Advancements in Synthetic Data Generation Techniques
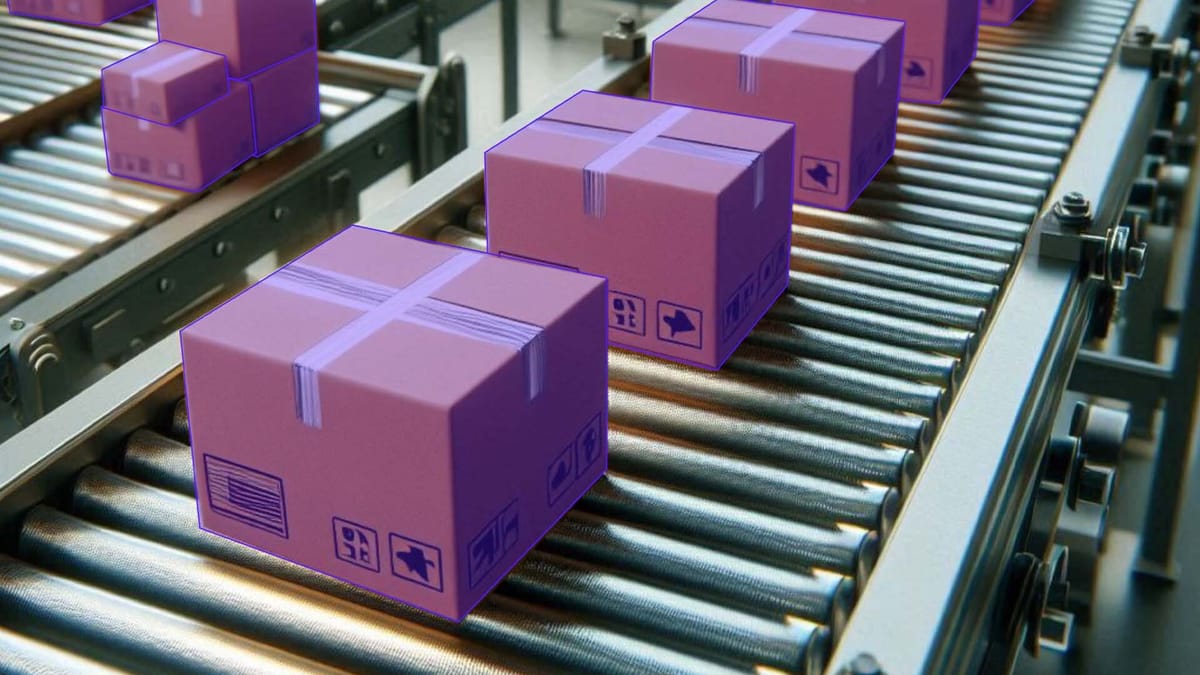
Around 90% of all data in the world was created in just the last two years. This staggering statistic underscores the pressing need for innovative data solutions. Synthetic data generation techniques are at the forefront, revolutionizing AI advancements and data-driven decision-making.
This field is transforming sectors from healthcare to finance, offering a robust alternative to traditional data collection methods. By replicating real-world data without compromising privacy, these techniques unlock new avenues for research and development.
As data generation trends evolve, the demand for high-quality, diverse datasets is skyrocketing. Synthetic data is a game-changer, addressing limitations, privacy concerns, and reducing the costs associated with data collection. It's becoming a pivotal tool in the AI toolkit, driving innovation in areas like autonomous vehicles and fraud detection.
Companies like Waymo and Tesla are pioneering the use of synthetic data to train their self-driving cars. They simulate rare events and edge cases that are impractical or impossible to replicate in real-world testing. This method is not only safer but also more efficient, hastening the advancement of autonomous technology.
Key Takeaways
- Synthetic offers solutions for data privacy, scarcity, and bias challenges
- Three main types: rule-based, simulation, and data-driven generation
- Used across industries like healthcare, finance, and autonomous vehicles
- Enables testing of rare events and edge cases safely
- Companies like Waymo and Tesla use synthetic data for development
- Language models open new possibilities for text data generation

Understanding Synthetic Data: Definition and Importance
Synthetic data is transforming various sectors. It's a form of artificially created information that closely resembles real-world data but safeguards privacy. Let's delve into the synthetic data definition and its increasing relevance.
What is synthetic data?
Synthetic data is the term for information generated by computers that closely matches real data in terms of statistical properties. It's produced through advanced algorithms and machine learning methods. This artificial data captures the essence of real data without infringing on personal privacy.
The growing importance of synthetic data in various industries
The significance of synthetic data is on the rise. Gartner forecasts that in 2024, 60% of AI and analytics projects will utilize synthetic data. Sectors such as finance, healthcare, and automotive are increasingly adopting this technology for its cost-efficiency and privacy safeguards.
Key advantages of using synthetic data
The benefits of synthetic data are manifold. For instance, artificially generated images are a fraction of the cost of real ones, making it a cost-effective solution. It ensures compliance with privacy laws like HIPAA and GDPR. Moreover, synthetic data facilitates flexible data sharing and use, all while maintaining confidentiality.
- Cost-effective: Synthetic crash data saves automakers money compared to real tests
- Privacy-compliant: Helps meet HIPAA, GDPR, and CCPA requirements
- Versatile: Useful for fraud detection, risk management, and AI training
- Scalable: Allows creation of large, diverse datasets quickly
Understanding synthetic data and its importance is vital in today's data-driven era. It presents a robust solution to data scarcity and privacy issues, opening up new avenues for innovation across sectors.
The Evolution of Synthetic Data Generation Techniques
The history of synthetic data stretches back decades, rooted in early methods of data collection. Ancient civilizations employed clay tablets and cuneiform writing to document information. As technology evolved, so did the pace of data generation.
The mid-20th century was a pivotal moment with the introduction of computers. This innovation transformed statistical analysis, setting the stage for modern data science. The fusion of mathematics, statistics, and computer science established the groundwork for sophisticated synthetic data creation.
AI has been instrumental in the development of synthetic data generation. Initially, first-generation techniques utilized traditional statistical approaches like randomization and sampling. However, second-generation methods now incorporate advanced machine learning algorithms. These include Generative Adversarial Networks (GANs) and Variational Autoencoders (VAEs).
Currently, synthetic data generation encompasses a broad spectrum of data types. It includes tabular data for e-commerce and healthcare, as well as non-tabular formats like text, images, and audio. These techniques are revolutionizing data-driven activities across various sectors.
Rule-Based vs. Model-Based Synthetic Data Generation
Synthetic data generation techniques have evolved to meet diverse industry needs. Two primary approaches stand out: rule-based generation and model-based generation. Each method offers unique advantages in creating artificial datasets that mimic real-world information.
Rule-Based Synthetic Data Generation
Rule-based generation involves creating data based on predefined rules and constraints. This method is straightforward and allows for precise control over the generated data. It's particularly useful when you need to create data that follows specific patterns or business logic. Rule-based techniques are often employed in software testing and scenario planning.
Model-Based Synthetic Data Generation
Model-based generation utilizes statistical models trained on real data to produce new samples. This approach captures complex relationships and patterns within the original dataset. It's ideal for creating large, diverse datasets for machine learning and AI training. Model-based methods excel in generating realistic data while preserving privacy and statistical properties.
Hybrid Data Generation Techniques
Hybrid data generation combines rule-based and model-based approaches. This fusion offers greater flexibility and can address more complex data generation needs. By leveraging the strengths of both methods, hybrid techniques can produce highly realistic and customizable synthetic datasets.
Method | Complexity | Control | Realism |
---|---|---|---|
Rule-Based | Low | High | Moderate |
Model-Based | High | Moderate | High |
Hybrid | Moderate | High | High |
Choosing the right synthetic data generation method depends on your specific needs. Consider factors like data complexity, privacy requirements, and intended use when selecting between rule-based, model-based, or hybrid approaches.
Generative Adversarial Networks (GANs) in Synthetic Data Creation
GANs have transformed synthetic data innovation. They feature two primary components: the Generator and the Discriminator. The Generator synthesizes data, whereas the Discriminator distinguishes real from fake samples. This duo engages in a continuous competition, enhancing each other's performance.
The Generator evolves to produce increasingly realistic data. It begins with random noise and refines its output based on the Discriminator's feedback. Conversely, the Discriminator becomes adept at identifying fake data. This relentless competition leads to synthetic data that closely resembles real-world data.
GANs are versatile, applied in fields from healthcare to finance. They generate synthetic data of high quality, akin to production-ready information. This innovation reduces the risks associated with handling confidential client data. GANs have significantly reduced the time-to-market for new data generation projects.
Training GANs poses challenges, requiring patience and a dataset that accurately represents real data. The Generator's performance heavily relies on the Discriminator's effectiveness. Despite these hurdles, GANs have proven crucial in computer vision, medical imaging, and natural language processing.
- GANs create synthetic data mimicking real information
- They consist of a Generator and a Discriminator
- GANs excel in healthcare, finance, and security applications
- Training requires patience and high-quality datasets
Synthetic Data Generation Techniques: Current State-of-the-Art
The field of synthetic data generation is rapidly evolving. Today's cutting-edge techniques offer powerful solutions for creating realistic and valuable datasets. Let's explore some of the most advanced methods currently in use.
Variational Autoencoders (VAEs) for Data Synthesis
VAEs have emerged as a powerful tool for synthetic data generation. These neural networks learn to encode real data into a compact representation and then decode it to create new, similar instances. VAEs excel at capturing complex data distributions, making them ideal for generating diverse and realistic synthetic datasets.
Advanced Statistical Modeling Techniques
Statistical modeling has come a long way in synthetic data creation. Modern approaches use sophisticated probabilistic models to capture intricate relationships within data. These techniques can generate synthetic datasets that closely mimic the statistical properties of real-world data, preserving important patterns and correlations.
AI-powered Synthetic Data Generation Tools
AI data generation tools represent the pinnacle of synthetic data technology. These advanced systems often combine multiple techniques, leveraging deep learning and reinforcement learning to create highly realistic and diverse datasets. They can handle complex data types and structures, making them versatile across various industries.
Technique | Key Features | Best Suited For |
---|---|---|
VAEs | Compact encoding, flexible decoding | Image and text data |
Statistical Modeling | Preserves data relationships | Tabular and time-series data |
AI-powered Tools | Combines multiple techniques | Complex, multi-modal datasets |
By 2024, experts predict that 60% of data used to train AI systems globally will be synthetic. This shift underscores the growing importance and effectiveness of these advanced generation techniques in creating high-quality, privacy-preserving datasets for AI and machine learning applications.
Applications of Synthetic Data Across Industries
Synthetic data is rapidly expanding its use across various sectors. Its ability to address data challenges while upholding privacy is crucial. Let's delve into the main industry applications and trends in synthetic data generation.
In healthcare, synthetic data safeguards patient privacy while aiding medical research. Hospitals employ it to train AI models for diagnosing diseases from images. Financial institutions use synthetic data for fraud detection and risk assessment, without compromising sensitive customer data.
The automotive industry taps into synthetic data for testing autonomous vehicles in simulated environments. This method cuts costs and speeds up development. Retailers apply synthetic data for managing inventory and analyzing customer behavior, enhancing sales strategies.
Industry | Synthetic Data Application | Benefits |
---|---|---|
Healthcare | Medical imaging analysis | Privacy preservation, improved diagnosis |
Finance | Fraud detection | Risk mitigation, regulatory compliance |
Automotive | Autonomous vehicle testing | Cost reduction, faster development |
Retail | Customer behavior analysis | Enhanced sales strategies, personalization |
The evolution of synthetic data generation is driving its adoption across sectors. Grand View Research projects the synthetic data market to expand from $1.63 billion in 2022 to $13.5 billion by 2030. This surge indicates the growing need for quality, privacy-respecting data in AI and machine learning.
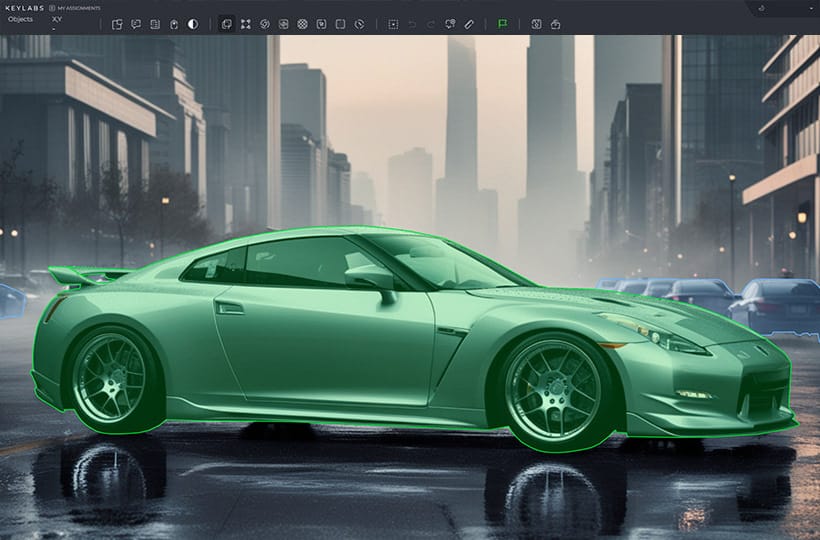
Overcoming Challenges in Synthetic Data Generation
Synthetic data generation offers significant advantages but also presents hurdles. Companies encounter issues with data quality, privacy, and the requirement for data diversity. This section delves into these challenges and proposes solutions.
Ensuring Data Quality and Realism
Creating synthetic data that accurately reflects real-world complexity is paramount. Businesses employ techniques like CTGAN, TVAE, and CopulaGAN to produce synthetic datasets. They assess data quality by utilizing utility measures from Random Forest models. These methods compare mean R2 scores for regression and F1 scores for classification tasks.
Addressing Privacy in Synthetic Data
Privacy is a major concern in synthetic data generation. Companies employ ε-Identifiability to measure re-identification risk. This ensures that sensitive information from original datasets remains protected. Striking a balance between data utility and privacy protection is crucial for maintaining trust and adhering to regulations.
Balancing Diversity and Representativeness
Ensuring synthetic datasets are diverse yet representative is crucial. It mitigates biases and enhances AI model performance with new data. Companies divide datasets into training (80%) and test (20%) sets, iterating multiple times for statistical significance. This method guarantees diverse yet representative synthetic data.
Addressing these challenges is essential for businesses utilizing synthetic data. By prioritizing data quality, privacy, and diversity, companies can fully harness the benefits of synthetic data generation techniques.
The Future of Synthetic Data: Emerging Trends
The future of synthetic data is promising, driven by ongoing AI advancements. With over 100 vendors now in the synthetic data market, the demand for both unstructured and structured data is skyrocketing. This surge is most notable in healthcare and finance, where applications are expanding swiftly.
Synthetic data generation is transforming how we construct datasets. It mirrors the statistical characteristics of original data but lacks real-world information. This innovation is crucial for pioneering research and development. For instance, in healthcare, researchers can now delve into patient data for insights while upholding privacy.
AI advancements are propelling the synthetic data revolution. Techniques such as generative adversarial networks (GANs) and variational autoencoders are evolving, mimicking real data with greater precision. This is essential for training intricate AI models and refining their adaptive abilities.
The future of synthetic data is marked by its scalability and cost-effectiveness. Companies can swiftly produce extensive datasets tailored to specific requirements, eliminating biases found in actual data. This method is not only more efficient but also fosters more equitable AI solutions. The influence of synthetic data is set to permeate various sectors, from automotive to retail.
"Synthetic data is the key to unlocking the full potential of AI while preserving privacy and reducing bias."
Looking forward, the fusion of synthetic data with federated learning and differential privacy will further bolster privacy-preserving machine learning solutions. This synergy will likely spur the next phase of AI breakthroughs, cementing synthetic data as a vital asset for model development and testing in the future.
Best Practices for Implementing Synthetic Data Generation Techniques
Implementing synthetic data generation techniques demands meticulous planning and execution. By adhering to synthetic data best practices, you can guarantee the success of your data generation efforts and integrate it smoothly into your workflow.
Selecting the Right Generation Method
It is essential to select a synthetic data generation method that suits your specific needs. Consider the type of data, its complexity, and how you plan to use it. Popular methods include GANs, VAEs, and Bayesian approaches. Each method has unique strengths, so assess them against your project's requirements.
Validating and Refining Synthetic Datasets
Thorough testing and validation are vital for ensuring data quality. Employ comprehensive metrics to evaluate the accuracy and utility of your synthetic data. The Synthetic Data Quality Report by Fabric provides insightful metrics for this purpose. Continually monitor and refine your generation process to maintain optimal performance.
Integrating Synthetic Data into Existing Workflows
Ensuring seamless integration of synthetic data is crucial for reaping its full benefits. Use tools like Fabric's Pipelines to construct, version, and refine data preparation solutions. This method facilitates efficient synthetic data generation and its incorporation into your current workflows.
When generating synthetic data, it is crucial to balance privacy, utility, and fidelity. By deeply understanding your original data, strategically choosing methods, and continuously evaluating them, you can successfully integrate synthetic data generation across various sectors, including healthcare and finance.
The Transformative Power of Advanced Synthetic Data Generation
The impact of synthetic data on AI transformation is profound. With 78% of companies facing data-related hurdles in AI, synthetic data generation emerges as a pivotal solution. It transcends mere data supplementation; it's about redefining how we approach data creation.
Synthetic data stands out as a crucial element in the AI arsenal. It ensures compliance with stringent privacy regulations such as GDPR and HIPAA. Moreover, it enriches machine learning models by introducing diverse data elements. This leads to enhanced performance, particularly when authentic data is scarce.
Adopting synthetic data is more than a fleeting trend; it's a strategic resource. It fuels growth, safeguards privacy, and expands AI's potential. The influence of synthetic data is evident—it's not just altering the game; it's rewriting the rules.
FAQ
What is synthetic data?
Synthetic data is artificially created datasets that mimic real-world data in terms of statistical properties and distributions. It's produced using algorithms, statistical models, or machine learning to replicate real data without actual observations.
Why is synthetic data important?
Synthetic data is crucial for addressing real data challenges like privacy concerns, high collection costs, and limited availability. It offers a cost-effective, scalable, and diverse solution for training and testing AI and machine learning models.
What are the key advantages of using synthetic data?
The main benefits of synthetic data include its cost-effectiveness, scalability, and diversity. It also provides greater control over data quality and preserves privacy by not using real, sensitive data.
How has synthetic data generation evolved over time?
Synthetic data generation has moved from simple rule-based methods to sophisticated model-based techniques. With advancements in machine learning, methods like Generative Adversarial Networks (GANs) and Variational Autoencoders (VAEs) have emerged, enabling highly realistic synthetic data creation.
What is the difference between rule-based and model-based synthetic data generation?
Rule-based techniques use explicit rules to create data that follows predefined patterns. Model-based approaches, on the other hand, use statistical models trained on real data to generate new samples. Hybrid techniques combine both for greater flexibility.
How do Generative Adversarial Networks (GANs) work in synthetic data generation?
GANs consist of two neural networks: a generator that creates synthetic data and a discriminator that distinguishes real from synthetic data. Through an iterative process, both networks improve, leading to increasingly realistic synthetic data.
What are some current state-of-the-art techniques for synthetic data generation?
Advanced techniques include Variational Autoencoders (VAEs), sophisticated statistical modeling, and AI-powered tools that blend deep learning and reinforcement learning. These methods create highly realistic and diverse synthetic datasets.
What are some applications of synthetic data across industries?
Synthetic data is used in healthcare for medical imaging analysis and patient diagnosis, in the automotive sector for testing autonomous vehicles, and in retail for inventory management and customer behavior analysis. It also aids in finance for fraud detection and risk assessment, and in training machine learning models.
What are some challenges in synthetic data generation?
Challenges include ensuring data quality and realism, addressing privacy concerns, and balancing diversity with representativeness. Creating high-quality synthetic data that accurately reflects real-world complexity while preserving privacy and avoiding biases is essential.
What emerging trends are shaping the future of synthetic data generation?
Emerging trends include integrating federated learning and differential privacy to enhance privacy-preserving machine learning. As AI expands into new areas, the demand for diverse and high-quality training datasets will grow, making synthetic data crucial.
What are some best practices for implementing synthetic data generation techniques?
Best practices include selecting the right method for your needs, validating and refining synthetic datasets, and ensuring data quality by comparing it to real-world benchmarks. It's important to maintain diversity to prevent bias and overfitting, prioritize privacy and security, and effectively integrate synthetic data into existing workflows.
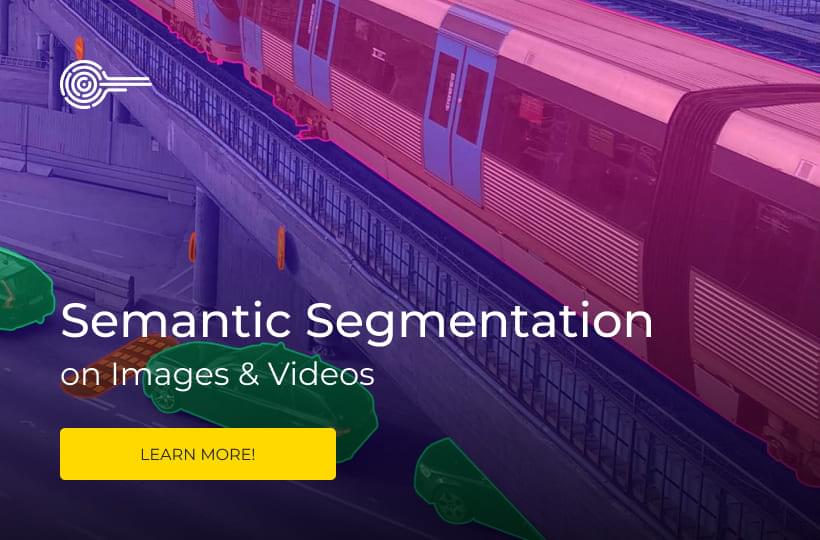