Data Labeling: Why Precision is Important
The main reason that precision is so important is that it impacts the quality and safety of your final AI product. This precision takes many forms. For example, if you want your AI to recognize a bicycle, it has to use pictures of bicycles labeled as bicycles in its training. If something is not a bicycle and it is labeled a bicycle. That's a big problem.
If it needs to recognize human beings, it needs images of humans with accurate labeling. It may also need images of humans and bicycles together and humans with other objects engaged in common human activities. For example, if the AI is driving a car, you really can't have it getting confused when it sees a woman on a bicycle across the street. That could lead to the tragic loss of life.
Of course, when something like that happens, it tends only to happen once because the AI can be easily updated to learn from that experience. So it will recognize a human with a bicycle and act appropriately every time in the future.
It would also be better to avoid such things from ever happening. You can do that with precise data annotation techniques. Other kinds of precision can be best explained with common measurements. Take, for example, a ruler that measures length in the metric system.
The smallest unit of measurement and the thickness of the lines of the ruler determine how precisely it can measure. There will almost always be a smaller, more precise unit of measurement, which means that all measurements are approximate. They all have a degree of uncertainty, which can also be measured.
Increasing precision in anything, including your data labeling projects means decreasing uncertainty. It requires a lot of attention to fine details. That is a real challenge since data training usually needs large datasets.
It is interesting to note that in the totality of the earth we now have a lot of data collected that could be used. So much that if you could get all 8 billion people to work on labeling it all, there would be more data than all humanity could label. More of this data is generated and collected all the time.
There is an extra problem that plenty of that data needs specialized, expert data annotation. For example, medical data annotation that doctors need. With an abundance of data, the real challenge is in labeling it all with precision.
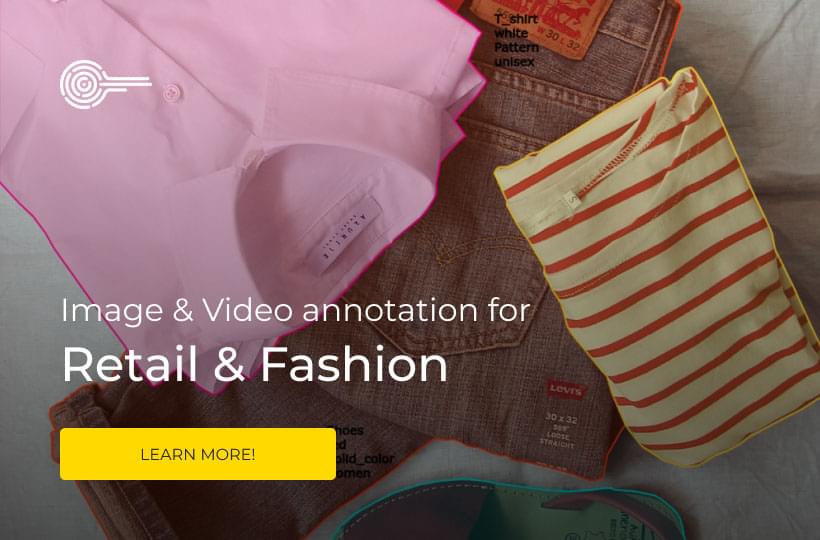
Precision In Data Labeling
- Accurate object recognition in context. When it sees a woman, it knows a woman is there. When it sees a bicycle it knows a bicycle is there. When it sees a woman with a bicycle there, it knows what it is seeing.
- Precision can also be a measure of uncertainty: the less uncertainty, the more precision.
- Many solutions to increase the precision of your data labeling project can be implemented before, during, and after training your AI.
- Precision impacts the quality and safety of your AI products and services.
Solutions For Increasing the Precision In Your Large Scale Data Labeling Projects
There are many solutions to this problem. For example, you could filter irrelevant or useless data out. The best of these solutions have humans working in collaboration with AI. Companies should collaborate with data annotation outsourcing services.
One solution is to use an active learning approach for your machine learning. That means iterating your data labeling tasks and datasets used to train your model. That also means the work is done in a series of data annotation jobs. This is an effective way to achieve your desired level of precision.
It is important to consider that active learning methods are only as effective as your ability to manage the project. Communication with your teams becomes vital as tasks and priorities change. That even goes all the way down to specific microtasks using active learning.
As you advance your AI model and data annotation project, it is possible to create or use automated data annotation tools. That can speed up a very large data annotation when certain critical milestones are achieved.
You could also refine your data annotation after your dataset has been used to train your model. You then send the data that AI is more uncertain about for humans to label. That won't be fed into the dataset your AI is currently being trained on. Instead, it is used for the next iteration.
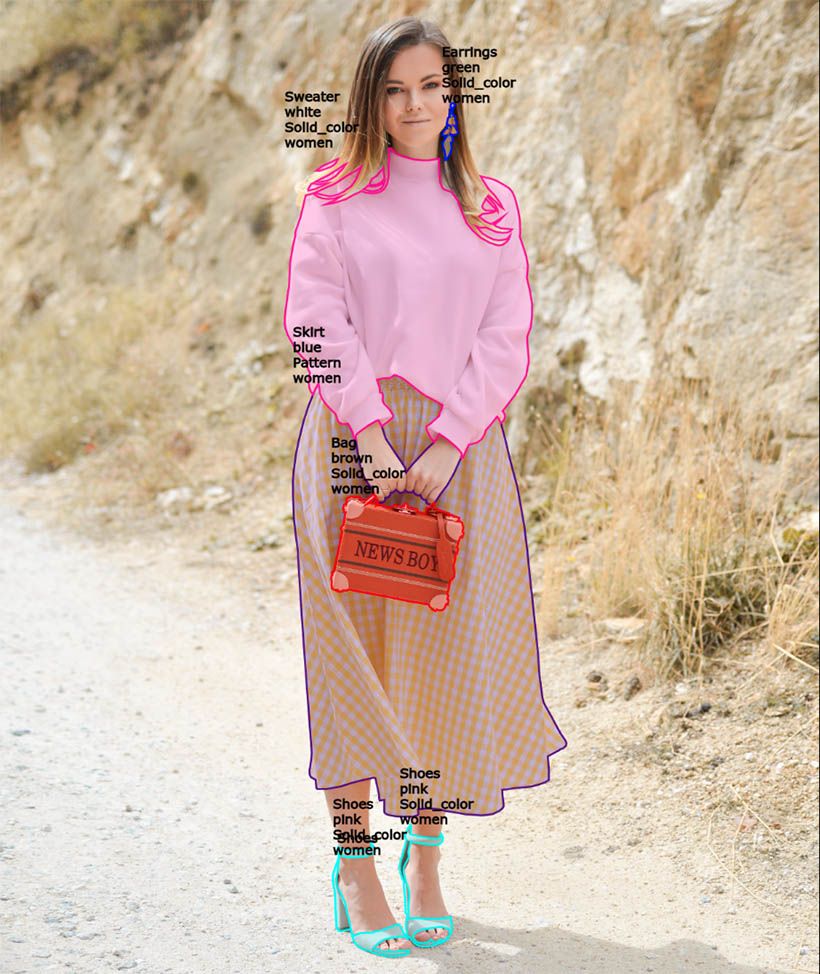
Conclusions
Precision is about accuracy and also a measurement of uncertainty. The challenge isn't having a lot of data to train your AI. Instead, the problems lay in the fine details of precision and accurately labeling it all.
Some of the solutions can be implemented throughout the entire data training process. Data can be labeled and filtered for more efficient supervised learning. Data labeling can be done while your AI is being trained using active learning methods. These are automated data labeling tools. Data that your AI is found to be less certain about can be sent to humans to be labeled.
All of these solutions are about optimizing your data, before using it for supervised learning. Optimizations that can save you time and money and save your development team headaches.
Of course, optimizing your dataset is complicated and best left to experts. That is why you should consider using data annotation outsourcing services that suit your needs.