The Ultimate Annotation Guide for Machine Learning
Think about a world where AI is everywhere, from our jobs to our daily tasks. This future is closer than we think. The field that helps AI learn, called annotation, is growing fast. Experts predict it will keep growing by 26.5% every year, reaching a market value of over USD 5,331.0 million by 2030. This big jump shows how important machine learning annotation tools will become.
Want to push AI further? Then, knowing about machine learning annotation is key. Making sure the data AI learns from is right is the first step. This guide shines a light on data labeling tools, focusing on image annotation techniques and Supervised Learning.
Key Takeaways
- Comprehend the rapid expansion of the annotation market and its impact on AI's future.
- Grasp the pivotal role of high-quality data labeling in vital industries.
- Explore standard datasets like COCO and ImageNet that bolster machine learning.
- Understand the substantial growth in machine learning techniques including Supervised and Unsupervised Learning.
- Recognize the essentiality of text, image, audio, and 3D sensor data annotation for advancing AI.
- Anticipate the increasing need for robust annotation tools suited for every AI enterprise.

Understanding the Role of Annotation in Machine Learning
In machine learning, a model's success depends on the data it uses. This is why image annotation techniques and supervised learning data labeling are key. They make models more accurate. They also help in areas like medical imaging and self-driving cars work better and safer.
The Importance of High-Quality Annotations
Good annotations make machine learning models smarter. In medical images, for example, detailed notes help diagnose diseases. This leads to better treatment outcomes. This kind of work, supervised data labeling, adds real-world details to each piece of data.
Customization of Annotations for Specific Use Cases
Custom image annotation techniques meet unique needs in different fields. In self-driving cars, precise annotations of things like cars, people, and signs mean safer tech. In farming, spotting crop diseases from above boosts yields and management. These adjustments help models work better for specific tasks.
Tailored annotations are refined over time, based on how well the model does. This ongoing work keeps data consistent and helps models learn about new situations.
Exploring Image Annotation Techniques
Learning about image annotation techniques is key as we explore machine learning. These methods help algorithms find and identify objects in images well. They are crucial in fields like self-driving cars and medical imaging.
Bounding box annotation and polygon annotation are important techniques. Bounding box annotation is quick and easy for putting a box around an object. This is perfect when you need to be fast and simple, like in many apps.
But sometimes, objects don't fit into neat boxes. Polygon annotation lets us draw complex shapes around objects. It's great for things with unique shapes, like a building or machine part. This method takes more time but can be very accurate for certain needs.
- Bounding Box Annotation: Often used in retail and surveillance to quickly find and follow objects.
- Rotating Bounding Box Annotation: More advanced form of bounding box that takes orientation into account.
- Polygon Annotation: Better for areas like GIS and self-driving cars to map landscapes and cities precisely.
- Cuboid Annotation: Allows extrapolations of 3D space from 2D images.
- Lane Annotation: Labels roads, paths, conveyor belts, and so on. Handy in logistics.
- Skeletal Annotation: Used for people and animals to keep track of movement in realistic situations.
- Key Point Annotation: Fine form of annotation used for more detailed things like facial expressions.
- 3D Point Cloud Annotation: Uses data from a lidar to label relationships between objects in a 3D space.
Choosing the right image annotation technique depends on your project's goals. You should think about the balance between quickness and detail. This lets you match the method to your needs.
Although tools are getting smarter, humans are still needed in the image annotation process. Leaders in the industry keep making better tools to help people label images faster and more accurately. This speeds up projects and makes our models better.
Deciding between simpler methods like bounding boxes and more complex methods like polygons matters. It affects how well your model can learn. Keeping up with the newest annotation methods will help your work stay ahead.
The Significance of Text Annotation in NLP
In tech, Natural Language Processing (NLP) helps computers understand and talk like us. This changes how we interact with technology and use data. Text annotation is key. It turns raw text into a form NLP can use, teaching machines to get our language right.
It's crucial to have good guidelines for text annotation. These guidelines should be strong and flexible. They help label text well, teaching NLP systems to understand language deeply. This training makes the models good at finding meaning, emotion, and intention in text.
Text Annotation Tasks: From Classification to Summarization
There are many text annotation tasks. These tasks help NLP systems do their job well. For example, text classification sorts texts into types based on content or tone. Sentiment annotation looks for positive, negative, or neutral feelings in text.
Named entity recognition (NER) is also important. It finds and labels specific people, places, or organizations. This helps with tasks like understanding the relationships between different things. It's crucial for projects like making knowledge graphs or understanding complex texts.
Navigating the Challenges in Data Annotation
Data annotation really affects the success of AI projects. Solving these problems is key. It makes AI and machine learning work better, from diagnosing diseases to self-driving cars.
Managing Inclusion of Background in Bounding Boxes
In image annotation, a big issue is including too much of the background. This can mess up the model's learning. To solve this, annotators need to decide what part of the image really matters. They should use tools that help them be precise, reducing unnecessary background.
Handling Ambiguity and Context in Sentiment Annotation
Pinpointing emotion or opinion in text is tricky because it's often unclear. Doing this well means understanding the text's deeper meaning and its cultural background. Training and constant quality checks are recommended to improve sentiment analysis.
By taking these steps, we make sure the data training AI is clear and accurate. This is crucial for creating dependable machine learning models.
Annotation Guide for Machine Learning: Best Practices
In the fast-growing world of machine learning, following best practices for data annotation is key. The value of data annotation is expected to hit USD 5,331.0 million by 2030. This makes getting data annotation right more important than ever. Here are some tips to improve your machine learning work.
- Meticulous Quality Control: Make sure every annotated item is checked twice. Good quality control reduces the chance of mistakes. These mistakes could mess up the model.
- Contextual Relevance in Text Data: Look closely at the details in text data. Understanding and annotating the context makes AI systems better at processing language.
- Consistency Across Annotations: Keeping annotations consistent maintains data set integrity. It makes sets reliable for training algorithms. This is crucial when many people are annotating.
- Frequent Validation: Regularly check and validate your data. This ensures it accurately represents the real world. It makes your model work better.
- Diverse Data Sets: Use a variety of data sets. This helps your AI models work well in many situations.
Industries like healthcare, autonomous vehicles, and finance need precise annotations. These help with tasks such as recognizing faces and understanding feelings. Focusing on best practices for data annotation improves the performance and accuracy of these applications.
To see why data annotation is so important, think about how different industries use it:
Industry | Key Annotation Applications |
---|---|
Healthcare | Medical image analysis, patient data management. |
Autonomous Vehicles | Object detection for navigation systems. |
Retail | Customer sentiment analysis, product categorization. |
Agriculture | Crop and field analysis through aerial data. |
The annotation market is growing at a rate of 26.5% yearly. It's crucial to use these best practices for data annotation. Doing so will improve your models. It will also get your business ready to use AI effectively by 2025.
Adoption of Machine Learning Annotation Tools
Adding machine learning annotation tools to your AI work is a big move. It's all about making automation better. You need to pick a tool that meets your needs. If you work with lots of data or have complex images, these tools are key for top-notch results.
Selecting the Right Annotation Tool
Finding the right data labeling tools for AI is crucial. It depends on what your project needs and how detailed your annotations must be. For tasks like autonomous driving or medical images, look for tools that can add polygon annotations. This lets you mark out objects more precisely. And that’s important for teaching models effectively.
Streamlining Annotating Process with Automation
Using machine learning annotation tools can speed up the labeling work. Data labeling tools for AI that automate these processes are a game-changer. They make marking data faster and more uniform. This means better learning models. Plus, they free up time for the team to handle more important jobs.
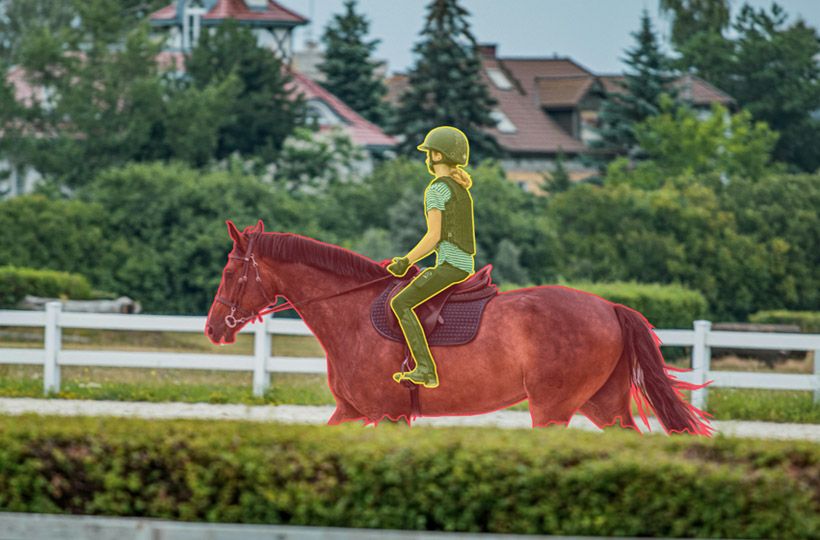
Image Annotation Techniques for Supervised Learning
In supervised learning, how we label data with image annotation is key. It helps machine learning models be more accurate. This is important for many tasks, like spotting faces or driving cars by themselves. When picking between polygon and bounding box annotations, it depends on what the dataset needs and how detailed you must be.
Polygon vs Bounding Box: Choosing the Right Technique
Need to outline things that are not a simple shape? Polygon annotations are perfect. They're great for areas like health and self-driving cars. But, using polygons takes more time because of their complexity. On the flip side, bounding boxes are quicker and simpler. They work well when you just need to find objects, like in basic model training or with simple shapes.
Effective Text Annotation Guidelines for Model Accuracy
Good text annotation rules are crucial for making sure models work well in machine learning. They help data annotators give right and useful info. They focus on marking information like feelings, meanings, and the topic in the text. This makes the machine smarter.
When you make text annotation guidelines, match them to your model's needs. It helps if you explain with clear meanings and examples. This greatly helps those labeling data. They then know what to do for tasks like spotting names or figuring out if text is positive or negative.
Understanding how to mark text is key. Things like spotting 'entities' and classifying text into types need clear steps. This is to make sure tasks are done the same way across different texts. A simple table can help a lot:
Annotation Type | Description | Application Example |
---|---|---|
Entity Annotation | Identification and labeling of specific entities within the text | Labeling names, companies, or locations in customer feedback |
Sentiment Annotation | Classifying the sentiment of the text segment | Determining customer sentiment from reviews as positive, negative, or neutral |
Intent Annotation | Categorizing text based on the underlying intent | Sorting customer emails into complaints, inquiries, or feedback |
Text Classification | Assigning predefined categories to a text segment | Categorizing news articles into sports, politics, or entertainment |
Say things in a way everyone understands. Avoid using fancy terms in your text annotation guidelines. This makes annotations quicker and cuts down on mistakes.
The clearer you are, the better your model works. Add lots of details and examples to help your team mark data right. This improves your model's smarts a lot.
It’s not just making tags. It's about creating a strong base for machines to really get what complex human language means.
Advancements in Data Labeling Tools for AI
The need for better AI is growing. So is the need for improved data labeling tools. These tools make labeling data faster and more accurate. They are key in managing a lot of unstructured data like text, images, and audio.
AI is now part of these tools, making everything quicker and more precise. This is important for teaching AI models correctly.
Incorporating AI to Simplify Data Labeling
Today, data labeling tools are becoming powered by AI. They help by automating tasks and improving the data annotation process. Platforms like Keylabs use AI to predict labels, which are checked by humans. This mix of AI and human work improves the quality of training data.
The Evolution of Annotation Tools and Their Impact
Annotation tools have come a long way, from manual to AI-driven. Older tools were simple and could only label images and categorize text. But now, these tools can handle complex video and object detection. This is crucial for AI in fields like self-driving cars and surveillance.
These new tools make it faster to label data. For example, one study showed they can cut time spent labeling by 50%. This speeds up the release of AI products and saves money on data prep.
So, improving data labeling tools is helping organizations start AI projects more easily. As these tools get better, they open up new possibilities for AI.
Summary
We're at the end of our guide on machine learning annotation. You've learned a lot about how important and vast this field is. The annotation market is growing fast, with an expected worth of USD 5,331.0 million by 2030.
AI needs well-annotated data to work well, whether it's for driving cars or making healthcare better. This shows how crucial good data labeling is. This is why every field is focusing on improving data annotation practices.
Annotating isn't just about pictures. It includes everything from marking images to noting down speech. Good tools make the work easier and help avoid mistakes. They also make it easier to grow as more companies start using AI by 2025.
Adding the best datasets and getting ongoing feedback make data annotation better. Specific guidelines for different fields also speed up the work. These steps are key to building a trustworthy AI environment. Armed with this, you can make AI work better for your business using the right tools. This lets you transform data into smart actions.
FAQ
What is the role of annotation in machine learning?
Annotations are key in teaching machines through supervised learning. They supply models with precise data to understand patterns and objects. This is vital for tasks such as object detection and understanding text.
How does image annotation affect the performance of AI models?
Image annotation boosts AI model performance. It does this by providing detailed training data. This data helps the model learn better, making it more accurate in real-world uses.
What are the different image annotation techniques, and how are they chosen?
The main techniques are bounding box and polygon annotations. Bounding boxes are quick and suit simple shapes. Polygons work for more complex shapes. The choice depends on the detection task’s demands.
What is the significance of text annotation in NLP?
Text annotation is crucial for NLP as it teaches algorithms human language use. It enables accurate task completion, such as text categorization. This is key for advanced AI-human language interaction.
What are the common challenges faced in data annotation?
Data annotation challenges include precision in bounding boxes and understanding complex sentiments. Also, it’s key to keep a uniform quality across big data sets which requires clear rules and careful quality monitoring.
What are the best practices for data annotation in machine learning?
Maintaining high standards in quality control and attention to detail are best. Ensure the data is consistent and reflects real-world diversity. These practices lead to more accurate AI applications.
How do I choose the right machine learning annotation tool for my project?
To choose the right tool, consider your project's needs and the tool's annotation types and support. Ease of use and integration with other tools are also important. Choose based on your specific requirements.
How can automation streamline the annotation process?
Automation reduces effort and increases the speed and accuracy of the annotation process. It's great for big data sets and minimizes human error. Machine learning tools that support auto-labeling are particularly helpful.
In supervised learning, when should I use polygon annotation over bounding box annotation?
Choose polygon annotation when the shapes are complex or need precise detail. It's best for tasks like medical image analysis. For simpler shapes, bounding box annotation suffices.
What are the essential text annotation guidelines to ensure high model accuracy?
Defining clear rules for sentiment, intent, and entity labeling is crucial. These rules should address various context to reduce errors. Ensure the data is diverse to improve the model's predictive strength.
How have advancements in data labeling tools improved machine learning?
Advancements in labeling tools such as Keylabs enhance machine learning by making annotation efficient and supporting various annotation types. These tools streamline the process and improve team collaboration. They also optimize dataset management and model performance.
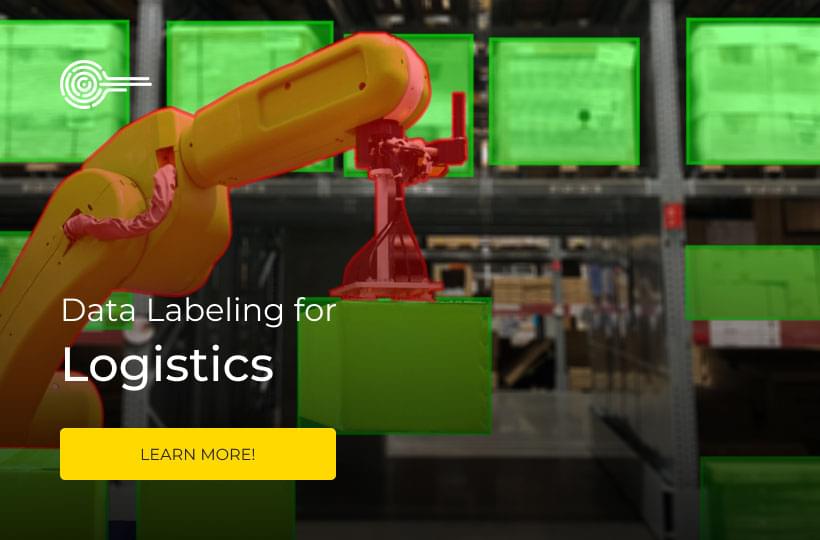