Data annotation quality for self driving vehicles: precision does matter
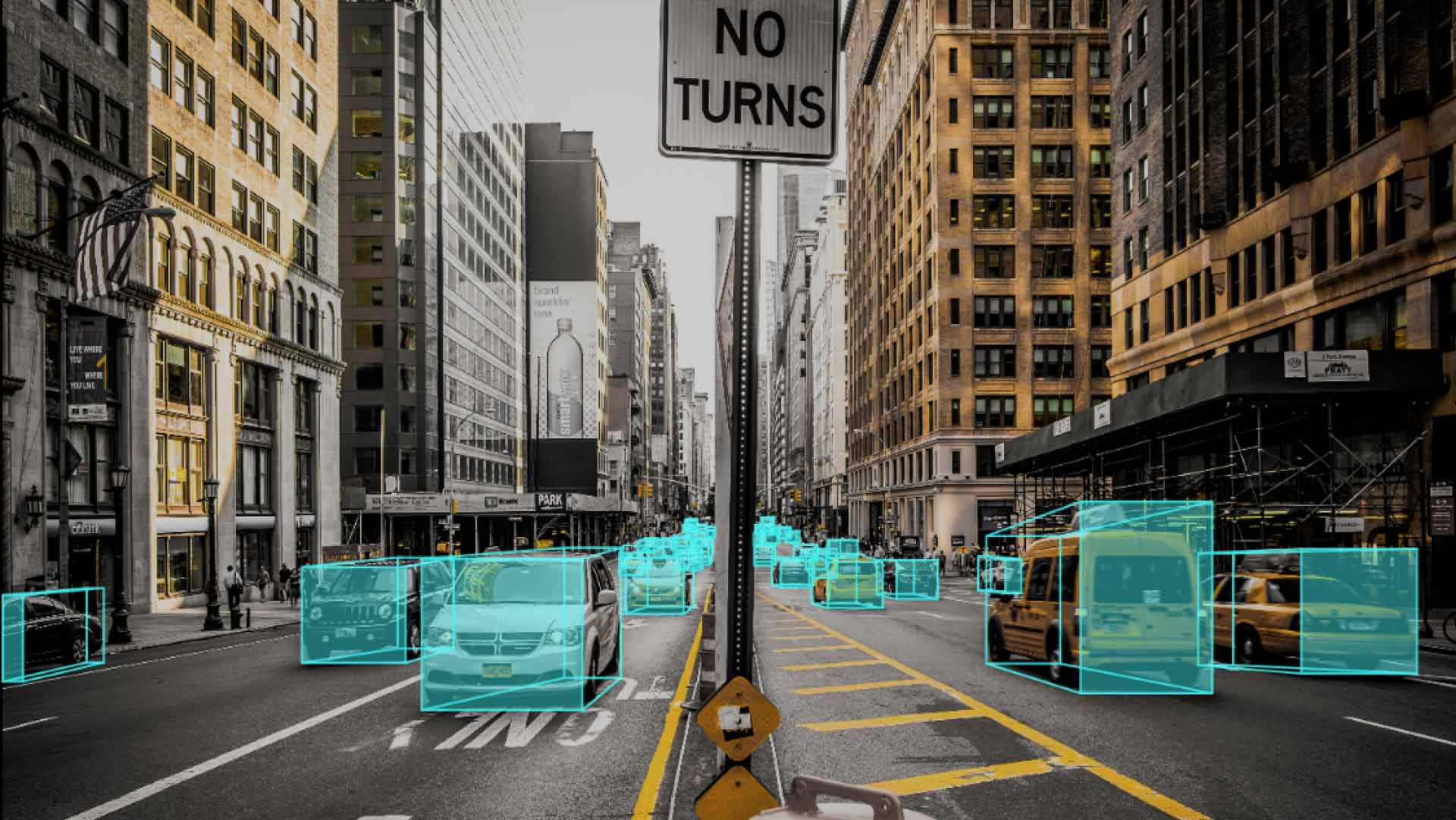
Self-driving vehicles are the future of transportation, and data annotation plays a crucial role in their development. Data annotation is the process of labeling and categorizing data to train machine learning algorithms. In the context of self-driving vehicles, data annotation involves labeling images, videos, and other sensor data to help the vehicle understand its surroundings and make decisions.
The quality of data annotation is essential for the success of self-driving vehicles. Inaccurate or imprecise data can lead to errors in the vehicle's decision-making process, which can be dangerous for passengers and other road users. Precision is crucial in data annotation, and even small errors can have significant consequences.
In this article, we'll explore the importance of data annotation quality for self-driving vehicles, with a particular focus on precision. We'll also examine the role of data validation in ensuring accurate and reliable data annotation. Finally, we'll look at case studies of successful data annotation for self-driving autos and discuss the future of data annotation quality in this exciting field.

Understanding The Importance Of Data Annotation Quality For Self-Driving Vehicles
Data annotation quality is a critical aspect of training self-driving cars. The more accurate and precise the data annotation, the safer and more reliable autonomous vehicles become when navigating through real-world environments. This process is fundamental in ensuring that the machines receive precisely labeled data for flawless decision-making.
Supervised deep learning remains a prevalent algorithm for autonomous driving models, making high-quality annotations even more important to improve their accuracy. Using superior data labeling methods allows these machine learning models to learn from various scenarios leading to better accuracy when on roads.
Self-driving cars' three primary sensors work together like human eyes and brains. They interpret information from their sensor suite - LiDAR, cameras, and radar – then use that information to identify objects' spatial locations, speed, height measurement in Three-dimensional visualization while driving on complex roadways.
In conclusion, impeccable accuracy of data annotation relevance cannot be overemphasized as it ensures the safety of passengers or any other objects encountered while offering us a glimpse into what our transportation infrastructure will look like in the not so distant future powered by AI's capabilities.
The Role Of Precision In Data Annotation For Self-Driving Vehicles
Precision in data annotation is crucial for the success of autonomous driving. The labeling of objects and features on roads, such as traffic signs, vehicles, pedestrians, and obstacles, must be accurate to train vehicles to navigate safely. Training with labeled data is necessary for responding to objects on the road in real-time.
However, generating high-quality labeled data can become challenging due to the volume of data generated by a single car that needs constant updating. Inadequate data annotation teams can cause a bottleneck in collecting and labeling this extensive dataset. Therefore, an efficient team with quick turnaround times will help manage this problem to avoid slowing down development progress.
Different annotation techniques can be adapted for specific needs like interpolation and segmentation. Advanced 3D cuboid annotation helps sensors process more distance information that enables self-driving cars even at high speeds. Precision mapping and obstacle avoidance functions are critical functions for autonomous vehicles leading research companies investing billions towards these directions.
To benefit from precision without significantly increasing human effort or fatigue costs - automation plays a significant role with companies leveraging machine learning models already generating 65% in savings facilitating quick progress towards full autonomy while maintaining privacy levels has also been taking priority with how many cyber-attacks daily compromise computer systems involving sensitive customer details making security monitoring essential as much as other aspects surrounding self-driving cars' development pipelines' requirements overall quality push culture aims at producing high-performance alternatives against traditional software solutions lowering error rates drastically helping pave the way forward successfully into level five autonomy ensuring we deploy safe vehicles into our roads reducing global fatalities offering economic opportunities through new business models moving forward rapidly using novel technical approaches pushing continually towards higher efficiency annotated datasets centered around safety.
Data Validation As A Crucial Part Of Data Annotation For Self-Driving Vehicles
Data annotation is a crucial part of training self-driving vehicles to navigate real-world environments, and the quality of training data directly affects the safety of autonomous driving. The computer vision system in self-driving cars relies heavily on structured, annotated, and labeled data. As such, ensuring the accuracy and effectiveness of data annotation for self-driving vehicles requires important consideration of data validation.
Data validation is essential to ensure that the annotated data is accurate, complete, and relevant for use in training algorithms. This process can help prevent errors in labeling before they affect the overall performance of an autonomous vehicle.
Good data validation practices highlight issues early on so they can be resolved before problems occur publicly. This process helps create high-quality datasets with fewer errors which increases the safety performance autonomy vehicles working under various weather and road conditions.Auto manufacturers must maintain proper record keeping documentation regarding their testing protocols to provide specific evidence to aid legal defense teams should any accidents happen while their product is being used; thus making it essential that car makers have appropriate processes for creating highly accurate datasets using strong validation techniques. Improving these critical steps will support public trust through emphasizing transparency throughout design phases while ensuring safe usage by customers once released into production environments Despite efforts made by automakers for responsible testing compliance challenges remain around Safety Risk Assessments , explainability gaps, robustness to change distribution between test environment & final destination places where each vehicle will travel at be put in action,e.g., Smart Cities or rural areas with mixed infrastructure/road welfare levels.
The Future Of Data Annotation Quality In Self-Driving Vehicles
As autonomous driving technology continues to advance, the quality of data annotation is becoming increasingly important. High-quality training data is crucial for ensuring the safety of self-driving vehicles and reducing the risk of accidents on the road.
AI-powered data annotation has become an essential tool in improving safety and accuracy for self-driving cars. The use of computer vision, cloud data, car-to-car communication, and car-to-infrastructure communication all require precise image classification and localization through accurate annotations.
As we transition from self-driving technology in laboratories to fully operational systems on the roads, high-quality annotated data will be instrumental in advancing autonomous driving capabilities. To provide an example, Waymo was able to train their machine learning models for different scenarios on the road using annotations as part of their training process.
In summary, as autonomous driving becomes more integrated into everyday life, ensuring that each vehicle has access to accurately annotated datasets will become increasingly imperative. With more reliable data annotation practices developed by industry leaders today and continual technological advancements continually developed tomorrow there is no doubt that the future looks bright for improving overall self-driving safety mechanisms.
Case Studies: Examples Of Successful Data Annotation For Self-Driving Autos
Successful data annotation is a vital aspect of the development of self-driving cars. Several tools, such as 2D boxing, 3D cube, lane line, polygon, semantic segmentation and 3D point cloud annotation are commonly used to train ML models to recognize objects on the road. Tesla leverages data annotation and generation to power their self-driving cars. LiDAR-sensing based vehicles use precise 3D point cloud annotations to identify objects from afar.
Higher-quality annotation enhances computer vision and enables AI-enabled parking management in autonomous cars. Analytics is an industry leader in developing high-quality error-free AI training data for autonomous vehicles. The accuracy of object detection in these machines is primarily dependent on quality annotations created by experts.
Moreover, annotated data facilitates complex tasks like driving vehicles in adverse weather conditions or responding in a dynamic roadside environment with multiple moving parts without any hiccups or delays. Experience and expertise are often vital components of successful completion of any project that requires data analysis; this is particularly valid when it comes down to product development.
Accurate and efficient data annotation for self-driving autos is crucial for successful machine learning implementations. Annotation helps translate human-like actions like recognition into artificial intelligence understandable commands that can function seamlessly within the real world environment without putting human lives at risk.
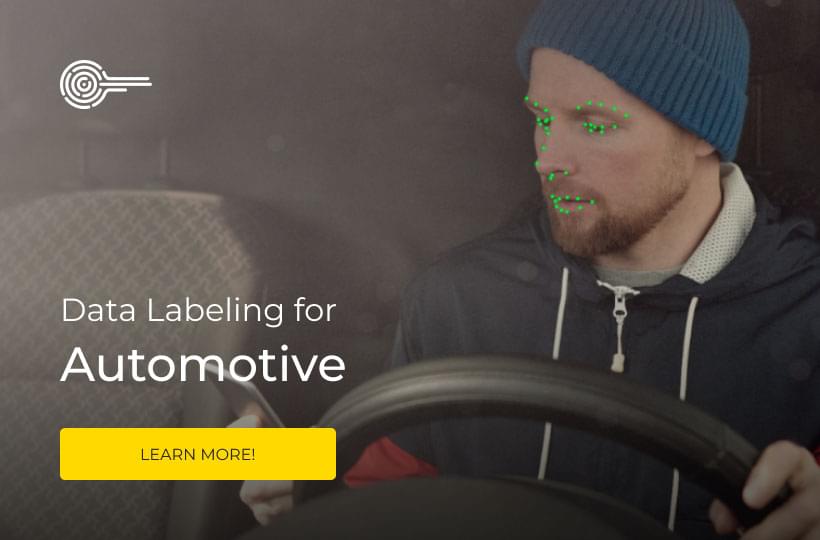
Conclusion
In conclusion, precision does matter when it comes to data annotation quality for self-driving autos. The importance of accurate and reliable data cannot be overstated in the development of self-driving vehicles. Data validation is a crucial part of the data annotation process, and the future of data annotation quality in self-driving autos looks promising with the use of advanced technologies such as machine learning.
Case studies have shown that successful data annotation is possible, and it is important for companies in the autonomous vehicle industry to prioritize data annotation quality in order to ensure the safety and reliability of their products. As the industry continues to grow and evolve, it is important to stay up-to-date with the latest developments and technologies in data annotation for self-driving autos.