The Problems of Bias and How Image Annotation Services Can Help
The problems of Bias in the industry are complex. The first definition is a human problem. The second definition of bias is a technical problem of data science. Both can affect the decision-making process. One affects humans, and the other affects machines. This article will focus only on the second definition and the technical challenges of bias in data used to develop AI.
Bias in an algorithm or AI can negatively affect how the AI makes decisions, very like how bias can negatively affect how humans make decisions. It is just that in the case of the algorithm, it is a code bug that can be patched. It is a glitch that can be fixed. Most commonly, more inclusive data is needed. That is something that the right image annotation service can help you with.
The technical problems of bias in AI are just a set of technical problems with technical solutions. It can be solved by programmers, engineers, hackers, and data scientists. For example, you could use the right image annotation outsourcing service.
The largest technical problem of bias comes from not having inclusive and comprehensive data. Instead of trying to exclude data add more inclusive data.
When adding a lot of useful data, some erroneous data usually still gets mixed into the dataset. That erroneous data is called noise. That noise can create issues like the bias that can negatively impact the accuracy of an AI's decisions.
The Problems of Bias
- Bias negatively impacts the ability to make decisions whether it is a human or machine.
- Bias in AI comes from the dataset being too small.
- Using the wrong data annotation tools and techniques can sometimes also cause bias.
- Bias decreases the accuracy of AI.
- Overcoming bias in your algorithms takes a lot more inclusive data that may also contain more noise.
The Solutions to the Problems of Bias
The main solutions to the problems of bias in AI are common in data science. The solutions are always pretty similar, more data and the right data annotation is the common best answer.
The technical problems of bias in AI can be solved by programmers and engineers, and hackers. For example, you could use the right image annotation outsourcing service. The largest technical problem of bias comes from not having inclusive and comprehensive data. Instead of trying to exclude data add more inclusive data.
Pretty much all of the problems of bias in AI can be overcome with more data and the right image annotation techniques. For example, to create a medical AI to help doctors diagnose diseases and solve a problem of bias in that, AI takes two things. The first thing that is needed is a very large, inclusive dataset. The second requirement is specialized, expert medical image annotation.
As it happens, we can help you with both of those things. We can provide the data and the data annotation.
Another classic problem that we have solutions to is the problem of bias in facial recognition algorithms. Like the kind used in the security industry. The fix is to add a lot more pictures of people's faces. You need to include people of all ages and demographics and include people who wear makeup, glasses, masks that cover the lower half of the face, and so on.
A common futuristic goal is to create a self-driving autonomous automobile. Something like the classic KIT from the 1980's series Knight Rider. If you were to try to emulate the fictional Knight Industries, you would have to overcome the problems of bias to create a safe self-driving car.
To do that would take a great variety of data, starting with the rules of the road for your target region or country. It would need the ability to recognize common road signs, traffic signals, other vehicles, and all potential obstacles. Having an issue of bias in such a self-driving car could be disastrous.
So, to avoid the problem of bias in your own version of KIT causing an accident would take both a very large amount of images and videos in your dataset. It would take several image annotation projects. That way, every vehicle, and common obstacle could be properly labeled so that the AI could recognize it.
It would also need to take into account the unpredictability of humans, both human drivers and pedestrians, in order to be safe. A common bias of drivers, whether human or AI, is that other vehicles will follow all of the rules of the road and so behave predictably. That’s one reason that object tracking in video data is so important.
It is also sometimes necessary to include humans in some decision-making processes. Humans and machines can work together and provide each other with checks and balances. That also helps eliminate bias.
Regardless of the kind of AI that you are developing, the problem of bias can be solved. You just need an inclusive dataset and the right image annotation solution.
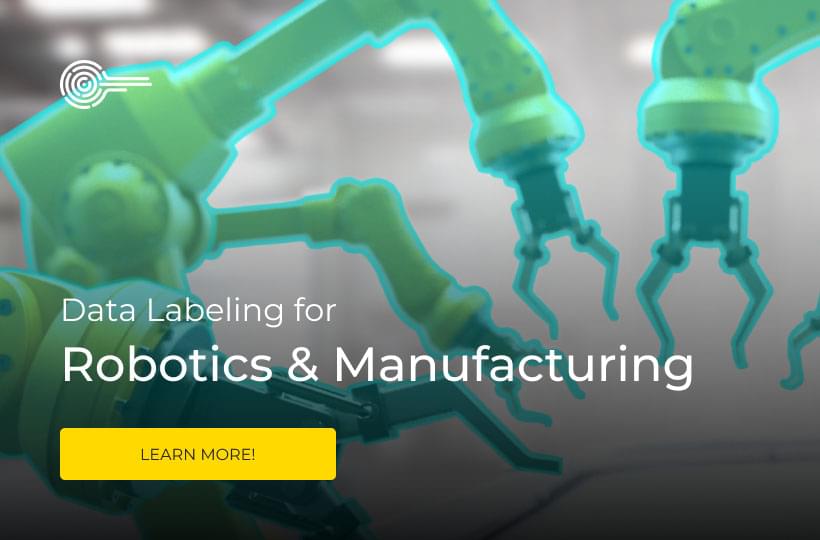