Data from the Field: The Importance of Data Training for AI in Agriculture
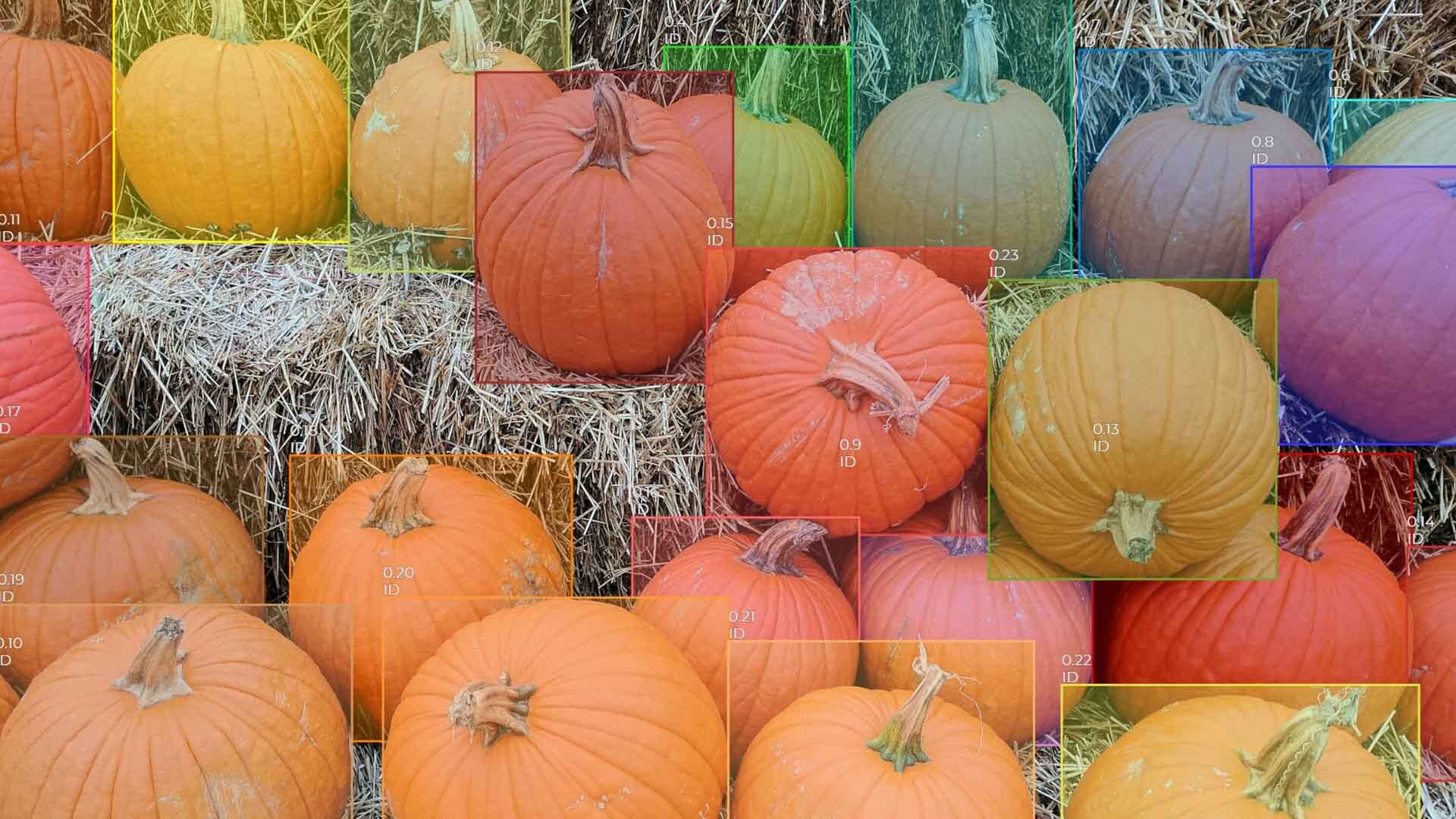
The integration of AI in agriculture could slash pesticide use by 90% for tasks like weed detection and pest control. This impressive figure underscores the revolutionary role of AI technology in the agricultural sector. By applying AI, farming practices become not only more efficient but also environmentally friendly.
For AI in agriculture to be effective, it demands rigorous data training. Such precision in AI tools is critical to improving farm efficiency and outputs. With the world's population expected to hit 10 billion by 2050, the agricultural industry needs AI to satisfy growing food demands and enhance crop yields.
Key Takeaways
- AI technology for farming can reduce pesticide usage by 90%, enhancing sustainability.
- Data training in AI is crucial for automating agricultural tasks like crop health monitoring and soil analysis.
- Leveraging AI in agriculture leads to significant improvements in efficiency and profitability.
- Accurate image annotation is vital for creating effective AI datasets in agricultural data analysis with AI.
- AI provides farmers with insights into market demand, helping optimize planting times and maximize profits.

Introduction to AI in Agriculture
With the global population soaring, agriculture faces new challenges in sustainable food production. The integration of machine learning into agriculture presents a robust solution. This approach aids farmers in increasing efficiency and making data-driven decisions. Advanced technologies are key in improving yields and managing resources effectively.
The Role of AI
AI serves various functions in agriculture, from analyzing market demands to improving crop genetics. For example, market demand analysis, intelligent spraying, and precise breeding are part of its realm. Through data training for AI in agriculture, algorithms can now predict crop health and soil conditions with more than 90% accuracy. These technologies, backed by AI sensors and imagery, empower farmers to preemptively address crop needs while cutting costs by nearly 30%.
Notable AI initiatives include analyzing soil quality and identifying crop diseases. Technologies like Computer Vision and Large Language Models (LLMs) drive these projects. They represent a significant leap forward, offering unprecedented insights into crop health and environmental adaptability.
Why AI is Important
AI's impact on agriculture is profound. Systems managed by AI reduce waste by 20% and improve the efficiency of deliveries. Moreover, autonomous farm machinery decreases labor costs by up to 30%, enhancing productivity by roughly 25%. Sustainable farming practices, powered by ethical AI, significantly reduce water and chemical use. This not only bolsters profitability but also protects the environment.
Understanding Data Training for AI in Agriculture
Data training in agriculture prepares data sets to improve AI performance in applications such as crop monitoring. Keymakr is known for creating custom datasets for agriculture. These datasets enhance AI systems' ability to manage tasks like pest control and soil analysis.
What is Data Training?
Data training organizes data to train AI models with efficiency and accuracy. In agriculture, this ensures AI models are reliable for tasks like disease detection. Techniques in precision agriculture utilize AI data training, supported by drone technology, to make informed decisions.
Types of Data Used
Training AI in agriculture involves diverse data types:
- Image Data: Drones and satellites capture high-resolution images, annotated to identify crop health and pest detections.
- Video Data: Real-time videos track crop development, facilitating early interventions for better yield.
- Soil Health Data: Information on soil elements informs AI models about fertilization and watering schedules.
- Weather and Climate Data: These data steers decisions on planting and irrigation based on weather trends.
- Crop Rotation and Planting Patterns: Analysis of yield history and crop rotations enhances model predictions for future farming.
Benefits of Data Training in AI for Agriculture
Data training in AI significantly transforms the agricultural field, offering vast benefits. It has become crucial in modern farming, enhancing insights and supporting decisions. This revolution in agriculture through AI is paving the way for more efficient and productive farming techniques.
Improved Crop Monitoring
AI significantly improves the way crops are monitored. It enables early detection of health issues and pests, leading to quick actions that boost yields. Drones combined with AI, for example, identify plant infections and nutrient lacks early. This ensures crops get timely treatments.
Moreover, AI-driven tools lower costs in agriculture. They automate processes like planting and harvesting, reducing labor expenses and boosting efficiency. This automation streamlines farming, making it more cost-effective and productive.
AI's role in agriculture grants farmers the insight to refine irrigation, fertilization, and pest control, maximizing resource use and productivity. Real-time data analysis for predictive insights also mitigates risks, enhancing profitability for farmers.
Enhanced Soil Health Analysis
AI also offers critical insights into soil health, aiding in better nutrient management. Through AI, farmers obtain detailed soil condition data, fostering improved crop quality. This enables creation of optimal conditions for crop growth.
AI's application in precision farming diminishes chemical use, lessening environmental harm and advancing sustainable agriculture. AI tools offer precise recommendations for managing fertilizers and water, promoting healthy soil and high crop yields.
Leading AI technologies in agriculture, like FarmBot, Agrix.in, and IBM Watson Decision Platform, drive these innovations. These technologies aid in monitoring crops and soil, optimizing resource allocation, and ensuring sustainable agricultural methods.
Data Annotation Techniques
In the dynamic world of agriculture AI training, data annotation is key. It forms accurate, robust datasets. These techniques ensure AI models can interpret agricultural data effectively. This interpretation aids in better decision-making and increases operational efficiency.
Image Annotation
Image annotation is essential in agricultural AI data analysis. It involves tagging elements in images to help machine learning. Techniques like bounding boxes, oriented bounding boxes, and polygons are used. They are crucial for identifying crops, weeds, and managing livestock accurately.
Companies like Keymakr provide advanced data annotation. Our services contribute to generating quality datasets. This data empowers AI to monitor crops, detect pests, and manage soil effectively. The result is enhanced agricultural efficiency.
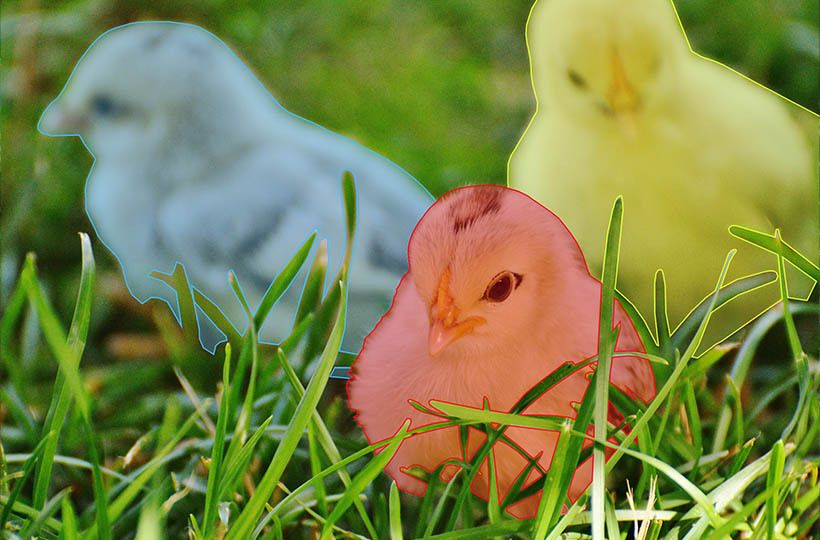
Instance Segmentation
Instance segmentation plays a vital role in agriculture AI training. It goes beyond simple pixel classification. This method annotates each object instance separately. It enables distinct recognition of plants or pests in images. Thus, it offers deeper insights into agriculture AI data analysis.
This technique is used in automated harvesting systems. Quality annotations allow for accurate fruit detection and positioning. This leads to efficient harvesting without harming the produce. It boosts sustainability and productivity in the agricultural sector.
- Remote sensing (RS) systems: Improve crop production through accurate crop yield estimates using various inputs.
- Machine vision: Facilitates plant disease and pest detection via deep learning approaches trained on large image datasets.
- Aerial crop analytics: Utilizes AI and drone platforms for efficient crop monitoring and early pest/disease detection.
- Automated systems: Depend on high-quality training data for accurate fruit detection and localization during harvesting.
- Produce grading and sorting: Leverages computer vision for time-saving, labor cost reduction, and enhanced accuracy.
Technique | Application | Benefits |
---|---|---|
Bounding Boxes | Crop and Pest Detection | Identifies area-specific anomalies efficiently |
Oriented Bounding Boxes | Weed Management | Offers precise angular annotations |
Polygons | Livestock Management | Delineates complex shapes accurately |
Instance Segmentation | Automated Harvesting | Detailed individual object recognition |
Applications of AI in Agriculture
The agriculture sector is evolving rapidly with the projected global population reaching 10 billion by 2050. AI technology plays a crucial role in responding to the increasing food needs. Through machine learning, agriculture is becoming more productive and efficient. Let's delve into the key applications of AI in the field.
Crop Health Monitoring
AI has revolutionized crop health monitoring. Early detection and predictions of diseases are now possible. Drones with AI capabilities offer precise real-time aerial images. They pinpoint crop issues, aiding in yield prediction and timely action to enhance productivity.
Machine learning, alongside satellite and drone images, is vital for weather prediction and analyzing crop sustainability. It detects pests and advises on soil health and the right pesticide use. These innovations support farmers in planning crop rotation and planting, promoting sustainability.
Soil and Field Monitoring
Sustainable farming necessitates comprehensive soil and field analysis. AI evaluates soil parameters like nitrogen and phosphorus content, guiding irrigation and fertilization. By applying machine learning, farmers use resources more efficiently, achieving optimal results.
PEAT's Plantix software, with AI, boasts up to 95% accuracy in identifying soil issues. Such accurate AI insights lead to improved soil management and productivity. It marks a significant advancement in agricultural practices.
AI-driven drones and UAV sprayers achieve uniform pesticide or fertilizer distribution. They identify targeted areas for spraying in real time. This technology lowers environmental harm while ensuring top crop quality and yield.
Precision Agriculture and AI
Precision agriculture combines AI to revolutionize farming. It boosts efficiency, productivity, and sustainability.
Improving Efficiency
Technologies like Variable Rate Technology (VRT), GPS/GNSS tracking, and data analytics improve farm efficiency. The Food and Agriculture Organization (FAO) notes they could cut costs by 10-20% and up yields by 10-15%. AI allows for real-time monitoring and predictive analytics. So, farmers can make decisions that use resources wisely and boost crop quality.
Automated Systems
Automated systems, thanks to AI, are enhancing agriculture. From driverless tractors to drones for monitoring crops and livestock, these advancements are key. John Deere’s technology, for example, precisely targets fertilizers and pesticides to needed areas. This smart approach reduces chemicals and supports sustainable farming.
AI’s role in agriculture promises significant industry evolution. McKinsey suggests digital farming could add $500 billion to $1.5 trillion annually by 2030. As AI evolves, its farm applications will grow, increasing productivity and sustainability. This is crucial for feeding the expanding global population.
Challenges in Data Training for AI in Agriculture
Incorporating AI in agriculture brings various challenges, like guaranteeing data quality and navigating cost barriers. For AI training to be successful, quality and comprehensive datasets are vital. Still, collecting agricultural data is tough, more so in less developed nations. This results in problematic data quality and accessibility for AI training.
Quality of Data
For AI in agriculture to work well, quality data is essential. The accuracy of AI solutions heavily depends on the training data. Innovations in farm management software improve data quality and availability. Yet, the data is often scattered and inconsistent. This complicates the development of dependable AI models. Collaboration among stakeholders is key to better data collection and quality.
Accessibility and Cost
Cost and accessibility are major hurdles for AI's broad use in farming. High expenses for necessary technology deter small-scale farmers. Additionally, many lack the technical know-how. Solutions like subsidies or tax breaks could ease cost barriers, enabling broader AI adoption. Furthermore, data privacy and security worries deter data sharing, due to misuse fears.
To make AI training in agriculture work, these obstacles need addressing. Strong data protection, government aid, and partnerships between Agtech firms and farmers are crucial. They will ensure AI's inclusive implementation in agriculture.
Technologies Supporting Data Training in Agriculture
AI's growth in agriculture emphasizes the significance of diverse technologies. They facilitate data training and boost agricultural performance. Tools like drones and IoT sensors are instrumental, enabling accurate data gathering and instant monitoring. This innovation steers decisive farming practices. Hence, these technologies transform raw data into valuable insights for farmers, promoting efficient agriculture.
Drones
Drones have revolutionized agriculture, providing crucial data through field mapping and real-time observation. Capturing high-quality images, drones assess various crop parameters effectively. Thus, UAVs can pinpoint plant health, evaluate crop progress, and identify water shortages. Such insights allow farmers to swiftly tackle issues. This tech supports sustainable agriculture, addressing climate change concerns.
IoT Sensors
IoT sensors play a pivotal role in agriculture by monitoring key factors like soil moisture, nutrients, and weather. Integrating IoT sensor data with AI enables informed, effective decisions. This cuts costs and reduces environmental damage by optimizing resource use. Therefore, these devices are essential for efficient farming operations.
The crucial role of data training in agriculture is evident. It underpins AI applications in the sector. Drones and IoT sensors enhance AI's capability, leading to more sustainable and productive farming practices.
FAQ
What is the role of AI in agriculture?
AI boosts agriculture by streamlining efficiency and productivity. This includes analyzing market demands, managing risks, and improving crop selection and protection. AI-driven strategies help farmers anticipate market trends, safeguard crops, and choose the best seed varieties. These innovations lead to higher profits and less environmental damage.
Why is AI important in agriculture?
AI is crucial in farming for enhancing output and reducing waste. It drives decisions that cut down environmental harm and the use of resources. With AI, farming becomes more efficient, outdoing traditional methods. Farmers gain from tech advancements, which boost productivity significantly.
What is data training for AI in agriculture?
Data training prepares datasets for AI in farming. Companies like Keymakr specialize in annotating image and video data. This is key for tasks such as robotics and pest detection. Annotated data ensures AI models work well in practical agricultural settings.
What types of data are used in AI training for agriculture?
AI relies on image and video data from drones and sensors. This data is marked using techniques like bounding boxes. Such detailed annotations allow AI to accurately assess and respond within the agricultural field.
How does AI improve crop monitoring?
AI refines crop monitoring by identifying health problems and pest issues early. Timely actions from these insights boost yields and crop quality. Farmers get real-time data, making field management more efficient.
How does AI enhance soil health analysis?
AI gives deep insights into soil health, guiding tailored farming decisions. By understanding soil and field specifics, farmers optimize irrigation and fertilizer use. This approach fosters a healthier crop environment and saves resources.
What is image annotation in the context of agricultural data?
Image annotation tags agricultural images for AI training. Methods include bounding boxes and polygons. It's essential for AI to recognize and classify farm imagery, making vision-based farm tasks more accurate.
What is instance segmentation?
Instance segmentation identifies individual items in data sets. This technique allows for a detailed analysis by agricultural AI, enhancing its ability to manage crops and pests accurately.
How does AI monitor crop health?
AI uses sophisticated means to detect diseases and deficiencies in plants. It ensures plants thrive by letting farmers act swiftly to maintain health. This results in greater yields and superior produce quality.
How does AI improve soil and field monitoring?
By analyzing soil and field data, AI optimizes farming techniques. It adjusts irrigation and fertilization based on current data. This promotes sustainable farming, boosts crop predictions, and conserves resources.
How does AI contribute to precision agriculture?
AI boosts farming efficiency and fosters the use of automated tools. Intelligent spraying minimizes chemical use. Meanwhile, automation like drones and smart irrigation cuts labor demands, streamlining farm operations.
What are the main challenges in data training for AI in agriculture?
Challenges include securing quality data for AI and handling the cost of gathering and annotating it. High-quality data is vital for function accuracy. Yet, acquiring and marking this data is pricey and labor-intensive. Access to comprehensive datasets remains a barrier for small-scale farmers.
How do drones support data training in agriculture?
Drones are pivotal for collecting precise agricultural data. They gather imagery for training AI, offering fast solutions to farm issues. Drones enable precise agriculture by providing up-to-date field maps and health assessments.
How do IoT sensors support data training in agriculture?
IoT sensors are key for monitoring soil moisture and weather. Paired with AI, they guide farmers in optimizing resources and keeping an ecological balance. These sensors make informed farming decisions easier, enhancing sustainability.
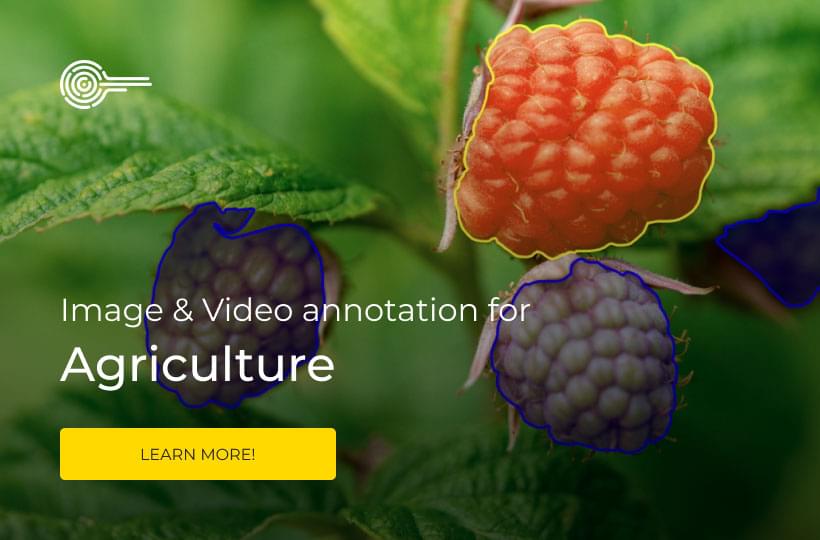