These systems learn from new data, enhancing their algorithms to counter evolving fraud techniques. This proactive approach is essential, given the projected increase in worldwide credit card losses in online transactions to $43 billion by 2026.
Fraud Type | AI Detection Capability |
---|---|
Payment Fraud | High |
Chargebacks | Effective |
Account Takeovers | Highly Effective |
Fake Account Creation | Very Effective |
Content Scams & Spam | Effective |
Return Fraud | Moderately Effective |
As fraud prevention technology evolves, the outlook is optimistic. Emerging technologies like Deep Learning, Behavioral Analytics, and Biometric Authentication promise to bolster security further. This will make online transactions safer for the 70% of Americans who shop online.
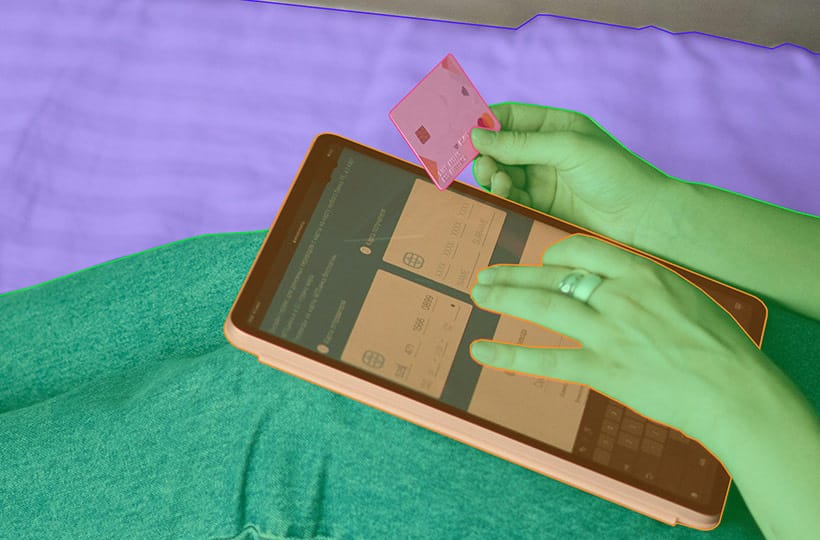
Common Types of E-commerce Fraud
E-commerce fraud types are evolving, posing significant risks to online businesses and consumers. It is crucial to understand these threats for effective identity theft prevention strategies.
Credit Card Fraud
Credit card fraud is a major concern in e-commerce. Thieves use stolen card details for unauthorized purchases, causing financial losses for merchants and cardholders. This fraud has increased with online shopping, where cards are not always needed for transactions.
Identity Theft
Identity theft in e-commerce can have severe effects. Criminals use stolen personal data for unauthorized purchases, new credit accounts, or fraudulent tax returns. Victims often face long-term financial and emotional damage.
Chargeback Fraud
Chargeback fraud happens when customers dispute valid charges with their banks. This results in merchants losing sales, products, and facing chargeback fees. It's a significant concern for online retailers.
Account Takeovers
Account takeover fraud involves unauthorized access to user accounts. Fraudsters use these accounts for illicit purchases or to steal sensitive data. This fraud can severely damage customer trust and a brand's reputation.
Fraud Type | Description | Impact |
---|---|---|
Credit Card Fraud | Unauthorized use of stolen card information | Financial losses for merchants and cardholders |
Identity Theft | Use of stolen personal information for fraudulent activities | Long-term financial and personal consequences for victims |
Chargeback Fraud | Disputing legitimate charges with banks | Revenue loss and additional fees for merchants |
Account Takeovers | Unauthorized access to user accounts | Damage to customer trust and brand reputation |
As e-commerce fraud evolves, businesses must remain vigilant and update their prevention strategies. Adopting strong security measures and keeping up with fraud trends is key to protecting both businesses and customers online.
How Machine Learning Algorithms Detect Fraudulent Activities
Machine learning fraud detection has transformed the battle against e-commerce scams. With an estimated $1 trillion lost to fraud from 2022 to 2023, companies are adopting advanced technologies to safeguard their assets and customers.
Pattern Recognition
Machine learning shines in identifying patterns within large datasets. These algorithms pinpoint fraudulent behavior with unmatched precision. By scrutinizing past data, supervised learning algorithms swiftly detect credit card fraud and identity theft.
Anomaly Detection
Anomaly detection is vital in preventing online fraud through machine learning. Unsupervised learning methods pinpoint unusual patterns that could indicate fraud. This method excels in identifying new fraud types that don't fit established patterns.
Behavioral Analysis
Machine learning algorithms delve deep into transactional behavior to detect fraud. They scrutinize various transaction aspects, including:
- User login patterns
- Purchasing habits
- Device information
- Geographic location
This detailed analysis enables real-time fraud detection, significantly cutting down financial losses for businesses.
The success of machine learning in fraud prevention is clear in real-world scenarios. Citibank cut phishing attacks by 70% with machine learning, while Walmart reduced shoplifting by 25% through ML-powered video analysis. These examples underscore the strength of AI in fighting fraud in the digital era.
AI for Fraud Detection in E-commerce
E-commerce businesses face a significant threat from fraudsters. AI-powered fraud detection offers a transformative solution to combat these risks. By harnessing machine learning in online retail, companies can identify suspicious activities with unprecedented speed and accuracy.
AI systems delve into vast datasets to uncover patterns and anomalies. This capability enables them to detect a variety of fraud types, including:
- Credit card fraud
- Identity theft
- Account takeovers
- Promo abuse
- Shipping fraud
E-commerce security solutions fueled by AI bring numerous advantages:
Benefit | Description |
---|---|
Real-time detection | AI monitors transactions instantly, flagging suspicious activity before it's too late |
Reduced false positives | Advanced algorithms minimize false alarms, improving customer experience |
Automated analysis | AI handles large volumes of data, freeing up human resources for complex cases |
Improved accuracy | Machine learning models become more precise over time, enhancing fraud detection rates |
With global e-commerce fraud losses hitting $48 billion in 2023, integrating AI into fraud prevention is essential for online retailers. By adopting these advanced technologies, businesses can foster safer shopping environments and enhance customer trust.
Benefits of Using AI and ML in Fraud Prevention
AI fraud prevention is revolutionizing e-commerce security. These technologies provide robust tools to safeguard businesses and their customers from financial losses and data breaches.
Real-Time Detection and Response
Real-time fraud detection is a pivotal advancement. AI systems swiftly analyze transactions, identifying suspicious activity as it occurs. This rapid response is essential in today's swift digital environment.
Reduced False Positives
One of the key advantages of AI in fraud prevention is the decrease in false positives. Traditional methods often incorrectly flag legitimate transactions, leading to customer frustration. AI's accuracy minimizes these errors, enhancing the shopping experience.
Adaptability to New Fraud Patterns
Fraudsters are continually evolving their strategies, but AI remains ahead. Machine learning algorithms adapt to new fraud patterns, ensuring continuous protection against emerging threats.
Benefit | Impact |
---|---|
Real-time detection | Immediate response to suspicious activity |
Reduced false positives | Improved customer satisfaction |
Adaptability | Protection against new fraud tactics |
By harnessing these AI fraud prevention benefits, businesses can foster a secure e-commerce environment. This approach not only safeguards revenue but also fosters customer trust and loyalty.
Implementing Machine Learning in E-commerce Fraud Protection
E-commerce fraud protection requires strong strategies to counteract the growing threats. Machine learning brings powerful tools to protect online businesses. In 2023, online fraud led to losses over $48 billion, underscoring the critical need for advanced fraud prevention tactics.
Key steps in machine learning implementation include:
- Continuous monitoring and updating of models
- Integration with existing fraud detection systems
- Mandatory two-factor authentication
- Device fingerprinting
- Velocity checks to limit transactions from new accounts
These strategies have shown significant success. For example, Microsoft reduced manual review rates by 80%, reaching a mere 0.03% review rate. This efficiency allows businesses to focus on real customer needs while significantly reducing fraud risks.
Machine learning models can identify emerging fraud tactics, detect patterns in data, and make predictions to combat fraudulent activities.
By applying machine learning to e-commerce fraud protection, businesses can swiftly adapt to new threats and lower false positives. This approach not only saves costs but also boosts customer trust and loyalty over time.
Challenges and Limitations of AI in Fraud Detection
AI fraud detection faces numerous challenges, despite its potential. Businesses aim to protect themselves but encounter obstacles in implementing effective AI solutions. Let's delve into the primary issues affecting AI-driven fraud prevention.
Data Quality and Quantity Issues
The success of AI in fraud detection relies heavily on data quality. Incomplete or inaccurate data can severely impact AI algorithms' performance. Organizations require vast amounts of high-quality data to train their models effectively. This demand often poses a significant challenge, especially for smaller businesses with limited resources.
- False positives, causing unnecessary customer friction
- Missed fraud attempts, resulting in financial losses
- Biased decision-making, potentially leading to legal issues
Keeping Up with Evolving Fraud Techniques
Fraudsters are constantly adapting their tactics, making it challenging for AI systems to stay ahead.
Challenge | Impact | Solution |
---|---|---|
Data Quality Issues | Reduced accuracy in fraud detection | Implement robust data collection and cleaning processes |
Evolving Fraud Techniques | Increased vulnerability to new fraud methods | Regular model updates and ongoing training with latest fraud data |
Integration Complexity | Difficulty in implementing AI solutions | Invest in modern, scalable IT infrastructure |
To overcome these challenges, businesses must invest in robust data management practices and continuously update their AI models. By addressing these issues head-on, organizations can fully leverage AI in combating fraud effectively.
Future Trends in AI-Powered Fraud Prevention
The future of fraud prevention in e-commerce is rapidly evolving. Advanced fraud detection algorithms are becoming more sophisticated, learning from complex data patterns to stay ahead of fraudsters. Real-time threat analysis systems are emerging as a key trend, offering instant fraud detection and prevention for businesses of all sizes.
AI trends in e-commerce security point towards increased collaboration among businesses. Sharing data and insights about fraudulent activities helps create a united front against cybercriminals. This collective approach strengthens the overall security landscape of the e-commerce ecosystem.
Predictive analytics is set to play a crucial role in the future of fraud prevention. By analyzing historical data, AI systems can forecast potential risks and take preemptive measures to block suspicious activities. This proactive stance significantly reduces the chances of successful fraud attempts.
Behavioral biometrics is another exciting development. AI systems are now capable of authenticating users based on unique patterns like keystroke dynamics and mouse movements. This adds an extra layer of security, making it harder for fraudsters to impersonate legitimate users.
- Machine learning models adapting to new fraud patterns
- Real-time monitoring for immediate threat detection
- Automated decision-making to reduce response times
- Ensemble learning for enhanced prediction accuracy
As AI continues to evolve, we can expect even more innovative solutions in the battle against e-commerce fraud. The future looks promising for businesses and consumers alike, with AI-powered security measures paving the way for safer online transactions.
Best Practices for Combining AI with Traditional Fraud Prevention Methods
In the dynamic world of e-commerce, effective fraud prevention is vital for protecting businesses and their customers. With fraud losses globally exceeding $5 trillion yearly, a comprehensive, multi-layered fraud detection strategy is vital. By integrating AI-powered systems with traditional methods, you can develop a strategy that evolves with new threats and utilizes human expertise.
Multi-layered Approach to Fraud Detection
A multi-layered fraud detection strategy combines AI and traditional methods effectively. This method has proven successful, with American Express enhancing fraud detection accuracy by 6% through deep learning models. By blending rule-based systems, machine learning algorithms, and human review, you can build a strong defense against various fraud types. Synthetic identity fraud, for instance, now makes up over 85% of identity fraud cases.
Regular Model Updates and Training
Staying ahead of fraudsters requires keeping AI models current. The rise in online shopping in the late 2000s brought new fraud challenges, underscoring the importance of continuous adaptation. Regularly updating your models with the latest data, including AI-generated synthetic data, aids in recognizing new fraud patterns. This is essential as e-commerce fraud has evolved from basic credit card fraud to sophisticated schemes like identity theft and account takeovers.
Human Oversight and Intervention
AI and machine learning are powerful tools for fraud detection, but human oversight is still essential. AI and human collaboration ensures that complex decisions and edge cases are managed well. Top financial firms are adopting AI for better fraud detection but still depend on human expertise to refine their systems and interpret outcomes. This balanced approach minimizes false positives and adapts to the fast-evolving fraud landscape. Digital fraud rates saw a 52% increase between 2019 and 2021 during the COVID-19 pandemic.
FAQ
What are the current statistics on e-commerce fraud losses?
E-commerce fraud is escalating, with retailers facing potential losses of over $20 billion in 2021, an 18% increase from the previous year. This issue is global, with fraud losses estimated at $1 trillion. Moreover, 26% of global citizens experienced financial loss due to scams or identity theft between 2022 and 2023. In the U.S., financial institutions reported a 65% increase in fraud losses during this period.
Why are advanced fraud prevention techniques necessary?
The surge in online shopping has led to an uptick in e-commerce fraud, necessitating advanced fraud prevention strategies. These strategies are crucial for safeguarding both businesses and consumers.
How do AI and machine learning help in fraud detection?
AI and machine learning are instrumental in the fight against fraud. They leverage vast amounts of historical data to identify new patterns and flag suspicious transactions. Over time, these systems refine their ability to detect anomalies and adapt to emerging fraud tactics.
What are some common types of e-commerce fraud?
Common e-commerce fraud types include credit card fraud, identity theft, and chargeback fraud. Other forms include account takeovers, card cracking, credential stuffing, fake account creation, and man-in-the-middle attacks.
How do machine learning algorithms detect fraudulent activities?
Machine learning algorithms detect fraud through pattern recognition and anomaly detection. They analyze vast datasets to uncover hidden patterns and red flags indicative of fraud.
What are the benefits of using AI and ML in fraud prevention?
AI and ML offer real-time detection and response to fraudulent activities, significantly reducing false positives. These technologies adapt to new fraud patterns, ensuring accurate transaction legitimacy checks. This results in a smoother customer experience and reduced operational costs.
How can businesses implement machine learning in e-commerce fraud protection?
Businesses can implement machine learning by collecting high-quality data and using advanced algorithms. Continuous monitoring and updating of models is essential. Integration with other fraud detection systems is also crucial. Best practices include mandatory two-factor authentication, device fingerprinting, and velocity checks.
What challenges exist in AI fraud detection?
Challenges include ensuring data quality and quantity, as well as keeping up with evolving fraud techniques. Fraudsters now employ AI and machine learning to enhance traditional scams. Therefore, businesses must continually update their AI models and datasets.
What are some future trends in AI-powered fraud prevention?
Future trends include the development of more sophisticated algorithms and real-time threat analysis systems. There will be increased collaboration among e-commerce businesses to share data and insights against fraudsters.
What are the best practices for combining AI with traditional fraud prevention methods?
Best practices include a multi-layered fraud detection approach, regular updates to AI models, and human oversight. Integrating AI with rule-based systems and human review ensures a comprehensive strategy. This approach leverages human expertise while maintaining adaptability.
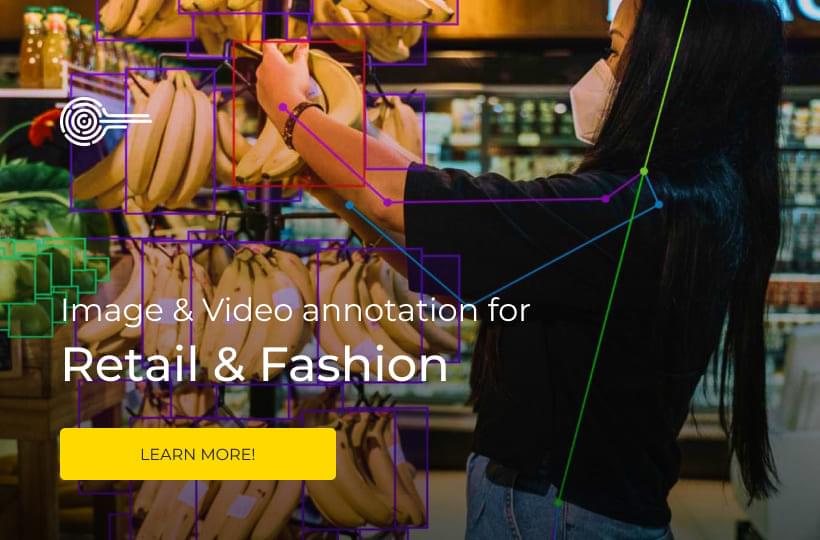