How Data Creation Services can Support AI Development
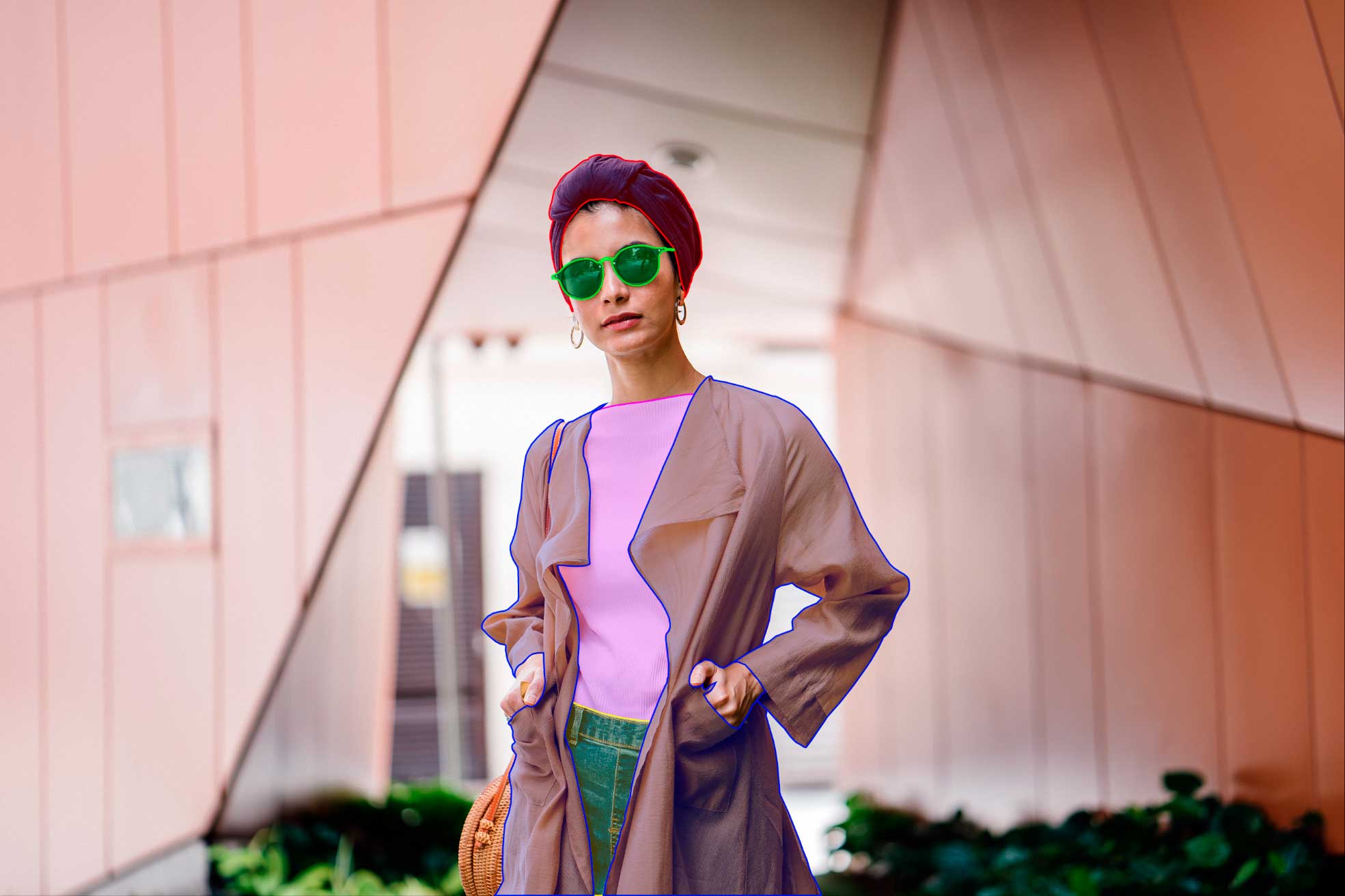
Most image and video training data for machine learning is collected from large open sources like Imagenet. These online repositories allow developers to assemble large pools of data that can then be annotated with labels and categories, creating functional datasets that power computer vision based applications. Whilst data collection resources are sufficient for many AI projects, there are times when it can be hard to find images and video that are suitable for very specific training needs.
Data creation allows AI innovators to access the particular images and video that their projects require. It means taking production facilities, such as cameras and studio spaces, and manufacturing images and video according to the needs of a given computer vision project.
This blog will lay out the most important features of powerful AI training datasets, and show how data creation can overcome a range of bias issues.
The Key features of effective training datasets
Data creation allows AI developers to access highly effective datasets that display a variety of important qualities:
- Variance: Dataset images and video need to contain a varied collection of objects that might be of relevance to a computer vision model. Training data must reflect the diverse range of people and things that make up real environments.
- Density: Images must feature a large enough number of objects so as to reflect more chaotic real-world situations. Under populated training images may result in poorly performing systems.
- Quantity: A dearth of quality images and video can be a problem for machine learning. A lack of data of sufficient quality of efficacy can lead to issues in development.
- Quality: Image quality can be determined by a number of factors. Clearly distinguishable objects, with defined boundaries, result in high-quality labeled images. Some image data can feature artefacts and oddities as a result of human error, and poor framing of objects could result in unusable training data outputs.
Accounting for bias with data creation
Creating new images and video is a means of avoiding bias in datasets. Data that does not reflect key aspects of the real world can lead to models that perform sub-optimally. Data creation results in training datasets that reflect complexity and difference:
- Light conditions: Training data needs to represent different light conditions. It is important for some AI applications to perform at night or in low light. If training data predominantly represents day time conditions it is necessary to create alternative low-light level data.
- Weather: Images and video taken in predominantly dry and sunny locations may not reflect the reality of other climates. Creating data that features rain or low-visibility weather allows models to function in a range of environments.
- Ethnic and gender diversity: When AI applications are asked to identify individuals and interpret human behaviour it is essential that they are trained with data that encompasses the diversity of different populations and societies. Data creation may be the only way to capture ethnic and gender diversity.
- Cultural contexts: Capturing diversity extends to representing distinct cultural practices in training images and video. Certain customs around eating or socializing, for example, may lead to difficulties in recognition for AI models.
- Road conditions: For automated vehicles it is essential that training data captures the differences in road conditions between countries. This could mean driving on the left, differing road markings, different coloured road material, and varied street furniture.
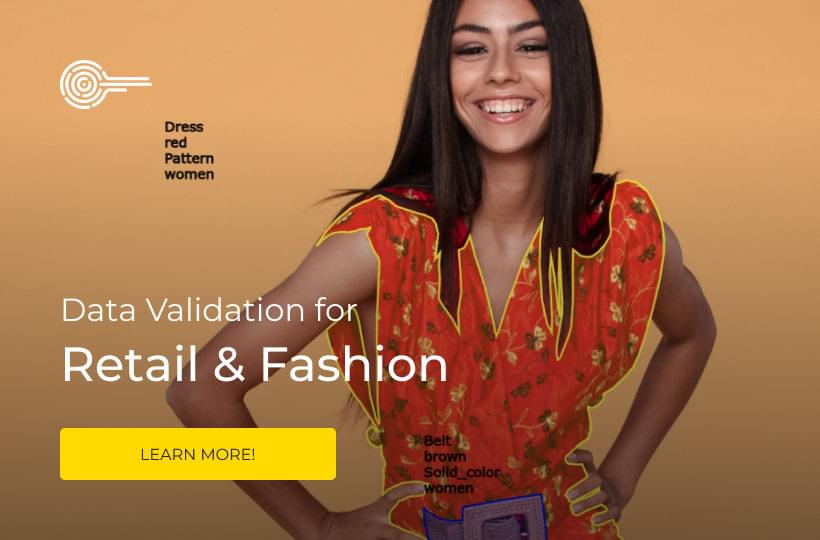
How to find data creation services
Data annotation providers, like Keymakr, are often best placed to provide data creation services to AI companies. By combining expertise in image and video labeling and verification with in-house production facilities Keymakr can construct bespoke training datasets that meet the needs of computer vision pioneers.