Data Labeling in Healthcare: Applications and Impact
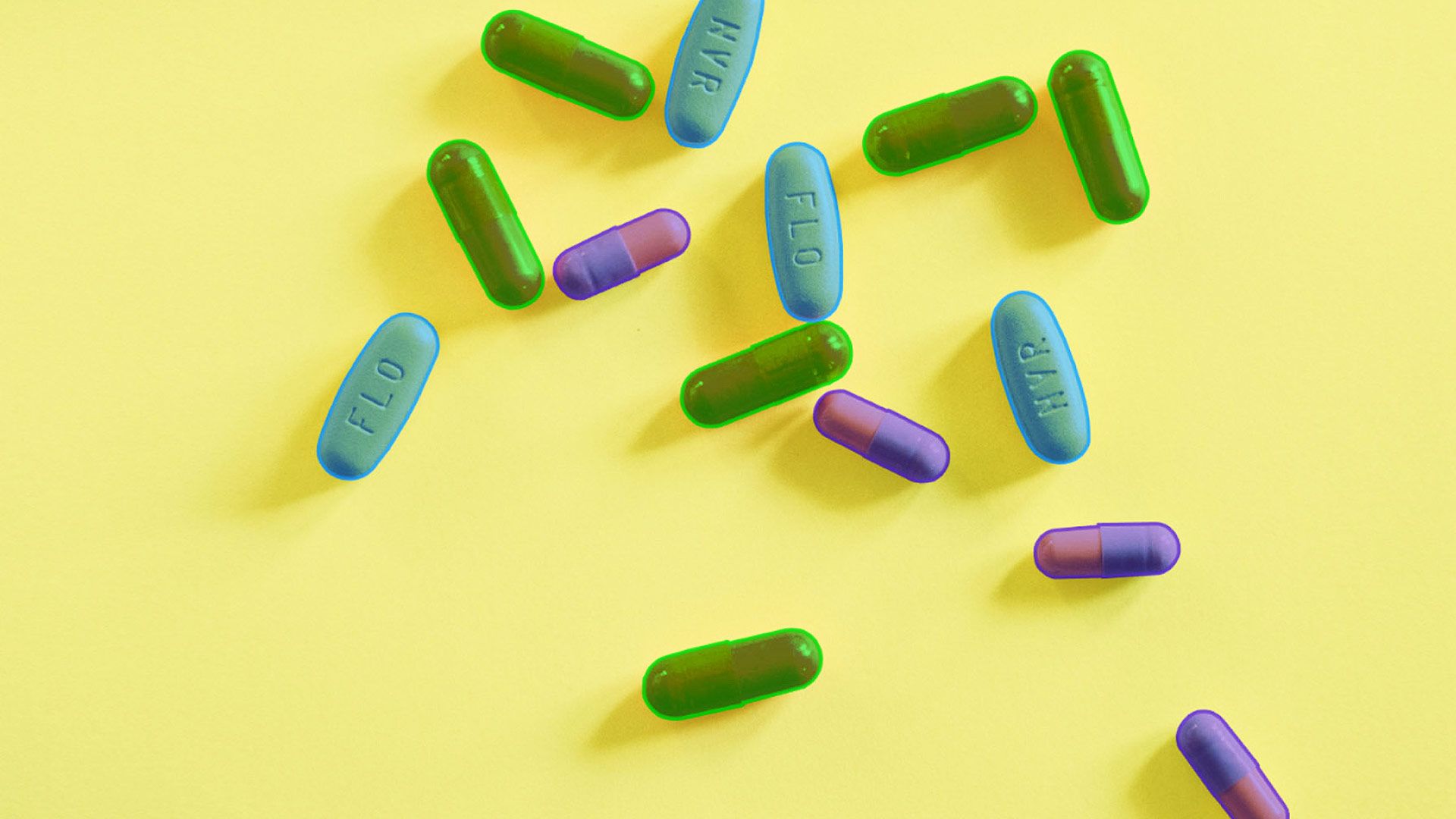
Healthcare data collection and labeling play a crucial role in improving the quality of healthcare services and driving innovation in the industry. Data labeling involves the process of categorizing and annotating healthcare data to make it more meaningful and useful for various applications. This article explores the applications and impact of data labeling in healthcare, highlighting the importance of accurate labeling in facilitating clinical research, personalized medicine, and decision support systems.
Key Takeaways:
- Data labeling in healthcare involves categorizing and annotating healthcare data to make it more meaningful and useful.
- Labeled healthcare data is used in various applications such as clinical research, predictive analytics, and personalized medicine.
- Accurate medical data labeling is essential for the development of healthcare applications, clinical decision support systems, and medical research.
- The healthcare data collection and labeling market is experiencing significant growth due to the increasing demand for healthcare analytics and AI applications in healthcare.
- Data labeling is a prerequisite for the successful deployment of AI/ML models in healthcare, enabling early disease detection and augmented clinical decision-making.

What is Medical Data Labeling?
Medical data labeling is a specialized form of data labeling that focuses specifically on healthcare-related information. It involves various tasks such as medical coding, clinical entity recognition, image annotation, EHR annotation, and sentiment analysis. Through accurate medical data labeling, healthcare professionals can extract valuable insights from vast amounts of data, enabling the development of healthcare applications, clinical decision support systems, medical research, and population health management.
Tasks in Medical Data Labeling:
- Medical coding: Assigning standardized codes to medical procedures and diagnoses for accurate documentation and billing purposes.
- Clinical entity recognition: Identifying and extracting specific clinical entities from medical texts, such as symptoms, diseases, medications, and procedures.
- Image annotation: Annotating medical images with markers and labels to highlight specific regions or features of interest for diagnostic and research purposes.
- EHR annotation: Annotating electronic health records (EHRs) with structured information, allowing for efficient data management and analysis.
- Sentiment analysis: Analyzing text data to determine the sentiment or emotions expressed, helping gauge patient satisfaction, and identify areas for improvement.
Accurate medical data labeling is essential for ensuring the integrity and reliability of healthcare data. It forms the foundation for developing robust healthcare applications that can improve patient care, optimize treatment plans, enhance clinical decision-making, and advance medical research. Furthermore, labeled medical data facilitates interoperability and data exchange between healthcare systems, leading to improved patient safety, quality of care, and overall healthcare outcomes.
Example Table - Comparison of Medical Data Labeling Tasks:
Task | Description | Use Cases |
---|---|---|
Medical Coding | Assigning standardized codes to medical procedures and diagnoses | Easier billing and reimbursement processes, accurate population health analysis |
Clinical Entity Recognition | Identifying and extracting clinical entities from medical texts | Disease surveillance, pharmacovigilance, clinical research |
Image Annotation | Annotating medical images with markers and labels | Computer-aided diagnosis, medical image analysis, telemedicine |
EHR Annotation | Annotating electronic health records with structured information | Supporting clinical decision-making, interoperability between healthcare systems |
Sentiment Analysis | Analyzing text to determine sentiment or emotions expressed | Patient feedback analysis, improving patient experience |
Medical data labeling plays a critical role in harnessing the potential of healthcare data and enabling the development of innovative solutions that drive advancements in patient care and medical research.
Why Label Medical Data?
Labeling medical data plays a critical role in various healthcare applications and processes. By categorizing and annotating healthcare data, it becomes more meaningful, allowing for improved analysis, research, and decision-making. Let's explore the key reasons why labeling medical data is essential:
Training Machine Learning Models
Accurate labeling of medical data is vital for training machine learning models in healthcare. Labeled datasets serve as the foundation for developing AI algorithms and models that can assist in diagnosing diseases, predicting outcomes, and providing personalized treatment plans.
Enhancing Data Analysis
Labeling medical data enhances data analysis capabilities by providing precise and structured information. With labeled datasets, healthcare professionals and researchers can uncover valuable insights, patterns, and trends that can greatly contribute to medical research, population health management, and disease surveillance.
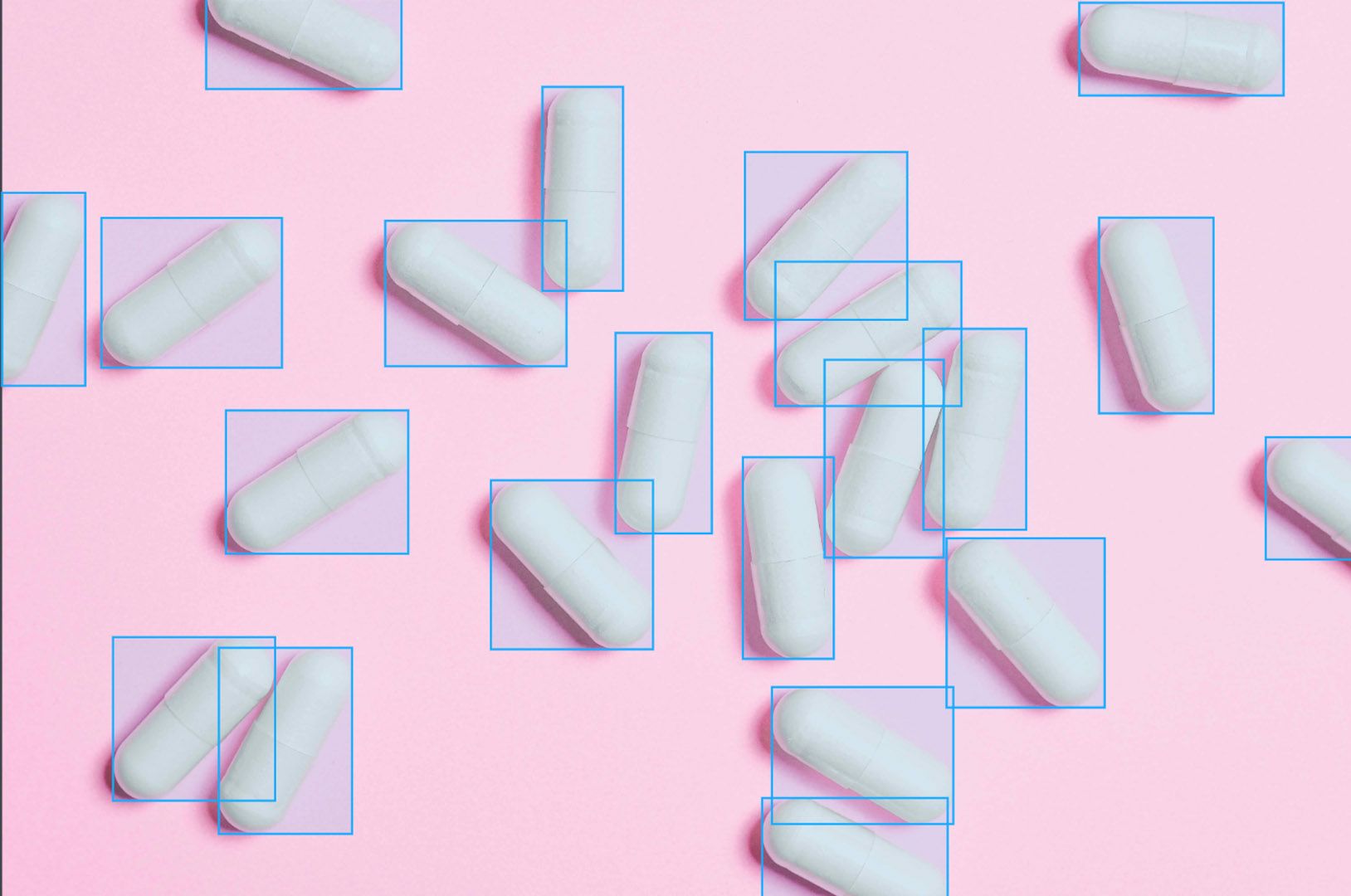
Enabling Clinical Research
Labeled medical data enables clinical research by providing a reliable source of information for scientists, researchers, and healthcare professionals. Research studies heavily rely on accurately labeled data to draw meaningful conclusions, develop new treatment approaches, and advance medical knowledge.
Supporting Clinical Decision-Making
"Accurate labeling of medical data is imperative for supporting clinical decision-making. By providing labeled data, clinicians can have access to comprehensive information that aids in accurate diagnosis, treatment planning, and monitoring of patient progress."
Labeling medical data ensures interoperability and seamless data exchange among different healthcare systems and entities. Consistent and well-labeled data enable smooth collaboration between healthcare providers, improving care coordination, and streamlining processes.
Ensuring Patient Safety
By accurately labeling medical data, healthcare organizations can prioritize patient safety. Labeled data supports the identification of potential risks, medication errors, and adverse events, enabling proactive measures to ensure patient well-being and avoid clinical mishaps.
Compliance with Regulations
Labeling medical data is necessary to comply with regulations and standards in the healthcare industry. Labeled datasets help organizations adhere to privacy rules, data protection laws, and ethical guidelines, ensuring the security and confidentiality of patient information.
Overall, the process of labeling medical data is crucial for the development and implementation of AI-driven solutions in healthcare. It empowers machine learning models, enhances data analysis, supports clinical research and decision-making, facilitates interoperability, ensures patient safety, and enables compliance with regulations.
Benefits of Labeling Medical Data |
---|
Training machine learning models |
Enhancing data analysis |
Enabling clinical research |
Supporting clinical decision-making |
Facilitating interoperability |
Ensuring patient safety |
Compliance with regulations |
Healthcare Data Collection and Labeling Market – Trends and Opportunities
The healthcare data collection and labeling market is currently undergoing steady growth, driven by various trends and opportunities in the healthcare industry. Factors such as the increasing demand for healthcare analytics, the widespread adoption of electronic health records (EHRs), and the integration of artificial intelligence (AI) and machine learning (ML) applications in healthcare are contributing to this market expansion.
The rise of telemedicine and remote monitoring technologies has also played a significant role in fueling the growth of this market. These advancements have revolutionized the way healthcare services are delivered, enabling remote consultations, continuous patient monitoring, and the collection of real-time health data. As a result, there is a growing need for accurate data collection and labeling to ensure the quality and reliability of the collected data.
Data privacy and security have become critical concerns in the healthcare industry, particularly with the increasing use of digital health technologies. The proliferation of connected devices and the exchange of sensitive patient information necessitate robust data protection measures. Consequently, healthcare organizations are investing in data labeling solutions that prioritize privacy and security to maintain patient confidentiality and comply with regulations.
AI-driven technologies, such as image recognition and natural language processing (NLP), are transforming the way healthcare data is analyzed and utilized. Image recognition enables the automatic labeling and categorization of medical images, optimizing diagnostic accuracy and improving patient outcomes. NLP, on the other hand, facilitates the extraction of valuable insights from unstructured clinical text, enabling researchers and healthcare professionals to uncover hidden patterns and trends.
Another significant area of growth in the healthcare data collection and labeling market is precision medicine and genomics. With advancements in genomic research and personalized medicine, there is an increasing demand for labeled genetic data to develop targeted therapies and diagnostic tools. Data labeling plays a crucial role in enhancing the accuracy and reliability of genomic data, enabling precise and tailored healthcare interventions.
In summary, the healthcare data collection and labeling market is experiencing substantial growth due to the increasing demand for healthcare analytics, the widespread adoption of EHRs, the integration of AI and ML applications, the expansion of telemedicine and remote monitoring, the focus on data privacy and security, advancements in image recognition and NLP, and the growth of precision medicine and genomics. This market presents significant opportunities for data labeling companies to provide accurate and reliable healthcare data that drives innovation and improves patient care.
Data Labeling: A Prerequisite for AI in Healthcare
Data labeling plays a crucial role in the successful integration of AI in healthcare. By accurately tagging and structuring medical data, data labeling ensures that AI algorithms can effectively analyze and interpret information. This process serves as a prerequisite for a wide range of applications in healthcare, enabling early disease detection, personalized medicine, augmented clinical decision-making, accelerated drug discovery and development, and even robotic surgery.
Accurate data labeling is essential for the development and deployment of AI-powered healthcare solutions. Through meticulous annotation and categorization, medical data can be transformed into valuable insights, enabling healthcare providers to make informed decisions and deliver targeted interventions. Whether it's identifying patterns for early disease detection or tailoring treatment plans to individual patients, accurate data labeling forms the foundation for leveraging AI's full potential in healthcare.
Applications of Data Labeling in AI Healthcare | Benefits |
---|---|
Early disease detection | Enables timely intervention and improved patient outcomes |
Personalized medicine | Tailors treatment plans to individual patient characteristics |
Augmented clinical decision-making | Provides healthcare professionals with data-driven insights for more accurate diagnoses and treatment decisions |
Accelerated drug discovery and development | Speeds up the research and development process, bringing new treatments to market more efficiently |
Robotic surgery | Enhances surgical precision and improves patient safety |
Data labeling empowers AI algorithms to make sense of complex medical data, enabling healthcare providers to harness the power of AI for better patient care. The accurate structuring and annotation of data ensure that AI models can uncover valuable insights, support clinical decision-making, and transform the healthcare landscape. As the field of AI in healthcare continues to evolve, the importance of accurate data labeling remains paramount in driving innovation and improving patient outcomes.
Challenges in Medical Data Labeling
Medical data labeling presents various challenges that organizations face when annotating and categorizing healthcare data. These challenges include limited scalability, cost and time constraints, subjectivity and consistency, as well as technology and quality control concerns.
1. Limited Scalability
The scalability of medical data labeling can be a significant challenge for organizations. As the volume of healthcare data continues to grow exponentially, scaling up labeling efforts to handle large datasets becomes complex and time-consuming.
2. Cost and Time Constraints
Cost and time constraints are key challenges in medical data labeling. The labeling process requires resources, including skilled annotators, advanced tools, and infrastructure. Additionally, meeting project deadlines within budgetary limitations can hinder the efficiency of the labeling process.
3. Subjectivity and Consistency
Subjectivity and maintaining consistency in medical data labeling are vital concerns. Manual labeling processes involve human annotation, which can introduce subjectivity and inconsistency in the labeled data. Ensuring a standardized approach and training annotators for consistency become essential to obtain accurate and reliable annotations.
4. Technology and Quality Control
The use of technology in medical data labeling introduces its own set of challenges. Implementing and managing annotation platforms, machine learning algorithms, and quality control mechanisms require specialized expertise. Handling large volumes of data while maintaining data integrity, privacy, and accuracy further add to the technological challenges in medical data labeling.
To overcome these challenges, organizations need to invest in scalable labeling solutions, optimize workflows, streamline processes, and integrate automated quality control mechanisms to ensure the accuracy and efficiency of medical data labeling.
Challenges in Medical Data Labeling | Solutions |
---|---|
Limited Scalability | Invest in scalable labeling solutions and infrastructure to handle large volumes of data. |
Cost and Time Constraints | Optimize workflows and leverage automated tools to improve efficiency and meet project deadlines. |
Subjectivity and Consistency | Train annotators for consistency and implement standardized annotation guidelines. |
Technology and Quality Control | Integrate advanced technology platforms and implement automated quality control mechanisms to ensure data integrity, privacy, and accuracy. |
Overcoming these challenges is crucial to ensure the accuracy and reliability of labeled medical data, enabling the development of robust healthcare applications, clinical decision support systems, and research initiatives in the field of healthcare.
Understanding Significance and Ethical Considerations of AI Data Annotation
AI data annotation plays a significant role in healthcare by ensuring accurate and ethical labeling of data. In an era where data is abundant but unstructured, AI data annotation provides the necessary framework for organizing and categorizing healthcare information. By labeling data with relevant tags and annotations, AI algorithms can efficiently process and analyze vast amounts of healthcare data, leading to valuable insights and improved decision-making.
However, the importance of ethical considerations and privacy safeguards cannot be overstated. In healthcare, data labeling must adhere to strict guidelines to protect patient privacy and maintain confidentiality. Data labeling companies employ privacy-centric approaches, ensuring that patient information is anonymized and encrypted before being used for training AI models.
"Ethical data annotation practices are crucial in healthcare, as they protect patient privacy and maintain trust in AI applications."
Moreover, ethical considerations go beyond privacy safeguards. It is imperative for data labeling professionals to address potential biases in labeling and ensure transparency in the annotation process. By applying robust quality control measures and involving diverse teams of experts, ethical data annotation practices can minimize biases and promote fairness in healthcare AI systems.
Understanding the significance of AI data annotation and the need for ethical practices is crucial for building trust in AI applications in healthcare. Ethically labeled data not only leads to more accurate AI models but also fosters a sense of confidence among healthcare professionals, patients, and regulatory bodies.
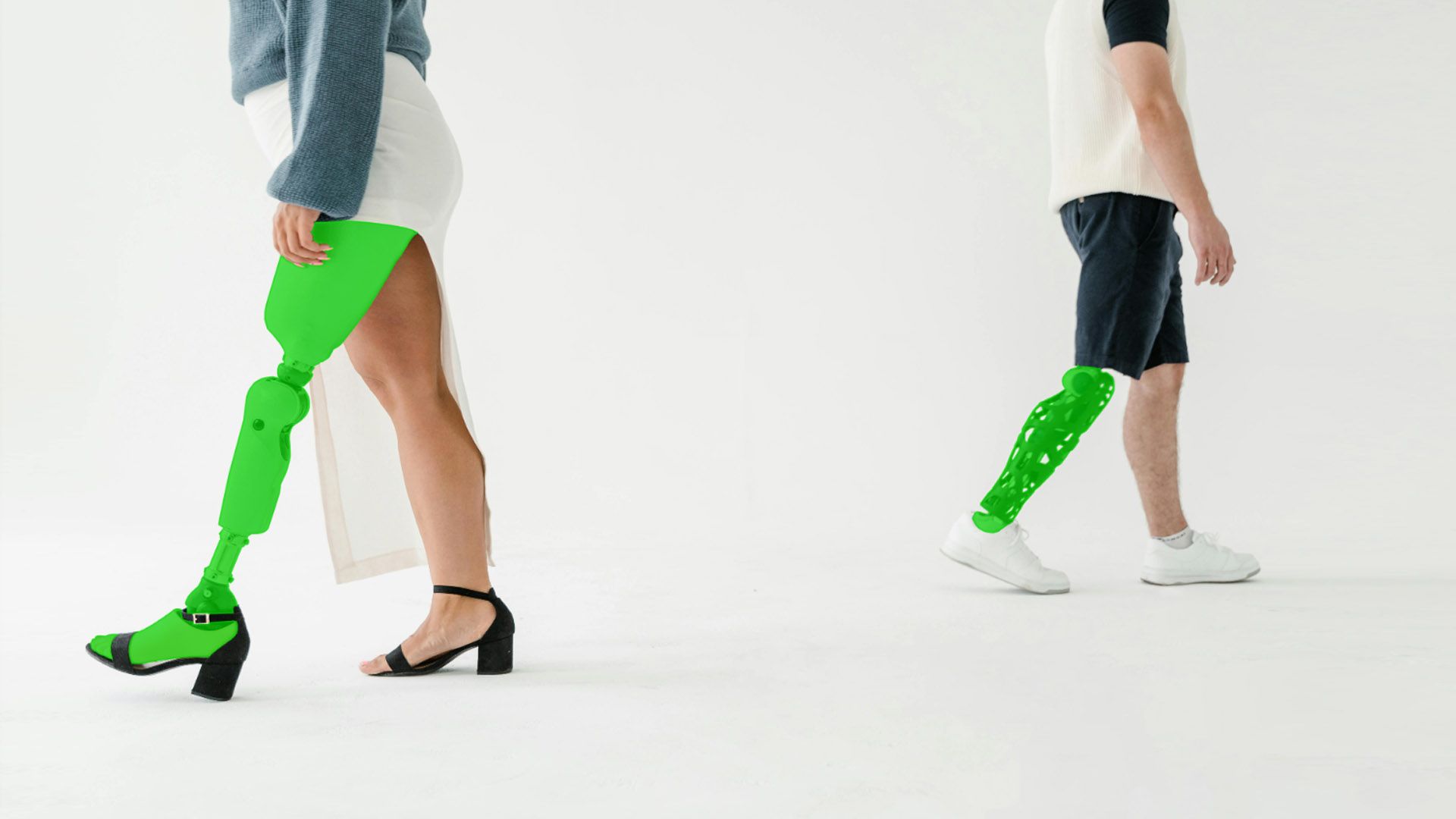
The Role of Privacy Safeguards in AI Data Annotation
Privacy safeguards form an integral part of AI data annotation in healthcare. These safeguards are designed to protect patient information and prevent unauthorized access, ensuring compliance with relevant regulations such as HIPAA and GDPR. By adopting stringent security measures, data labeling companies mitigate the risk of data breaches and safeguard sensitive healthcare data.
Moreover, privacy safeguards extend beyond data protection. They also facilitate patient consent and transparency in the data labeling process. Patients have the right to know how their data is being used and should have the opportunity to provide informed consent. Transparent data annotation practices help establish trust between patients, healthcare providers, and data labeling companies.
By prioritizing privacy safeguards and ethical considerations, AI data annotation in healthcare can unlock the true potential of AI, enabling innovative solutions that improve patient outcomes, enhance clinical decision-making, and revolutionize the healthcare industry as a whole.
Conclusion
Data labeling companies have emerged as key players in the advancement of AI in healthcare by meticulously curating and refining healthcare datasets. Their specialized expertise enables AI models to deliver accurate diagnoses, personalized treatment plans, and ultimately, improved patient outcomes. The collaboration between data labeling companies and AI development represents a transformative force in medical diagnostics and decision-making.
By meticulously categorizing and annotating healthcare data, data labeling companies ensure that AI models have access to high-quality and well-organized datasets. This allows AI algorithms to learn and analyze vast amounts of healthcare information, empowering them to make accurate predictions and recommendations.
The integration of AI in healthcare has the potential to revolutionize the quality of patient care. With the support of data labeling companies, AI models can process complex medical data and generate personalized treatment plans, enabling healthcare providers to offer targeted and effective interventions. This personalized approach has the potential to greatly enhance patient outcomes, leading to improved overall health and well-being.
The partnership between data labeling companies and AI development holds great promise for the future of healthcare. As AI technologies continue to advance, data labeling companies will play a critical role in ensuring the availability of high-quality labeled datasets to fuel the development of cutting-edge AI models. Together, these advancements will lead to more accurate diagnoses, personalized treatment options, and ultimately, better healthcare outcomes for patients worldwide.
FAQ
What is data labeling in healthcare?
Data labeling in healthcare refers to the process of categorizing and annotating healthcare data to make it more meaningful and useful. It involves organizing and labeling data related to various aspects of healthcare, such as medical coding, clinical entity recognition, image annotation, EHR annotation, and sentiment analysis.
Why is medical data labeling important?
Medical data labeling is crucial for training machine learning models, enhancing data analysis, enabling clinical research, supporting clinical decision-making, facilitating interoperability and data exchange, ensuring patient safety and quality of care, and compliance with regulations and standards. Labeled medical data enhances the utility, accuracy, and usability of healthcare data.
How does data labeling benefit AI in healthcare?
Data labeling is a prerequisite for AI in healthcare as it ensures accurate tagging and structuring of medical data. It enables early disease detection, personalized medicine, augmented clinical decision-making, accelerated drug discovery and development, and robotic surgery. Accurate data labeling is essential for the successful deployment of AI/ML models in healthcare.
What are the challenges in medical data labeling?
Medical data labeling faces challenges such as limited scalability, cost and time constraints, subjectivity and consistency, and technology and quality control. Scaling up data labeling efforts can be a hurdle for organizations, and cost and time constraints can impact the labeling process. Subjectivity and consistency are challenges in manual labeling, while technology and quality control are important considerations in managing large volumes of data.
What are the significance and ethical considerations of AI data annotation in healthcare?
AI data annotation plays a significant role in healthcare by ensuring accurate and ethical labeling of data. Ethical considerations and privacy safeguards are essential in the healthcare sector, and data labeling companies adopt privacy-centric approaches to protect patient information. Understanding the significance of AI data annotation and the need for ethical practices is crucial for building trust in AI applications in healthcare.
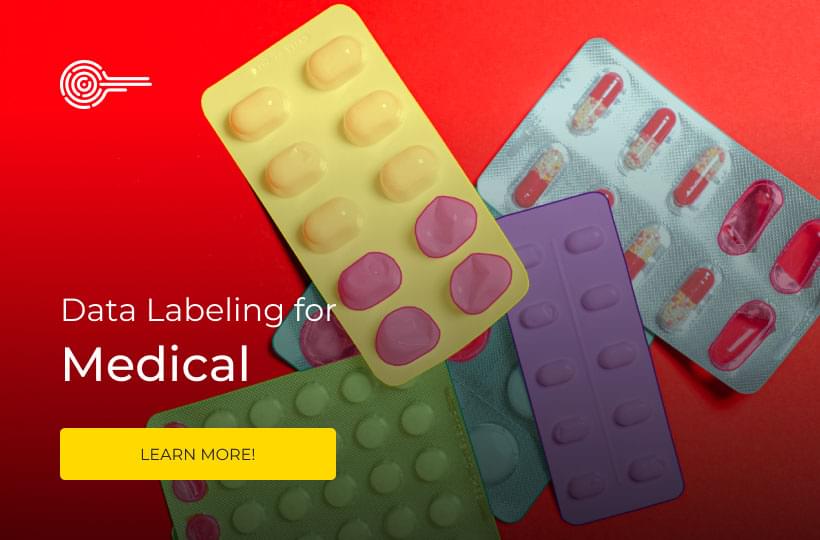