Data Bias in AI Agriculture: Ensuring Fairness and Sustainability
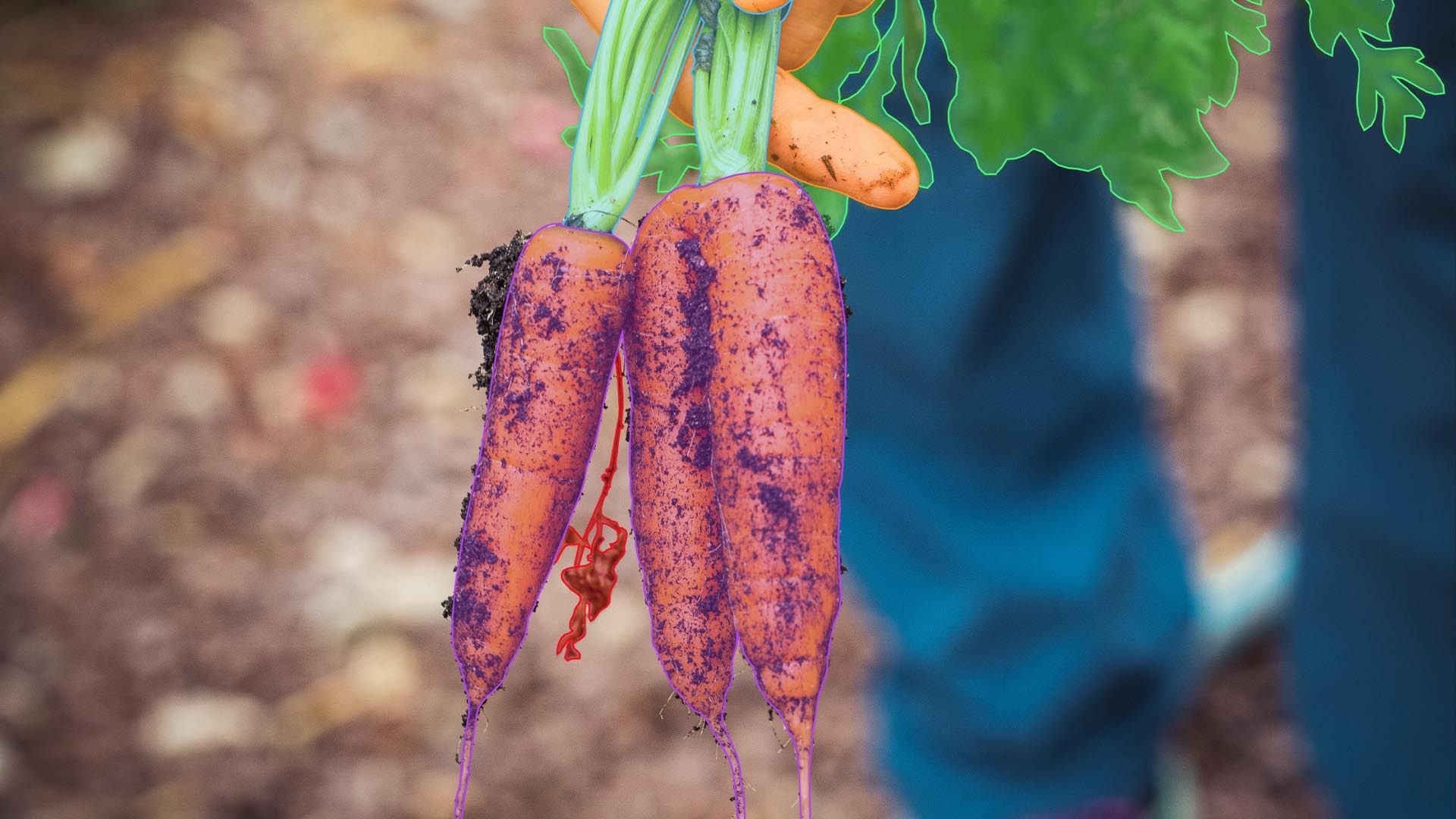
Did you know that AI tech in agriculture is expected to jump from $1.1 billion in 2019 to over $3.8 billion by 2024? The rise of artificial intelligence in farming needs to be mindful of ethical issues. Though AI could change farming for the better, it raises concerns like data bias and fairness's effects on development.
AI is quickly becoming crucial for agriculture, promising to make better use of resources and increase productivity. Yet, using AI in farming raises ethical issues. These include privacy worries, who owns the data, and how AI might make the gap between farmers and tech companies bigger. So, using AI in farming rightly needs clear planning.
To make AI in agriculture fair and sustainable, we need to focus on key ethics like being clear, responsible, private, and solid. By setting up rules and practices that prioritize these ethics, tech companies and those who make the rules can lower the risks of data bias. They can also help AI in farming grow ethically.
Key Takeaways
- AI-based technologies in agriculture are expected to grow a lot, hitting over $3.8 billion by 2024.
- While AI has many benefits for farming, like using fewer chemicals and growing crops better, it also brings up ethical concerns about data fairness and bias.
- Rightly using AI in farming means tackling issues beforehand, such as privacy, data ownership, power differences, and taking responsibility for mistakes the systems might make.
- Ethical ways to use AI in farming should include fairness, clearness, responsibility, sustainability, privacy, and strength.
- Working together, tech companies and rule makers are key to reducing ethical problems and making sure AI is used fairly and sustainably in farming.

The Rise of AI in Agriculture: Opportunities and Challenges
AI in farming uses smart algorithms, software, and even robots to help farmers. It gives them better predictions, suggestions based on data, and help in making quick decisions. These tools are used for many parts of farming, including predicting the weather, choosing crops, and managing livestock.
Benefits of AI in Farming: Efficiency, Precision, and Sustainability
AI in farming offers many benefits. It can make farming more efficient and help the environment by using less water and chemicals. By using AI, farmers can grow more food with less impact on the planet. Some benefits of AI in farming are:
- Increased crop yields through precise monitoring and timely interventions
- Reduced water and chemical usage through intelligent irrigation and fertilization systems
- Improved crop health and disease management with AI-driven pest and disease detection
- Optimized farm management and decision-making based on real-time data analysis
- Promotion of sustainable farming practices by minimizing environmental impact
AI doesn't just help farmers make more money. It also helps them take better care of the earth. By using AI, farmers can save water, reduce waste, and make sure their farms do not harm the environment.
Potential Pitfalls: Data Bias and Its Implications
AI in farming, while promising, does pose challenges. One big issue is data bias. If the data used to teach AI systems is not fair or diverse, the outcomes can be wrong. This can make things worse, rather than better.
responsible AI development for farms
Biased AI may not help all farmers equally. It might prefer some crops or farming methods over others. This can hurt small farmers and those already struggling. They may not get the help they really need, widening the gap between rich and poor.
AI Application | Potential Benefits | Data Bias Concerns |
---|---|---|
Crop Yield Prediction | Accurate forecasting, optimized resource allocation | Bias towards high-yielding varieties, neglecting local adaptability |
Precision Irrigation | Water conservation, improved crop health | Bias based on field characteristics, favoring certain regions |
Pest and Disease Detection | Early detection, targeted interventions | Bias in identifying pests/diseases prevalent in specific areas |
Crop Recommendation Systems | Personalized advice, optimized crop selection | Bias favoring commercially popular crops over traditional varieties |
It's crucial to fight data bias in AI. We should teach AI systems on a wide range of data to avoid bias. Auditing for bias and making AI decisions clear are also key. All this needs a team effort, with tech experts, lawmakers, and farmers working together. They must set up rules and suggestions for fair AI use in farming.
Finding a good balance in using AI in farming is important. We must make sure it helps everyone fairly, and that it doesn't make existing problems worse.
Understanding Data Bias in AI Agriculture
AI tech is becoming a big part of farming. It's key to know and deal with data bias risks. Data bias in AI farming happens when models learn a favored pattern over the real data. This is because of biased assumptions during setup phases or biased training data. It can mean bad predictions, unfair decisions, and issues for farmers and the farming industry.
Types of Data Bias: Selection, Measurement, and Algorithmic Bias
There are different types of data biases in AI farming:
- Selection bias: It occurs when the AI model's training data doesn't represent the full farming scene. For instance, if an AI uses data from big farms but not small ones, it might not do well on those smaller farms.
- Measurement bias: This happens when data collection methods are wrong or different. Using bad sensors or subjective judgements can mess up the data and the AI models.
- Algorithmic bias: If the AI's design or the goals it's set to meet are narrow, it can miss out on important things. For example, focusing only on how much we grow can ignore the need to take care of the environment or be fair to people.
Causes of Data Bias: Insufficient Diversity, Flawed Data Collection, and Human Prejudices
Lots of things can lead to data bias in AI farming:
- Insufficient data diversity: AI can struggle if it's only fed data from a limited view. It needs to see and understand a wide variety of farms and situations.
- Flawed data collection methods: Mistakes or inconsistencies in gathering data create biases. This can be because of broken sensors, biases in sampling, or not labeling data consistently.
- Human prejudices in AI training data: The way we choose and label training data can accidentally add our biases into AI. If the past data reflects bad actions or unfair treatments, the AI might repeat those mistakes.
Industry | Example of AI Bias | Consequence |
---|---|---|
Criminal Justice | COMPAS system showed bias against African-American defendants | Unfair sentencing recommendations |
Healthcare | AI system predicting mortality rates assigned higher risk scores to African-American patients | Potential disparities in healthcare resource allocation |
Facial Recognition | Less accurate for darker skin tones | Higher rates of false positives, wrongful arrests |
Generative AI | Gender bias in generated images of workers, racial bias in generated images of criminals | Reinforcement of stereotypes and prejudices |
Tackling data bias is important to make fair and solid AI for farming. This means involving many people from the start, checking data carefully, and always looking out for biases and bad outcomes.
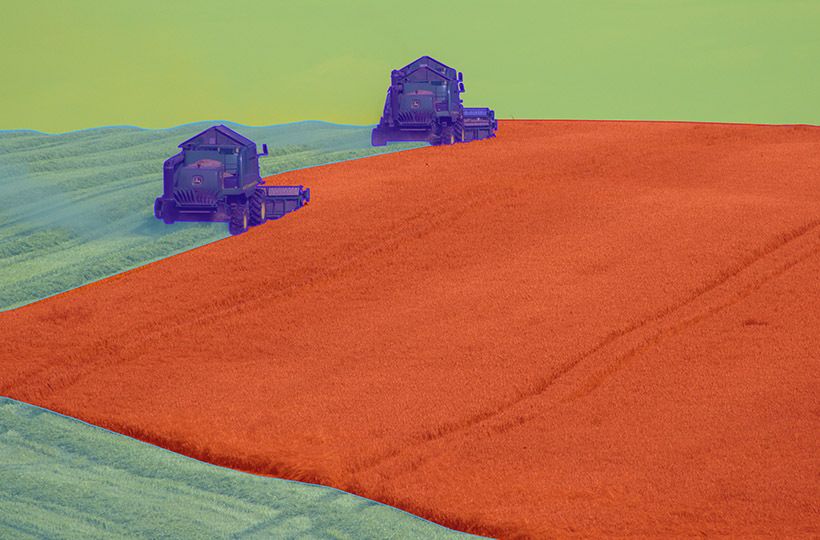
Impact of Data Bias on Agricultural Stakeholders
Data bias in AI agriculture affects many in the farming world. Farmers face the worst of it. They use these systems for key decisions. But if the AI is biased, it gives bad advice. This can lower yields, raise costs, and harm the environment.
Firms that rely on AI for planning are at risk too. They might make the wrong calls due to AI's bias. This can lead to losing money and hurting their image. As people learn more about AI, trust in these firms could drop if they don't fix bias.
Unfair AI hurts not just farmers and firms, but whole communities. It hits small farmers and those with less access to tech the hardest. This makes the gap between them and others even bigger. It slows down everyone's progress in farming.
"Addressing data bias is essential to ensure fair and equitable outcomes for all stakeholders in the agriculture value chain. It requires a concerted effort from technology providers, policymakers, and agricultural organizations to develop inclusive AI solutions that account for the diverse needs and contexts of farming communities."
Consumers may also feel the effects of bias. Biased AI can impact the food we get, its cost, and availability. It might favor a certain type of crop. This could make food less varied and sturdy.
Stakeholder | Impact of AI Bias |
---|---|
Farmers | Unfair resource allocation, inaccurate recommendations, reduced yields, increased costs, environmental damage |
Agribusinesses | Misguided strategic decisions, financial losses, reputational risks |
Farming Communities | Exacerbation of inequalities, disproportionate impact on smallholder farmers and marginalized groups |
Consumers | Influence on food quality, prices, and availability, reduced diversity in the food supply chain |
It's important to fight AI bias in farming. This means being fair, clear, and responsible with AI. We can do this by:
- Using varied data to teach AI models
- Spotting and fixing bias in AI early on
- Talking to farmers to learn about their needs
- Teaching people about AI and making AI tools accessible to all
- Setting rules for using AI in farming
Working against data bias helps the farming world. It lets AI help us farm better, safely, and fairly. This way, everyone benefits.
Fairness in AI-Driven Agriculture: Ensuring Equal Opportunities
The world of agriculture is changing with AI. We must make sure that everyone gets a fair chance. This means we have to check the data and models for bias. Diverse training data and fair algorithms are key to this.
Identifying and Mitigating Unfair Practices in AI Agriculture
Farmers, especially from marginalized groups, can suffer from unfair AI practices. This happens when:
- AI models are not fair because the data they learn from is biased.
- Farmers don’t understand how AI makes decisions, so they can’t trust it.
- Not all farmers get the same access to AI, which keeps some behind.
To fight these issues, we must listen to what farmers need. Technology should be developed with them directly involved. This way, AI can truly help all farmers.
Promoting Inclusive AI Solutions for Diverse Farming Communities
Everyone should have a fair shot with AI in farming. This means:
- Creating AI that meets the unique needs of small farmers and minority groups.
- Teaching farmers how to use AI in ways that help them.
- Ensuring AI tools are available to everyone, no matter their situation.
Getting this done needs everyone to work together. Tech companies, governments, and farmers can set fair rules. This makes sure AI helps everyone fairly.
Aspect | Importance | Strategies |
---|---|---|
Diverse representation in training data | Ensures AI models are inclusive and unbiased | Collect data from various farming communities and regions |
Regular audits for bias | Identifies and addresses unfair practices in AI systems | Conduct periodic assessments using fairness metrics and stakeholder feedback |
Inclusive AI solution design | Caters to the needs of diverse farming communities | Engage with farmers to understand their requirements and constraints |
AI literacy programs | Empowers farmers to leverage AI technologies effectively | Provide accessible training and support for farmers to adopt AI tools |
By prioritizing fairness and inclusivity in AI-driven agriculture, we can unlock the full potential of these technologies to support sustainable and equitable farming practices for all.
Focusing on fairness in AI farming is critical. It's not just about doing the right thing; it's about making farming better for everyone. As AI becomes more common in farming, let's make sure it helps all farmers, no matter where they come from.
Transparency and Accountability in AI Agriculture
Integrating AI into farming needs transparency and accountability for trust and responsible tech growth. This means clearly sharing info on data use and AI model operation. Tech makers should explain how their AI systems work, helping farmers make smart choices.
Explainable AI is key for clear farming practices. It shows farmers how AI reaches its decisions. This demystifies AI, earning trust and letting farmers use AI well.
Importance of Explainable AI Models in Farming
Explainable AI models are crucial for farming:
- They boost trust and tech adoption because farmers get how AI decides.
- They help farmers choose using clear, AI-backed advice.
- These models find and fix AI bias, making results more accurate.
Stats show why explainable AI is vital for farming:
Statistic | Implication |
---|---|
Over 40% of "AI start-ups" don’t really use AI. | Knowing the tech is key to telling real AI apart from bluff. |
AI software recognizes plant health from images, aiding farmers. | Explainable AI models make this insight clear and trustworthy for farmers. |
Establishing Accountability Frameworks for AI-Driven Decisions
In AI farming, being accountable means setting rules for AI’s choices and effects. This involves:
- Putting in place who does what in AI use and oversight in farming.
- Checking AI for biases and fairness to meet ethical rules.
- Having ways for farmers to report and solve AI issues.
Lawmakers must guide AI use in farming, setting rules. This helps make ways for responsible AI on farms.
By working together, tech folks, farmers, and lawmakers can make AI in farming open and fair. Putting transparency and accountability first allows AI to better agriculture in balanced, trustworthy ways.
Sustainability and Ethical Considerations in AI Agriculture
While we aim for better farm efficiency, we must not forget our social duties. We need smart rules in place that care for the Earth, treat animals right, and assure fair practices. Six main ethical guidelines underpin the use of AI in farming, ensuring fairness, clearness, responsibility, eco-care, privacy, and reliability.
Fighting AI bias is a must – think of the harm a partial apple-picking robot could do. We need to train our AI models on a wide range of data to fight bias. And, making sure the AI is easy to understand might also help keep outcomes fair.
The eco-impact of AI farming tools is another big issue. These tools can help save resources but must be used wisely to avoid harming the planet. Watching out for issues like soil damage and pollution is vital. We should always aim for AI to help farming that isn't just efficient but also eco-friendly in the long run.
To make AI in farming truly sustainable, we need everyone on board, from farmers to environmentalists. All should have a say in how we use AI in farming and towards what end. A fair sharing of voices ensures that we don't overlook the real needs of those affected.
Ethical Principle | Implications for AI in Agriculture |
---|---|
Fairness | Ensuring equitable access to AI-driven solutions, especially for small-scale farmers and agricultural communities |
Transparency | Clear communication about AI systems' functioning and decision-making processes to build trust with farmers and stakeholders |
Accountability | Establishing frameworks to determine responsibility for AI-driven decisions and their consequences |
Sustainability | Designing AI solutions that optimize resource use, reduce environmental footprint, and support eco-friendly farming practices |
Privacy | Implementing robust data privacy measures to protect farmers' rights and secure sensitive information |
Robustness | Developing reliable and resilient AI systems that can withstand real-world challenges and uncertainties in agricultural contexts |
It's also vital to keep close tabs on how AI helps or hinders farming's eco-goals. We must often check how our AI systems are doing, talk with those involved, and tweak things as our understanding grows. Making sustainability and ethics a priority means AI can truly change farming for the better, for everyone.
Collaborative Efforts to Address Data Bias in AI Agriculture
Many groups, including farmers, tech experts, policymakers, and scientists, must work together. They aim to reduce bias in AI used in farming. By joining forces, they can make sure AI tools treat everyone fairly and openly in the farming world.
It's important for farmers to help shape AI tools. This ensures these tools match the real challenges they face. It also checks for any hidden errors in AI results. Plus, their insights can make AI better over time.
Technology companies also have a big role to play. They should make fairness, clearness, and responsibility a priority in their AI. This means designing AI that considers everyone and always checking for biases. They must also explain clearly how their AI works.
Engaging Stakeholders: Farmers, Technology Providers, and Policymakers
Making rules and guidelines for using ethical AI in agriculture is a job for policymakers. They can set up laws and encourage good behavior. This way, fair and honest AI can help everyone in the farming world, especially those who need it most.
Everyone working together can do a lot. For instance:
- Having discussions on how to best use AI in farming ethically
- Working with farmers, tech companies, and policymakers to design AI tools together
- Joining efforts between the public and private sector to try out and expand ethical AI use in farms
- Teaching farmers and farm advisors more about AI to make them stronger partners
Promoting Research and Development in Ethical AI for Agriculture
Researchers and tech companies need to work hand in hand with farmers to make sure AI in agriculture is right. Together, they can come up with new, fair ways for AI to support farming. They invent new software and methods to avoid biases in farm data and solutions.
There are some exciting areas in ethical AI in farming:
- Making sure our data in AI truly covers how farming is done in different places, environments, and communities
- Creating AI models that farmers can understand, to help them make good decisions
- Using what farmers know and do best to check on and make AI better
- Looking at how AI's impact is just as good for people and nature in the long run
Sharing what we know, how to do things right, and helping each other learn is key to using AI fairly in farming. Websites, online talks, and learning centers help everyone keep in step with new ideas and actions.
Stakeholder | Key Responsibilities |
---|---|
Farmers | Share what they know and help make AI tools right |
Technology Providers | Make sure their AI is fair, clear, and has good results |
Policymakers | Put in place rules and goals for using AI in good ways |
Researchers | Invent new ways to keep AI in farming free from bias |
United efforts can lead to AI in farming that is honest, clear, and helps everyone, including farmers, communities, and nature.
Summary
It's crucial to fight data bias in AI for farming to be fair and sustainable. By recognizing, the issue, and its effects, we can strive for AI that's both responsible and fair. Getting farmers, tech experts, and policy makers to work together is key for AI in farming to be ethical.
For AI farming to be fair and good for the environment, ethics must come first in AI development. Steps include making sure AI isn't biased, using data fairly, and letting all farmers benefit from AI. We need to be open and honest about data use, so everyone trusts AI farming.
The goal of fair AI in farming depends on us keeping up research and sharing knowledge. If we make AI to fit the needs of different farmers, we can enjoy the good it brings without causing harm. With a focus on being fair, green, and thinking ahead, AI can offer lasting positive change in farming.
FAQ
What is data bias in AI agriculture?
Data bias in AI farming occurs when AI systems prefer a certain pattern. They may not consider the full data. This happens during the creation of algorithms or because the data itself carries assumptions. It can cause AI in farming to make unfair or wrong decisions.
What are the types of data bias in AI agriculture?
There are three main types of data bias. First, selection bias, which means the data used is not truly representative. Second, measurement bias happens when data is collected wrong. Third, algorithmic bias is when the AI system's design has flaws. These issues come from not having diverse data, collecting data poorly, or embedding human bias into AI.
How can data bias impact agricultural stakeholders?
Data bias affects everyone in the agricultural chain. It can cause unfair resource distribution, leading to wrong farming decisions. Businesses might make bad choices or face a bad image based on biased AI. It also impacts consumers by changing food quality, prices, and availability unfairly. Overall, it deepens the divide, affecting those already struggling the most.
What can be done to ensure fairness in AI-driven agriculture?
To fight data bias in AI farming, we need to do a few things. First, regularly check AI for any bias. Make sure the data used is varied and reflective of the real world. Also, keep fairness in mind when designing AI. And don't forget to involve farmers and spread knowledge about AI.
Why are transparency and accountability important in AI agriculture?
Being clear and responsible in using AI in farming keeps it ethical. AI that explains its decisions builds trust with farmers. It helps them understand why certain recommendations are made. Having clear rules and people taking responsibility for AI's effects are also important. This might include checks and balances to make sure AI is used fairly.
How can sustainability be integrated into AI agriculture?
Making AI in farming support the environment means focusing on using resources wisely. It's about creating AI that supports farming without harming the earth. Key values like caring for animals, the planet, and people should guide AI's development. Looking at the long-term impact of AI and encouraging farming that doesn't waste resources can make agriculture more sustainable.
What collaborative efforts are needed to address data bias in AI agriculture?
There's a need for all parties - farmers, tech developers, lawmakers, and researchers - to work on this. It's key to involve farmers in designing AI. Focus should be on making AI fair and accountable. Laws and benefits can be set up to encourage bias-free AI. And research should continue to make AI in farming meet high ethical standards.
Are there any successful case studies of unbiased AI implementation in farming?
There are indeed successful cases where AI has helped farming fairly. For example, using AI in precision farming has cut down on using harmful chemicals. It’s also shown to use resources better and leave a smaller environmental mark. AI designed for small farmers in poorer regions has boosted their farming methods. This has helped them grow more crops and improve their living conditions.
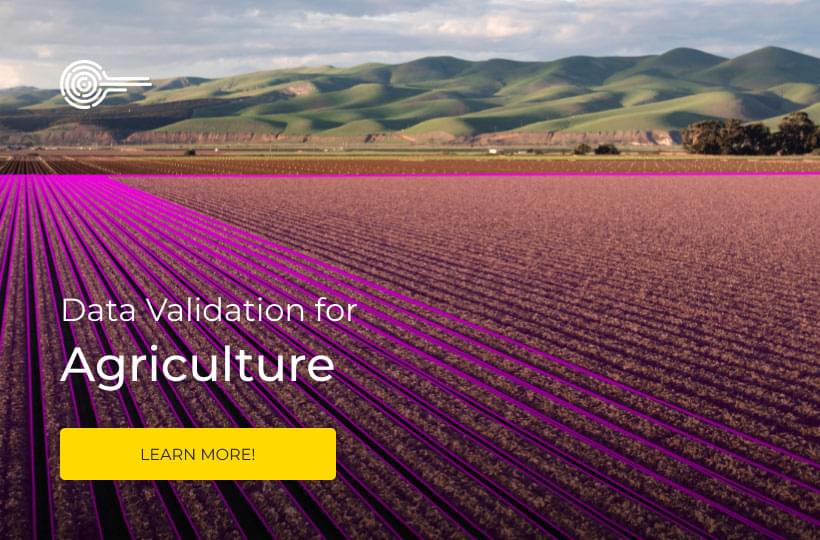