Advanced Error Analysis: Identifying and Correcting Annotation Mistakes
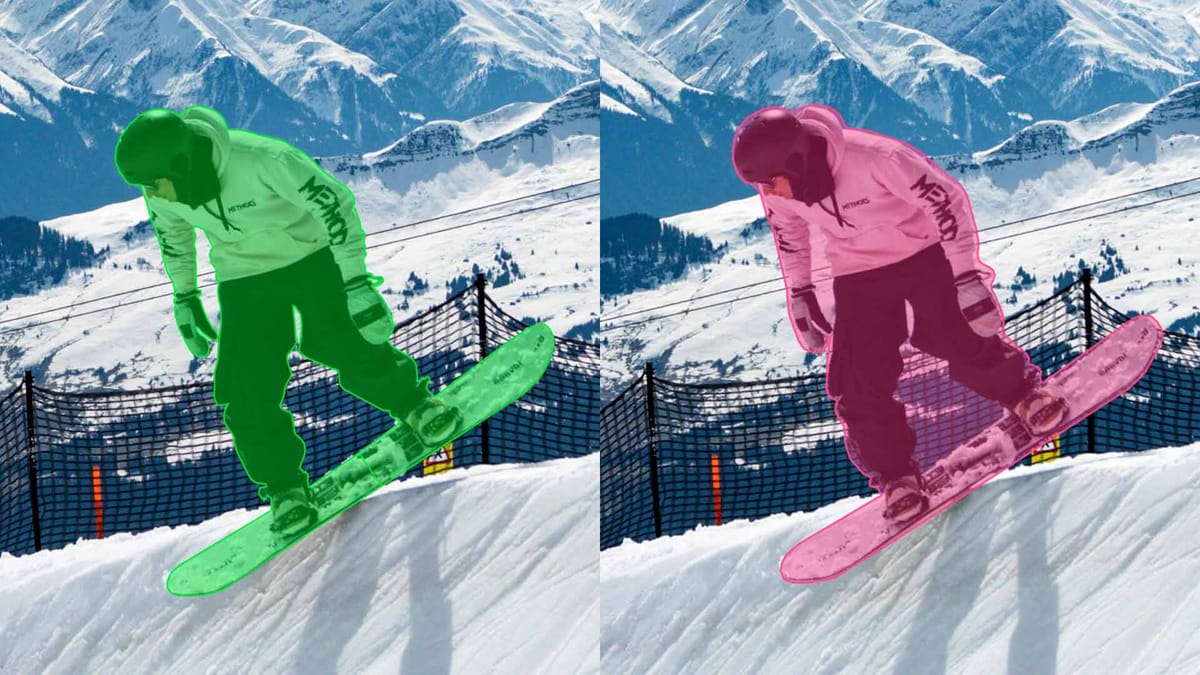
Data annotation plays an essential characteristic in satisfying AI and object detection applications. Incorrect annotations can cause setbacks in AI-installed systems, such as fault prediction in self-reliant automobiles or diagnostic accuracy in medical AI. Let’s explore sensible strategies and discuss the real-worldwide outcomes of annotation inaccuracies, putting the extent for an in-intensity speak on improving annotation practices and tools.
Key Takeaways
- Data annotation is critical for AI and machine learning success.
- Inaccurate data annotation can severely impact systems like autonomous vehicles and medical diagnostics.
- Automated tools for annotating sequence records increase error likelihood, requiring robust error analysis techniques.
- Integrating error analysis tools into annotation processes improves data quality and model accuracy.
- Real-world consequences of annotation errors underscore the need for precise and accurate data annotation.
- Practical strategies and practices are essential for improving AI training data quality.

Understanding Annotation Error Analysis
This consists of identifying, classifying, and correcting mistakes in movie pictures, substantially influencing the reliability and overall performance of AI models.
Definition and Importance
Annotation mistakes evaluation is set by reviewing annotated records to identify errors. This is crucial in healthcare, finance, and law, in which first-class records are essential. Errors can stem from misinterpreting guidelines, subjective views, or human mistakes. Implementing first-class control primarily based totally on mistaken evaluation advantages businesses. Tools like automated mistake detection or peer review can help. Evaluation of practical records annotation mistakes guarantees accuracy and consistency. It accelerates tasks and decreases remodel costs, enhancing records' first-class and reliability.
Common Types of Annotation Errors
- Incorrectly labeled data points.
- Incorrectly labeled attributes to features.
- Poor level of precision in annotation.
Studying mistakes helps evaluate community architectures like UNet, SegNet, and MSD in deep learning for molecular segmentation. Variances in segmentation annotations among annotators spotlight the need for consistent, excellent schooling facts for deep learning.
Techniques for Identifying Annotation Errors
There are two main methods, manual testing and automated troubleshooting tools. Each technique offers unique benefits, and when combined, they create a robust troubleshooting strategy.
Automated Error Detection Tools
Automated gear offers scalability and flexibility for error detection. It uses superior algorithms to experiment with large record units and effectively discover errors. Automatic first-class manipulation additionally simplifies error detection throughout datasets and exclusive tasks. Cleanlab methods, when used with a segmentation model, substantially enhance the detection of decided-on pictures in segmented datasets. This new technique is vital for preserving annotation first-class, integrating nicely with current workflows, and generating steady results.
Clear Annotation Guidelines
This guideline should provide explanations, real-world examples, and guidelines for resolving ambiguity, reducing disagreement between speakers, measuring agreement between speakers, and demonstrating the nature of the information.
Training Annotation Teams
Thorough annotator training is vital for excellent records labeling. Training will include realistic workshops, simulation exercises, and common assessments, keeping annotators updated with contemporary strategies and expectations. Quality control mechanisms, like peer evaluations and automatic checks, also are for preserving accuracy. They assist in aligning the team's know-how of guidelines, minimizing human mistakes, and improving records excellent. By adopting those first-rate practices, companies can ensure the accuracy of excessive record annotations. This results in extra dependable and independent AI models.
Tools and Technologies for Annotation Error Analysis
In the closing decade, the significance of information annotation has grown significantly. This is increasing, mainly because of the development of device mastering systems. As a result, the need for particular and green annotation mistake evaluation has become essential. The high satisfaction of annotations without delay influences the performance of device mastering models.
Integrating Error Analysis Tools
Integrating superior mistake evaluation equipment into annotation software is critical. It complements annotation accuracy and efficiency. Transform r-primarily based total models, added in 2017, have drastically advanced context information and long-variety dependencies in language tasks.
Technologies like reinforcement studying from human feedback (RLHF) are also being used. Manual annotation optimizes version effects by rating them with numerical entries. Professionals in numerous fields, which include self-sufficient vehicles, agricultural automation systems, and scientific imaging, depend closely on correct photograph class and item detection. As such, mistake evaluation equipment is vital to their annotation software suites.
Analysis of High-Stakes Industries
In healthcare, the accuracy of clinical records annotation is vital. One study determined 58 658 reviews of medicine mistakes out of 121,244, displaying the excess danger of annotation mistakes. Human evaluation is key, as datasets blanketed 478,175 entities associated with medicine mistakes, attaining F1-ratings of 0.97 and 0.76 in particular areas.
In autonomous driving, annotation errors may have dire outcomes. The Open Images dataset confirmed that around 36% of fake positives were caused by mistakes. The 2019 Open Images Detection Challenge highlighted how moderate implied standard precision (mAP) variations can appreciably affect version performance.
lessons Learned from Past Errors
It's essential to research beyond annotation errors to enhance destiny practices. Studies display that combining human and device efforts is critical for correcting mistakes. Error correction needs an intensive approach.
These examples underscore the significance of gaining knowledge beyond e errors to decorate annotation methods. Annotations are greater than information quality; they may be essential to AI success, particularly in high-hazard areas. By usually inspecting and gaining knowledge from mistakes, groups can decorate annotation precision, leading to more secure and more powerful AI applications.
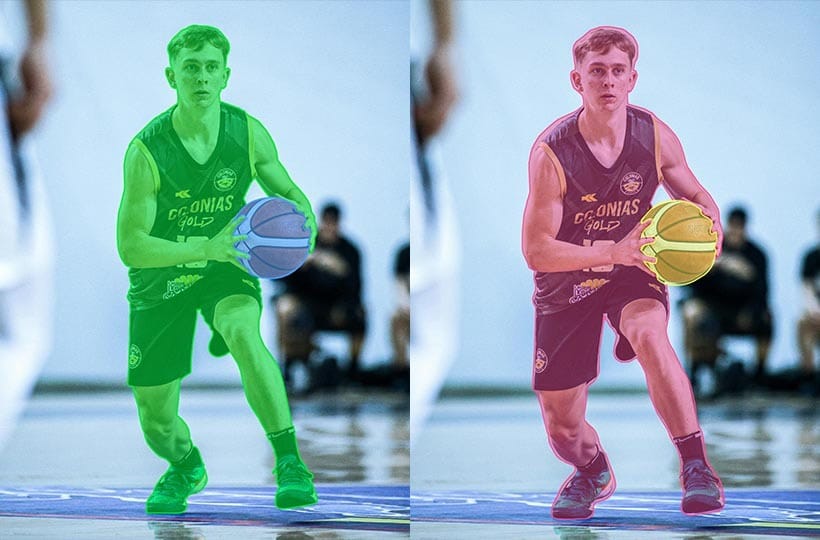
Implementing Continuous Feedback Loops
Continuous remark loops are critical for maintaining high annotation standards. These loops contain daily critiques and updates based totally on instant remarks, enabling spot and connect mistakes early on. A dependent remarks technique can considerably lessen charges and rebuild considered misplaced or mislabeled data.
- Real-time remarks improve annotation accuracy.
- Automated gear can expedite the labeling process.
- Data audits in sectors like felony companies assist in keeping quality.
Encouraging Collaborative Efforts
Collaboration among facts scientists, engineers, and area professionals is vital for addressing inaccuracies. When two annotators paint together, the danger of human biases and inconsistencies drops significantly. Training applications and clean suggestions for facts annotation are crucial for accomplishing those goals. Creating a tradition of collaboration guarantees an extra dependable and error-unfastened facts annotation process. A look at well-written annotation manuals indicates how they are able to standardize practices and decrease subjective variations amongst collaborators.
Collaborative annotation Reduces human biases and improves data consistency.
Automated Tools Speeds up the data labeling process efficiently.
Training Programs Ensures standardized processes and reduces labeling disparities.
Revision Processes or Annotations
The revision methods for annotations contain numerous steps to become aware of and accurately correct mistakes systematically. An essential approach is reevaluation, in which annotated information is periodically reviewed in opposition to required requirements. This manner consists of each guide and automatic reviews:
- Manual Review. Experts manually investigate annotated information, evaluating it with baseline requirements and jousting inconsistencies.
- Automated verification. AI equipment spotlights feasible inaccuracies inside the dataset primarily based totally on found-out error patterns.
Prioritizing Error Corrections
Prioritizing error corrections is vital for efficient resource allocation and first addressing the most impactful faults. This prioritization can be based on several factors:
- Impact on Model Performance. Errors that substantially modify consequences must be addressed immediately. For example, mislabeling in essential classes like healthcare may have extreme consequences.
- Frequency of Errors. Common mistakes affecting large quantities of the dataset must be resolved to prevent considerable inaccuracies.
- Complexity of Correction. Simple mistakes may be speedily fixed, considering an unbroken development in statistics quality.
In conclusion, adopting robust revision strategies and prioritizing mistakes can significantly improve the precision and accuracy of your annotated datasets. Regular statistics reevaluation and prioritized correction procedures ensure sustained development in AI-pushed insights.
Expected Developments in Error Detection Technology
Future mistake detection generation will introduce new approaches to visualize and examine mistake patterns. This will assist us in spotting biases or anomalies in facts. For example, anomaly detection has already exposed great facts and first-class troubles by specializing in massive deviations from the mean.
New gear will permit real-time validation, elevating facts accuracy and reliability standards. As tech evolves, the focal point will shift to post-prediction analysis. This will make specific versions of equity and align with moral and cultural values. The destiny of annotation appears promising, with AI and mistake-detection tech on the way.
We're transferring closer to wholly automated, fantastically correct systems. Mastering specific annotation strategies for a successful device and gaining knowledge of initiatives can't be overstated.
FAQ
What is annotation error analysis, and why is it important?
Annotation blunders evaluation identifies and fixes errors in statistics tagging for AI version training. It guarantees the statistics software and accuracy, affecting version reliability. AI packages might not be carried out reliably without the proper blunders evaluation, leading to misguided results.
What are common types of annotation errors?
Common annotation errors include data misclassification due to human subjectivity or language and image ambiguities. These errors lead to inconsistent AI behavior.
How do annotation errors affect machine learning models?
Annotation mistakes can lead to erroneous AI predictions, which poses substantial risks, especially in critical regions like healthcare and finance. For instance, in scientific imaging, wrong annotations can cause misdiagnoses, impacting affected person outcomes.
What is the impact of annotation errors on research outcomes?
Poor data quality from annotation errors can undermine the integrity of study findings. This impacts secondary packages like predictive analytics and consumer experience enhancements. High-quality annotations are crucial for dependable AI version education and correct study results.
What are the methods for identifying annotation errors?
The two main methods are manual review by human experts and automated troubleshooting tools. Manual reviews provide greater accuracy and contextual insights. Automated tools scan large data sets for errors and provide scale and consistency.
How can annotation accuracy be improved?
Improvement involves setting clear, detailed annotation guidelines and extensive training for annotators. Regular assessment and calibration exercises are key to maintaining high annotation accuracy standards.
What tools and technologies are available for annotation error analysis?
Popular annotation software program consists of AI-assisted gear that advocates possible annotations to hurry up the process. This reduces human workload and mistakes rate. Integrating mistake evaluation gear into current strategies complements annotation capabilities.
What are some examples of real-life annotation error scenarios?
Annotation errors have caused severe consequences in high-stakes industries like healthcare and autonomous driving. Case studies show how past mistakes have improved annotation practices and solutions, highlighting the critical need for accuracy.
How does feedback help in reducing annotation errors?
Continuous feedback loops and collaborative efforts among annotators improve annotation accuracy. Sharing real-time insights and corrections helps create more reliable data sets for AI model training.
What are the strategies for correcting annotation mistakes?
Strategies include structured methods for revising faulty annotations and setting priorities for error correction based on their impact on AI performance. Systematized approaches to re-evaluate and update annotated datasets ensure ongoing relevance and accuracy.
What future trends are expected in annotation error analysis?
Future developments will likely feature advanced AI applications that lead to sophisticated tools for detecting, analyzing, and correcting annotation errors. The aim is to achieve fully automated, high-accuracy AI-driven systems that improve data annotation processes.
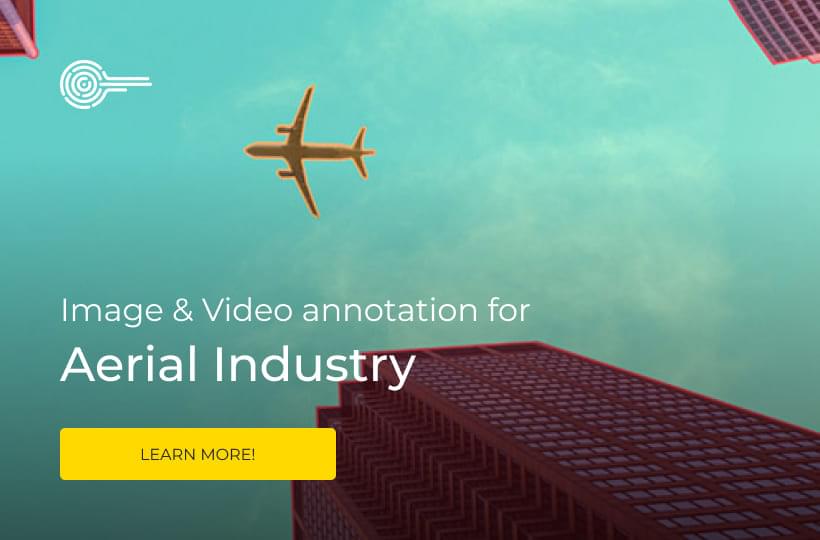