Emerging Applications for AI in Microbiology and Chemistry
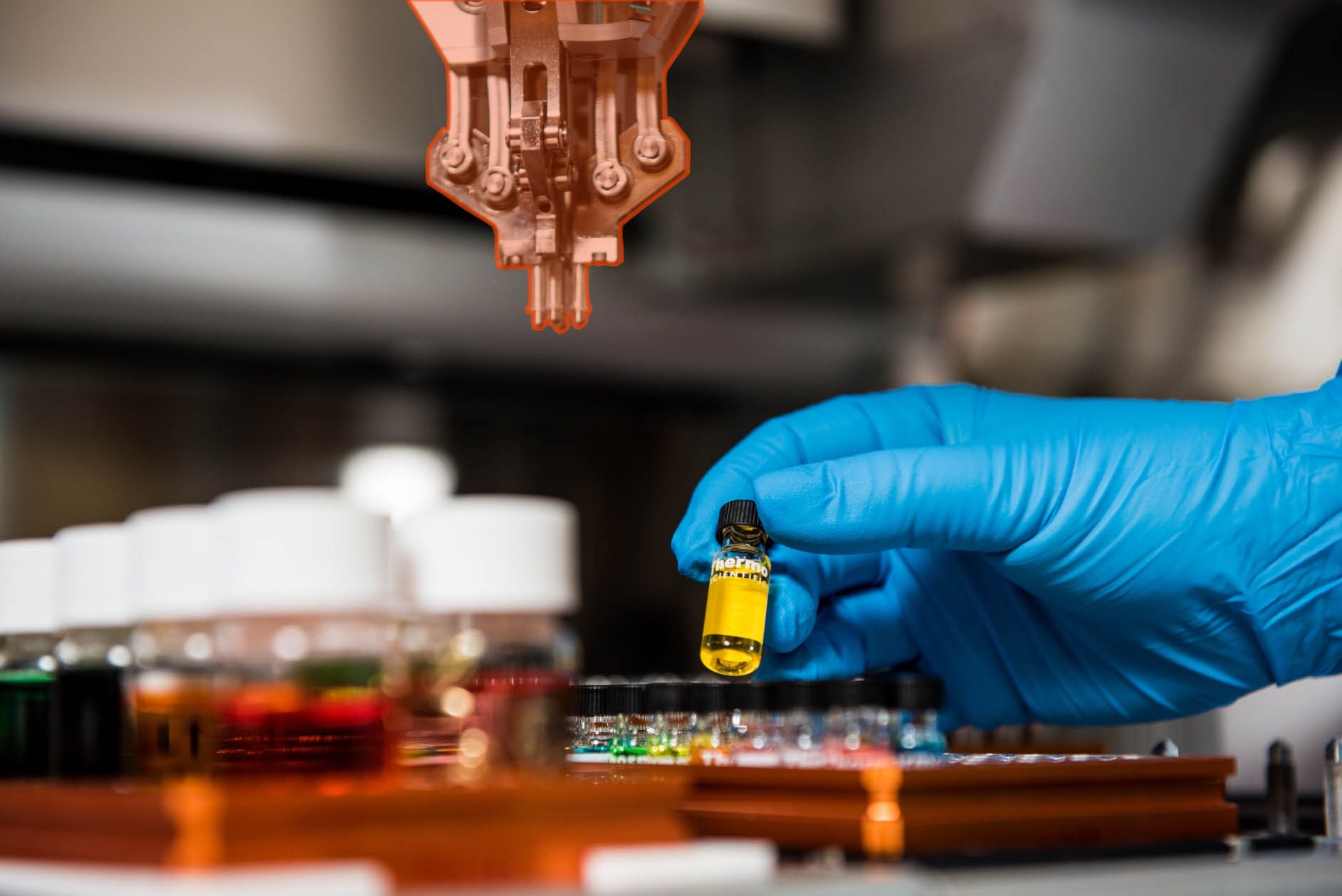
Microbiology and chemistry researchers are beginning to examine the potential of computer vision based AI technology in their fields. Machine learning techniques could open up an exciting range of possibilities, from investigating novel chemical compounds to quickly identifying cells and microorganisms.
Much of this technology is in its infancy and needs support from investors and institutions. It also requires increasing access to high quality, annotated training images. To achieve consistent functionality AI models need images of cells or lab equipment that contain additional information in the form of labels.
The progress of machine learning in this industry will rely heavily on the scaling up of training data to fit evolving needs.
This blog will look at two of the most promising applications for AI in the lab. We will also show how the development of these important use cases can be accelerated with the help of professional annotation providers, like Keymakr.
Cell biology
Computer imagining is central to many practices in cell biology. Computers can measure different parameters and help visualize the microscopic world of the cell. However, the task of interpretation is left to scientists who ultimately decide what is important to focus on, or noteworthy, within a given cell.
Cell biologists often need to laboriously search through images of cells to find the information that they need, for example the presence of a mutated genome or a cell undergoing mitosis. Recent developments in cellular imaging have exponentially increased the number and detail of images that are in need of interpretation, creating a potential workload blockage in many labs.
Automation of this investigative process could significantly improve the speed of research in a variety of fields. Recent models have shown that computer vision systems have the ability to recognize and label a wide range of organelles (subcellular structures such as nuclei) at a faster rate than human biologists.
This technology has the potential to transform our understanding of the inner workings of cells, and provide researchers with novel insights.
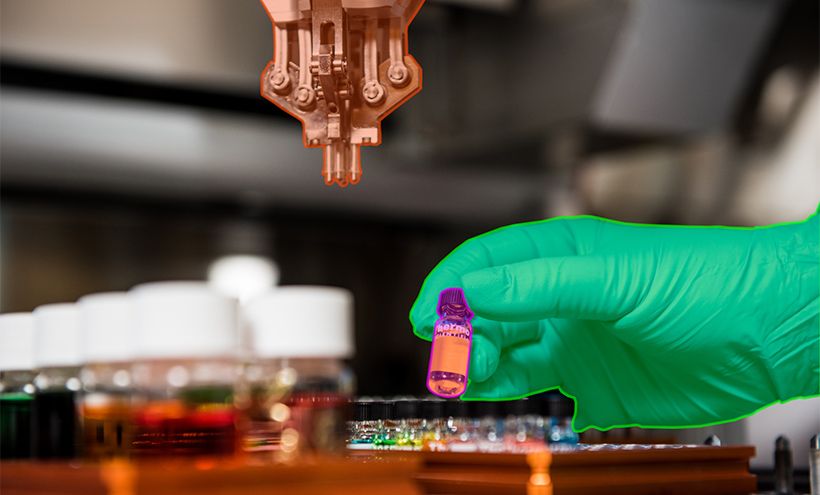
Chemistry lab settings
In order to create new compounds researchers combine and experiment with different chemicals with the aim of developing new, and useful, molecular structures. Developments in deep learning algorithms have led to the creation of artificial neural networks that can predict chemical interactions and help scientists.
This technology is being integrated with computer vision powered robotics to create automated labs that can be supervised remotely by researchers, allowing for far greater flexibility and remote working. AI models can be trained to recognise the fluid contents of transparent containers allowing them to perform essential research activities.
Robots powered by these systems can also tell what material phase (liquid, solid, foam, suspension, powder, etc) the substances they are “handling” are. The end result of this integrated and automated lab environment could be an acceleration of chemical research which could benefit us all.
Finding the right image data
For computer vision applications to continue to thrive in these important research fields it is essential that developers have access to annotated training images. Annotating images of cells requires expertise and conscientious verification practices.
Annotation service providers are often best placed to provide data of this kind. This is due to their experience working with experts to annotate specialist data. For many AI companies assembling an image annotation team can be a distraction from their core research goals.
Outsourcing to a responsible and experienced provider, like Keymakr, can relieve this management burden whilst maintaining data quality.
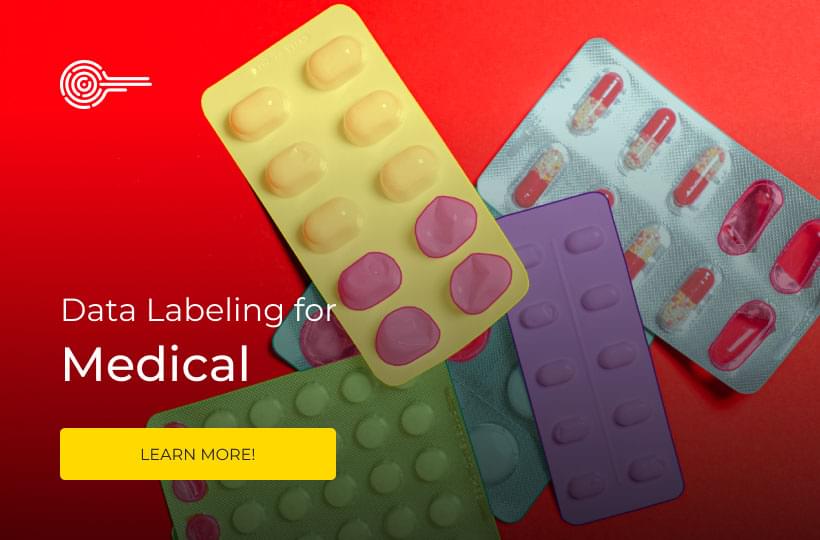