Bias Challenges, Data Annotation and Precision Agriculture
Computer vision AI is helping farmers to improve yields and strengthen the global food supply. Advances in machine learning have led to a range of applications that help growers to monitor and protect their crops.
However, these important use cases are made possible by data annotation, the process that creates training images that AI models learn from. If this training data does not reflect the reality of real-world agriculture then AI models may not work as well as they could. Overcoming bias in training data will help precision agriculture AI models to reach their potential.
In this blog we will first look at the important AI applications that are being introduced to the agriculture industry. Secondly we will examine specific bias challenges. And finally we will show how data annotation services can help developers find the right images.
Exciting applications
The following use cases for AI in the agricultural sector promise to increase the efficiency and profitability of crop production:
- Aerial crop monitoring: Drones, powered by AI systems, can give farmers an accurate picture of their crops. These machines can autonomously monitor large areas of farmland and provide valuable information to growers, such as coloration and density. Farmers could also use drones to spray crops in hard to reach areas.
- Growth monitoring: AI models can detect changes in plant size and colour with the help of data annotation. AI systems with this capability can remotely monitor crops and provide early warning of poor plant health, lack of water and nutrient deficiency.
- Ripeness detection: It is vital for farmers that fruit crops are picked at the correct level of ripeness. AI systems can identify the colour of fruit and then assess how ripe they are. When the fruit has sufficiently ripened alerts can be sent to growers ensuring optimal yields.
- Disease detection: Crop monitoring systems can be trained to recognise harmful diseases and parasites. When the tell-tale signs of disease present themselves on leaves or fruit AI models can alert farmers who can act to protect their crops.
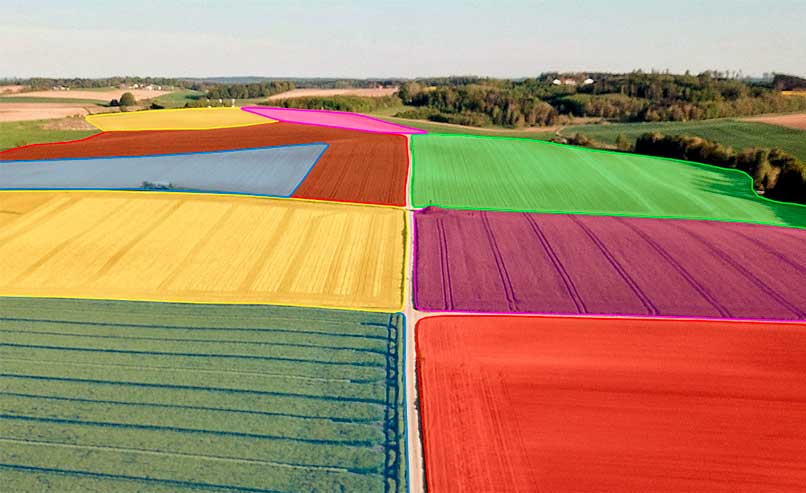
Varied data that reflects the real world
In order to be effective training data for precision agriculture needs to be varied. The following factors are important for developers to consider if they want to overcome bias:
- Differences in climate: Different growing regions of the world have different climates. This can affect weather conditions and light levels. Models that are trained with images from one climatic region may work less well when presented with images from elsewhere.
- Diverse landscapes: Farming landscapes can look vastly different between countries. A model that works in the fields of North America may not function as well in Asia. It is important that training data reflects these varied environments.
- Visibility and light levels: Crop monitoring systems need to be able to work in low light conditions and in poor weather. To achieve this training images must reflect varied visibility conditions.
Finding the right image quality
Finding the right images can be a challenge for precision agriculture developers. As a result many innovators in this sector turn to data annotation services, like Keymakr:
- Data collection: Data annotation providers are experts at finding the right training images from publicly available sources. Keymakr has a wealth of knowledge when it comes to finding high quality training images in online repositories or with web scraping tools.
- Data creation: Sometimes it is not possible to find the right training images for your AI model. As a result Keymakr has developed in-house data creation facilities that allows AI companies to craft highly specific training datasets.
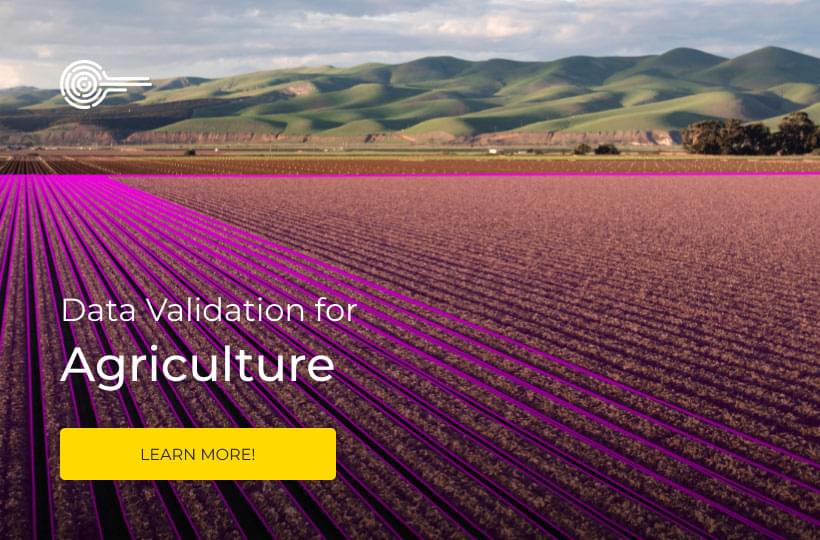