Exploring Open-Source Data Annotation Tools
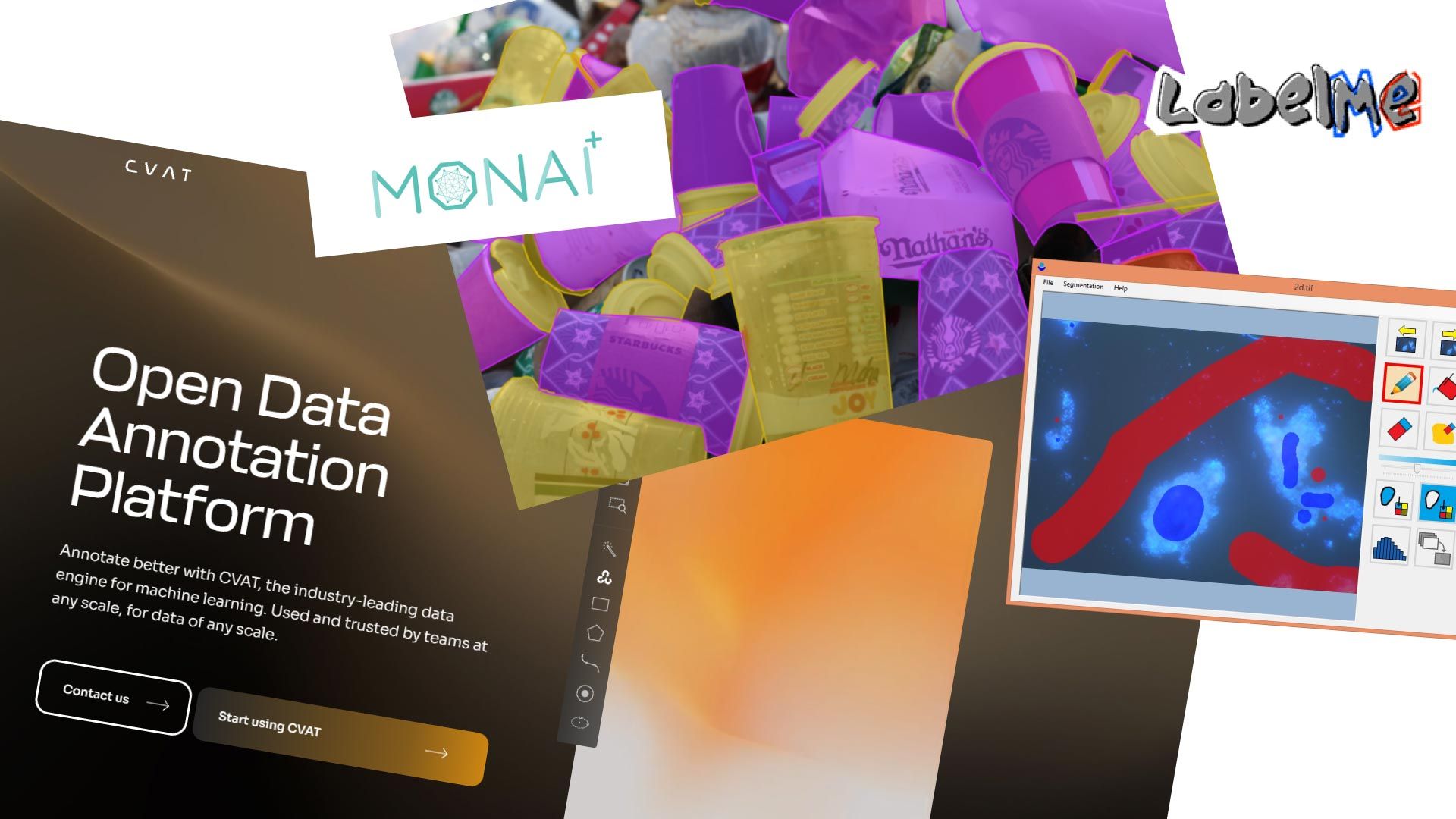
In the field of computer vision and machine learning, open-source data annotation tools play a crucial role in accurate AI data labeling and machine learning model training. These tools are freely available for use, making them a popular choice for startups and academic projects with limited budgets. In this article, we will delve into the details of open-source data annotation tools, discussing their pros and cons and exploring some popular options in the market.

Key Takeaways:
- Open-source data annotation tools are widely used in computer vision and machine learning projects.
- These tools are free to use and are preferred by startups and academic projects with limited budgets.
- Open-source tools may have limitations in terms of scalability and security compared to commercial solutions.
- Understanding the pros and cons of open-source annotation tools is crucial in choosing the right tool for your project.
- Consider the specific needs of your project and weigh the pros and cons of open-source options against commercial solutions.
What is an Open-source Data Annotation Tool?
An open-source data annotation tool is a software specifically designed for image labeling and data annotation in image and video datasets. These tools play a crucial role in training computer vision models by providing the necessary labels and annotations. Open-source tools are freely available for download and use, and they are typically maintained by foundations or sponsored by tech companies. They have gained popularity, particularly among academic projects and startups with limited budgets, due to their affordability and flexibility.
Image labeling and data annotation are essential steps in the development of machine learning models. These tools enable users to annotate objects and regions of interest within images and videos, associating them with corresponding labels or tags. By providing annotated data, open-source data annotation tools contribute to the training and improvement of machine learning algorithms, aiding in accurate object recognition, image segmentation, and various computer vision tasks.
Open-source data annotation tools come with diverse features that allow users to perform a range of annotation tasks. These commonly include image segmentation, bounding box annotation, polygon annotation, semantic segmentation, keypoint annotation, and more. The versatility of these tools makes them suitable for a wide array of applications across industries, such as autonomous vehicles, medical imaging, surveillance systems, agriculture, and quality control in manufacturing settings.
Moreover, open-source data annotation tools are often customizable, allowing developers to modify and extend their functionalities according to specific project requirements. This flexibility makes them a preferred choice for researchers and developers who need tailored solutions for their machine learning projects.
Benefits of using an Open-source Data Annotation Tool:
- Cost-effective: Open-source tools are free to download and use, eliminating the need for expensive licenses or subscriptions.
- Community support: Open-source projects often have active communities, providing resources, tutorials, and assistance to users.
- Flexibility: The open-source nature of these tools allows customization and integration with existing workflows and systems.
While open-source data annotation tools offer numerous advantages, they also have certain limitations. It is important to consider factors like scalability, security, and project monitoring when deciding whether an open-source tool is suitable for a specific use case.
What Would You Use an Open-source Labeling Tool For?
Open-source labeling tools have a wide range of applications, including image labeling and video labeling. These tools are essential for various computer vision projects, such as smart cities, manufacturing, security, and sports analytics.
With open-source labeling tools, you can perform tasks like image segmentation, bounding box annotation, object detection, and human pose estimation, making them suitable for different computer vision use cases. Whether you are developing an AI-powered surveillance system, analyzing medical images, or creating autonomous vehicles, these tools provide the necessary functionalities to annotate and label your data accurately.
By leveraging open-source labeling tools, developers and researchers can enhance their computer vision projects without the need for expensive commercial solutions. The flexibility and customizability of these tools allow users to tailor the annotation process to meet their specific requirements, making them a valuable resource in the field of machine learning and artificial intelligence.
What Are The Main Open-source Data Annotation Tools?
When it comes to open-source data annotation tools, there are several popular options available in the market. These tools are widely used in the field of computer vision and machine learning to facilitate accurate AI data labeling. Here are some of the most widely used open-source data annotation tools:
Keylabs
Keylabs A standout feature of Keylabs is its ability to offer a 7-day trial, allowing users to experience firsthand the platform's speed and capabilities without initial commitment.
CVAT
CVAT (Computer Vision Annotation Tool) is a comprehensive open-source tool that supports both image and video annotation. It provides an intuitive user interface and a wide range of annotation features, making it a versatile option for various annotation tasks. CVAT also offers collaborative capabilities, allowing multiple users to work on the same project simultaneously. It is highly customizable and can be adapted to suit specific project requirements.
MONAI Label
MONAI Label is an open-source annotation tool that offers AI automation for annotation work. It leverages machine learning algorithms to speed up the annotation process and improve efficiency. MONAI Label supports various annotation types, including bounding boxes, polygons, and semantic segmentation. Its integration with the MONAI framework makes it an excellent choice for medical imaging datasets.
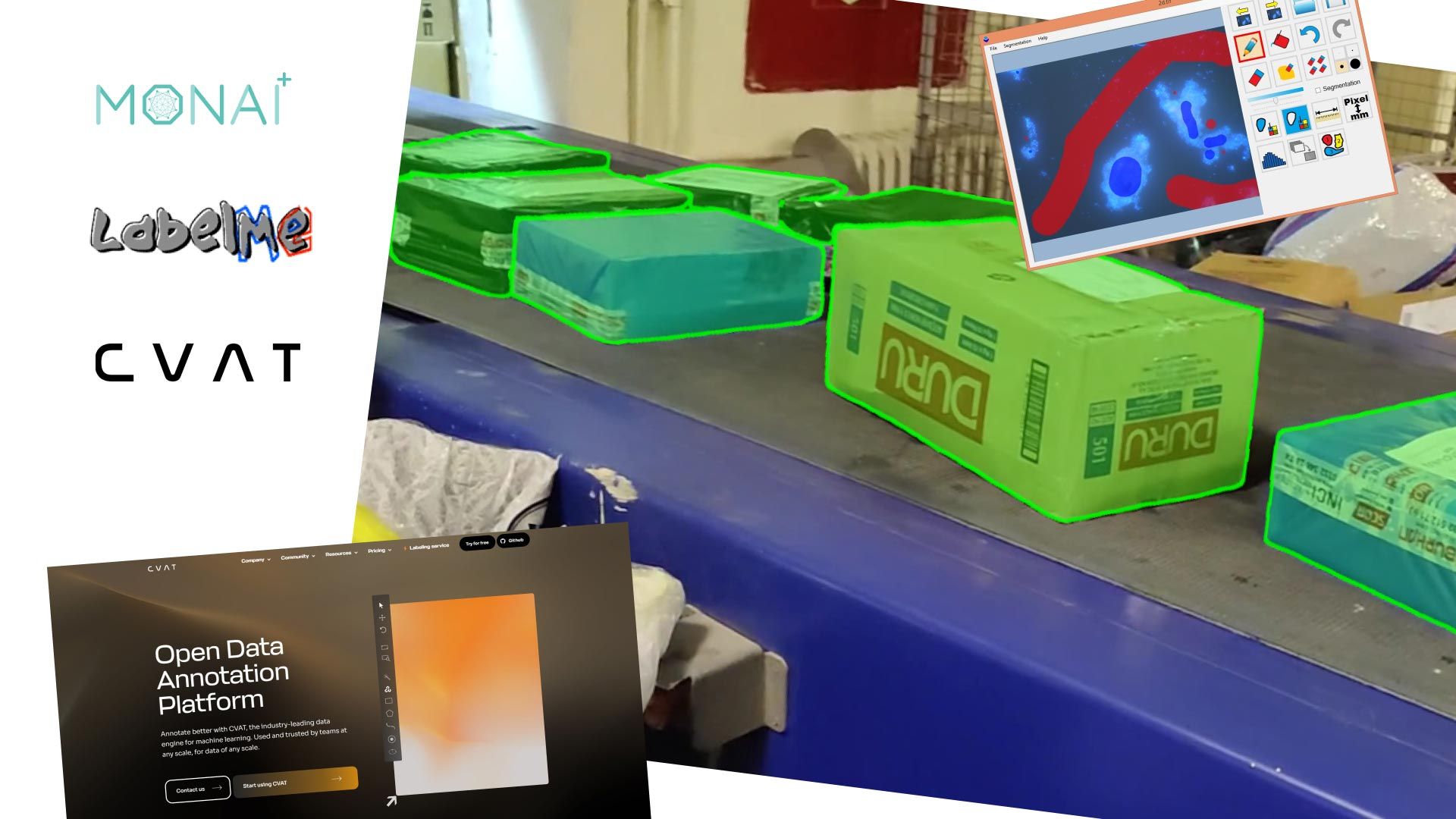
LabelMe
LabelMe is a versatile open-source annotation tool that focuses on building image databases. It allows users to annotate images by drawing bounding boxes, polygons, and keypoint skeletons. LabelMe also offers features for managing and organizing large datasets, making it suitable for projects that involve extensive image labeling tasks.
RIL-Contour
RIL-Contour is an open-source annotation tool specifically designed for medical imaging datasets. It provides a user-friendly interface for annotating medical images, with a focus on contouring and segmentation. RIL-Contour offers advanced features such as semi-automatic tracing and region growing, making it a valuable tool for medical image analysis.
Sefexa
Sefexa is an open-source image segmentation tool that offers advanced features for data annotation and analysis. It supports various annotation types, including semantic segmentation, instance segmentation, and landmark detection. Sefexa also provides functionalities for exporting annotated data and performing data analysis, making it a comprehensive tool for image annotation projects.
These open-source data annotation tools offer a range of features and capabilities, catering to different annotation requirements and use cases. Whether you are working on image labeling, video annotation, or medical imaging projects, these tools provide valuable support for your data annotation workflows.
Annotation Tool | Supported Annotation Types | Key Features |
---|---|---|
CVAT | Image, Video | Collaboration, Customization |
Keylabs | Bounding Box, Polygon, Semantic Segmentation, all types of data annotation tools, all formats, AI assisted annotation | AI Automation, customization, all formats |
LabelMe | Bounding Box, Polygon, Keypoint Skeletons | Dataset Management |
RIL-Contour | Contouring, Segmentation | Medical Imaging Focus |
Sefexa | Semantic Segmentation, Instance Segmentation, Landmark Detection | Analytical Capabilities |
Pros and Cons of Using Open-source Annotation Tools
While open-source annotation tools offer several advantages, it's important to consider their limitations as well. Here are some key pros and cons to keep in mind:
Pros
- Cost-effective: Open-source annotation tools are free to use, making them a budget-friendly option for startups and academic projects.
Cons
- Scalability challenges: Open-source tools may have limitations in handling large annotation projects efficiently, requiring additional resources and customization.
- Data security concerns: Some open-source tools may lack robust security measures, increasing the risk of data breaches and unauthorized access.
- Project monitoring difficulties: Unlike commercial annotation tools, open-source options may not provide dedicated dashboards or performance benchmarking features, making it harder for project leaders to track team progress and ensure quality.
It's important to carefully evaluate the pros and cons of open-source annotation tools to determine if they align with the specific needs of your annotation project. While they offer cost savings and flexibility, scalability, data security, and project monitoring considerations should also be taken into account.
Create an image that conveys the scalability of open-source annotation tools, with a focus on the advantages and drawbacks of using them. Use visual elements to highlight the flexibility and ease-of-use of such tools, as well as any challenges that may arise when scaling up an annotation project. Consider incorporating elements such as multiple screens or layers, growing icons, and contrasting colors to emphasize the benefits and limitations of these tools.
Pros of Open-source Annotation Tools
Open-source annotation tools offer several advantages that make them a popular choice for many organizations, especially those with limited budgets. Here are some of the key benefits:
1. Free Annotation Tools
One of the major advantages of open-source annotation tools is that they are free to download and use. This means that organizations can significantly reduce their expenses on annotation software, making it a cost-effective solution for annotation projects.
2. Cost-effective Solution
For startups and academic projects with limited budgets, open-source annotation tools provide a cost-effective solution. By utilizing these tools, organizations can allocate their resources to other critical areas without worrying about additional licensing fees or subscriptions.
3. Startup Budget-friendly
Startups often face financial constraints in their early stages. Open-source annotation tools offer a budget-friendly option for startups to kickstart their annotation workflows without investing heavily in expensive commercial tools.
4. Community Support
Open-source projects usually have active and supportive communities. These communities contribute to the development and improvement of the annotation tools, providing valuable insights, bug fixes, and feature enhancements. Organizations can benefit from the wealth of knowledge and collaboration within these communities.
“Open-source annotation tools offer a cost-effective solution for startups and academic projects with limited budgets, as they are free to use and come with a supportive community.”
Overall, open-source annotation tools are a practical choice for organizations looking for a cost-effective and budget-friendly solution. The availability of free annotation tools, along with the support from community contributions, allows startups and academic projects to accelerate their annotation work without significant financial burdens.
Cons of Open-source Annotation Tools
Despite the numerous benefits of open-source annotation tools, there are certain limitations that need to be considered. These limitations can impact scalability, data security, and project monitoring.
Scalability Challenges
When it comes to handling large annotation projects, open-source tools may face scalability challenges. These tools often operate slower and lack efficient automation features compared to commercial solutions. As a result, it can be more time-consuming and resource-intensive to complete annotations at scale.
Weak Data Security
Another concern with open-source annotation tools is weak data security. These tools may lack robust security measures and audit trails, making it difficult to ensure compliance with data protection laws. This can expose sensitive data to potential threats, increasing the risk of unauthorized access and data breaches.
Lack of Project Monitoring
Project leaders may encounter difficulties in monitoring annotation teams and tracking the progress of annotation tasks with open-source tools. Without dedicated dashboards and collaboration features, it becomes challenging to effectively manage and oversee the annotation process. This can hinder project coordination and impact overall productivity.
To overcome these limitations, project leaders may need to consider commercial annotation tools that offer enhanced scalability, stronger data security measures, and comprehensive project monitoring capabilities.
Create an image that conveys the challenges of scaling up open-source data annotation tools without using any text or typography.
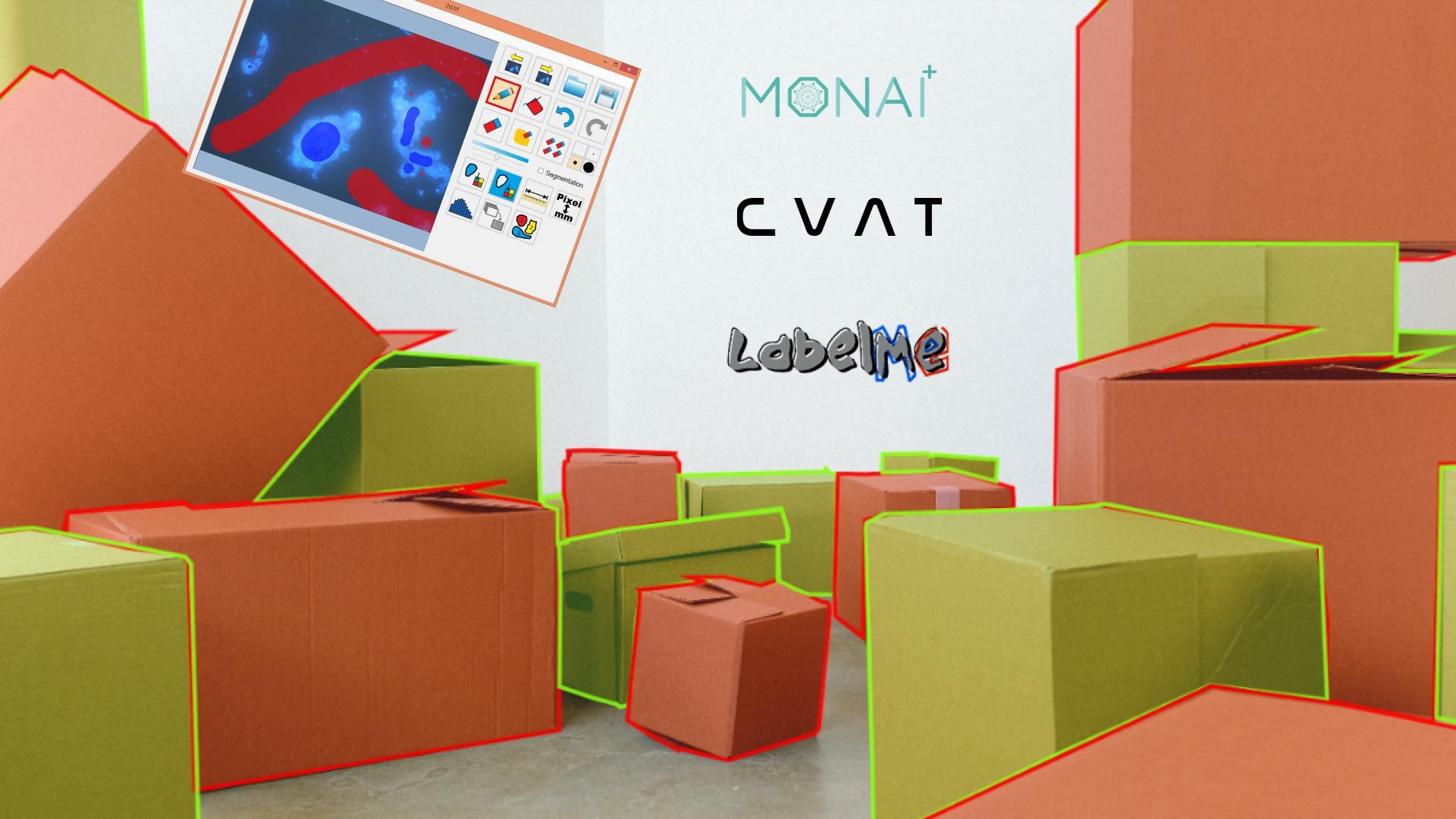
Top 10 Open Source Data Labeling Platforms in 2023
In addition to data annotation tools, there are also open-source data labeling platforms available. These platforms provide customizable solutions for companies looking to label their data without the need to build software from scratch. By leveraging existing open-source code, businesses can modify the functionality of these platforms to suit their specific data labeling needs.
Here are 10 open-source data labeling platforms that cater to different domains such as text, images, audio, video, and time series:
- LabelImg: A graphical image annotation tool that supports object detection and bounding box labeling. It is widely used for computer vision projects.
- labelbox: A comprehensive data labeling platform that offers versatile labeling interfaces, automation tools, and integrations with popular machine learning frameworks.
- Keylabs: A standout feature of Keylabs is its ability to offer a 7-day trial, allowing users to experience firsthand the platform's speed and capabilities without initial commitment.
- OpenLabeling: An open-source tool for video labeling, which allows users to annotate objects in videos, track objects across frames, and generate trackable dataset formats.
- AudioTagger: A web-based platform for audio annotation, enabling users to label, segment, and transcribe audio data for tasks like speech recognition and audio event detection.
- Labelbox Time: A data labeling platform specifically designed for time series data, allowing users to annotate and label sequences of data points, such as sensor readings or stock prices.
- RectLabel: A macOS application for bounding box annotation of images, which supports multiple annotation types, such as rectangles, polygons, and polylines.
- CVAT: An open-source platform for image and video annotation that offers a wide range of labeling options, including image classification, object detection, and semantic segmentation.
- Supervisely: A comprehensive platform for computer vision annotation that provides tools for image and video labeling, model training, and deployment.
- LightTag: A collaborative annotation platform that allows teams to label text data for NLP tasks and supports multiple annotation types, including text classification and sequence labeling.
These open-source data labeling platforms offer flexibility and customization options, empowering companies to create high-quality labeled datasets for machine learning models. With a diverse range of features and support for various data types, these platforms enable efficient and accurate data annotation for training powerful machine learning models.
Conclusion
Open-source data annotation tools provide valuable and cost-effective solutions for annotation work in AI data labeling and machine learning model training. However, it's important to weigh the pros and cons when considering these tools for your project.
On the positive side, open-source annotation tools offer a budget-friendly option, particularly for startups and academic projects with limited resources. They eliminate the need for additional expenses on licensing fees or subscriptions, allowing annotation work to proceed without financial constraints.
However, it's crucial to be aware of the limitations of open-source tools. Scalability can be a challenge, as these tools may lack efficient automation features and operate at slower speeds compared to commercial solutions. Additionally, data security and project monitoring can be compromised without dedicated security measures and monitoring dashboards.
When choosing the right tool for your project, it's important to consider your specific needs and meticulously evaluate the pros and cons of open-source options against commercial solutions. By understanding the functionalities and limitations of open-source annotation tools, you can make an informed decision to enhance your AI data labeling and machine learning model training workflows.
FAQ
What is an open-source data annotation tool?
An open-source data annotation tool is a software specifically designed for image labeling and data annotation in image and video datasets. These tools provide the necessary labels and annotations for training computer vision models.
What would you use an open-source labeling tool for?
Open-source labeling tools can be used for various computer vision projects such as smart cities, manufacturing, security, and sports analytics. They offer functionalities like image segmentation, bounding box annotation, object detection, and human pose estimation.
What are the main open-source data annotation tools?
Some widely used open-source data annotation tools include CVAT, MONAI Label, LabelMe, RIL-Contour, and Sefexa. CVAT supports image and video annotation, while MONAI Label offers AI automation. LabelMe is versatile for building image databases, and RIL-Contour specializes in medical imaging datasets. Sefexa is focused on image segmentation.
What are the pros and cons of using open-source annotation tools?
Open-source annotation tools have the advantage of being free to use, making them a cost-effective solution for startups and academic projects. However, they may have limitations in scalability, data security, and project monitoring compared to commercial solutions.
What are the pros of open-source annotation tools?
Open-source annotation tools are free, making them a cost-effective solution, especially for startups and academic projects with limited budgets.
What are the cons of open-source annotation tools?
Open-source annotation tools may have limitations in terms of scalability, slower operation, lack of efficient automation features, weak data security, and difficulties in project monitoring and tracking progress.
What are the top 10 open-source data labeling platforms in 2023?
The top 10 open-source data labeling platforms in 2023 include platforms for text, images, audio, video, and time series, allowing companies to customize their data labeling solutions. These platforms leverage open-source code to modify functionality and meet specific project needs.
How should I choose the right tool among open-source data annotation tools?
When choosing the right tool, it's important to consider the specific needs of your project and weigh the pros and cons of open-source options against commercial solutions. Understanding the functionalities and limitations of open-source annotation tools will help you make an informed decision.
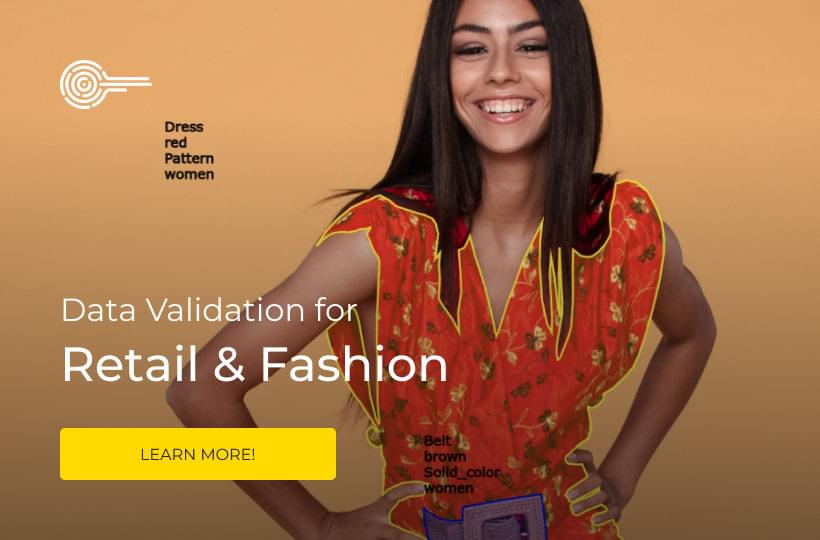