Revolutionizing Medical Imaging with Semantic Segmentation
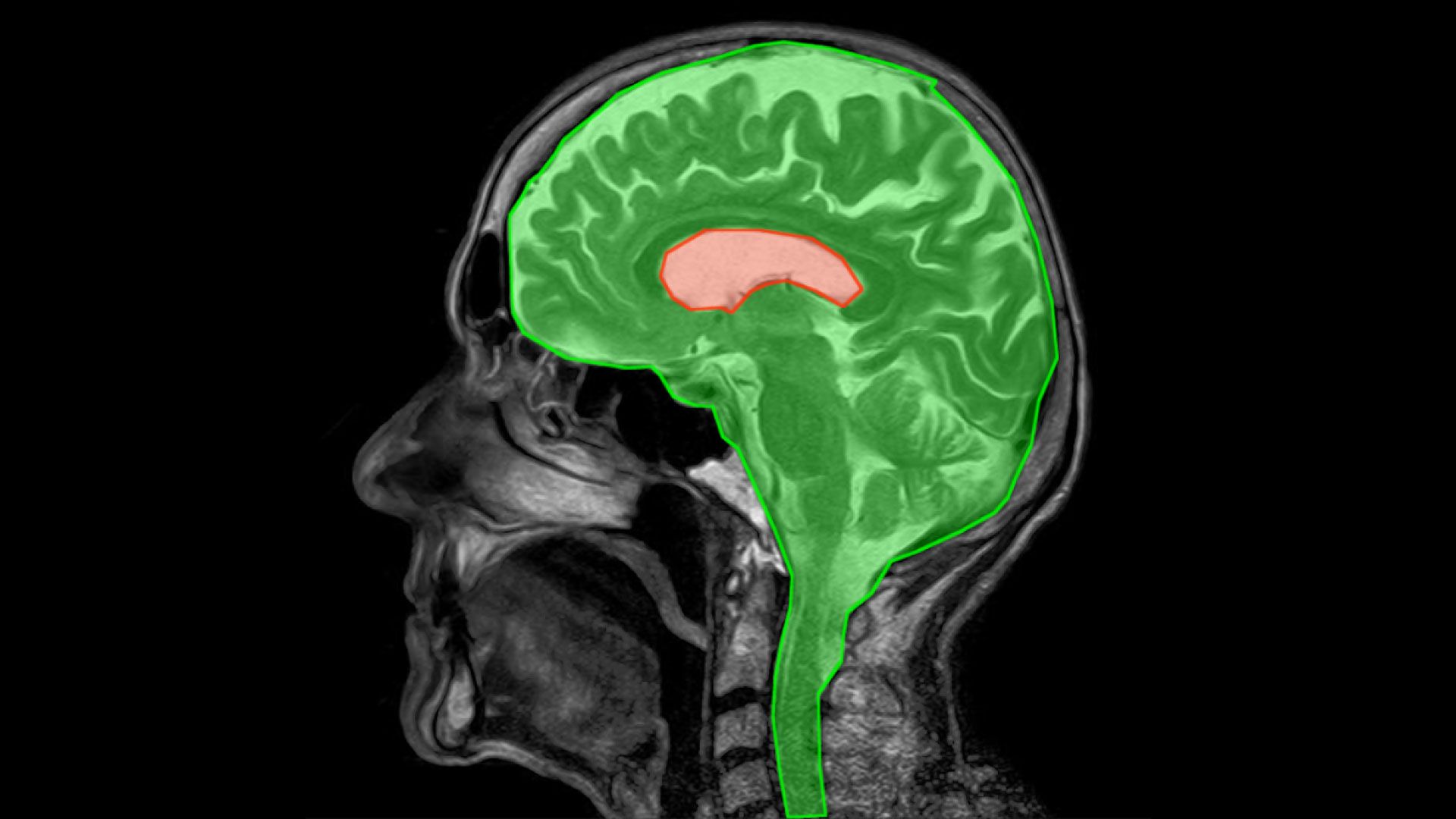
Medical image analysis has witnessed a remarkable transformation with the integration of semantic segmentation, a cutting-edge technique that enables the extraction of relevant information from complex medical images. By leveraging deep learning and computer vision, semantic segmentation is revolutionizing the field of medical imaging, offering unprecedented accuracy and efficiency in diagnoses and treatment planning across various medical domains.

The use of deep learning algorithms, particularly Convolutional Neural Networks (CNNs), has paved the way for remarkable advancements in medical image analysis. CNNs excel at identifying intricate patterns and features within medical images, enabling accurate segmentation with minimal human intervention. However, challenges such as image quality variations, diverse anatomical structures, and noise still exist, necessitating the development of advanced segmentation techniques to overcome these obstacles.
From detecting anatomical structures to identifying pathological abnormalities, medical image segmentation plays a crucial role in providing comprehensive insights for healthcare professionals. It allows for efficient partitioning of medical images into regions of interest, facilitating precise analysis and quantitative measurements.
Key Takeaways:
- Semantic segmentation revolutionizes medical image analysis by extracting relevant information from complex medical images.
- Deep learning algorithms, especially CNNs, offer unparalleled accuracy, efficiency, and automation in medical image segmentation.
- Medical image segmentation enables the identification and analysis of specific anatomical structures and pathological abnormalities.
- Challenges such as image quality variations and diverse anatomical structures require advanced segmentation techniques for reliable results.
- Accurate segmentation plays a pivotal role in precise diagnoses, treatment planning, and monitoring of diseases in various medical domains.
Understanding Medical Image Segmentation
Medical image segmentation is a fundamental process in medical imaging that involves partitioning a medical image into distinct regions of interest. This technique enables clinicians and researchers to identify and analyze specific anatomical structures or pathological abnormalities with precision.
The purpose of medical image segmentation is twofold: isolation and identification. It isolates specific regions of interest within a medical image, facilitating the extraction of relevant information and enabling quantitative analysis of anatomical structures or pathological lesions.
There are three main types of medical image segmentation techniques:
- Manual segmentation: This technique involves trained experts meticulously delineating regions of interest. It requires human intervention and expertise but provides precise results.
- Semi-automatic segmentation: This technique involves initial automated processes followed by manual refinement. It combines the advantages of automation and human expertise.
- Automatic segmentation: This technique relies on advanced algorithms that automatically identify and delineate regions of interest without human intervention. It offers efficiency and scalability.
Medical image segmentation plays a crucial role in various healthcare applications, including:
- Diagnosing and monitoring diseases
- Planning surgical interventions
- Assessing treatment response
- Quantitative analysis of anatomical structures
- Creating anatomical atlases
Accurate and reliable medical image segmentation is essential for precise and meaningful analysis of medical images. It allows healthcare professionals to focus on specific areas of interest, enhancing their ability to detect, diagnose, and treat various conditions effectively.
The Rise of Deep Learning in Medical Image Segmentation
Deep learning algorithms, particularly Convolutional Neural Networks (CNNs), have revolutionized the field of medical image segmentation. With their ability to learn intricate patterns and features within medical images, deep learning methods have surpassed traditional approaches in terms of accuracy and performance. The integration of deep learning in medical imaging began in the early 2010s when CNNs emerged as a powerful architecture for image recognition tasks.
One influential architecture that has played a significant role in the widespread adoption of deep learning in medical image segmentation is the UNet architecture. Introduced in 2015, the UNet architecture has proven to be highly effective in extracting meaningful information from medical images.
Deep learning offers numerous advantages over traditional methods in medical image segmentation. One of the key advantages is the ability to automatically extract and represent features, eliminating the need for manual feature engineering. CNNs, particularly, excel in capturing complex patterns, enabling accurate segmentation of anatomical structures and pathological abnormalities.
To provide a visual representation of the rise of deep learning in medical image segmentation, the following table showcases the performance comparison of deep learning and traditional methods in different image segmentation tasks:
Image Segmentation Task | Deep learning | Traditional Methods |
---|---|---|
Brain Tumor Segmentation | 93% Dice Similarity Coefficient | 87% Dice Similarity Coefficient |
Lung Nodule Segmentation | 92% Intersection over Union | 84% Intersection over Union |
Cell Nuclei Segmentation | 85% F1 Score | 77% F1 Score |
As demonstrated in the table, deep learning consistently outperforms traditional methods, highlighting its superiority in various medical image segmentation tasks.
UNet Architecture and Its Variants
The UNet architecture, introduced in 2015, has revolutionized medical image segmentation. Its unique U-shaped design incorporates a contracting path for feature extraction and an expanding path for precise localization. The contracting path involves applying convolution and downsampling operations, while the expanding path employs upsampling and convolution.
One of the key advantages of the UNet architecture is the utilization of skip connections between corresponding layers in the contracting and expanding paths. These skip connections facilitate the retention of fine-grained details during the upsampling process, improving the accuracy of the segmentation results. By combining information from different resolutions, the UNet architecture enables the network to capture both local and global context, enhancing its ability to accurately delineate structures in medical images.
Furthermore, the UNet architecture allows for the incorporation of additional layers to further enhance its performance. Variants of the UNet, such as the UNet++, UNet 3+, and Attention UNet, introduce modifications to the original architecture to address specific challenges in medical image segmentation.
The UNet architecture has been widely implemented in various medical image segmentation tasks, including the segmentation of organs, tumors, and pathological regions. Its effectiveness in accurately delineating structures and abnormalities has made it a popular choice among researchers and medical professionals.
Key Features of the UNet Architecture:
- U-shaped design with contracting and expanding paths.
- Contracting path for feature extraction.
- Expanding path for precise localization.
- Skip connections for retaining fine-grained details.
- Ability to incorporate additional layers and variants.
Advantages and Applications of Deep Learning in Medical Image Analysis
Deep learning has revolutionized medical image analysis, offering numerous advantages in various applications. With its exceptional capabilities, deep learning has achieved remarkable success in disease diagnosis and classification, providing accurate detection of various conditions in medical images. By leveraging its ability to learn and extract meaningful features from large datasets, deep learning models have proven to be highly effective in identifying and categorizing diseases with a high level of accuracy and precision.
One of the key areas where deep learning excels is image segmentation and localization. Through advanced algorithms and convolutional neural networks (CNNs), deep learning enables precise delineation of structures and abnormalities within medical images. This not only aids in accurate diagnosis but also assists in treatment planning and monitoring of diseases. The ability to localize and segment specific regions of interest within medical images greatly enhances the analysis and understanding of complex anatomical structures and pathological lesions.
Quantitative image analysis is another crucial application of deep learning in medical imaging. Deep learning models facilitate the extraction of meaningful measurements and biomarkers from medical images, enabling quantitative analysis and objective evaluation of diseases. This quantitative approach provides valuable insights into disease progression, response to treatment, and prognosis, leading to more personalized and targeted healthcare interventions.
Deep learning also enables the multimodal fusion and integration of different imaging modalities. By combining data from multiple imaging sources, such as MRI, CT, and PET scans, deep learning models can generate comprehensive insights into complex diseases. This integration of multimodal data enhances the accuracy and robustness of disease diagnosis, helping healthcare professionals make more informed decisions and improving patient outcomes.
Deep learning in medical image analysis disease diagnosis and classification image segmentation and localization quantitative image analysis multimodal fusion and integration
Advantages of Deep Learning in Medical Image Analysis | Applications of Deep Learning in Medical Image Analysis |
---|---|
|
|
Training and Learning from Data in Deep Learning for Medical Imaging
Deep learning models for medical imaging undergo extensive training on large labeled datasets to learn the intricate relationships between input images and their corresponding annotations. The training process involves forward propagation, where the model processes the input images and generates predictions, and backpropagation, where the model's parameters are adjusted based on the computed loss. This iterative process allows the model to improve its performance over time, fine-tuning its ability to accurately analyze medical images.
However, one of the challenges in deep learning for medical imaging is the availability of labeled datasets. Creating and labeling medical image datasets can be a labor-intensive and time-consuming task, especially for rare diseases or specific patient populations. The scarcity of labeled data can limit the ability to train deep learning models effectively, potentially impacting their performance.
To overcome this challenge, transfer learning has emerged as a powerful technique in the field of medical image analysis. Transfer learning leverages pre-existing models that have been trained on large-scale datasets such as ImageNet, which contains millions of labeled images across various classes. These pretrained models have learned rich representations of visual features and can be used as a starting point for training deep learning models in specific medical imaging tasks.
The process of transfer learning involves initializing the weights of a pretrained model and fine-tuning them on the target medical imaging task using a smaller labeled dataset. This allows the model to benefit from the knowledge and features learned from the large-scale dataset, even in the presence of limited labeled data. By leveraging transfer learning, deep learning models can be adapted to specific medical image analysis tasks more efficiently and effectively.
Benefits of Transfer Learning in Medical Imaging
Transfer learning offers several advantages in the context of medical imaging:
- Improved convergence: With pretrained models as a starting point, deep learning models can converge faster during training, reducing the time and computational resources required.
- Enhanced generalization: Pretrained models have learned generic visual representations, enabling deep learning models to generalize well to new and unseen medical images, even with limited labeled data.
- Reduced overfitting: Transfer learning helps mitigate the risk of overfitting, as the pretrained models have already learned to extract meaningful features from large-scale datasets.
- Efficient feature extraction: By leveraging pretrained models, deep learning models can benefit from complex feature extraction capabilities, saving computational resources.
Transfer learning is a valuable tool in the training and learning process of deep learning models for medical imaging. By utilizing pretrained models and adapting them to specific medical image analysis tasks, researchers and clinicians can overcome the limitations posed by limited labeled data. This enables the development of accurate and effective deep learning models that contribute to advancements in medical imaging and healthcare.
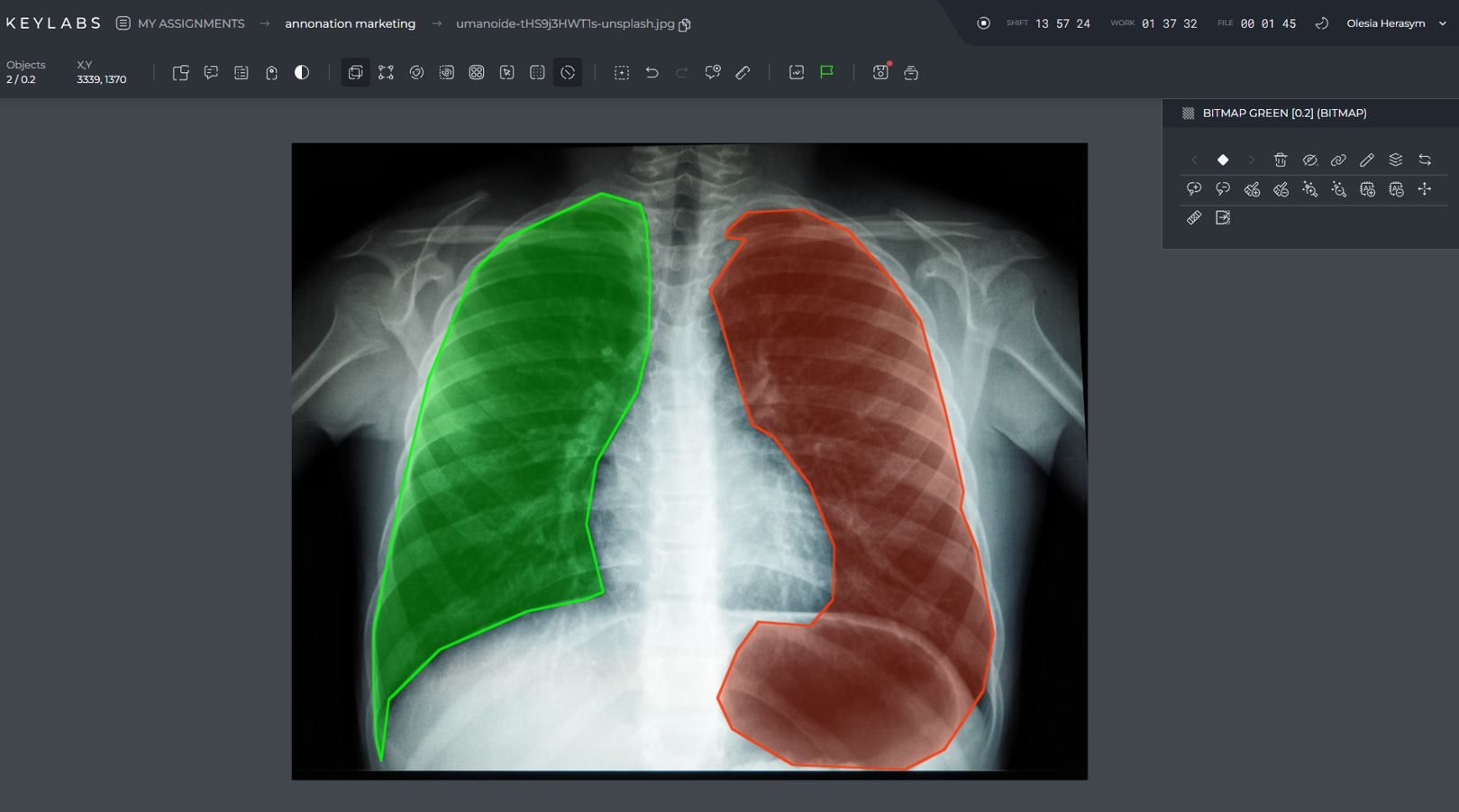
Interpretability and Explainability in Deep Learning for Medical Imaging
Interpreting and explaining the decisions of deep learning models in medical imaging is crucial for building trust and confidence in their application. As deep learning models become increasingly complex, it becomes essential to develop interpretability and explainability techniques that provide insights into the learned features and decision-making processes.
Attention mechanisms are one such technique that helps understand which parts of an image the model focuses on when making predictions. By assigning importance weights to different regions of an image, attention mechanisms can highlight the areas that contribute the most to the model's decision. This allows healthcare professionals to gain a better understanding of how a model arrives at its predictions.
Another technique is the use of saliency maps, which visualize the regions of an image that have the highest influence on the model's output. Saliency maps provide a visual representation of the areas of interest within an image, helping to identify the features that the model considers important for its decision-making process.
Additionally, gradient-based visualization techniques provide insights into how changes in input data affect the model's output. By analyzing the gradients of the model's output with respect to the input image, it is possible to understand which features and regions in the image have the most impact on the final prediction.
By understanding the reasoning behind a deep learning model's predictions, healthcare professionals can make more informed decisions and gain confidence in the accuracy and reliability of the model's output.
Example of attention mechanism:
To illustrate the application of attention mechanisms in medical imaging, consider the task of classifying different types of skin lesions from dermatology images. By using an attention mechanism, the model can focus on the specific areas of the image that contain important features for lesion classification, such as irregular borders or distinct color patterns. This attention mechanism not only improves the model's accuracy but also provides insights into which features are most indicative of different skin conditions.
Example of saliency map:
In the field of radiology, saliency maps can be used to identify the regions within a medical image that contribute the most to a model's diagnosis. For instance, in the case of chest X-rays, a saliency map can highlight the areas of the image that are most relevant for detecting the presence of lung abnormalities, such as nodules or infiltrations. This visual representation can assist radiologists in focusing their attention on the critical regions, potentially improving diagnostic accuracy and efficiency.
Example of gradient-based visualization:
Gradient-based visualization techniques, such as guided gradients or occlusion sensitivity, can provide insights into deep learning models' decision-making processes. For instance, in the domain of brain tumor segmentation, gradient-based visualization can reveal the areas in an MRI scan that have the most impact on the model's prediction. This analysis allows researchers and clinicians to understand which regions of the brain image the model relies on the most for accurate tumor segmentation.
Technique | Description | Use Case |
---|---|---|
Attention Mechanisms | Assign importance weights to different regions of an image to highlight areas that contribute most to the model's decision-making process. | Dermatology image classification |
Saliency Maps | Visualize the regions of an image that have the highest influence on the model's output, providing insights into important features for diagnosis. | Chest X-ray analysis |
Gradient-based Visualization | Examines the impact of changes in input data on the model's output and identifies critical regions for accurate segmentation. | Brain tumor segmentation |
Challenges and Limitations of Deep Learning in Medical Image Analysis
Deep learning has significantly advanced medical image analysis, but it is not without its challenges and limitations. These obstacles must be addressed to ensure the responsible and effective use of deep learning models in healthcare.
Data Scarcity and Quality Issues
Data scarcity and quality are major challenges in medical image analysis. The limited availability of labeled data poses a significant hurdle in training deep learning models. Additionally, there is often a lack of standardization in imaging protocols, leading to variations in image quality and complicating the development and generalizability of models.
Table: Examples of Challenges in Medical Image Analysis
Challenges | Description |
---|---|
Data Scarcity | The availability of labeled data for training deep learning models is limited, specifically for rare diseases or specific patient populations. |
Variability in Imaging Protocols | There is a lack of standardization in imaging protocols, leading to variations in image quality and acquisition techniques. |
Noise and Artifacts | Medical images often contain noise and artifacts, which can hinder accurate analysis and segmentation. |
Interpretability and Explainability Challenges
The interpretability and explainability of deep learning models remain significant challenges in medical image analysis. While these models can achieve high accuracy, understanding the reasoning behind their predictions is often difficult. This lack of transparency limits their applicability in critical healthcare decisions.
"The lack of interpretability and explainability in deep learning models makes it challenging for healthcare professionals to understand why a particular diagnosis or prediction was made."
List: Interpretability and Explainability Challenges
- Difficulty in understanding the factors influencing the model's decision-making process.
- Unexplained biases in the model's predictions.
- Limited insight into the learned features and representations.
Ethical Considerations and Biases
Deep learning models are not immune to biases present in the training data, which can lead to disparities in accuracy and fairness. It is crucial to address these biases and ensure the responsible application of deep learning models in healthcare settings.
Table: Ethical Considerations in Deep Learning for Medical Image Analysis
Ethical Considerations | Description |
---|---|
Data Bias | Inherent biases in the training data can lead to disparities in accuracy and fairness, particularly for underrepresented populations. |
Privacy and Confidentiality | Deep learning models may require access to sensitive patient information, raising concerns about privacy and confidentiality. |
Accountability and Liability | The use of deep learning models in healthcare raises questions of accountability and liability in the event of errors or adverse outcomes. |
Addressing these challenges and limitations is vital to maximize the benefits of deep learning in medical image analysis. By ensuring data quality, improving interpretability, and addressing ethical considerations, deep learning can unlock its full potential in transforming healthcare.
Conclusion
Deep learning has revolutionized medical image analysis, providing a paradigm shift in the field of medical imaging. The application of deep learning techniques, particularly Convolutional Neural Networks (CNNs), has led to unprecedented levels of accuracy, efficiency, and automation in the analysis of medical images. As a result, deep learning has significantly improved the ability to make precise diagnoses, plan treatments, and monitor diseases.
Despite the challenges and limitations faced by deep learning in medical image analysis, ongoing research and development efforts are focused on addressing these issues. Interpretability, data scarcity, and ethical considerations are some of the key areas of focus. Researchers are striving to develop methods for interpreting and explaining the decisions made by deep learning models, as interpretability is crucial for building trust and confidence in their applications.
Looking towards the future, deep learning holds great promise in medical image analysis. The potential applications are vast, ranging from personalized medicine to improved patient outcomes and advancements in healthcare research. With further advancements in interpretability, overcoming data scarcity, and addressing ethical considerations and biases, deep learning will continue to shape the future of medical image analysis, bringing remarkable advancements to the field of healthcare.
FAQ
What is medical image segmentation?
Medical image segmentation is the process of partitioning a medical image into distinct regions of interest, enabling the identification and analysis of specific anatomical structures or pathological abnormalities.
What are the main types of medical image segmentation techniques?
The main types of medical image segmentation techniques are manual segmentation, semi-automatic segmentation, and automatic segmentation. Manual segmentation involves expert delineation, semi-automatic segmentation involves automated processes followed by manual refinement, and automatic segmentation involves advanced algorithms without human intervention.
How has deep learning revolutionized medical image segmentation?
Deep learning, particularly Convolutional Neural Networks (CNNs), has revolutionized medical image segmentation by accurately extracting meaningful information and features from medical images. CNNs outperform traditional methods and enable precise segmentation for accurate diagnoses and treatment planning.
What is the UNet architecture?
The UNet architecture, introduced in 2015, is a distinctive U-shaped design for medical image segmentation. It consists of a contracting path for feature extraction and an expanding path for precise localization. Skip connections between corresponding layers retain fine-grained details, making it widely used in medical image segmentation tasks.
What are the advantages and applications of deep learning in medical image analysis?
Deep learning offers advantages such as accurate disease diagnosis and classification, precise image segmentation and localization, quantitative analysis, and multimodal fusion. It has applications in personalized medicine, improved patient outcomes, and advancements in healthcare research.
How are deep learning models trained in medical imaging?
Deep learning models for medical imaging are trained on large labeled datasets by iteratively updating the model's parameters through forward and backward propagation. Transfer learning is also used to adapt pretrained models to specific medical image analysis tasks.
How can deep learning models in medical imaging be interpreted and explained?
Interpretability and explainability techniques, such as attention mechanisms, saliency maps, and gradient-based visualization, provide insights into the learned features and decision-making processes of deep learning models in medical imaging.
What are the challenges and limitations of deep learning in medical image analysis?
Challenges include data scarcity and quality issues, interpretability and explainability challenges, and ethical considerations regarding biases. Limited availability of labeled data and variability in imaging protocols can hinder model development and generalizability.
How has deep learning revolutionized medical image analysis?
Deep learning has revolutionized medical image analysis by offering unprecedented accuracy, efficiency, and automation. It enables precise diagnoses, treatment planning, and disease monitoring, with potential applications in personalized medicine and advancements in healthcare research.
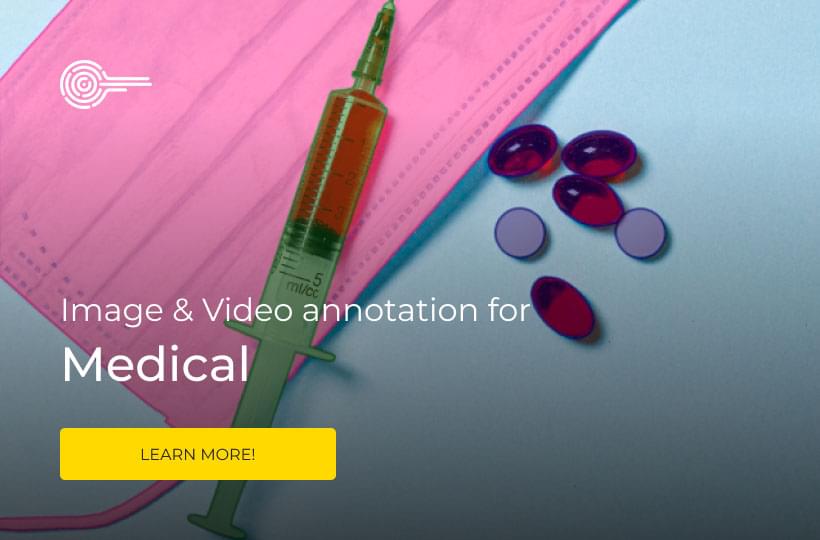