The Human Touch in AI: The Importance of Data Annotation in Finance
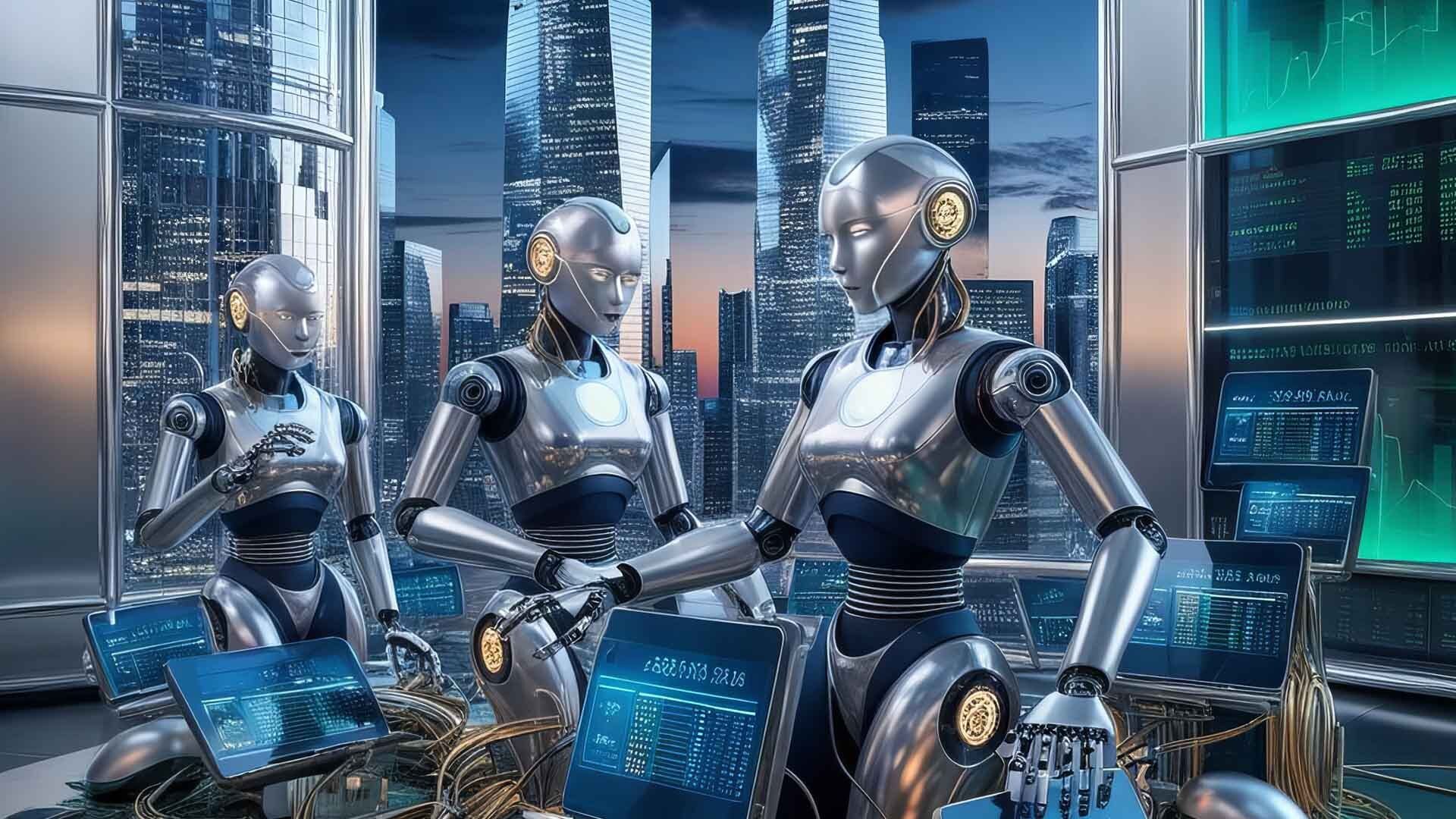
Data annotation for AI in finance is a key challenge for unlocking value of ML models. Annotated data is essential for building AI models that are both accurate and fair for finance tasks. It allows computers to understand data like financial reports or market trends. Adding human insights to data makes it more precise and helps to train AI and machine learning models better.
Data annotation makes AI tools in finance much better. This means they can understand financial data more accurately and make better decisions. Different ways of annotating data include doing it in-house which monitors quality but can be expensive, having others do it for you which gives access to skills but may lower quality, using the public to help which is cheap but harder to control the quality, and relying on AI for annotation which is fast but needs setting up.
Using people to help label your financial data improves your AI. Your AI will then make more precise predictions and help reduce financial risks. Making data annotation a key part of your AI plan will help you be a leader in AI's use in finance.
Key Takeaways
- Data annotation is crucial for developing representative, successful, and unbiased AI models in finance.
- Human-annotated data provides a layer of cognitive understanding and interpretation that automated systems may lack.
- Data annotation significantly enhances the capabilities and practicality of AI-powered solutions in finance.
- Main data annotation techniques in finance include in-house, outsourced, crowdsourced, and AI-driven annotation.
- Embracing data annotation as a core component of your AI strategy will position you at the forefront of AI in finance.

Understanding the Role of Human-Annotated Data in AI
In the world of artificial intelligence, human-annotated data matters a lot. It is vital for teaching AI models in many areas. This carefully labeled data is crucial for creating advanced algorithms. These algorithms are used in everything from healthcare to self-driving cars.
Definition of Human-Annotated Data
Human-annotated data is reviewed, marked, or sorted by people. Experts put in their knowledge of the subject to make sure the labels are right. They understand the details and context better than machines. This makes the data more meaningful.
Comparison with Machine-Generated Annotations
Machine-made annotations are fast and can handle lots of data. But they often miss the deeper meaning or cultural hints. Humans are better at understanding these subtleties. For example, they can tell different meanings of a word depending on how it’s used.
Industry | Application of Human-Annotated Data |
---|---|
Healthcare | Assists in developing diagnostic tools and personalized medicine by accurately labeling medical images and patient data |
Finance | Utilized for fraud detection and risk assessment, training models to identify unusual patterns or anomalies in transaction data |
Autonomous Vehicles | Heavily relies on human-annotated data for training models to navigate complex traffic scenarios and pedestrian interactions safely |
The work human annotators do is crucial for AI to learn well. They provide rich, detailed datasets that machines learn from. As AI technology grows, human expertise in labeling data will keep being important. This ensures AI systems are reliable and fair.
The Critical Role of Human Intuition and Understanding in Finance AI
In finance AI, human intuition and understanding matter a lot. Humans add their unique viewpoint when annotating data. They help AI understand complex financial matters. Humans' input is vital for tasks like spotting fraud and assessing risks. Machines learn to act more like us this way.
Even though machines can process lots of data quickly, they don't always get metaphors or hidden meanings. Humans are great at this, giving richer insights with their annotations. Also, human annotators are key because they can keep up with the changing world of finance data. They quickly update how they analyze new information. This keeps finance AI relevant and on target.
Human intuition and understanding are central to finance AI's data annotation. They help AI "get" complex financial stories in ways that match human thought and action.
Human knowledge is vital for making finance AI better over time. By combining what people and machines do best, AI models can become more accurate and trustworthy.
Aspect | Human Annotators | Automated Systems |
---|---|---|
Contextual Understanding | Excel at interpreting nuances and complexities | Can struggle with sarcasm and cultural subtleties |
Adaptability | Can quickly adjust to new and evolving financial data | May require significant retraining for new data types |
Iterative Improvements | Provide valuable insights to guide model refinement | Rely on human expertise for iterative enhancements |
By using both people and machines together, finance AI gets smarter in a way that feels right to us.
Key Applications of Human-Annotated Data in Finance
1. Fraud Detection and Risk Assessment
Fighting fraud is a big deal thanks to human-annotated data. AI learns to spot odd patterns. This keeps everyone's money safer.
People who add notes to data are crucial. With their help, AI gets smarter at catching fraud. The more they teach AI, the better it gets.
2. Personalized Financial Services
Humans are making AI services feel personal. They mark data about us in useful ways. So, AI can suggest or do things just for us.
This makes us happier and ties us closer to finance services. People who mark the data make sure AI really gets us. They catch all the little details that make all the difference for customers.
3. Algorithmic Trading and Investment Strategies
In stock trading, smart data labeling is key. It teaches AI to find great chances. And to handle quick changes in the market well.
With human input, AI sees the market clearer. It sorts through lots of data to find the best moves. This helps financial groups do better and win more.
Application | Key Benefits |
---|---|
Fraud Detection and Risk Assessment |
|
Personalized Financial Services |
|
Algorithmic Trading and Investment Strategies |
|
Analyzing data at a deep level changes finance. It pushes AI and services forward. It's a game-changer for the industry.
Ensuring High-Quality and Consistent Financial Data Annotation
Keeping data annotation at a high level is key for accurate AI models in finance. Issues with annotations can come from different places. These include different people seeing things differently, varying levels of expertise, and mistakes.
To make sure data labels are always right, we need strong training, clear rules, and regular checks.
Training our annotators well is the first step to top-notch data annotation. By teaching them about financial data in detail, we help them make fewer mistakes.
They all understand and follow the same steps. This means the data they create is more dependable for AI teaching.
Robust Training and Clear Annotation Guidelines
Creating detailed guidelines for annotation is crucial. These rules should touch on everything in the marking process. This includes:
- Determining the financial terms and concepts to note
- Giving examples of right and wrong annotations
- Explaining how to structure the data
- Determining how to find and report mistakes
Having these clear guidelines helps annotators stay consistent and accurate. Updating the guidelines with time makes sure the annotations stay high-quality.
Management should frequently check and review the work to avoid issues. These checks should happen at different times, including before starting to train the AI.
- Looking at some examples of annotated data for accuracy
- Comparing what different annotators did to find differences
- Scanning for any obvious formatting or structure problems
- Inspecting all annotations thoroughly before use
Fixing any problems early helps create a trusted final dataset. Doing regular checks also keeps the data good over time, no matter how the needs change.
Quality Assurance Measure | Benefit |
---|---|
Robust annotator training | Guidelines are more likely to be used correctly and consistently |
Clear annotation guidelines | Ensure correct and consistent annotation |
Regular quality checks | Helps catch and fix mistakes early |
Comprehensive dataset audits | Makes sure the data's trustworthy and accurate |
By following these quality steps, we make sure our human-in-the-loop learning method is strong. This leads to AI making better choices and outcomes in finance.
Addressing Subjectivity and Biases in Financial Data Annotations
Data labeling for financial data faces a big challenge. It's because people's personal views can sneak into the data. Even with the best intentions, human annotators might add their own biases. This could lead to bias in the data. And that, in turn, could make AI financial models give inaccurate results.
To deal with these risks, it's important to spot and handle subjectivity and biases in financial data annotations. A mix of strategies helps:
- Diverse Annotator Teams: Gathering a variety of annotators with different backgrounds can balance biases. They can bring a wider knowledge to the data.
- Continuous Training: Teaching annotators regular bias recognition and handling techniques makes them more self-aware. This decreases their biases' impact on the data.
- Regular Review and Correction: Creating a process for ongoing data review and correction can fix any hidden biases. This ensures the data stays balanced and fair.
Tackling subjectivity and biases in financial data annotations helps build more accurate AI models for finance. This approach can foster fair and precise results in various finance areas, like:
Financial Application | Impact of Addressing Subjectivity and Biases |
---|---|
Fraud Detection | Reduces false positives and ensures that all customers are treated fairly, regardless of their background or characteristics. |
Credit Risk Assessment | Ensures that credit decisions are based on objective financial data, rather than subjective biases. |
Personalized Financial Services | Provides tailored recommendations and solutions that are truly relevant to each individual customer, without any bias. |
"Addressing subjectivity and biases in financial data annotations is not just a technical necessity, but an ethical imperative. By creating fair and unbiased AI training data, we can ensure that our financial AI models are not only accurate but also just and equitable for all."
Identifying and reducing subjectivity and biases in financial data annotations is key for trustworthy AI in finance. Focusing on this during annotation can lead to AI models that add value while meeting high ethical standards in the finance sector.
Managing the Cost and Time Implications of Human Annotation in Finance
Using people to label data is key for creating AI models in finance. But, marking data can take a lot of time and money, especially with tough financial data. It's important for companies to think about how much marking the data will cost and how long it will take. This ensures they pick the best way that works well and doesn't cost too much.
In finance AI, getting the right mix of cost and quality is tough. Having high-quality annotations is crucial for teaching AI good skills, but they can be expensive. Cheaper options might mean lower data quality and worse AI performance. Balancing quality and cost is critical to success.
Balancing Outsourcing and In-House Annotation
Options for handling data annotation costs and time include outsourcing. Using outside experts can save you money and let your team do what they're best at. They provide accurate and quality work.
But, outsourcing can bring up issues about who sees the data and how accurate it is. Doing it in-house means you have more control and know the data is secure. Yet, you might have to spend more on training your staff. Some companies mix both by outsourcing some tasks and keeping others in-house.
Trained annotation teams, such as those at Keymakr, can often deliver higher quality data in shorter time due to domain expertise and scalable enterprise tools.
Leveraging Semi-Automated Annotation Tools
Using tools that mix human and AI power can make data labeling more efficient. These tools help prepare data for labeling by people, cutting down the time and effort needed. They're a great help.
Take text annotation for finance as an example. Tools can mark parts like company names or numbers. Then, people check and make them accurate. This way, you save time and get more consistent work.
Annotation Method | Cost | Time | Quality Control |
---|---|---|---|
In-House | High | Moderate | High |
Outsourced | Moderate | Fast | Moderate |
Crowdsourced | Low | Fast | Low |
AI-Driven | High (Initial Investment) | Very Fast | Moderate |
In the end, the best annotation method will be different for each finance group. By thinking about cost, time, and quality, and using a mix of outsourcing and in-house work with special tools, you can label data in the best way for your needs. This brings the best value and performance from your data work.
Navigating Ethical and Privacy Concerns in Financial Data Annotation
Starting to use human-annotated data for AI in finance means facing many ethical and privacy issues. This data often includes personal details, so keeping it safe is key. We must make sure the process always respects people's privacy and security.
There are strict laws to follow when using financial data, including the GDPR and CCPA. It's important to have clear rules on how data should be handled, use strong methods to keep data private, and plan safe ways to work with data. This is crucial for keeping customers’ trust in the finance world.
Ethical issues include more than privacy. They also touch on fairness, transparency, and being accountable. Using biased training data can make AI systems unfair to users, so we must check for and fix these issues. Using as little data as possible and focusing on responsibility in AI creation can control privacy risks. This makes sure AI is used wisely in finance.
"The importance of user consent and control over personalization processes cannot be overstated in AI systems. Transparency and explainability are key to building trust and ensuring the ethical deployment of AI in finance."
To handle these ethics and privacy issues well, use these tips:
- Use tough data encryption and control who gets to see the data to keep user information safe from start to finish.
- Make use of privacy techniques to hide user identities in AI systems trying to predict or help make decisions.
- Always check for bias in AI systems, making sure they treat everyone fairly. Fixing any issues helps AI work better for everyone.
- Always think about what's right from the start in your AI work. This includes always having people check important decisions AI might make.
AI Model Type | Transparency Level | Potential Applications in Finance |
---|---|---|
Black-box AI | Low | Fraud detection, risk assessment |
Explainable AI (XAI) | High | Personalized financial advice, credit scoring |
By taking ethics and privacy seriously in your financial data work, you can earn trust, follow the rules, and fully use AI in finance. It’s all about being responsible while developing AI.
The Synergy of Human Expertise and AI in Finance
The finance industry is changing. Artificial intelligence (AI) is becoming a big part of it. But, AI shines most when working with people who have a deep understanding of finance. Together, AI and humans create better financial solutions. They can adapt to new data quickly and accurately.
Combining Human Intuition with Computational Power
Financial experts are key in perfecting AI for finance. They understand the world of finance in a unique way. They can spot trends, solve tricky problems, and make smart choices based on their gut feelings. Working with AI, these experts ensure the tech fits real financial needs.
AI comes in with its powerful number crunching. It can process huge amounts of data faster than we can. AI finds hidden patterns and predicts outcomes using its complex math. Combined with human insight, AI helps make decisions that are backed by real analysis. This aids in making smarter business choices and spotting new chances for growth.
Adapting to Evolving Financial Data Landscapes
Data in finance is always growing. New data types are added all the time. Working together, humans and AI are needed to keep up. Human experts add context to new data. AI, on the other hand, processes large amounts of this data. They do it faster and better than humans can on their own.
Alternative data like social media activity and satellite images are now being used. Humans are needed to make sense of this data for finance. AI then turns this data into useful information. This combo helps financial groups stay ahead.
Application | Human Expertise | AI Contribution |
---|---|---|
Fraud Detection | Identifying complex fraud patterns | Analyzing vast transaction data |
Risk Assessment | Contextual understanding of risk factors | Quantifying and modeling risk |
Investment Analysis | Market knowledge and intuition | Processing and analyzing financial data |
As finance changes, human expertise and AI working together become even more important. This partnership leads to innovation and keeps businesses competitive. By combining their different strengths, teams can create solutions that face today's financial challenges head-on.
Real-World Examples of Data Annotation for AI in Finance
Financial institutions are using AI more and more for innovation and efficiency. They need high-quality annotated data for their AI projects. The finance industry's top firms use human-annotated data to succeed. This shows how important and beneficial data annotation is for finance.
The Future of Human-Annotated Data in Finance AI
The finance world is turning more to artificial intelligence (AI), making human-annotated data very important. This future will see a big change, focusing more on human involvement together with AI. This mix makes AI models in finance more precise, trustworthy, and easy to understand.
A big trend is the growth of annotation tools specifically for finance. These tools meet the financial world's unique needs, making sure the data fits just right for financial AI. Using people who know finance well, these tools mark the data in ways that help AI find fraud, assess risks, and give financial advice better.
Also, we're seeing more use of teamwork in marking data with new online platforms. These let annotation teams work together from far away, making sure their work matches up. This teamwork makes labeling financial data faster and less costly, thanks to special tools and methods.
Key Trends | Impact on Finance AI |
---|---|
Automation and AI-driven annotation techniques | Reduces manual effort and accelerates data labeling for large financial datasets |
Multi-modal annotation | Enables AI models to process and understand diverse financial data types for enhanced insights |
Quality assurance and bias mitigation | Ensures accuracy, consistency, and fairness in annotated financial datasets, building trust in AI systems |
Privacy-preserving annotation techniques | Addresses data privacy concerns by anonymizing sensitive financial information while complying with regulations |
As financial data gets more complex, human help with marking it is key. Human touch is great for understanding the fine points, which AI often misses. By keeping annotation methods sharp and following new trends, finance companies can use AI even better down the road.
The future of finance AI lies in the synergy of human expertise and machine intelligence, with human-annotated data serving as the bridge between the two.
To do well in this changing scene, companies need solid plans to mark their data right. Investing in good, human-made data will really push AI to bring new ideas, work better, and grow in finance.
Summary
Human-annotated data plays a key role in the success of AI in finance. It's used in everything from spotting fraud and assessing risk to creating personalized services and making trades with algorithms. For AI to reach its full potential, financial firms need to value human skills in labeling data.
They should develop methods to make sure the data annotations are top-notch, fair, and consistent. This way, you can make AI models that are strong and dependable. These models can truly make a difference in the financial world.
Those who use human-annotated data well will lead in finance's future. The tools and methods for labeling data are getting better all the time. With new technology, AI's potential in finance is growing. Keeping up with the latest in data annotation will help your business grow. It'll shape the finance future in the age of AI.
FAQ
What is human-annotated data, and how does it differ from machine-generated annotations?
Human-annotated data comes from people who have checked and labeled the data. They understand the deeper meanings and details in the information. This approach adds a layer of insight that machines might miss.
Why is human-annotated data critical for AI in finance?
In finance, AI models need this data to be accurate. For tasks like spotting fraud or assessing risks, human interpretation is key. It allows the AI to understand finances better.
What are some key applications of human-annotated data in finance?
This type of data is used in fraud detection and risk assessment. It helps AI models find strange patterns in accounts. It's also crucial for personalized finance plans and for making investment decisions with AI.
How can you ensure high-quality and consistent financial data annotation?
To make sure data is labeled accurately, good training and clear guidelines are a must. Regular checks prevent mistakes. This makes the data used to train AI more trustworthy.
What challenges are associated with managing the cost and time implications of human annotation in finance?
Labeling data by hand for finance is slow and expensive. Finding a balance between cost and quality is important. Outsourcing is a common solution. Tools that help people label data faster and cheaper are also used.
How can organizations navigate ethical and privacy concerns in financial data annotation?
Keeping financial data safe while labeling it is vital. Organizations should follow laws like GDPR. They must use methods that keep data anonymous. This is important for customer trust and privacy.
What does the future hold for human-annotated data in finance AI?
The role of human-annotated data in AI finance is getting bigger. In the future, AI and human knowledge will work together more closely. New tools and more complex data will need this combined approach.
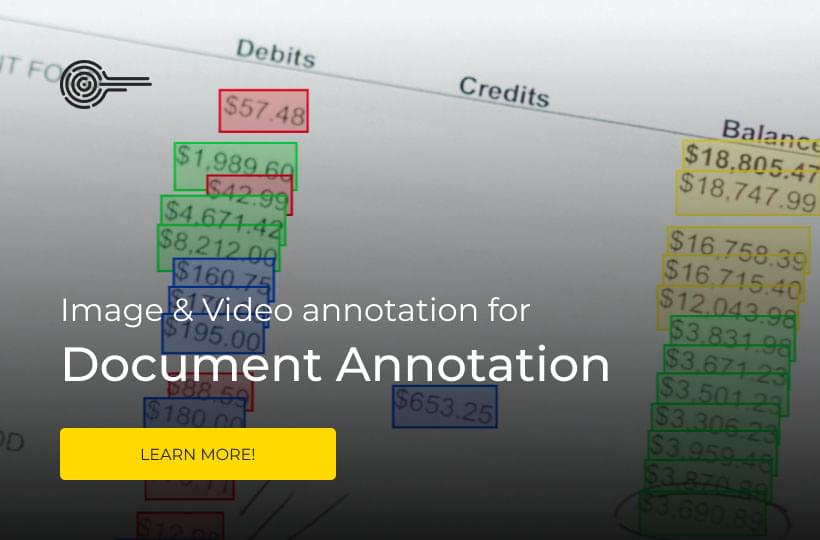