GDPR and Data Labeling: Best Compliance Practices for EU Markets
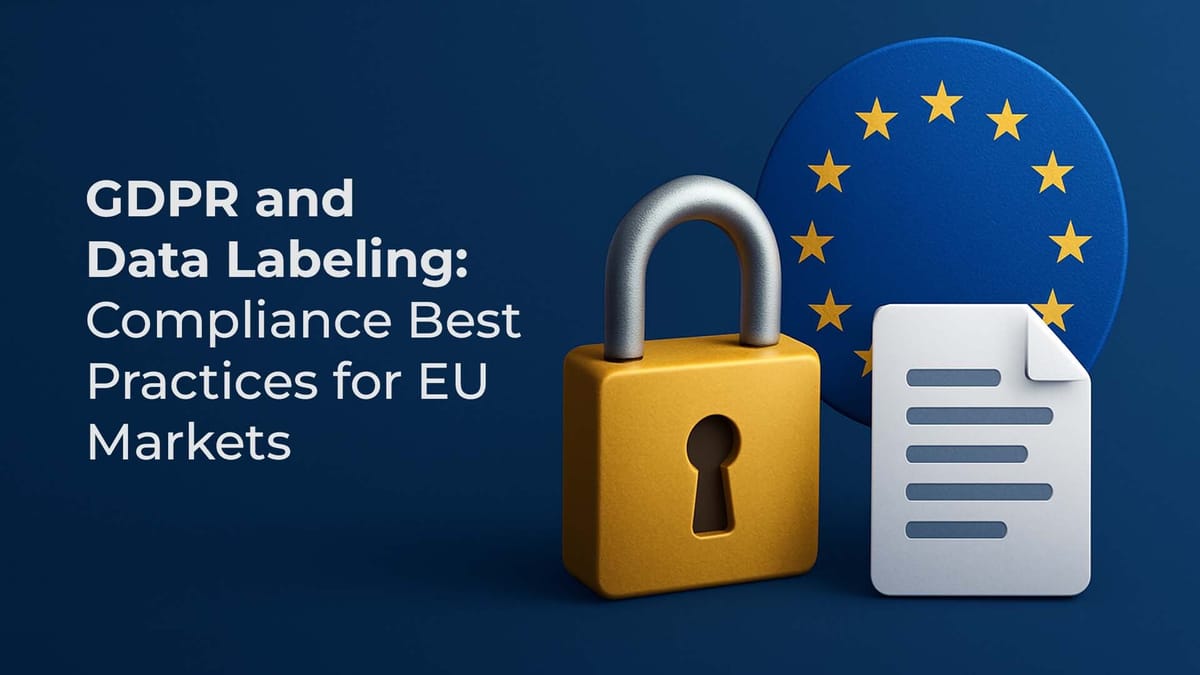
Organizations that process personal data understand the impact of GDPR on data protection and labeling practices.
The GDPR, established in 2018, ensures data integrity and confidentiality. However, labeling personal data in a data protection-compliant manner can be challenging, especially when working with raw data.
Quick Take
- Accurate labeling of personal data preserves data integrity.
- Obtaining informed consent and ensuring data protection are key GDPR requirements.
- Working with raw data requires transparent processes to comply with GDPR guidelines.

Understanding GDPR for Data Management
The GDPR (General Data Protection Regulation) is a key EU regulation enacted on 25 May 2018 to ensure GDPR compliance in how organizations collect, store, and protect personal data.
One key aspect of the GDPR is access control. This means that only authorized personnel can work with sensitive information. The regulation also requires clear communication protocols to ensure people understand how their data is used. With this guidance, organizations can improve accuracy and reduce the risk of data breaches.
Principles of Data Labeling and Privacy
Privacy is the main ethical rule of data processing, ensuring the transparent handling of personal information. In data labeling, privacy defines how personal information is protected.
Thus, accurate labeling and adherence to privacy principles create a solid foundation for ethical data use.
Obtaining informed consent from data subjects
Building trust between data subjects ensures the relevance of annotated data. Obtaining informed consent from data subjects is a principle of personal data protection under the GDPR and other similar regulations. This means an organization must obtain an individual's free, specific, informed, and unambiguous consent to process their data.
Granular consent options
Different consent options allow people to make informed choices. This means separate consent for other data uses, such as marketing or analytics. Keymakr ensures that consent mechanisms are voluntary, accessible, and easy to withdraw consent, which is in line with general data protection standards.
- Provide clear and concise information about data use.
- Ensure that consent is specific to each purpose.
- Establish easy opt-out options.
- Maintain detailed records of consent.
GDPR and Data Labeling
Compliance with the GDPR is essential to ensure the lawfulness of data collection, processing, and storage, especially when it comes to personal information. Companies must obtain informed consent from data subjects before labeling, provide transparency about the purposes of the processing, and guarantee the right to access, rectify, or delete data. In addition, the risk of identifying individuals should be minimized using anonymization or pseudonymization techniques. Data labeling should be part of a secure process that considers users' rights as defined by the GDPR and protects against unauthorized access.
These practices are essential for full GDPR compliance and alignment with evolving EU regulations.
Distinguishing Data Privacy from Data Security in Data Annotation
Data privacy and security are important concepts that overlap in data annotation but serve different purposes.
Confidentiality thus ensures "what" cannot be disclosed, while security ensures "how" it is protected. Both aspects are critical to maintaining trust and compliance with legal regulations such as GDPR.
Methods for securing data annotation processes
- Work in a secure environment with limited network access.
- Role-based access controls to ensure that only authorized personnel handle sensitive information.
- Regular compliance training to stay up-to-date on new security practices.
Technical security measures
- End-to-end encryption protects data during transmission and storage.
- Multi-factor authentication verifies user identity and grants access.
- Regular audits to identify and address potential vulnerabilities.
Organizations can ensure high data accuracy and security by combining human controls and technical security measures.
Raw Data Management and Data Accuracy
Organizations process various data types, including text, images, sensor readings, and audio files, each requiring precise management to maintain integrity. In healthcare, accurate labeling of medical images is used for diagnosis and treatment.
- Automated tools help to categorize and validate data consistently.
- Regular quality checks ensure accuracy throughout the data lifecycle.
- Cross-validation techniques maintain data integrity and reliability.
Poorly planned data management leads to inaccurate AI model results and non-compliance with regulatory standards. To avoid these risks, validation procedures such as continuous monitoring and periodic audits should be used. This will ensure a high level of accuracy in projects.
Keymakr combines advanced technology with expert oversight to transform raw data into reliable information that can be used to make informed decisions and adhere to industry standards.
Industry regulations and global data protection laws
Data protection laws set standards for collecting, using, and sharing personal information.
Organizations must adapt their systems to these regulations to ensure data protection and compliance.
Data Leakage Prevention Strategies
- Control access to only authorized users to process sensitive information.
- Regular security audits to identify and address vulnerabilities.
- Establish industry standards for a secure data processing environment.
- Multi-factor authentication will provide an additional layer of security for accessing systems.
- These strategies will help organizations reduce the risk of hacking and reliably protect sensitive information.
Automated and Manual Approaches to Data Labeling
Manual approaches to data labeling involve human intervention in annotating data for further use in machine learning. These methods allow for accuracy through a deep understanding of context and nuance. Humans analyze complex situations and recognize irony, sarcasm, or ambiguous wording. Manual labeling is used in the initial stages of a project or to create reference (gold standard) datasets. Although this approach is time-consuming and requires significant resources, it is an important tool in complex tasks.
Advantages of automated labeling
Automated labeling is suitable for processing large data sets. AI-based tools pre-annotate data in hours, reducing the workload. Computerized systems allow teams to focus on complex tasks. AI-powered quality checks maintain high standards and reduce errors in the data annotation process.
Organizations can streamline their workflows by combining manual and automated approaches. Manual labeling accurately performs complex tasks, while automation quickly processes large volumes of data.
Summary
Combining advanced technologies with rigorous compliance protocols helps overcome the complexities of modern data protection. As this article has shown, achieving data compliance and security requires a systems approach that includes privacy and efficiency.
The abovementioned methodologies combine tools with human oversight to help annotated data meet standards. Secure data labeling provides opportunities for innovation and growth in this field. Organizations prioritizing modern technologies and regulatory compliance will be at the forefront as this field evolves.
FAQ
How does GDPR impact data labeling processes in the EU?
GDPR requires companies to ensure that personal data is protected when labeling, including by obtaining informed consent from data subjects.
What steps are needed to ensure data protection compliance?
Privacy policies should be implemented, data audits should be conducted, and sensitive information should be encrypted. Informed consent should also be obtained from users and access to data should be controlled.
How can businesses maintain data accuracy when labeling?
Standardized labeling guidelines and regular quality checks can help achieve accuracy in data annotation.
What steps should companies take to prevent data leakage?
Encryption, access control, and regular auditing of security systems.
What are the key differences between privacy and data security?
Data privacy refers to the information that is collected and used, while data security protects data from unauthorized access and breaches.
What are the benefits of using automated labeling tools?
Automated tools increase the speed of data annotation and reduce human error, making them valuable in large-scale data mining projects.
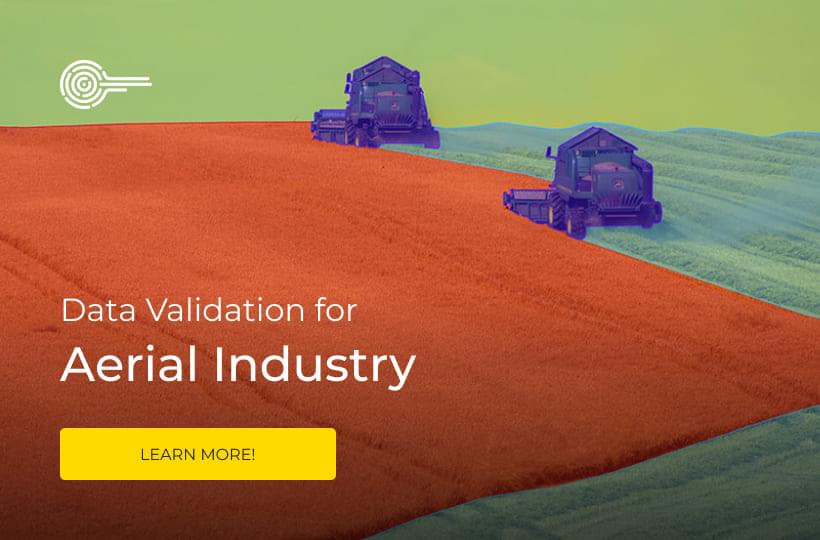