Unlocking Data Annotation: Best Practices Guide
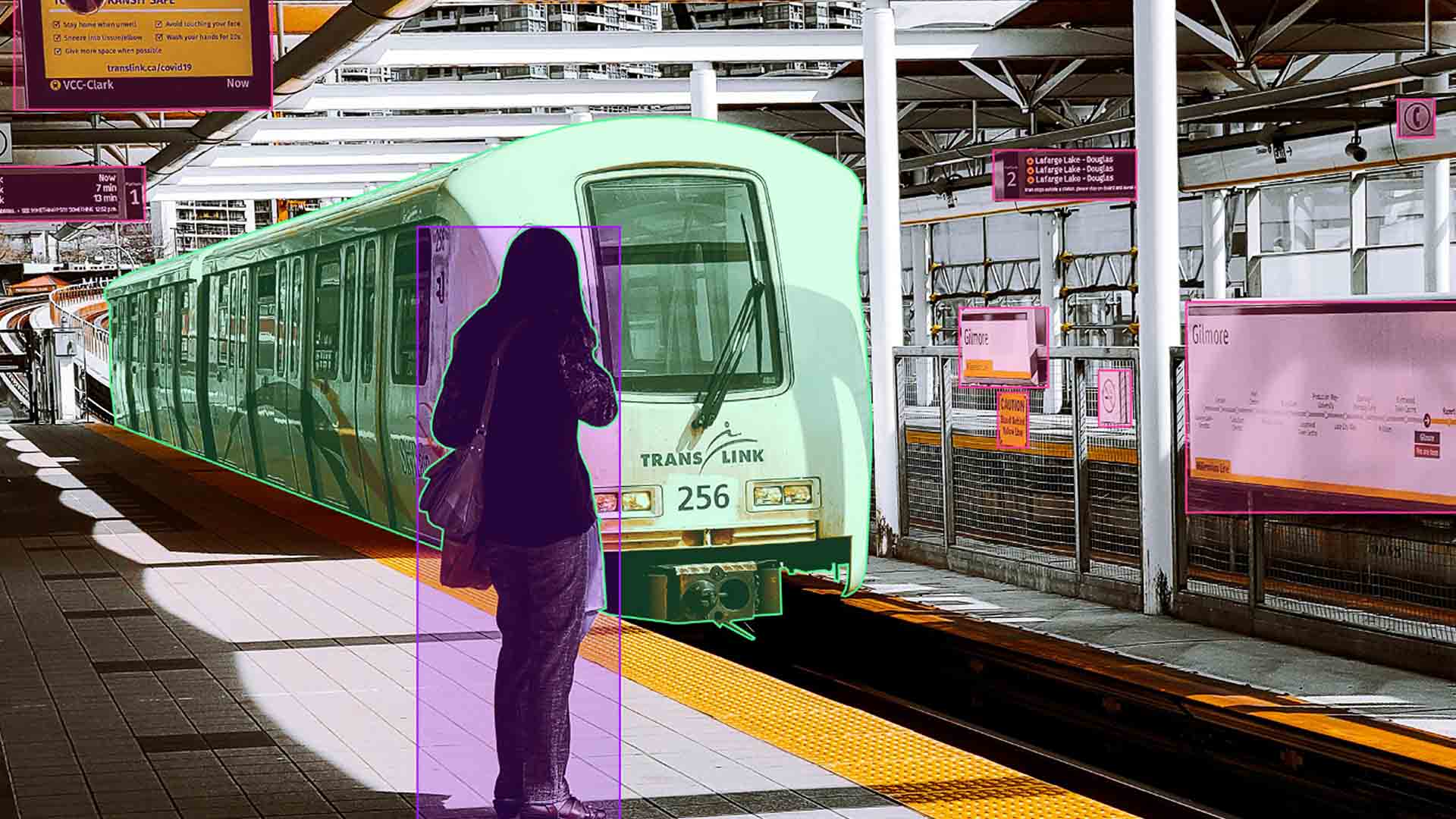
By 2025, nearly every company will use artificial intelligence. Data annotation plays a key role, helping create machine learning models. These models change how we work. They rely on labeled data to learn and make decisions. This work combines precision, foresight, and strategy.
Think about a market growing 26.5% every year until 2030. It’s set to reach USD 5,331.0 million. This shows how crucial well-annotated data is. It drives innovation and efficiency in AI projects. Data annotation powers everything from healthcare to self-driving cars.
Data annotation affects more than just machine learning. It's key to creating lifelike games, improving health treatments, and personalizing shopping recommendations. To succeed in AI, mastering data annotation is essential.
Key Takeaways
- Every enterprise needs to understand and implement best practices for data annotation to stay competitive in the AI-powered future.
- Data annotation significantly contributes to the efficiency and accuracy of machine learning models, impacting various sectors such as healthcare, retail, and transportation.
- While the art of data annotation is complex, it is critical for the generation of reliable, data-driven insights that drive AI innovation.
- Organizations that follow data annotation best practices are likely to outperform their competitors in project success rates and model accuracy.
- Understanding the growth, scope, and impact of data annotation is crucial for any business invested in or planning to embark on AI projects.

Understanding the Value of Accurate Data Annotation
Data annotation is key for machine learning and AI to work well. It helps them understand and learn from lots of raw data. So, making sure data is annotated accurately and precisely is vital. This makes AI more reliable and wise. It also cuts down on biases and betters the results of machine learning.
When it comes to labeling data, it’s important to follow clear guidelines that fit your project's goals. The tools you use should protect data privacy and be easy to use. They should also work well with the AI systems you’re using.
Focusing on detailed data annotation can greatly enhance how AI systems perform. It helps businesses extract valuable insights from complex data. This improvement leads to better decision-making. It also gives them an edge in the technology-driven market.
Best Practices for Data Annotation
Data annotation is key for the success of machine learning. It involves smart data tagging, careful project management, and using efficient methods. With these, raw data turns into valuable sets that boost the precision and usefulness of algorithms.
The Role of Clear Annotation Guidelines
Having clear annotation guidelines is crucial for project management. They serve as a map for annotators, making sure data is tagged in line with what the models need. A strong guideline system includes detailed definitions, examples, and instructions for handling tricky cases. This reduces confusion and makes the marked data better.
Quality Assurance: Ensuring High Standards
Quality checks in data annotation make machine learning models more reliable. Setting tough quality standards and using agreed-upon metrics helps keep data right. Regular checks and open feedback boost the process over time, keeping it effective and the models top-notch.
Employing Advanced Annotation Tools
Using top-notch annotation tools improves the annotation process. These tools should be easy to use, keep data safe, and work well with big data sets. They should allow for collaboration, make tracking projects easy, and merge with other systems. This makes annotation smooth and productive.
Focusing on these best practices not just enhances data quality but also speeds up machine learning training. This leads to better AI use and new innovations. As AI develops, keeping up with data annotation strategies and tech is vital for data-focused groups using machine learning.
Choosing the Right Data Annotation Tool
AI technologies are advancing fast. So, picking a top-notch data annotation tool is vital. These tools provide the data-driven insights necessary for training advanced machine learning systems. It's key to look for a tool that meets your project's needs and has high accuracy guidelines for data annotation.
Your choice will really affect how well and smoothly you label data. Make sure the tool is secure to guard private info. It should also work well with your current setup.
Here are key points and features to consider when picking a data annotation tool:
- Dataset Management Features: It's important to find tools with strong dataset management. They should offer ways to search, filter, merge data. They should also work with various file storage types.
- Annotation Techniques: Choose a tool that can handle many types of annotation. This includes image, video, and text annotation for different data formats like JSON, XML, and CSV.
- Workforce Management: Opt for tools that make managing your team easier. They should allow for easy task assignment, time tracking, and using resources well.
- Security Protocols: Look for tools that take security seriously. They should protect your data from unauthorized access to keep it safe.
To get a clear picture of how tools compare, do a comparison analysis:
Feature | Tool A | Tool B | Tool C |
---|---|---|---|
Dataset Management | Advanced | Basic | Moderate |
Annotation Types Supported | Image, Video, Text | Image, Video | Text |
Security Measures | High | Medium | Low |
Pricing Flexibility | Yes | No | Yes |
Choosing the right tool is a big decision that needs careful thought. Understand what your project needs, and compare it with what tools offer. The best tool will help your team gain data-driven insights accurately. This will aid in building trustworthy and effective AI solutions.
Data Labeling Tips for Efficient Annotation Workflows
Integrating AI-supported data labeling tips improves workflow speed and model accuracy. These methods make operations smoother and boost quality in machine learning models.
Using AI tools for efficient data annotation is smart. They allow for quick and accurate labeling, especially in tough datasets. This saves time and effort, letting annotators handle more complex data issues.
Feature | Benefits | Impact on Machine Learning Model Accuracy |
---|---|---|
AI-driven automation | Reduces manual labeling workload | Enhances learning speed and accuracy |
Multi-object tracking | Improves data consistency across frames | Higher precision in object recognition tasks |
Collaborative annotation capabilities | Enables real-time teamwork and error correction | Refines data quality and model reliability |
By using innovative techniques, your workflow becomes more flexible. This is critical for projects needing quick machine learning model application. Such tools lead to faster, more precise work, key for top results in machine learning.
Data Tagging Strategies for Diverse Data Sets
Using good data tagging strategies is key. It helps make machine learning models better. Good strategies work well for many types of people, avoiding unfair results. This also makes AI more accurate and fair across different fields.
For top-notch guidelines for data annotation accuracy, you must look at different things. You need a clear goal, know how much data you need, and where to find it. This makes sure your tagging suits your needs perfectly.
Integrating advanced tech and human skills into data tagging is a big plus. Human-in-the-loop (HITL) and active learning techniques speed up work and make it more accurate. They help create top-quality training info for complex models.
Aspect | Importance | Impact on ML Models |
---|---|---|
Variety in Data | High | Prevents bias, enhances real-world applicability |
Data Annotation Tools | Critical | Ensures efficient and accurate tagging |
Human Expertise | Essential | Introduces contextual understanding |
Active Learning Techniques | Beneficial | Speeds up the annotation process |
Creating clear guidelines for data annotation accuracy helps avoid mistakes. Using various data sources and keeping a high standard in tagging is crucial. This ensures the insights we get are accurate and useful in many areas. It helps make AI projects better and more effective.
Quality Assurance in Data Annotation
Good annotation boosts model accuracy, improves workflow, and fights bias. We'll explore how to set up strong review cycles and keep annotation precise as projects change.
Setting Up Review and Feedback Cycles
High data quality demands regular reviews and feedback. These serve as important stops to check annotations closely and fix errors quickly. Such checks ensure data tags are reliable and help produce trustworthy machine learning results. Feedback also spurs on-going improvements in how data is annotated.
Addressing Bias and Maintaining Annotation Accuracy
Keeping annotated data unbiased is critical for fair machine learning results. It's done by using diverse data and rigorous reviews. This ensures each piece of data is checked for bias, keeping annotations top-notch. Such care is vital for creating effective and fair machine learning models.
Using advanced technologies can boost annotation quality. Systems based on neural networks can help review annotations on new data quickly and more accurately. This tech not only saves time but also provides key insights for project betterment.
Skilled annotators and evolving quality benchmarks are fundamental. They lift the quality of data notably. With constant learning, annotation teams can surpass the quality needs of modern machine learning models.
When working on data annotation, remember that a solid quality assurance plan is fundamental. Meticulous reviews, addressing bias, and using the latest tools will guide your AI projects to success.
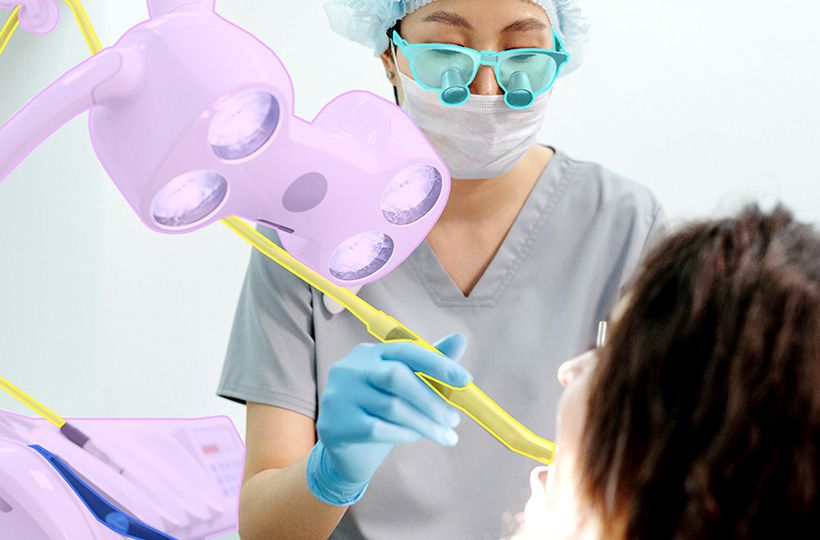
Annotation Workflow Optimization
Optimizing your work with annotations is key to better results. It means making everything work together smoothly. You need the right tools, a well-organized team, and clear rules to ensure data is correct. These steps help work on projects with a lot of data, making sure it's done accurately and quickly.
Efficient data annotation methods help speed things up. Using the best tools and fitting them into your way of working brings big improvements. These tools make working together easier and help with managing the whole project well.
Getting the guidelines right is crucial for annotation workflow optimization. They need to be clear and always up to date. This keeps everyone on the same page and helps maintain quality. It's also important to check the work closely to make sure it's reliable.
Feature | Impact on Annotation Workflow |
---|---|
Advanced Annotation Tools | Enhance speed and accuracy, support a wide range of data types and formats. |
Skilled Annotation Team | Ensures consistency, leverages expertise to handle complex data scenarios. |
Clear, Precise Guidelines | Maintain data integrity, guide annotators to achieve uniform annotation standards. |
Quality Assurance Protocols | Monitor and maintain the quality of data annotations, detect inconsistencies. |
Improving how you work with annotations is vital for AI projects' success. By using good methods and managing the work well, you pave the way for top-notch data. This data is crucial for training AI models you can trust.
Summary
Enterprises face a sea of digital documents, from invoices to health records. Document AI becomes a key assistant in this digital journey. With precise data annotation, these solutions are able to classify documents and extract important information. This process turns scattered data into valuable insights, pushing AI and machine learning to higher levels of performance.
Following detailed annotation guidelines is crucial for high-quality Document AI. Companies focus on training and ensuring the accuracy of annotations. By regularly improving their methods through teamwork and communication, they achieve better outcomes. This continuous improvement cycle adapts to the changing AI landscape while respecting privacy laws.
The mix of clear guidelines, thorough training, and careful quality checks leads to excellence in data annotation. As someone leading AI implementation, embracing these approaches is essential. Your company will not just excel in AI but also maintain a competitive advantage in a future that prizes accurate, adaptable, and insightful use of data.
FAQ
What are the best practices for data annotation in developing machine learning models?
Establishing clear annotation rules is key. It's crucial to check the quality often. Use advanced tools and manage your team well. Strategic tagging and regular quality checks are a must. This keeps your data accurate.
Why is accurate data annotation so valuable for AI projects?
Accurate data annotation is key for AI success. It offers the correct starting point for machine learning. AI learns accurately from well-labeled, reliable data. This improves its predictions greatly.
How do clear annotation guidelines influence data annotation?
Clear guidelines help annotators stay on track. They make sure annotations match the project's goals. Good guidelines keep data quality high. This is vital for the machine learning model.
What role does quality assurance play in data annotation?
Quality assurance is critical. It checks the data for the right standards. It fixes any errors found. This guarantees data used is trustworthy for training models.
Can you recommend some advanced annotation tools?
Tools like Keylabs are great picks. They blend powerful tools with ease of use. They support automation and teamwork well. Choose tools that fit your project's needs.
What are the key considerations when choosing a data annotation tool?
Consider goals, data safety, and ease of use. Ensure it fits your current setup smoothly. A good tool supports large-scale projects. It should help make annotation easy and efficient.
What tips can streamline the data annotation workflow?
Use tools that help automate and tag data quickly. Organize tasks in a simple way and process in batches. Set clear priorities and encourage teamwork for better speed and quality.
How should an efficient data annotation team be structured?
An ideal team mixes skilled annotators and reviewers. Roles should be clear. Regular training and strong communication are essential. Good management strategies keep the work consistent and of high quality.
What strategies can be employed for effective data tagging?
For good tagging, know the dataset and set diverse standards. Prevent bias by striving for fairness. Always refine your criteria and check tags to make sure they're accurate.
How are review and feedback cycles set up for quality assurance in data annotation?
Create regular review periods and appoint skilled reviewers. Use efficient feedback tools. Develop a clear process for correcting and updating data as needed.
Why is it important to address bias in data annotation and how can this be achieved?
Eliminating bias ensures your models are fair and effective. Include a variety of data and regularly audit for biases. Train annotators against bias and check data often to remove any bias.
What does optimizing an annotation workflow entail?
Optimizing means using the right tools and streamlining processes. Good communication is vital. Manage your team and time well to meet your project's goals.
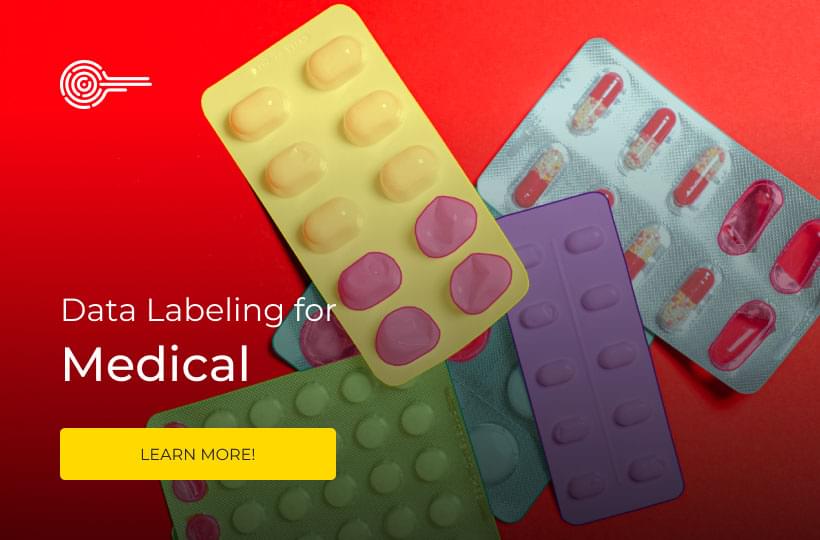