Geospatial Data Annotation
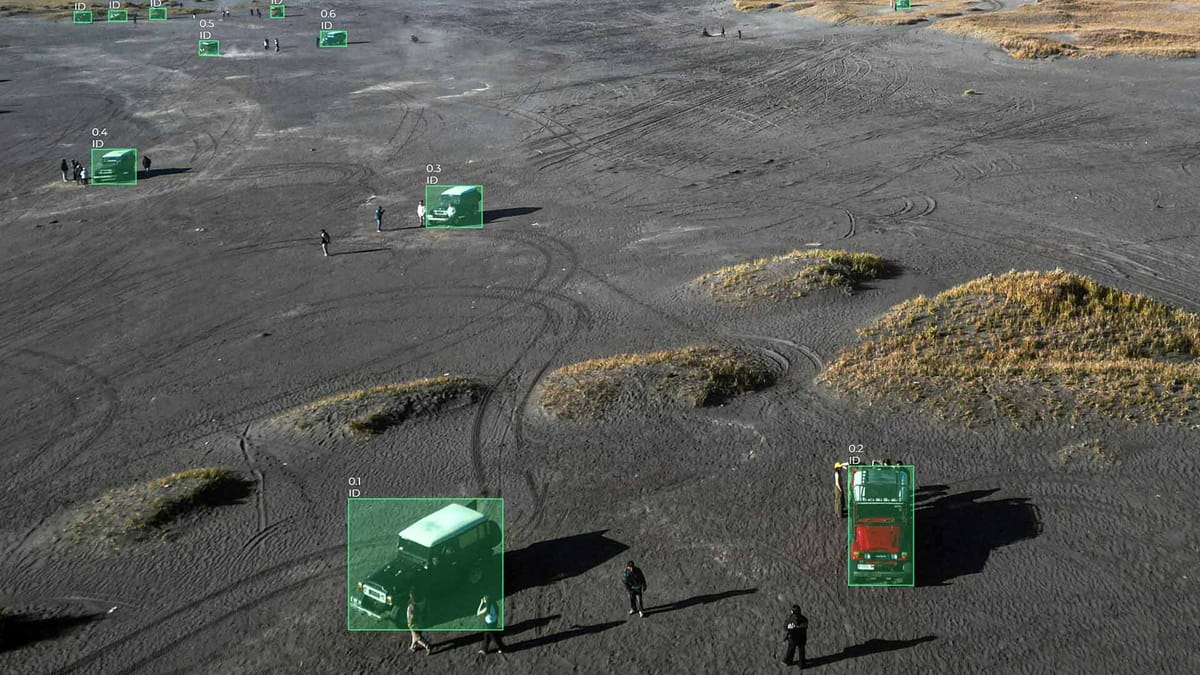
Geospatial data annotation allows us to extract meaningful information from satellite imagery. Labeling features, buildings, roads, and vegetation can improve agriculture and construction processes and support aerospace and defense operations. Logistics planning and critical disaster management efforts rely on accurate spatial data labeling, essential for making informed decisions across various sectors.
Advanced tools help annotate complex geographic data through satellite imagery analysis. Geospatial data annotation supports various sectors, from urban management to forest analysis.
Quick Take
- Geospatial data annotation improves agriculture by optimizing irrigation, fertilizer use, and pest control.
- Construction processes are improved by analyzing and annotating GPS data and satellite imagery.
- Threat detection in the aerospace and defense industries is improved by converting aerial imagery into geospatial 3D models.
- Route planning is optimized using methods like polyline annotation.
- Geospatial annotation for mapping disaster areas helps in rescue operations.

Definition and Importance of Geospatial Data Annotation
Geospatial data annotation analyzes and labels geographic images from satellites or aerial photographs. It labels features, buildings, roads, and vegetation. The importance of geospatial data is highlighted by its use in urban planning, agriculture, and disaster management.
Application Benefit
The applications of geospatial data are extensive and diverse.
Types of Geospatial Data
Different types of geospatial data contain information that supports decision-making in different industries. We will explore their benefits and applications in different geospatial data formats.
- Satellite imagery provides panoramic views of large areas, capturing data at a macro scale. They are needed for climate monitoring and urban planning, often covering large areas. Satellite imagery is a type of raster data consisting of a matrix of cells, where each pixel represents a specific spectral value.
- Aerial imagery is high-resolution data from airborne sensors that provide detailed images for analysis. It is needed for surveying, real estate valuation, and agriculture. Unlike satellite imagery, aerial imagery allows for localized information.
- LiDAR data uses laser light to measure distance, creating accurate 3D models. It is needed for forestry management and urban reconstruction. LiDAR data is important for creating digital terrain models and other geospatial products.
The Process of Geospatial Data Annotation
Several steps are required to ensure the quality and accuracy of geospatial data annotation.
Annotation Techniques
Let's consider the main annotation techniques:
- Semantic segmentation divides an image into regions and classifies each pixel into a specific class.
- Object detection is the identification and localization of specific objects in an image using bounding boxes.
- Polygon annotation is the creation of detailed outlines around objects to reflect their shape.
Quality Control Measures
Key Quality Control Measures:
- Consensus scores are scores on specific classifications, ensuring consistency in annotations.
- Active learning improves labeling accuracy by integrating AI models that indicate areas where human supervision is needed.
- Software quality assurance automates workflows to validate datasets.
Tools and Technologies for Annotation
The development of geospatial annotation software and the integration of AI have revolutionized this field, significantly improving the accuracy of annotation.
Platform Keylabs has various geospatial data annotation tools that allow you to accurately label satellite imagery, aerial photography, and LiDAR data. With features like polygon annotation, movement tracking, and automated segmentation, Keylabs accurately processes geospatial data sets. Integrating workflows with artificial intelligence optimizes data labeling, providing high-quality annotations for machine learning models in geospatial analysis.
Automation and AI in Annotation
Automation tools increase efficiency by processing large datasets and complex images with high accuracy. Autosave features in geospatial annotation software are also critical. Keymakr has an updated platform with an autosave feature that reduces annotation errors and improves accuracy. They allow data labeling applications to work with massive images without losing progress. The combination of software and AI ensures accurate data processing.
Challenges in Geospatial Data Annotation
Let's consider the most common problems in Geospatial Data:
Areas of Annotated Geospatial Data
Let's consider three main areas: urban planning, environmental monitoring, and disaster response.
Urban Planning
Geospatial data helps manage resources and develop strategies more effectively. Planners create detailed land use maps using feature recognition, area segmentation, and landscape classification techniques. These maps highlight buildings, roads, and water bodies, improving the accuracy of urban planning projects.
Environmental Monitoring
In environmental monitoring, geospatial data is vital. It tracks changes like deforestation, pollution, and habitat destruction. Annotated data allows for the precise location and timing of these changes. It helps in creating effective conservation plans.
AI tools process complex data efficiently. They provide a clear view of environmental trends.
Disaster Response
Annotated geospatial data helps visualize damage, plan evacuation routes, and assess satellite imagery and other data to reduce the impact of a disaster.
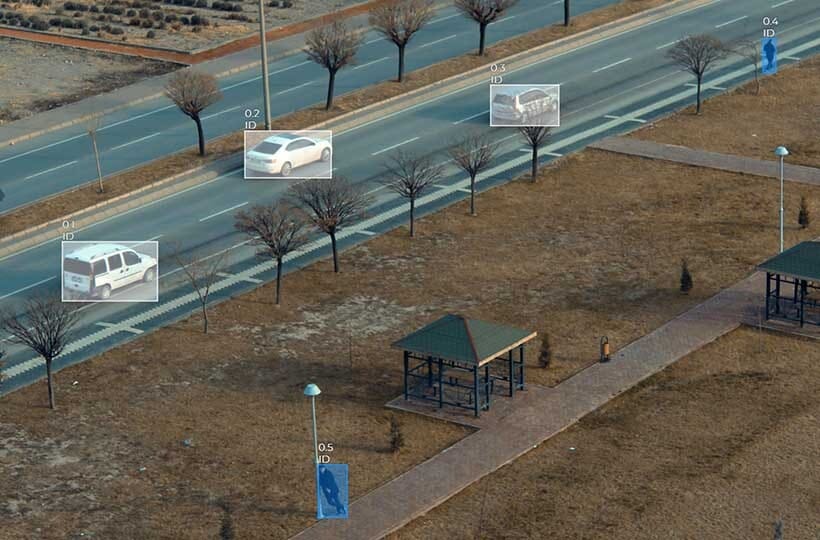
Practices for Annotation
We'll look at two important methods: data standardization and team collaboration.
Standardization of Data
Data standardization ensures that data adheres to established formats, making integration and analysis seamless. It is important in complex satellite, aerial imagery, and LiDAR data projects.
- Consistency removes discrepancies, simplifying analysis.
- Interoperability allows teams to share and use datasets across platforms and applications.
- Accuracy ensures high-quality annotated data, which is vital for machine learning models.
Regular audits and feedback loops are essential to maintain these standards.
Team Collaboration
Collaborative geospatial projects leverage team expertise.
Collaboration leads to data-driven AI solutions that improve the dataset. Key aspects of team collaboration include:
- Communication aligns team members' goals and expectations.
- Shared tools manage scalability and support team efforts.
- Feedback mechanisms ensure continuous improvement of annotated data.
Data annotation platforms often lack these features. Investing in advanced tools can reduce redundancy while improving the dataset's quality.
Innovation in Technology
The global data annotation market, valued at USD 695.5 million in 2020, is expected to grow at a CAGR of 27.1% from 2021 to 2028.
It highlights the importance of accurate data annotation for advancing computer vision technologies. The need for precise object detection, face recognition, and automatic image labeling are key aspects driving the growth of this field.
Increasing Demand Across Industries
The demand for geospatial data is increasing across various sectors. Autonomous driving, augmented reality, and climate change research are examples.
The adoption of LiDAR annotation in urban planning and video annotation techniques like frame-by-frame annotation and action recognition are also rising. The geospatial market is predicted to reach $256 billion by 2028.
This growth shows the increasing demand and the need for advanced technologies in geospatial annotation.
Environmental research
Carbon monitoring projects are annotated satellite images for assessing carbon footprints and biodiversity.
Annotating features in ecological zones has allowed tracking of deforestation and environmental disasters.
It helps environmental agencies manage waste and control pollution. Annotating high-resolution aerial imagery allows for tracking landfills.
Accurate annotations in agriculture have increased yields and minimized costs. It allows for resource management and crop control.
Impact on Urban Development
Urban planning projects have detailed road segmentation and building area annotation thanks to geospatial data. It is necessary for infrastructure development and city management. Accurate mapping of urban areas helps city planners and authorities. They make informed decisions about
Future Outlook for the Industry
Geospatial data annotation is critical in urban planning, environmental monitoring, and disaster response. It provides data accuracy for AI models, application methods, land cover classification, and feature detection.
Thanks to drones, AI, and machine learning, the future of this field predicts significant discoveries. The growing need for accurate data will make annotation a key element of global solutions and technological breakthroughs.
FAQ
What is geospatial data annotation?
Geospatial data annotation analyzes and labels geographic images from satellites or aerial photographs. It labels features, buildings, roads, and vegetation. This process transforms raw data into structured data.
Why is geospatial data annotation critical?
It transforms raw spatial data into actionable information, supporting areas such as urban planning, agriculture, disaster management, and environmental monitoring.
What are the key applications of geospatial data annotation?
Key applications include urban planning, environmental monitoring, disaster management, and precision agriculture. It offers detailed spatial data for thoughtful city planning, tracking environmental changes, assessing disaster impacts, and optimizing agricultural operations.
What types of geospatial data are there?
Geospatial data includes satellite imagery, providing a broad overview of large areas. Aerial imagery offers high-resolution data for detailed analyses. LiDAR data captures precise measurements for creating detailed 3D environmental models.
How is geospatial data annotated?
The process begins with data preparation, including cleaning and organizing data. Techniques like semantic segmentation, object detection, and polygon annotation are used for accurate labeling. Quality control involves thorough checks and expert validation to ensure data accuracy and reliability.
What challenges are in geospatial data annotation?
Challenges include maintaining accuracy and precision with large and complex datasets, addressing geometric distortions and image quality variability, and handling sensitive location-based data requiring stringent data security measures.
How does annotated geospatial data support urban planning?
Annotated geospatial data provides detailed information for urban planning. It helps planners design sustainable and smart cities.
What is the role of annotated geospatial data in environmental monitoring?
In environmental monitoring, annotated geospatial data tracks changes like deforestation, urbanization, and pollution levels. It provides critical information for conservation efforts.
How does geospatial data annotation aid in disaster response?
Annotated geospatial data allows for rapid assessment of affected areas during disasters. Aid distribution, infrastructure restoration, and fast disaster response are necessary.
What are the best practices for geospatial data annotation?
A method for standardizing data for consistency and compatibility between datasets and team collaboration.
What is the future of geospatial data annotation?
Advances in AI and machine learning are improving annotation tools. The growing demand for detailed geospatial data is driving further innovation and adoption of the technology.
What are some examples of successful use of geospatial data annotation in different industries?
Environmental research, where annotations track and preserve biodiversity. Applications for urban development help design sustainable cities.
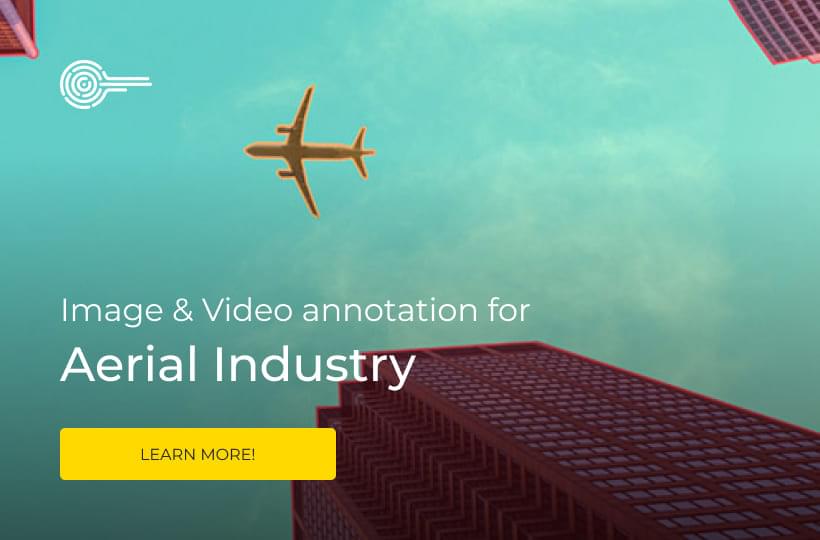