Polygon Annotation for Precision Agriculture
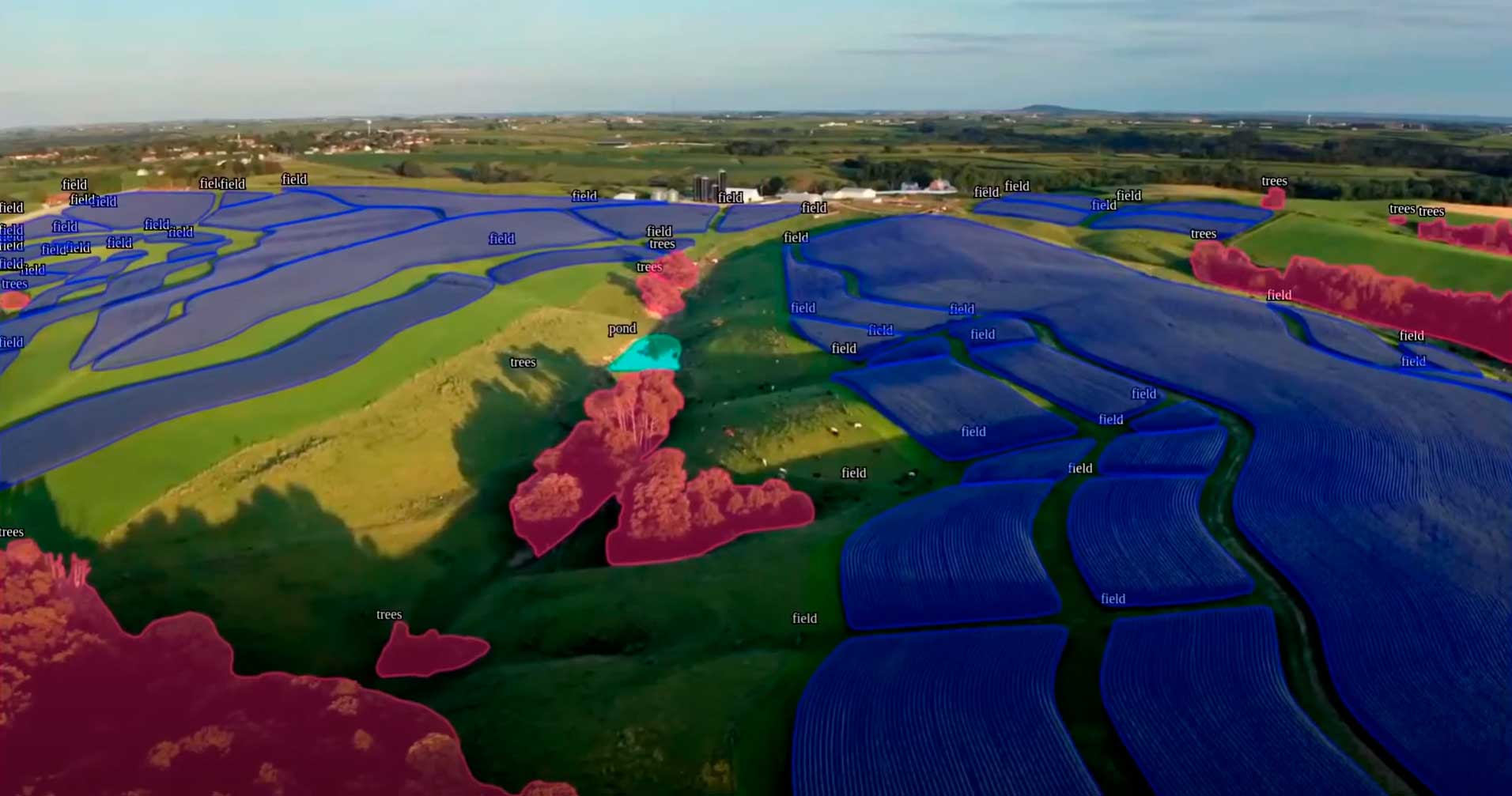
Agriculture is being made more efficient and more profitable by a new generation of computer vision based AI applications. These machine learning driven models can analyse crops and livestock and accurately infer a range of information from what they see. The implications of this technology are significant, precision agriculture will play an important part in securing the food supply as the global climate changes. Image and video annotation are key to creating the training data that allows agricultural AIs to learn.
Polygon annotation is one specific technique that is crucial for the construction of affective datasets. This blog will focus on this annotation method and highlight some of the emerging use cases that it enables. As the agricultural AI sector grows so will demand for this technique. To ensure quality polygon annotation many companies are now reaching out to service providers, like Keymakr, and this blog will identify the key advantages of this kind of outsourcing.
What is polygon annotation?
Polygon annotation is used to capture the outlines of complex or irregular shapes in images and video frames. To achieve this annotators use multiple small lines connected together to chart the edges of a given object. This annotation technique can account for the angles and curves that bounding boxes cannot. In training images and videos for agricultural AI polygon techniques can effectively define fruits, vegetables, and even livestock.
Agricultural AI use cases enabled by polygon annotation
Polygon annotation accurately delineates any shape imaginable. In the agricultural sector polygon annotation is being used to train models with a wide range of exciting capabilities:
- Ripeness monitoring: AI models can determine when fruit is ripe and ready to be harvested. They do this by identifying the colour of the fruit and inferring its level of ripeness from that. Polygon annotation is used to trace the outline of individual fruits, that can then be colour coded according to ripeness levels.
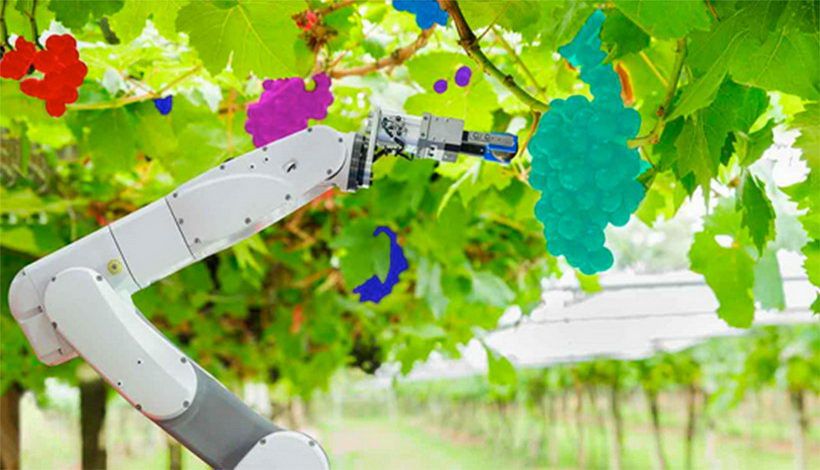
- Aerial crop monitoring: AI powered drones allow farmers to survey large areas of crops. This allows them to assess, plant growth, diseases, field conditions, and where to spray. This technology in part relies on polygon annotation to map correct areas of crops in training images.
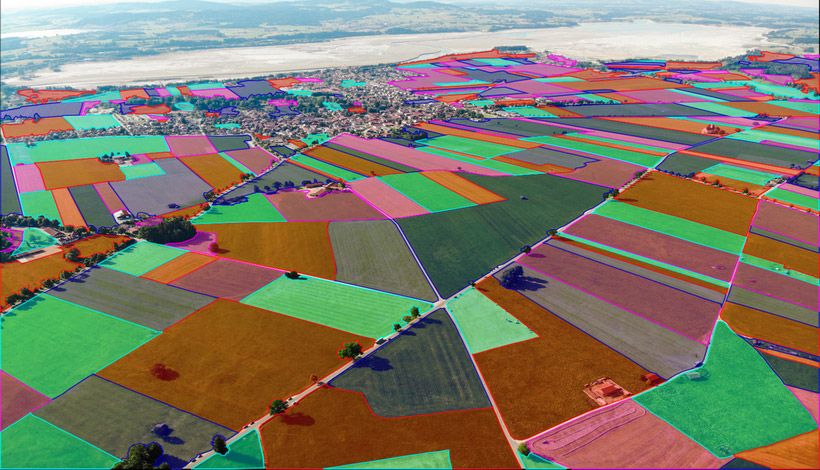
- Livestock management: Livestock management AI are helping to increase yields and safeguard animal welfare on farms. These models can identify individual animals and ensure that none are missing. It can also identify if an animal is sick or injured, providing vital early warning. Polygon annotation can be useful in training livestock management AIs because it can accurately define the irregular shapes of different species and breeds of animal.
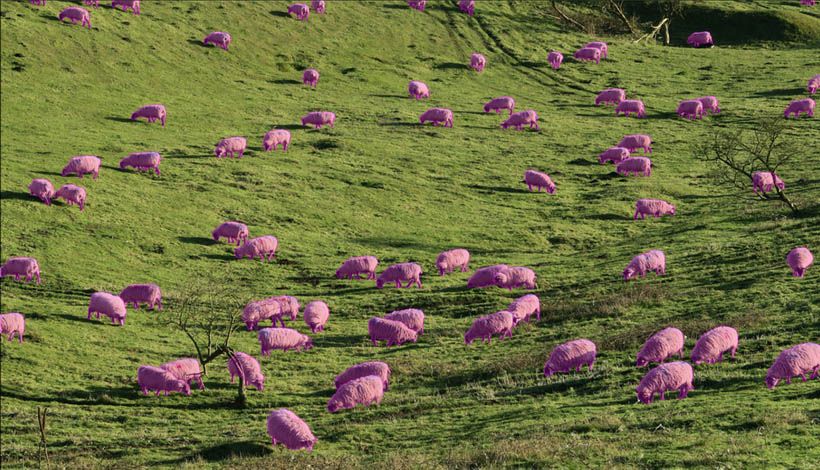
How to maximise polygon annotation
Polygon annotation is an essential component in the development of AI systems for precision agriculture. Outsourcing to experienced service providers can allow developers to access polygon annotation without the burden of in-house management:
- Management: Managing a large annotation project can detract from the core mission of any AI company. Providers, like Keyamakr, have large teams of skilled annotators that are capable of meeting the demands of most annotation tasks. Experienced managers also help to ensure that troubleshooting and quality issues are communicated with annotators quickly and accurately.
- Cost: Outsourcing to annotation experts can often be more cost effective than building an in-house annotation process. Many providers offer discounts for larger volumes of data, and annotation costs can be easily matched to development budgets.
- Proprietary technology: Outsourcing can also allow AI companies to take advantage of cutting edge annotation platforms. These tools can accelerate annotation work whilst providing managers with detailed analytics that improve project planning.
Keymakr is working with leading innovators to make AI for precision agriculture a reality.
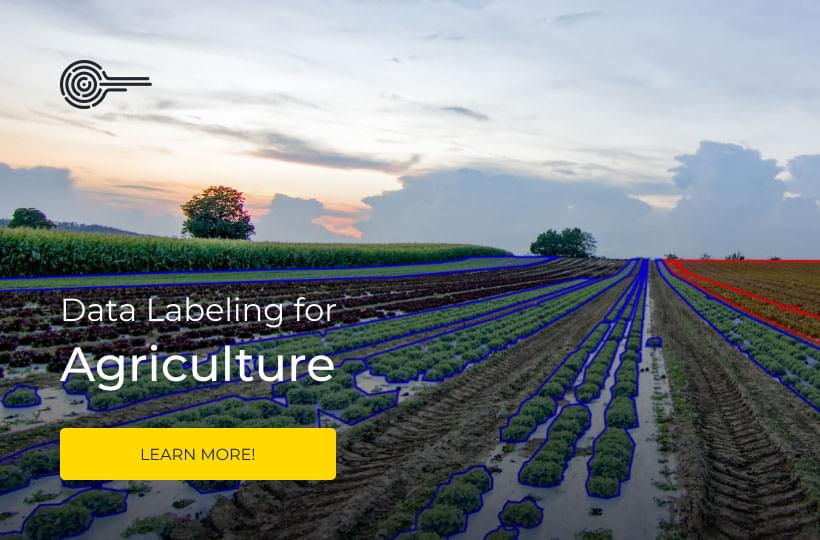