Must Read AI Papers On Semantic Segmentation [2023 Update]
Researchers produced AI papers on segmentation techniques at lightning speed in 2023. Reading them without Elon Musk’s Neuralink isn’t possible, so we’ll highlight a few worthy papers. These papers note AI face segmentation, object detection, and instance segmentation developments.
Segmentation is the process of breaking down an image into specific labels. A photo of a city street, for example, needs labeling for each pixel. It’s an important step for the machine vision we demand for autonomous driving. Without accurate AI segmentation data, we can’t expect safety from self-driving vehicles. We also need segmentation for AI image generation, plus other applications.

Common Real-World Uses For Segmentation
Interpreting data from autonomous vehicles
Understanding spatial information is key to self-driving software. Using segmentation to define each pixel is necessary too. For a variety of reasons, AI segmentation methods need to define and give value to objects in their field of view.
Diagnostics using medical imagery
Defining body areas is valuable, especially for those needing medical attention. Medical care and early diagnoses improve as a result of AI segmentation.
Take a quick look at these must-read 2023 AI papers on segmentation.
Autonomous Driving: 4D-Net for Learned Multi-Modal Alignment
Walking without sight can be hazardous. Autonomous driving without machine vision is even more dangerous. Waymo and Google Research produced this paper about 4D-net. The program combines data points from 3D LiDAR and regular cameras. That information improves autonomous driving technology.
Most self-driving cars use a combination of LiDAR and cameras. Tesla only uses data from cameras. They do this by utilizing data from Autopilot cameras provided by customers. Other manufacturers believe in two forms of machine vision, not one. They prefer depth perception from LiDAR with object detection and instance segmentation.
Set your Tesla to Autopilot and check out the paper right here.
Turkish Survey On Deep Learning For Semantic Segmentation
This paper focuses on the current state of 2D image AI segmentation. It’s a worthy starting point for anybody wanting to be abreast of relevant advances. Semantic image segmentation is the process of defining an image down to the last pixel. Imprecise machine vision undermines the entire deep learning process, so this area is vital. The author’s goal is to aid future directives by understanding past methods.
This paper summarizes semantic segmentation advances of the past decade. Additionally, the author included recent developments for comparison. That’s what makes it such a worthwhile read; it’s many papers compressed into one. First, you’ll be able to see the evolution of 2D image semantic segmentation models. Then, the author compares them to current state-of-the-art applications. Finally, helpful visuals and tables display information alongside comparisons of current and prior techniques.
If you want to bring yourself up to speed on semantic segmentation, read the paper here.
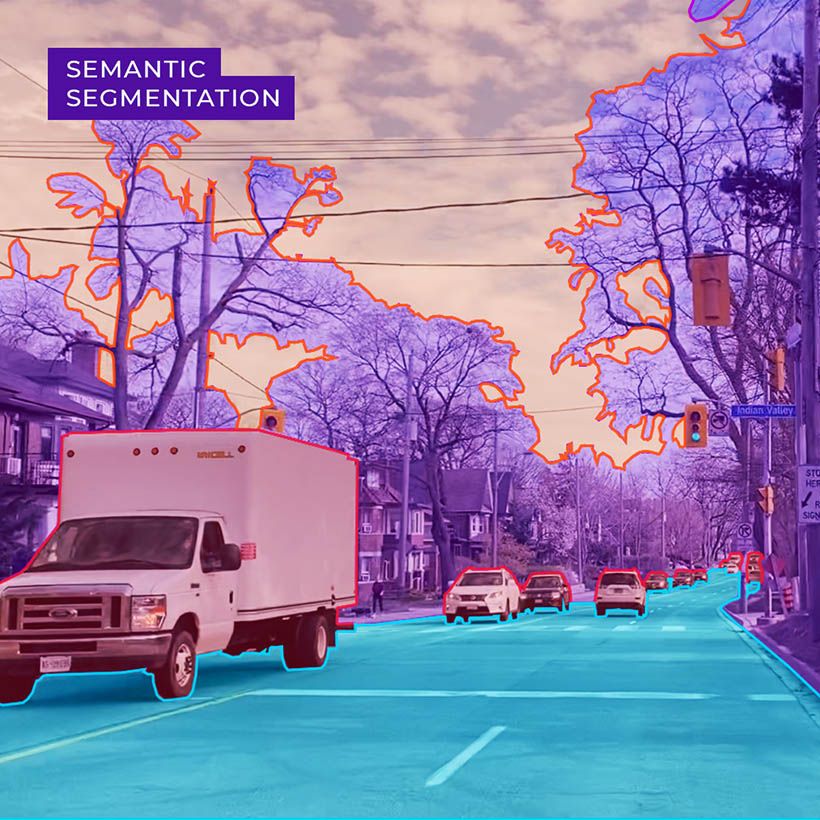
A Personalized Generative Prior For Real-Time Facial Photoshop
MyStyle is a genius program that uses AI face segmentation to alter facial features. The software creates expressions at a level we’ve not seen before. The creators say that their program surpasses current leaders in this software niche. They’re saying it produces higher-quality results in less time.
Think of MyStyle as a deepfake program with customizable parameters. First, it works by building a reference dataset of a subject's facial proportions. It uses around 100 portrait images to form this model. Next, a pre-trained program called StyleGAN takes the data. With it, software builds an accurate low-dimensional model. By building an accurate reference, MyStyle can realistically alter details about a subject’s face.
The paper from Google Research and Tel-Aviv University is here.
Learning Perpetual View Generation of Scenes from Single Images
InfiniteNature uses image segmentation techniques to transport us worlds away. The creators are from Google Research, Cornell Tech, Cornell University, and UC Berkeley.
We’ve all zoomed in using Google Earth, but this software’s level of detail is leaps and bounds ahead of that. Imagine flying into a photograph. You'd have control over your perspective as you zoom through it with great detail. InfiniteNature needs a single reference image to create this experience, not hundreds.
InfiniteNature sends a virtual camera on several trajectories. As it moves, it measures perspective angles, building a viewable model. By using AI segmentation, we can map features like mountains and skylines, and adjust the camera's trajectory. Since it moves rapidly, the sky can be difficult to generate. InfiniteNature accounts for this by using segmentation techniques and dynamic image blending.
Check out the 20-page paper on InfiniteNature right here.
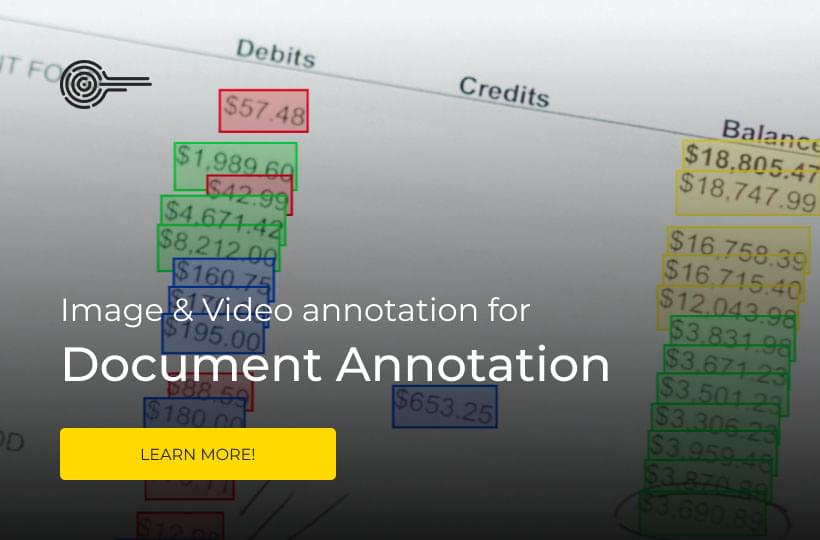