Enhancing Machine Learning Accuracy with Data Labeling
Data labeling is a crucial step in improving the accuracy and performance of machine learning models. By annotating datasets with relevant information, such as object boundaries and text categorization, data labeling helps machines learn and make informed decisions. High-quality data labeling is essential for applications like computer vision, natural language processing, and autonomous vehicles.

Data labeling companies offer specialized services in image annotation, text annotation, video annotation, and audio annotation to ensure accurate and reliable labeled datasets. These services provide crucial details and context that enable ML models to recognize patterns and make accurate predictions. Quality assurance measures are implemented to enhance the overall accuracy of labeled data, ensuring that the models receive the necessary context to learn from.
Outsourcing data labeling to experienced professionals brings significant advantages, including improved data quality, cost efficiency, faster model development, scalability, and domain-specific knowledge. Choosing the right data labeling partner is crucial, considering expertise, data security, turnaround time, cost-effectiveness, references, and communication and support.
Key Takeaways:
- Data labeling is vital in enhancing the accuracy and performance of machine learning models.
- Data labeling companies offer specialized services in image annotation, text annotation, video annotation, and audio annotation.
- Outsourcing data labeling offers advantages such as improved data quality, cost efficiency, and faster model development.
- Choosing the right data labeling partner requires considering expertise, data security, turnaround time, and communication and support.
The Importance of Accurate Data Labeling for ML Models
Accurate data labeling is a crucial factor in determining the performance of machine learning (ML) models. A small deviation or inaccuracy in the labeled data can have significant consequences, leading to suboptimal model performance and potentially adverse outcomes, particularly in critical domains.
When data is labeled accurately, it establishes a reliable reference point for ML models, enabling them to learn from the labeled datasets effectively. This alignment between the labeled data and the true attributes of the data ensures that the models make accurate predictions.
Precision in data labeling holds immense importance across various applications. In computer vision, for example, accurately labeled images enable models to detect objects, recognize patterns, and make informed decisions. Similarly, in natural language processing, precise labeling of text helps models understand language semantics, sentiments, and categorizations. The accuracy of data labeling is equally vital in domains such as autonomous vehicles, healthcare, and many others.
"An accurate labeling process not only equips ML models with reliable information but also ensures that these models perform optimally in critical domains."
The consequences of suboptimal data labeling can be far-reaching. ML models heavily rely on accurately labeled data to learn and generalize patterns. Inaccurate labeling can introduce biases and distortions, leading to a compromised model's ability to handle real-world scenarios. In domains like healthcare, where even minor inaccuracies can impact patient outcomes, the importance of accurate data labeling cannot be overstated.
To illustrate the significance of accurate data labeling, consider the potential consequences in the context of autonomous vehicles. Inaccurate labeling of objects, such as misidentifying pedestrians as lampposts, can have severe safety implications. It highlights the critical role accurate data labeling plays in ensuring the reliability and performance of ML models in safety-critical applications.
It is evident that the precision in data labeling is a fundamental pillar for ML models' success, enabling them to operate effectively and deliver reliable results in various domains.
Suboptimal Model Performance and the Need for Precision
Suboptimal model performance resulting from inaccurate data labeling can manifest in various ways:
- Poor prediction accuracy
- Inconsistent model behavior
- Increased false positives/negatives
- Unreliable decision-making
- Failure to generalize expected outcomes
Inaccurate data labeling introduces noise and inconsistencies into the training process, affecting the model's ability to learn and make accurate predictions. Precision in data labeling ensures that the ML models can capture the true patterns and attributes of the data, leading to improved performance and more reliable outcomes.
Key Services Offered by Data Labeling Companies
Data labeling companies provide a comprehensive range of services tailored to meet the specific requirements of various applications. These services ensure accurate and reliable labeled datasets, enabling machine learning models to learn and make informed decisions. The key services offered by data labeling companies include:
- Image Annotation:
Image annotation is a fundamental task in computer vision, involving the labeling of images with object boundaries, classifications, and segmentation masks. This process provides crucial details required for training computer vision models.
- Text Annotation:
Text annotation involves tasks such as named entity recognition, sentiment analysis, and text categorization. These annotations are crucial for natural language processing models to understand and interpret textual data accurately.
- Video Annotation:
Video annotation focuses on annotating individual frames of videos to provide valuable information for training models. This service is particularly useful in surveillance systems, autonomous vehicles, and other applications that rely on video analysis.
- Audio Annotation:
Audio annotation involves the transcription and labeling of speech segments for speech recognition and audio processing models. Accurate audio annotations contribute to the development of robust voice-based applications.
- Quality Control and Validation:
Data labeling companies prioritize quality control and validation processes to ensure the accuracy and reliability of labeled datasets. These measures involve thorough checks to identify and rectify any errors or inconsistencies in the annotations.
- Security and Privacy:
Data labeling companies implement robust security and privacy protocols to safeguard sensitive information contained within the labeled datasets. These measures ensure data protection and confidentiality.
These services offered by data labeling companies play a vital role in enhancing the performance and accuracy of machine learning models across diverse applications. By leveraging expert annotation techniques, these companies enable businesses to develop intelligent solutions and drive innovation.
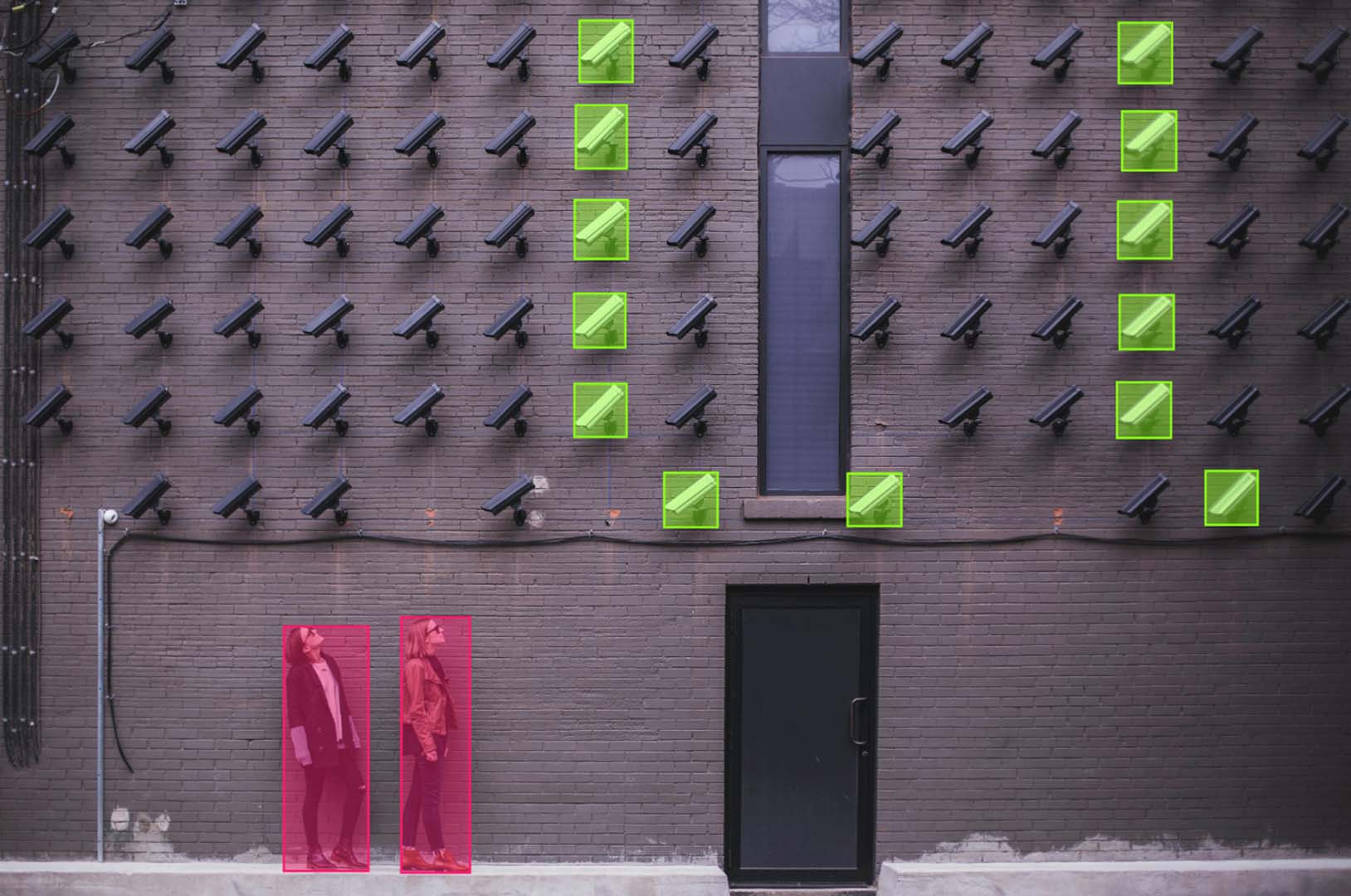
Advantages of Data Labeling in AI/ML Model Training
Data labeling plays a pivotal role in the training of AI/ML models, offering numerous advantages that contribute to their accuracy and effectiveness. By providing a ground truth for models to learn from, data labeling establishes a reliable reference point for making accurate predictions. This ensures that the models are equipped with the necessary information to recognize patterns and make informed decisions.
One of the key advantages of data labeling is iterative learning. ML models have the ability to learn from their past mistakes and continuously improve their accuracy and reliability over time. The feedback loop created through iterative learning enables models to refine their predictions, resulting in enhanced performance and better outcomes.
Data labeling also helps in reducing bias in AI/ML models. Through proper labeling, models can be trained on diverse and representative datasets, ensuring that biases are minimized or eliminated. This promotes fairness and transparency in decision-making processes, making the models more reliable in real-world applications.
Furthermore, accurate data labeling addresses the challenge of low-quality data. Inaccurate or low-quality data can have a detrimental impact on model learning and performance. By filling gaps in training data and providing correctly labeled examples, data labeling improves the model's ability to generalize and make accurate predictions on unseen data.
Automated labeling with a human-in-the-loop approach offers scalability without compromising label accuracy. While automated processes can provide initial labels for large datasets, human supervisors play a critical role in ensuring the quality and precision of the labeled data. They can review and correct automated labels, ensuring that the final dataset is reliable and accurate.
Overall, proper data labeling is essential for enhancing the accuracy and utility of AI/ML models. It provides the necessary foundation for model training, enabling models to learn from ground truth data, reduce bias, and improve their generalization capabilities. By harnessing the power of data labeling, organizations can develop robust and effective AI/ML models that deliver accurate predictions in a wide range of real-world scenarios.
Conclusion
Data labeling plays a critical role in enhancing the accuracy of machine learning models. It provides the necessary context for ML models to recognize patterns and make informed decisions, making it essential for various real-world applications in industries such as healthcare, automotive, and online advertising. However, manual data labeling can be time-consuming, costly, and prone to errors and bias.
Outsourcing data labeling to experienced professionals offers numerous advantages. Firstly, it ensures enhanced data quality, as experts with domain expertise can accurately and contextually label the data. Additionally, outsourcing data labeling provides cost efficiency, faster model development, and scalability. By partnering with the right data labeling provider, organizations can benefit from their domain-specific knowledge and expertise.
When choosing a data labeling partner, several considerations should be taken into account. These include expertise in the relevant domain, data security protocols, turnaround time, cost-effectiveness, references, and communication and support. By outsourcing data labeling to a trusted partner, organizations can strengthen their AI/ML models, ultimately improving their performance in real-world applications.
FAQ
What is data labeling and why is it important for machine learning accuracy?
Data labeling involves annotating datasets with relevant information to help machines learn and make informed decisions. It plays a crucial role in improving the accuracy of machine learning models by providing a reliable reference point for model training.
How does inaccurate data labeling impact the performance of ML models?
Anomalies or inaccuracies in labeled data can lead to suboptimal model performance and potentially harmful consequences in critical domains. Accurate data labeling is essential to ensure that predictions align with the actual attributes of the data and improve overall model performance.
What are the key services offered by data labeling companies?
Data labeling companies offer specialized services such as image annotation, text annotation, video annotation, and audio annotation. These services involve tasks like labeling object boundaries, classifications, segmentation masks, named entity recognition, sentiment analysis, video frames, speech transcription, and more.
What are the advantages of data labeling in AI/ML model training?
Data labeling provides a ground truth for ML models to learn from, enabling iterative learning, reducing bias, improving model accuracy, and enhancing model generalization. Automated labeling with human-in-the-loop allows for scalability without compromising label accuracy, and proper data labeling helps fill gaps in training data.
Why should organizations consider outsourcing data labeling?
Outsourcing data labeling to experienced professionals offers advantages such as enhanced data quality, cost efficiency, faster model development, scalability, and domain-specific knowledge. It helps overcome the challenges of manual data labeling, ensures accurate and contextually relevant labeling, and improves the accuracy of AI/ML models.
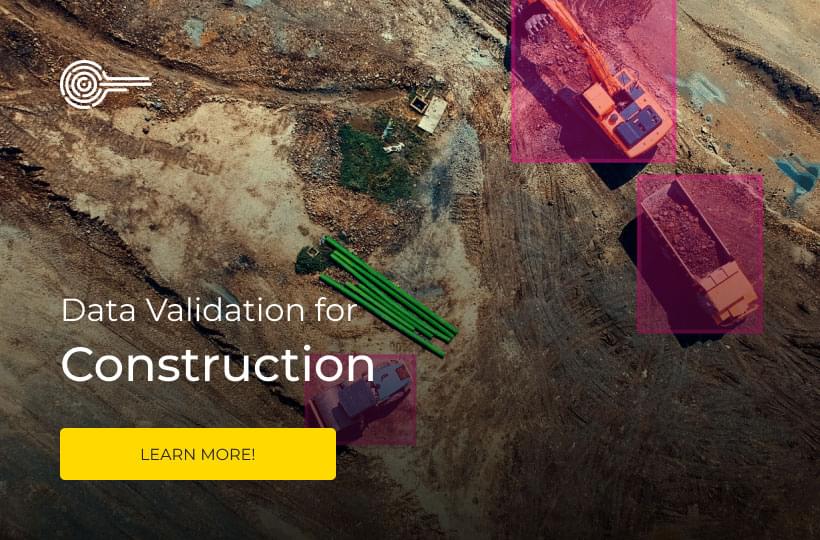