Improving Harvesting Robots With Data Annotation
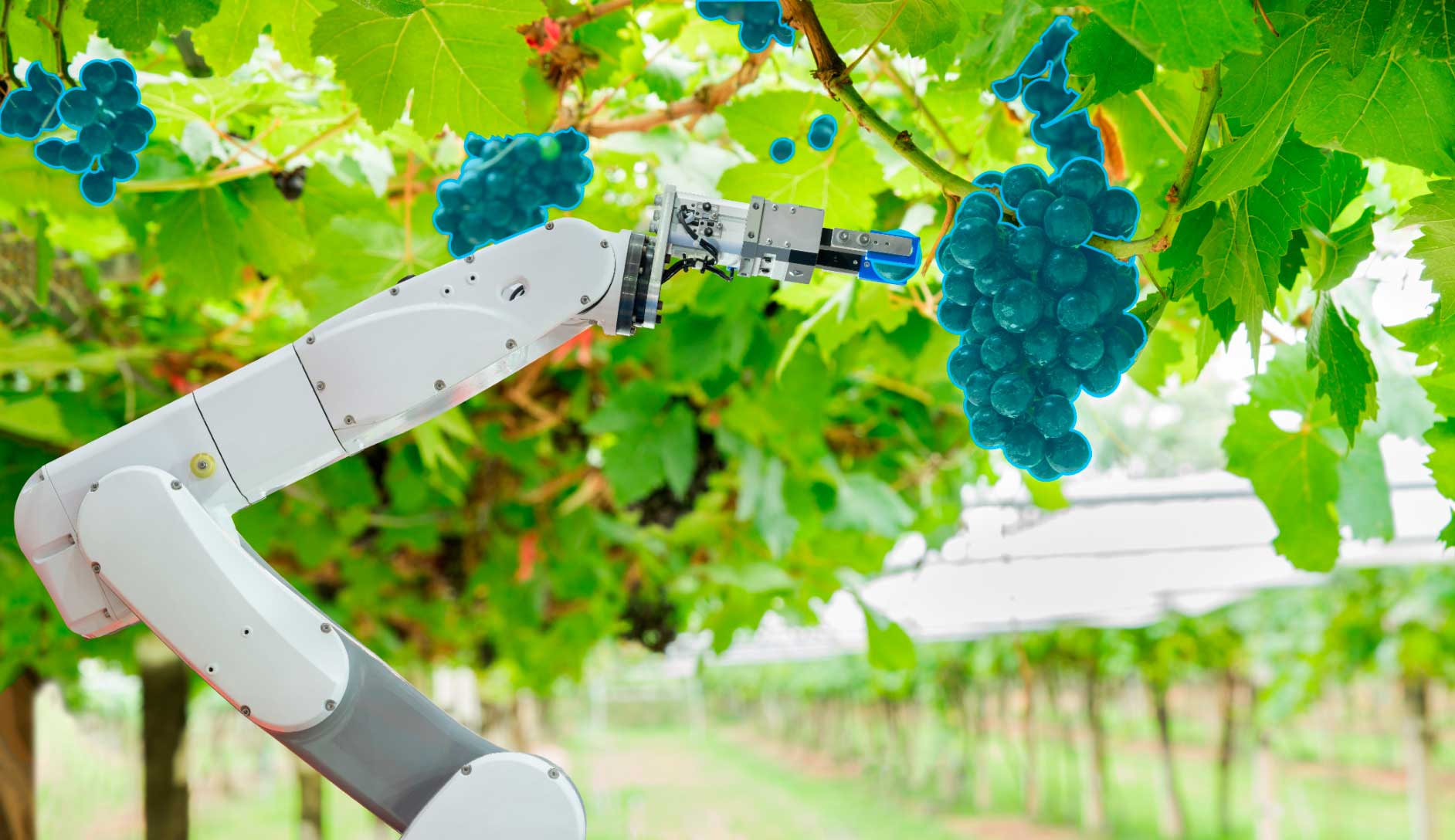
COVID-19 had led to changes in many industries. Many offices have moved to a remote working model, and supply chain stresses have impacted many businesses. In the agricultural sector the pandemic has led to a labour crisis. The industry has responded by looking to new technology for solutions.
Computer vision models in combination with new robotic systems are being developed with the aim of automating harvesting. Harvesting robots can ensure that the food supply remains secure, even as the labour market changes.
However, challenges still remain for this computer vision technology. Precise data annotation can help AI companies to realise the potential of harvesting robots. Data annotation services can provide innovators with high quality datasets that improve model performance.
Firstly, this blog will look at why we need robotic harvesting systems. Secondly, we will examine how systems currently in development work. Thirdly, we will identify the ways in which data annotation supports innovation in this sector. And finally, we will show how data annotation services help companies access the best training data.
The need for automated harvesting
The pandemic has shone a light on some of the problems facing the agricultural sector. Automated harvesting systems can help to overcome these challenges:
- Labour shortages: COVID-19 had led to major problems with finding and retaining farm workers. The illness prevents people from working on farms and forces others to isolate after close contacts. Migrant workers who normally take on the bulk of harvesting have been unable to travel. The pandemic exacerbated long standing problems in the sector. Many potential workers are put off the industry by the difficulty of the work and the low pay.
- Fragile fruit: Lots of crop types are difficult for robots to pick. Fruit might be obscured by leaves for example. Produce like tomatoes are also extremely delicate, and need to be handled with care. This can be difficult for robots to achieve. As a result, computer vision development is necessary if these systems are to be widely used.
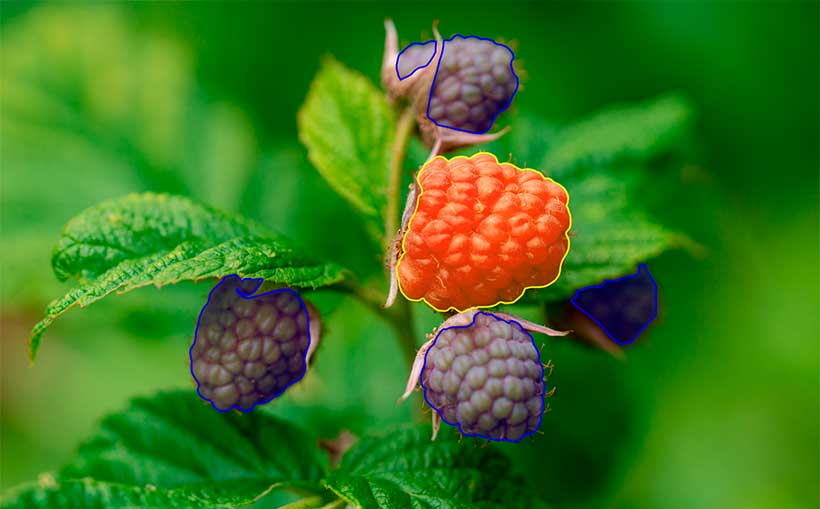
The future of AI harvesting
Computer vision models can be trained to recognise objects, like fruit, and distinguish from other objects, like leaves and branches. In addition, AI systems can be taught to recognise how ripe a piece of fruit is. Annotated images show the different colours of fruit at different stages of ripeness. This data trains AI models to spot perfectly ripe fruit in the real world.
Computer vision models can be integrated with robots. AI tells the robot what to pick, and robotic arms are now capable of gently grasping soft fruits. Once this technology is refined and made cheaper it could revolutionise the harvest.
Data annotation is important
The computer vision models that make automated harvesting possible need to learn from annotated image and video data. The following annotation methods are a vital part of creating datasets for this technology:
- Segmentation: Images can be divided in classes. Segmentation assigns each pixel in a digital image to a class. This added level of contextual information helps harvesting AIs to spot ripe fruit more easily.
- Varied data: Harvests take place all over the globe. This means that training data needs to reflect the variety of environments in which harvesting robots could operate.
- Quick outlining: This function creates automatic outlines for shapes in digital images. This means that annotators can label fruit in harvesting AI training data much faster.
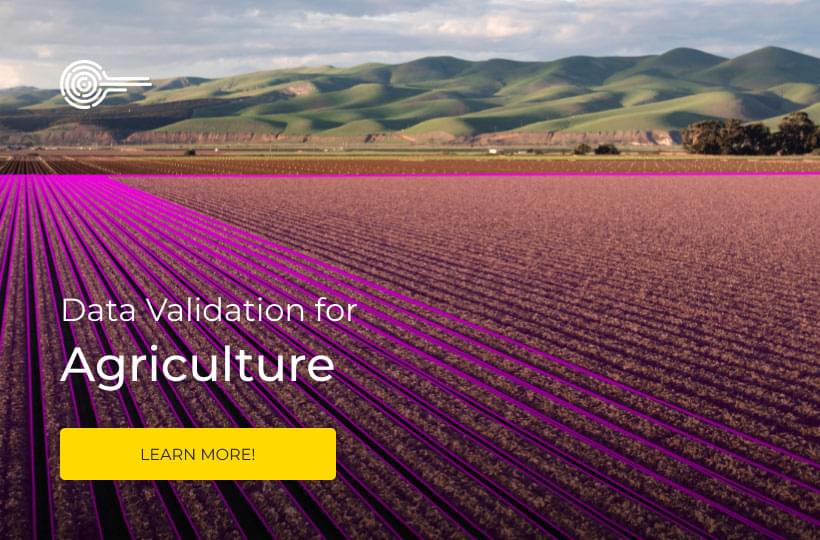
Annotation service advantages
Keymakr is an image and video annotation provider with a track record of producing outstanding training datasets. Partnering with Keymakr allows AI companies to scale data labeling in line with the needs of their models. Keymakr’s in-house annotation team ensures precise, affordable annotation services.
Contact a team member to book your personalized demo today.