Overcoming Bias Challenges in Aerial AI
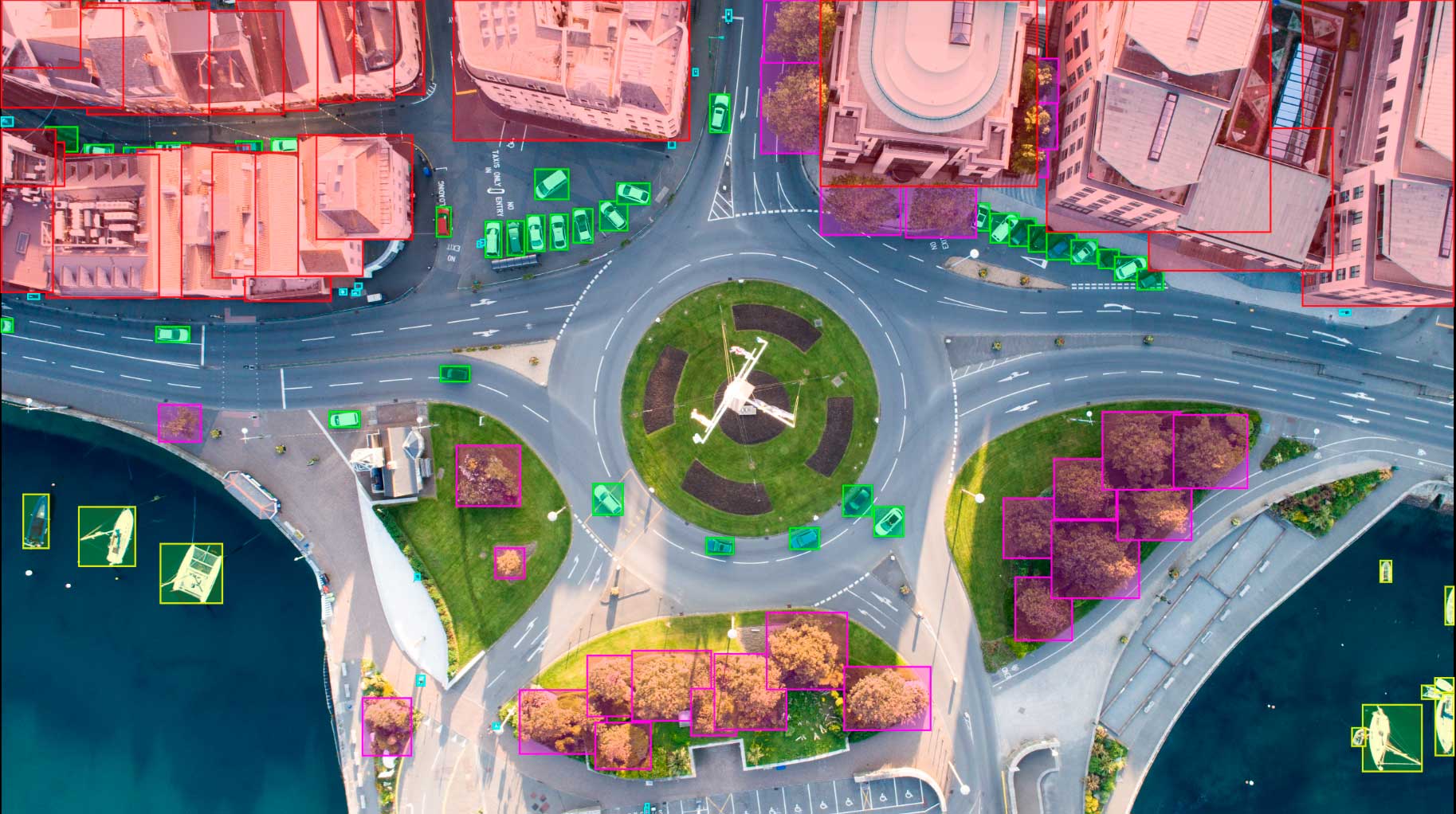
Drone based computer vision AI systems operate in a wide range of environments and perform a variety of important functions. Automated drone systems can be used to monitor livestock, chart the growth of agricultural crops, and even find missing people in the aftermath of natural disasters.
In each use case effective performance is dependent on the foundation of accurate and varied image and video training data. If training data does not accurately reflect the diversity and complexity of the real world then AI models will display biases that will affect their functionality. To overcome these problems it is necessary to collect and create data that tackles specific bias issues.
This blog will examine four bias challenges that can adversely affect aerial AI applications. Data annotation services can help developers to overcome these hurdles by providing expert data collection and creation services in addition to accurate labeling and verification.
Variable weather conditions
Most training data for drone AI applications features good weather conditions, and is captured in a relatively small number of climate regions. This may leave drone AI systems vulnerable when they are called on to operate in radically different weather conditions. A model trained in southern california, for example, may be less functional when deployed in a tropical region, or a drizzly northern european one.
Images and video for drone AI training datasets must therefore include a range of weather types. It is important to maintain precision when annotating varied weather data, as target objects may be harder for annotators to locate and segment.
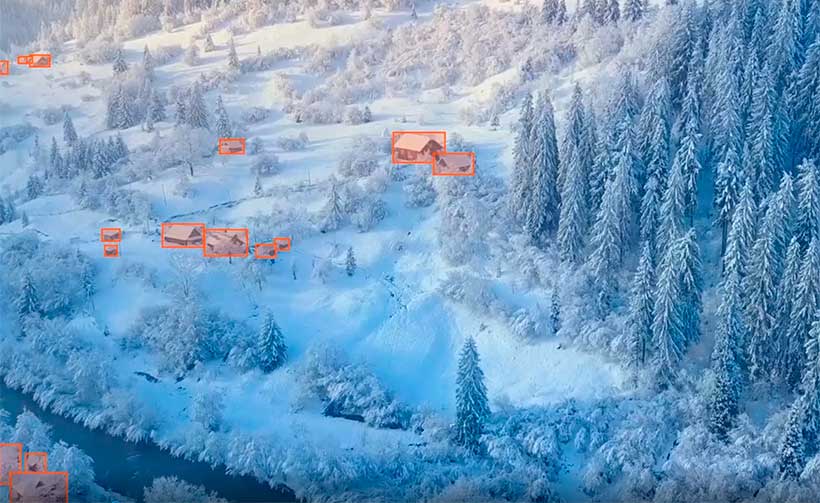
Landscape and ecological diversity
Landscapes and natural environments can differ radically between and within global regions. AI training data tends to mostly originate from the United States and as a result it may not be able to capture the differences present in other landscapes. This is particularly important for drone based systems which need to analyze birds eye view images of particular terrain, buildings, vegetation etc.
Lack of topographical variety in training data can cause specific issues for search and rescue AIs. If a sea rescue model has been exclusively trained on relatively flat rivers and lakes it may be unable to operate when asked to find people or vessels on the open ocean. Collaboration with data annotation services may help developers to access data that emcompasses a range of landscapes.
Changing light levels
Training data that predominantly features clear, high-visibility, light conditions may be problematic for drone AI models. Many drone AI systems will be asked to function in early morning or evening scenarios, particularly if they are engaged in search and rescue operations. Dealing with fluctuating light levels requires varied and comprehensive training datasets.
Diverse livestock breeds
Livestock monitoring drone AI applications have shown exciting potential to increase yields and animal welfare. Drone mounted camera systems can identify illness or injury in large herds and can even be used to quickly locate missing animals.
However, in order for this important technology to function reliably it is necessary for training data to reflect diversity of livestock types as well as intra-species breed diversity. Attention paid to this complexity will also allow livestock management applications to perform well in a wide range of cultural contexts.
Finding smart data collection and creation services
As has been shown, overcoming bias in drone based AI models is in part dependent on the intentional creation of varied and precise training datasets. Keymakr, is a data annotation service that specializes in collecting images and video that fits the specific needs of today’s most exciting AI projects.
If the right data cannot be located through open sources, Keymakr’s in-house production facilities allow for flexible data creation.
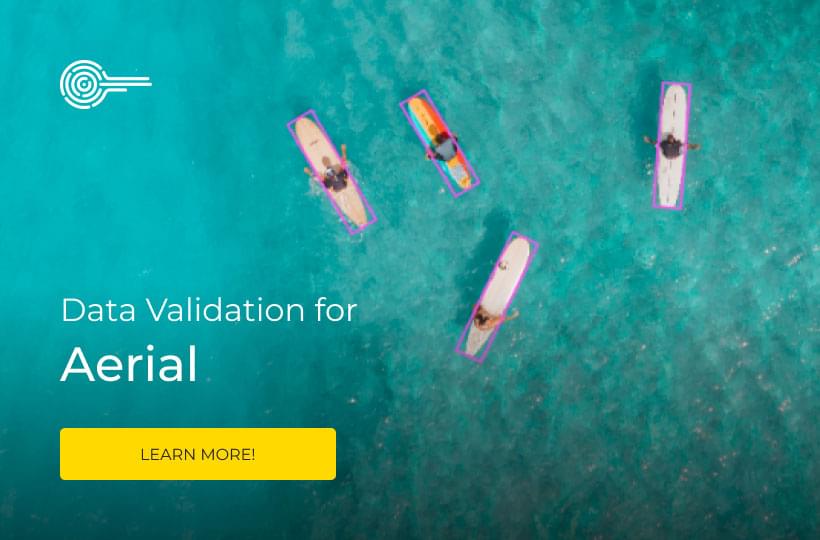