Developing AI Crop Harvesting Systems With Image Annotation
Agricultural robotics is a booming industry. Digital agriculture combines digital technologies, such as image annotation, sensors, and software. It helps to improve efficiency, sustainability, and profitability.
The digital revolution is bringing a new era. Digital agriculture transforms the way we grow food and manage our environment. For example, farmers can decide when to harvest crops using integrated, data-driven technologies.
Agricultural robotics leverages AI and computer vision to complete crop harvesting. Farmers worldwide boost yields, reduce labor costs, increase efficiency and reduce environmental impact.
Tractors cut down crops like wheat or corn. Then, laborers come in with shears and tools to gather plants. The process is time-consuming.
In contrast, robotic harvesting uses autonomous robots equipped with cameras. They identify which plants to harvest based on size, coloration patterns, or both. As a result, farmers save money, reduce carbon dioxide emissions and increase efficiency. Moreover, fewer people need to do all this work.
What Is AI Crop Harvesting?
Artificial Intelligence (AI) streamlines traditional farming practices like irrigation. It also improves modern ones like satellite imagery for precision planting.
The following are some examples of technologies used with this approach:
- Data mining extracts patterns from large datasets to predict future crops.
- Pattern recognition finds similarities between different sets of data. For example, it finds similar vegetation patterns in satellite images.
- Machine learning uses data from past experiences to predict future outcomes. For example, it knows how much fertilizer a given crop needs.
- Image annotations for robotics labels images and data to train AI systems.
Once companies collect this information, they analyze it using artificial intelligence (AI) algorithms. Then, you can deploy these in decision engines using an AI crop harvesting solution.
At the same time, farmers save time and money on manual labor costs such as harvesting crops. Depending on where they live, farmers may need personnel up to seven months per year.
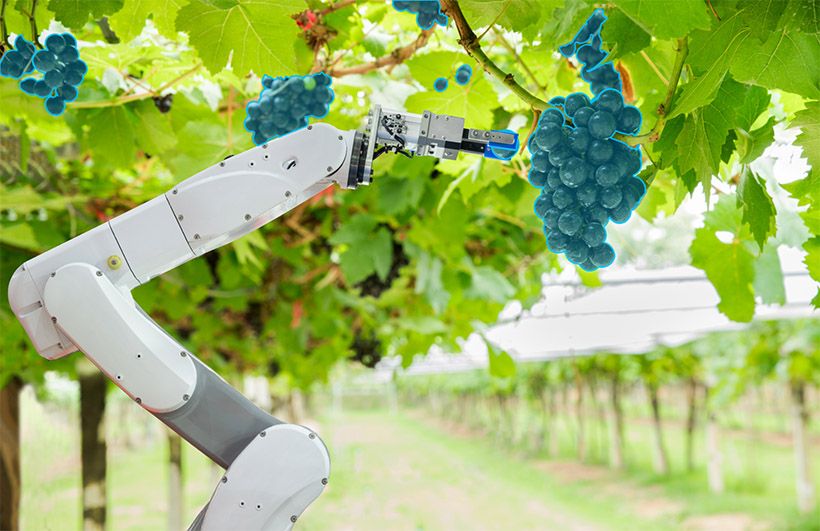
Developing Efficient AI Crop Harvesting Systems
Harvesting crops is laborious and time-consuming. Using AI can reduce losses, costs, environmental impact, and food waste. Machines developed from AI have already reduced harvesting times by up to 80%.
Manual harvesting is hazardous for farmers when machines are unavailable or not working. Up to 250 tractor accidents result in death or severe injury.
Injury rates could decrease with better decision-making capabilities like GPS technology. Then, they could operate in areas where dangers may occur and avoid obstacles.
Reducing Harvest Losses at the Field Level
High-speed precision agriculture is becoming popular. It reduces labor costs and increases productivity. AI crop harvesting enables automated crop selection and accurate planting depth control.
Advancements in autonomous vehicle technology fuel agricultural robotics growth. Robotics allows people to navigate rugged terrains like rocks or uneven ground. This work is something manual laborers wouldn't be able to do.
Field Robotics and Agricultural Applications
Field robotics is its application to agricultural tasks. Agricultural robots improve crop yields and quality, post-harvest crop handling, irrigation, and fertilization. Field robotics applications also increase in food processing, animal husbandry, and aquaculture.
The Role of Image Annotation in AI Crop Harvesting
Image annotation labeling improves the efficiency and accuracy of the process. It identifies relevant features of plants, fruits, vegetables, and weeds in an image. Without data annotation, there would be no algorithm to know what to look for in a crop.
Image and video annotation label data to make it machine-readable. This labeling allows for more efficient harvesting methods and fewer human workers. High-quality labels are essential. They ensure that AI data is accurate.
Image annotation for machine learning provides instructions to train a particular task. For example, let's say you want to collect data on soil moisture levels in your field.
You can collect this information with an AI-powered device. Or you could use drone image annotation or satellite images. But, this data will be meaningless unless you know what the various moisture levels mean. Thus, you need to annotate this information with metadata. Then, you can determine what these levels mean and whether they are good for our crops.
Data Annotation for AI Crop Harvesting
There are many opportunities for agricultural robotics to help farmers and their crops. The digital revolution in agriculture is changing how people do business. It will only continue growing from here.
Keymakr specializes in machine learning image annotation for agriculture. For AI to benefit farmers, they need more than one product. They also need well-trained datasets. Keymakr is an AI technology company. It has a proven track record of creating custom training datasets. These datasets deliver state-of-the-art AI solutions in agriculture.
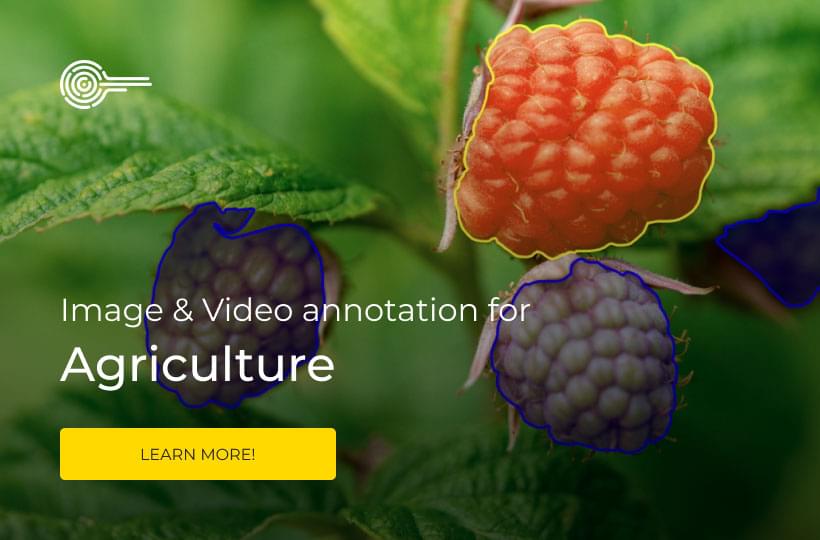