Seeing the Unseen: Data Annotation for AI-powered Defect Detection in Construction
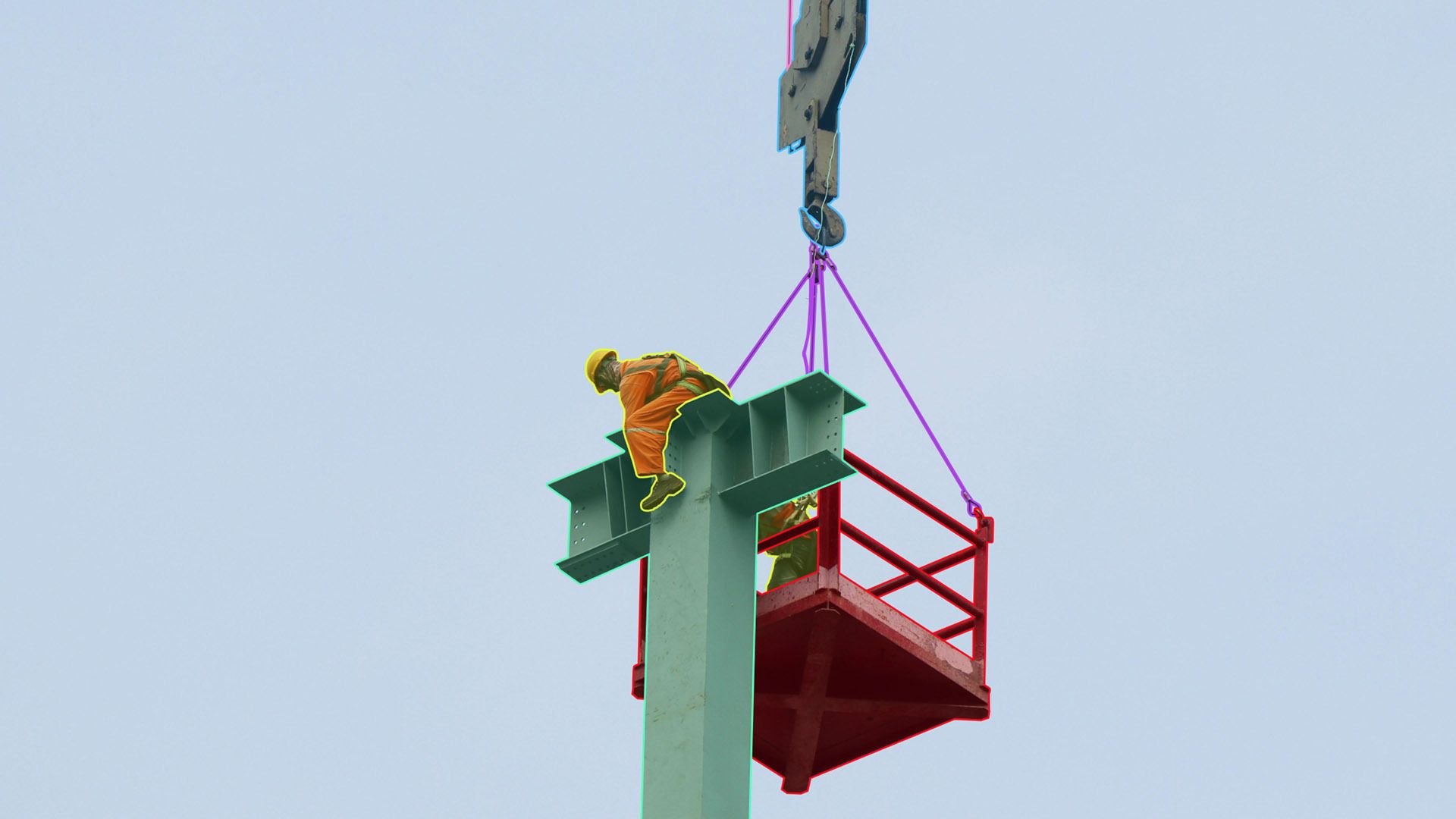
The construction industry is worth over $10 trillion yearly. It is about 13% of the world's economy. Defect detection is a key area, where AI tools are spotting issues by analyzing huge data sets. They look for patterns that suggest problems to be solved. It's all powered by accurate data annotation for AI in construction..
Key Takeaways
- AI-powered defect detection systems are transforming quality control in the construction industry.
- Data annotation, particularly image annotation, is crucial for training accurate AI models.
- Human-in-the-loop learning ensures AI systems continuously learn from domain experts.
- Early defect detection minimizes waste, reduces costs, and upholds quality standards.
- The global AI in construction market is projected to reach $8.6 billion by 2031.

The Role of AI in Revolutionizing Construction Defect Detection
In AI-powered defect detection, data annotation is central. It uses image annotation to teach AI to spot and classify defects. Workers refine AI's knowledge constantly. They make sure it learns from the best, making it smarter over time. As computer vision tech gets better, so will AI in finding defects. This will help builders use data to improve project quality and speed.
AI's early defect spotting saves time and money on construction projects. By analyzing video feeds, it pinpoints flaws before they cause big problems. This boosts a project's quality and safety without a big resource drain.
AI also bolsters construction site safety. It watches sites live, spotting dangers and warning management. For example, it makes sure workers wear proper safety gear and avoid risky work conditions. This cuts down on accidents and makes job sites safer.
AI in construction
Furthermore, AI improves how resources like materials and labor are used. It crunches data from drones and cameras, highlighting better ways to work and cutting waste. This helps projects finish both on schedule and within budget.
AI Application | Impact on Construction |
---|---|
Defect Detection | Early identification of flaws, saving time and resources |
Safety Compliance | Real-time monitoring of PPE usage and hazardous conditions |
Resource Optimization | Improved material management and reduced waste |
Predictive Maintenance | Proactive identification of potential equipment failures |
As the industry adopts more AI, its future looks promising. AI offers ways to avoid common construction headaches like delays and safety risks. This shift promises a brighter, more efficient sector ahead.
Understanding Data Annotation for AI in Construction
Image annotation for defect identification is key in making construction AI usefil. It labels and sorts data like images or readings so AI can tell what's a defect. This makes sure AI systems are accurate and reliable when checking for flaws in construction.
Through data annotation, AI systems study lots of data from sites. This includes images from cameras and data from sensors on things like temperature and vibrations. By marking this data, it's easier to see patterns or differences. Also, it helps with making better budget estimates and finding ways to save money on construction projects.
Types of Data Annotation for Construction AI
In construction, we use different types of data annotation to teach AI well. They include:
- Bounding box annotation: This involves drawing boxes around defects in pictures. It tells the AI exactly where the defect is.
- Semantic segmentation: This labels each pixel in an image so the AI understands how defects are arranged.
- Instance segmentation: It allows the AI to pick out and separate different instances of defects within an image.
These annotation methods help AI find and understand defects in construction. This makes quality control and risk management better and more efficient.
Importance of High-Quality Data Annotation
Getting data annotation right is crucial for construction AI to spot defects accurately. The AI's success relies on the quality of its training data. Wrong or inconsistent labels can make the AI miss defects, leading to problems in construction.
Benefits of High-Quality Data Annotation | Consequences of Poor Data Annotation |
---|---|
Improved accuracy in defect detection | Missed or misidentified defects |
Enhanced reliability of AI models | Inconsistent performance of AI systems |
Reduced false positives and false negatives | Increased costs due to rework or delays |
Better decision-making based on accurate insights | Potential safety risks and compliance issues |
To do data annotation well, experienced annotators are crucial. They should understand construction. Working with a skilled partner and the best tools ensures your AI system has precise data.
By focusing on top-notch data annotation, construction firms can fully benefit from AI in finding defects. This can lead to better projects, increased safety, and lower costs.
Image Annotation Techniques for Defect Identification
Image annotation is key for training AI in detecting defects in construction. It uses techniques like bounding box annotation, semantic segmentation, and instance segmentation. These methods help AI spot and find defects accurately in photos. Using these, we can make defect-finding systems better, changing how we check quality in building projects.
Bounding Box Annotation
In bounding box annotation, we draw rectangular boxes around the objects we're interested in in a picture. For defect spotting, this means outlining issues like cracks, spalling, or corrosion. It helps the AI model learn where different defects are, making it easier to spot these issues in real life. This can lead to faster fixing and upkeep.
Semantic Segmentation
Semantic segmentation goes even further by marking every single part of an image with a label. For defects, it helps AI see how these problems fit into their surroundings. By noting every pixel related to a defect, like for cracks, holes, or delamination, the AI can understand the full shape and position of an issue. This is especially helpful when defects are irregular or mixed up with other materials.
Instance Segmentation
Instance segmentation is a mix of bounding box and semantic segmentation, offering more details on defect identification. It not only marks different parts but also recognizes each unique defect in an image. This detail is crucial for getting exact data about defects. That data is important for choosing what to fix first and how to best use resources in a project.
Annotation Technique | Defect Detection Capability | Use Cases |
---|---|---|
Bounding Box Annotation | Localizes defects within an image | Identifying cracks, spalling, corrosion |
Semantic Segmentation | Assigns class labels to pixels, providing spatial context | Delineating the extent and severity of defects |
Instance Segmentation | Distinguishes individual instances of defects | Counting and measuring defects for prioritization |
The DIGI-TEXX team labeled over 1,000,000 images in construction in just 7 months. They made the process faster, cutting the time for one image from 9 to less than 4 minutes. They also used different techniques for spotting objects, showing how versatile annotations can be in construction.
The construction field is embracing AI and seeing the need for precise data more than ever. With advanced annotation techniques and help from experts like TagX, the sector can fully benefit from AI in finding defects. This could lead to major steps forward in control, safety, and how efficient projects are.
Leveraging Human-in-the-Loop Learning for Construction Safety
Human-in-the-loop learning is changing the game for construction AI, especially in detecting defects. It mixes powerful machine learning with the wisdom of human experts. This makes AI models get better over time, adjusting to the tough issues in construction.
The secret to this learning is top-notch data annotation. AI needs well-labeled data to work properly, and that's where the experts come in. Their deep knowledge helps AI learn better, making more accurate predictions.
Human-in-the-loop learning truly shines in accurately handling tricky situations. AI isn't perfect and can get things wrong. But, people step in to fix those mistakes, making the models more accurate. With human help, the data annotations become richer, improving the understanding of complex tasks.
Benefit | Description |
---|---|
Improved accuracy | HITL enhances accuracy and annotation quality by using human insight. This improves defect detection reliability in construction AI. |
Continuous improvement | HITL helps AI models get better over time by feeding back insights. This ensures the AI keeps up with construction's specific needs. |
Optimization of annotation processes | HITL makes annotation smoother with active learning. This makes the data labeling process faster and more efficient. |
Enhanced quality control | Quality control benefits from HITL by sticking to strict annotation rules. This includes human annotators fixing conflicting data, promoting consistency. |
Human-in-the-loop learning has a major impact on the construction sector. It takes up over 80% of AI/ML project time for tasks like creating sound annotations. Human help is more important than ever. With constant oversight, model accuracy can get a 15% boost. This ensures AI stays reliable in changing conditions.
The demand is growing fast for third-party annotation services, especially in fields like medical testing and self-driving cars. In the future, big teams of skilled professionals using smart tools might replace low-cost annotators for making high-quality annotations.
As the construction field adopts AI defect detection, human-in-the-loop learning will be key for accurate, reliable systems. It combines human skill with AI power. This helps construction pros achieve higher efficiency, safety, and quality in their work.
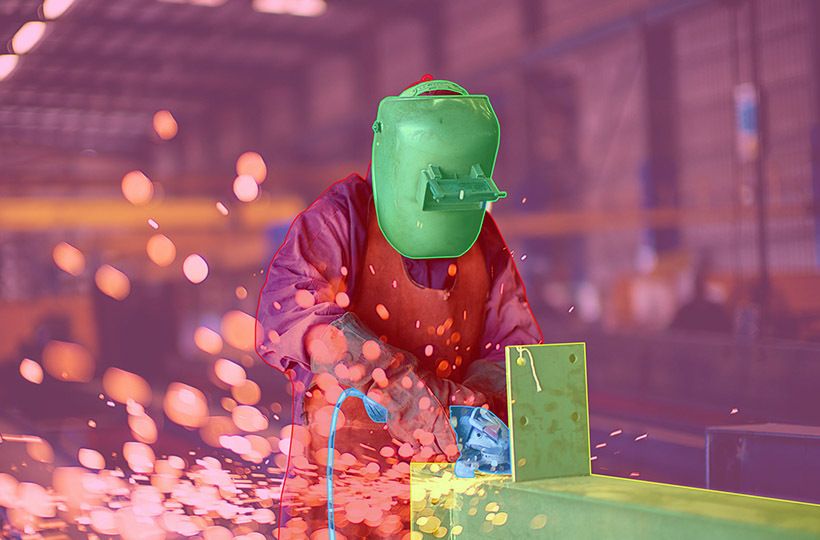
Case Studies: Successful Implementation of Data Annotation for AI in Construction
The construction field has seen big steps forward in spotting defects with AI. This is all thanks to data annotation techniques. By adding notes to images, videos, and sensor data, AI is taught to pick out and assess construction problems. We'll look at some cases that show how this has changed the game in construction.
Bridge Inspection and Maintenance
Checking and fixing bridges is key to keeping everyone safe and the bridges lasting longer. Before, looking at bridges needed lots of time, work, and it could be wrong. But now, thanks to AI learning from tagged data, spot checks are faster and more correct.
A case used drone photos and computer algorithms to find cracks and rust. By marking up thousands of images, engineers help AI see problems and judge how bad they are. This saves time and money. It also helps keep the bridges working well by solving issues before they get worse.
Building Facade Assessment
Checking building facades is important to keep them strong and efficient. Before, this task was slow and might miss things. But by using tagged images and data, AI spots problems like leaks and material wear very well.
In a study, a company detailed building photos to let AI find issues. This helps owners plan repairs smarter. It makes checks quicker and more focused.
Road Surface Monitoring
Keeping roads in good shape is important for drivers and cars. Before, just looking at roads could be off and repairs slow. Now, AI helps catch problems earlier and better.
In one case, a city used cars with cameras to take pictures of the roads. They marked these pictures with tech so AI could find issues like potholes. This made fixing roads better and cheaper, making them safer for all.
Case Study | Data Annotation Technique | Key Benefits |
---|---|---|
Bridge Inspection and Maintenance | Bounding Box Annotation | Efficient defect detection, proactive maintenance, enhanced safety |
Building Facade Assessment | Semantic Segmentation | Accurate defect classification, targeted repairs, improved energy efficiency |
Road Surface Monitoring | Instance Segmentation | Optimized maintenance schedules, cost reduction, enhanced road safety |
These studies show how noting data in construction boosts safety and efficiency. AI, with tagged data, can revolutionize building work. As AI grows, labeling data will be key in the construction world's growth and success.
Challenges and Considerations in Data Annotation for Construction AI
The construction industry is using AI for tasks like spotting defects and improving quality. Getting the data right is key. But, there are hurdles to clear. We need effective ways to label data to make AI work well in construction.
Data Quality and Consistency
Getting data right is a big challenge in construction AI. If data is poor or labeled inconsistently, it confuses the AI. We must make sure the data we use shows real-life situations.
This means collecting and checking data carefully. Training annotators, or data labelers, well is also crucial. They need to follow clear guidelines and do quality checks.
Domain Expertise and Collaboration
Working with experts in construction matters a lot in data labeling. If the annotators don't know much about construction, they might get it wrong. This can make our AI miss important details.
Having construction pros help in the labeling ensures we catch all the fine details. It's also key to keep talking and giving feedback between the labeling teams and the experts. This makes sure our data is top-notch.
Scaling Annotation Processes
As projects get bigger, we need more data to train our AI. And we need it fast. Slow labeling can mess up project timing and costs.
We must find ways to label data quicker. This includes using automation and teaming up with annotation experts. Efficient workflows and tools help us get the right data on time.
Challenge | Impact | Mitigation Strategy |
---|---|---|
Data Quality and Consistency | Impaired model performance, inaccurate defect detection | Robust data collection and validation, clear annotation guidelines, quality control measures |
Domain Expertise and Collaboration | Interpretive disconnects, inaccurate annotations | Engage construction professionals, regular communication and feedback loops |
Scaling Annotation Processes | Delayed project timelines, budget overruns, competitive impacts | Efficient workflows, automation tools, partnering with experienced annotation service providers |
Overcoming these challenges and focusing on quality data is crucial in construction AI. By ensuring data is well-labeled, involving experts, and setting up effective processes, the industry can fully benefit from AI. This will lead to better project results.
Best Practices for Effective Data Annotation in Construction
To succeed in AI-powered defect detection in construction, following best practices is key. These steps ensure the data annotations are of high quality and dependable.
Different AI projects need different kinds of annotations. For projects that use images, like spotting cracks in concrete, you might use bounding boxes or key points. But for those that deal with text, such as reading reports, you might need to recognize entities or analyze feelings. Knowing which annotations to use is vital.
Using automated tools for annotation can also be a great help. They can speed things up by suggesting annotations thanks to pre-trained models. Combining automated tools with human workers can make your work faster while staying precise. This method works well with big tasks, like sorting through a lot of images from job sites.
When you need to process a large amount of data, crowdsourcing can be very beneficial. Tools like Keylabs let you label data quickly and efficiently. This is a smart way to handle diverse datasets full of images of different areas in construction, like waste or safety risks.
An active learning strategy can make your models get better over time. It focuses on marking the challenging parts of data to train the model further. This method efficiently uses annotation resources and can enhance how well the AI detects defects.
Consistency keeps the model training effective. With clear guidelines and quality checks, you can correct any mistakes in the data, which betters the overall quality of your annotations.
Creating a loop where those who label data can talk with those developing the model is a must-do. This teamwork helps refine the model and the guidelines for marking data, improving accuracy over time. Keeping communication open and working together is essential for the model to get better.
To make sure your data is right, quality checks and feedback for those who annotate are very important. These steps are part of ensuring the AI is taught with the best data. Correcting any mistakes early keeps your project on the right track.
When hiring outside help for data annotation, security and privacy are a top concern. Make sure there are rules in place, like non-disclosure agreements. Secure ways to share data keep your system for detecting defects safe and trusted.
Best Practice | Benefit |
---|---|
Tailored annotation types | Ensures annotations align with specific project requirements |
Automated annotation tools | Enhances efficiency without compromising accuracy |
Crowdsourcing for scale | Provides cost-effective and swift annotation of large datasets |
Active learning strategy | Optimizes annotation resources and improves model performance |
Consistency in annotation | Contributes to improved overall quality of annotated data |
Active feedback loops | Ensures continual improvement in model accuracy |
Quality assurance measures | Contributes to the reliability of annotated data and project success |
Security and privacy protocols | Protects sensitive information and maintains data integrity |
By sticking to these best practices, those in construction can make the most of AI in finding defects. This leads to safer, more efficient, and better construction projects.
The Future of AI-powered Defect Detection in Construction
The construction world is moving fast towards digitization and new tech. The future for AI in finding defects looks bright. The global AI in construction market is set to grow to $8.6 billion by 2031. From 2022 to 2031, it's expected to grow at a rate of 34.1%.
As technology advances, we can look forward to better, faster, and more detailed defect spotting.
Advancements in Computer Vision and Deep Learning
Computer vision and deep learning are making big strides. Through these, AI can scan massive amounts of data. This includes images, readings, and different details to find defects. As these technologies get better, so will our ability to spot a wider range of issues accurately. This helps construction pros pinpoint problems early, leading to better decision making and use of resources.
Integration with Drone Technology and IoT Sensors
The link between AI, drones, and IoT sensors is very exciting. Drones with cameras and sensors can go where it's hard for us to, like high-up buildings. This lets them gather lots of data and images. AI systems can then check this data quickly to find potential problems early. This can lead to maintenance rather than costly repairs later on.
IoT sensors also play a key role. They keep an eye on things like structures, the environment, and how well equipment is working. Their data helps AI systems understand how healthy and safe a project is. This means construction pros can do smarter, safer work.
Industry | Applications of AI-powered Defect Detection |
---|---|
Manufacturing | Quality control, process optimization, predictive maintenance |
Automotive | Identifying defects in parts, ensuring assembly quality |
Electronics | Detecting faults in PCBs, ensuring component quality |
Pharmaceuticals | Identifying contaminants, ensuring product safety and efficacy |
Food Processing | Detecting foreign objects, ensuring food safety and quality |
Aerospace | Identifying structural defects, ensuring aircraft safety |
The construction industry's use of these technologies is set to brighten the future. With tech like computer vision, deep learning, drones, and IoT sensors, we're on a path to safer, more efficient projects. This will push the industry ahead, opening up new chances for progress and innovation.
Empowering Construction Professionals with AI-driven Insights
AI-powered systems in construction do more than spot problems. They provide key insights. These insights help pros make smart, data-driven choices for project success. It's crucial to use these AI tools to stay ahead. The market for AI in construction is growing fast, predicted to hit USD 3.5 Bn by 2027. So, using AI is a big step for any company.
Using AI changes how risks are managed, resources are used, and teams talk. Construction firms using AI save time and cut waste, getting up to 15% more done. Cameras with AI at job sites watch work update, keeping everyone in the loop. They also help fix issues right away.
Now, construction companies face a big choice: move forward with AI or not. AI's predictive models speed things up, cut costs, and boost results. To make projects better, safer, and more efficient, AI is the way to go. It's all about using AI to stand out in a tough market, for now and the future. Need data labeling, creation, or annotation for an AI projecti n construction? Start here!
FAQ
What are the advantages of AI-powered defect detection systems in construction?
AI-powered systems find defects early, maintain quality, and cut costs. They also make sure projects meet rules and improve efficiency. These systems help monitor work as it happens and improve how projects are done over time.
Why is data annotation crucial for AI in construction?
Data annotation is vital for training AI in construction. It labels and sorts data, teaching AI what a defect looks like. Good data labeling is key for accurate defect spotting. It makes AI more reliable.
What are the different types of image annotation techniques used for defect identification?
Techniques for defect finding in images include drawing boxes around issues (bounding box). There's also giving each pixel a label (semantic segmentation). Finally, there's wrapping defects with a box and labeling their parts (instance segmentation).
How does human-in-the-loop learning improve defect detection accuracy in construction?
Human-in-the-loop learning boosts defect detection accuracy by mixing human insight with AI. Experts aid in data annotation, sharing their knowledge. This method helps AI get better, tackling construction industry needs.
What are some successful case studies of data annotation for AI in construction?
AI success stories in construction are many. For example, it helps in bridge inspections, spotting cracks and corrosion. In building inspections, it finds moisture issues and worn-out materials. In monitoring roads, it locates potholes and cracks.
What are the challenges and considerations in implementing data annotation for AI in construction?
Implementing data annotation for AI faces a few hurdles. It needs to ensure data quality and teamwork between experts and annotators. It must also handle big data. Overcoming these requires good data management, efficient processes, and some automation.
What are the best practices for effective data annotation in construction?
For good data annotation, set clear guidelines and quality checks. Always involve experts to review annotations. Use the right tools to make annotation smooth. Also, regularly check and adjust AI models with real feedback.
How will advancements in computer vision and deep learning shape the future of AI-powered defect detection in construction?
Getting better in computer vision and deep learning will sharpen AI's defect tracking. Drones and IoT sensors will gather data from far-off and risky spots. These advancements will make defect detection systems more powerful.
How can AI-driven insights empower construction professionals?
AI insights deepen the understanding of defects and their causes. They spot trends and offer strategies to prevent issues. They help manage risks better, allocate resources wisely, and improve communication on projects. This makes projects safer, more quality-focused, and efficient.
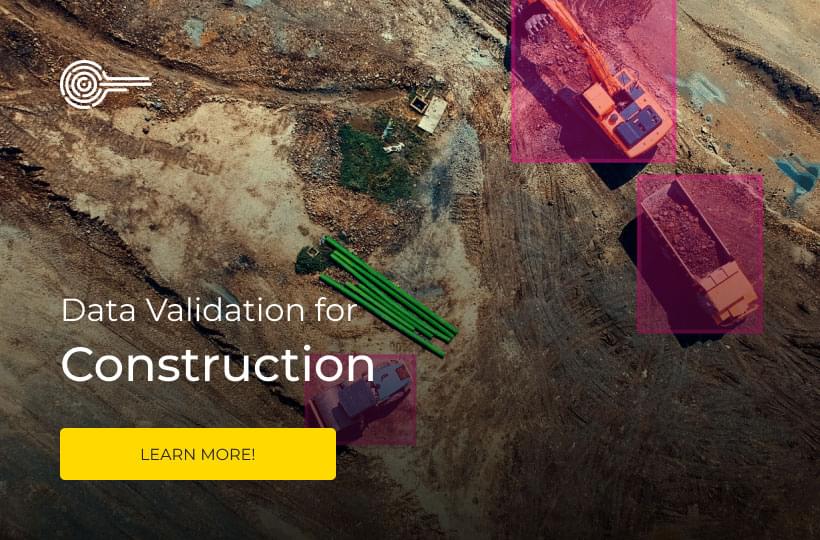