Data Annotation Made Easy: A Beginner's Guide
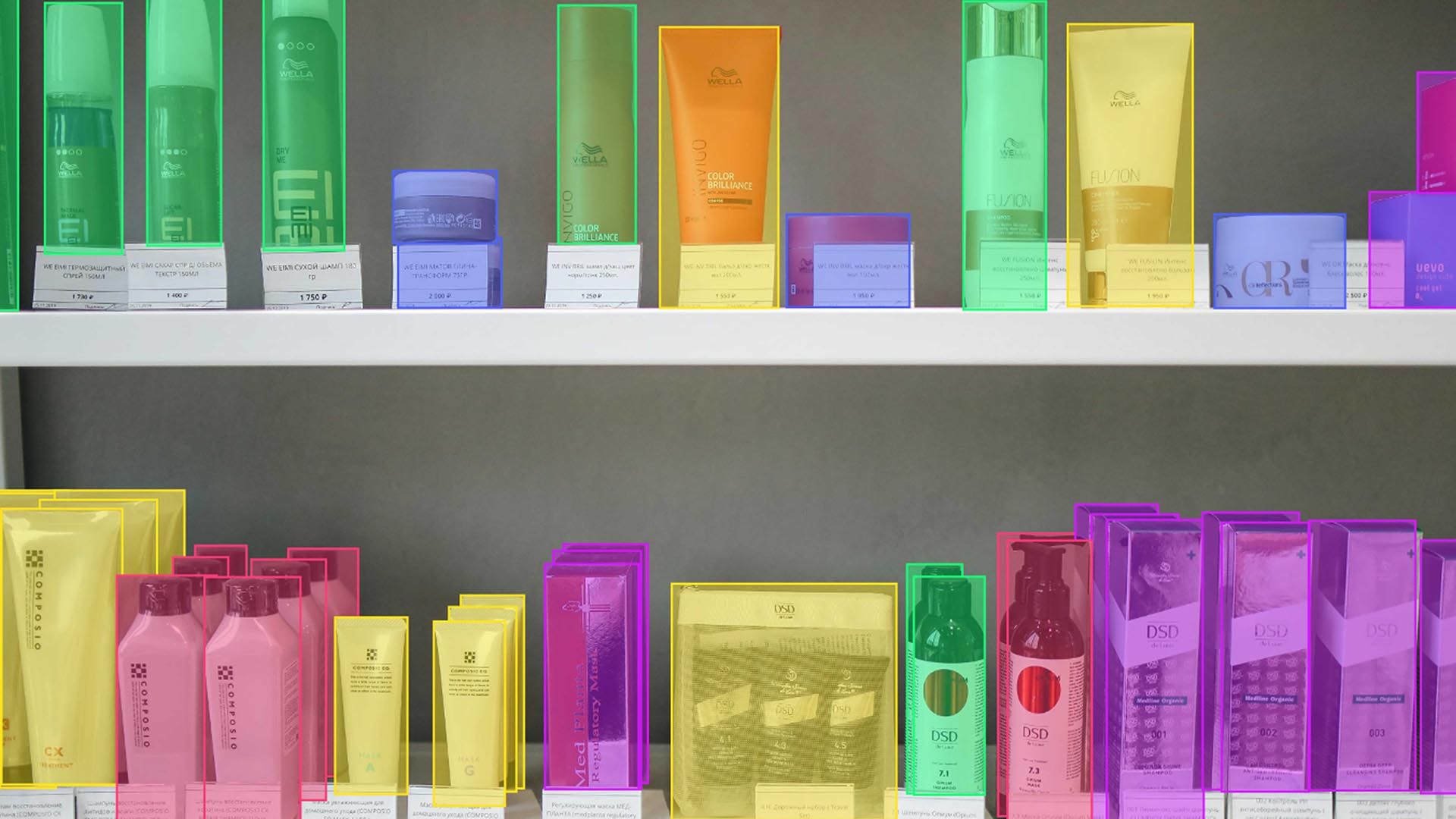
If you're stepping into the world of machine learning and computer vision, you must know about data annotation. This data annotation guide for beginners will show you the basics. You'll learn how detailed labels are the key to training machines. They can then understand images and videos accurately. This lets AI act more like we do, recognizing things in its environment.
This guide will help you understand how to annotate data for beginners. You'll see why this task is so important for AI's growth.
Key Takeaways
- Embrace the significance of data annotation in nurturing precise computer vision models.
- Comprehend the diverse range of annotation types, from bounding boxes to keypoint annotations.
- Recognize the importance of high-quality, accurate annotations in enhancing AI reliability.
- Understand the balance and challenges of manual and automated annotation tools.
- Discover the essential role of labeled data in groundbreaking applications, including healthcare and environmental monitoring.
- Appreciate that easy data annotation for beginners is accessible through modern tools and platforms, opening opportunities for involvement.

Understanding the Role of Data Annotation in Machine Learning
In the world of machine learning, data annotation is key. It's like the training for algorithms, helping them view the world rightly. For newcomers, knowing about data annotation's mechanics is vital. It helps AI see patterns and objects right, shaping their choices. Let's explore why careful data labeling is so important in AI.
The Crucial Nature of Accurate Data Labeling
Data labeling is crucial for AI, just like solid foundations are for a house. When you label data correctly and with detail, AI learns to apply this in real situations. Top-notch data labeling practices improve machine learning's quality and dependability. Think of it like teaching a child to spot items correctly, with every accurate label boosting their knowledge.
Impacts of High-Quality Annotation on AI Performance
Good data labeling has major benefits for AI. Important tech like self-driving cars and medical imaging need perfectly labeled data for precise results. In self-driving cars, mistaking a person for a sign could lead to an accident. So, superb data labeling is a must for any newcomer in data annotation to build strong AI models.
When starting on data annotation, understanding the challenges is key. It's not just about putting accurate labels; you also need to handle big data sets. Here's a quick look at those challenges:
Challenge | Description | Impact |
---|---|---|
Consistency among annotators | Variance in labeling due to subjective interpretation | Can lead to inconsistent AI training data |
Large dataset management | Handling extensive volumes of data efficiently | Crucial for scaling AI models |
Annotation tools and technology | From manual tools to advanced automated platforms | Affects the speed and quality of annotations |
Grasping these elements and their role in machine learning's journey is critical. This guide to data annotation explains how managing these aspects can spark AI innovation.
Data Annotation Guide for Beginners: Starting on the Right Foot
Starting in Artificial Intelligence means picking the right beginner data annotation tutorial to lay your groundwork. Data annotation labels data, making sure machine learning models work well. This task is key in areas like image recognition and autonomous driving. They need it to perform their best.
Learning about data annotation in AI sets you up to work on many cool projects. These could include spotting cancer or examining satellite images for erosion signs. This knowledge helps in jobs where precision and wide data analysis are key.
Breaking Down Types of Data Annotations
Starting with data annotation means learning its many types. Each type is important for different uses in AI and machine learning. Let's look at some key types. These are core for teaching AI to understand images correctly and make sense of them.
Image Labeling and Its Significance
Image labeling is crucial in data annotation. It puts images into categories by adding tags. This helps algorithms understand and sort images. From sorting photos to complex computer vision tasks, it's key.
Bounding Boxes: The Basics
Bounding boxes are rectangles drawn around objects in images. They’re vital for systems spotting objects. This includes self-driving cars knowing where objects are and stores keeping track of products.
Exploring Semantic and Instance Segmentation
Semantic segmentation labels every pixel of an image with an object type. It helps AI understand images better. Instance segmentation goes further. It identifies each object in detail. This is important for scenes with multiple similar objects.
The Intricate World of Landmark Annotation
Landmark annotation marks unique points on images, like in facial recognition tech. It's important for accurate measurements and recognizing detailed features.
Polygonal Annotations for Detailed Outlining
Polygonal annotations use shapes to outline objects in images. They make it easier to remove backgrounds or identify objects. This improves the accuracy of AI systems.
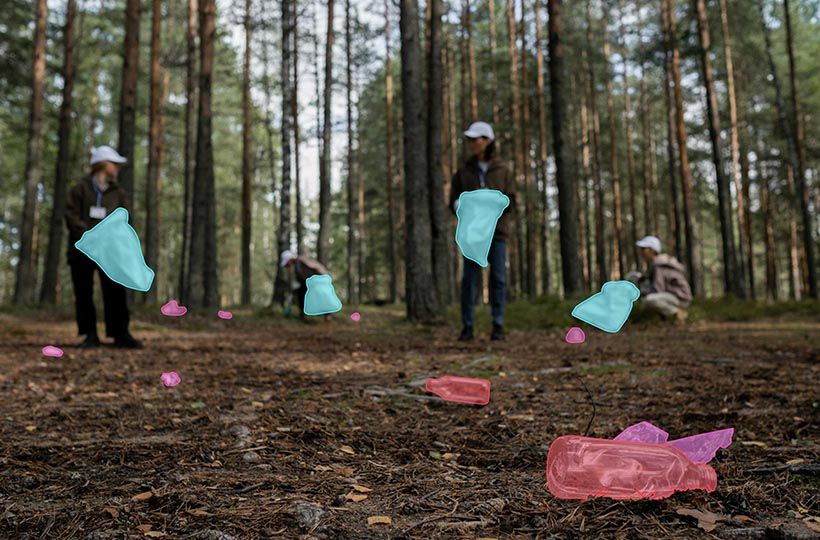
The Art and Science of Annotating Visual Data
Annotating visual data isn’t just a task; it's an art and science mix. It's important for creating top-notch AI and machine learning. Those new to data annotation must learn to tackle challenges and make their practices better.
Key Challenges in Data Annotation
Starting with data annotation, you'll see it comes with its hurdles. Keeping quality high in large sets is hard. Especially with complex pictures, like detailed medical scans or city photos packed with details. And, people may see things differently, so clear rules and good training are musts.
Achieving Consistency in Annotated Datasets
But how can you keep annotations top-notch for sharp AI? Setting clear rules everyone can follow is step one. For hard cases, rely on people more than machines. Regularly checking and fixing annotations also keeps things sharp over time.
Technique | Description | Implementation Strategy |
---|---|---|
Auto Object Segmentation | Automated identification and localization of objects using vector labels. | Use in preliminary rounds of annotation to define and correct boundaries. |
Model-assisted Labeling (MAL) | Utilizes AI tools during annotation of moving or complex objects. | Deploy for dynamic environments like videos to enhance tracking accuracy. |
Bounding Boxes | Common method to encapsulate objects within images for easier identification. | Apply for primary object detection tasks to train detection models. |
Polygon Annotation | Used to outline clear, defined shapes and paths of objects. | Implement in scenarios where shape precision is necessary for model performance. |
Robust documentation on a platform such as Keylabs helps show you the ropes and why it matters. Learning proper annotation can really help push AI forward. This supports work that can change the world for the better.
How Data Annotation Fuels Computer Vision Innovations
Accurate data annotation is key for computer vision. It helps AI understand our visual world. For those new to the field, knowing how annotated data impacts AI is important. It's crucial in areas like healthcare, self-driving cars, and safety, making AI navigate our world better.
As you learn about data annotation, know it's essential. It boosts AI's skills and effectiveness. There are many annotation types, from simple boxes to detailed points, all improving AI learning.
For new learners, data labeling is more than marking images. It's about teaching machines to recognize the world. This can lead to futuristic AI solutions.
Automated data tools have made labeling quicker and less error-prone. But, every industry has unique labeling needs. Healthcare, for instance, needs extra care due to life-critical decisions. Your specific labeling approach will highly depend on the industry.
Industry | Application of Data Annotation | Benefits |
---|---|---|
Healthcare | Cancer detection, medical diagnostics | Increases diagnostic precision, speeds up patient care |
Autonomous Driving | Object and obstacle detection | Enhances vehicle safety, enables reliable autonomous navigation |
Public Safety | Surveillance, behavior analysis | Improves security measures, aids in crime prevention |
Navigating the Complexities of Data Labeling
Starting with data annotation needs careful thought. This is especially true for those new to it. Knowing how much to do manually and with tech helps a lot. It's explained well in the data annotation guide for beginners.
Striking a Balance: Manual Versus Automated Annotation
Doing it by hand means you get precise results. This is key for jobs like looking at medical images. But, it takes a lot of time and effort, which might not be the best for first-timers. On the other hand, using technology can speed things up but doesn't always catch the fine details in images. This can make the data less accurate.
Scalability and Complexity in Annotating Large Datasets
Big data tasks require more effort to keep the quality high. Entering automated tools can help deal with a lot of data quicker. This makes managing big tasks easier. Yet, for complex jobs, a mix of tech and manual work is best to keep everything just right.
If you are new and looking for easy data annotation, try doing it by hand and then using tools like Keylabs to see the difference. This mix can work well in fields like health and self-driving cars. It helps, whether it’s spotting diseases or planning cities from above. The right tools can help a lot. They make tough jobs doable and do them well.
Deciding between doing it by hand or with tech depends on your project. It aims to keep a good balance of being right, affordable, and fast. For new folks in data annotation, getting to know how this works is key. It makes you good at helping AI grow in useful ways.
Tips for Efficient and Effective Data Annotation
If you're starting in machine learning, learning easy data annotation for beginners is key. It improves AI systems by giving them better training data. Here, we offer tips to boost your data annotation game.
First, pick the right data annotation types for your project. This might be labeling images, setting bounding boxes, or noting things in videos. Each kind supports the AI in different ways. Know which tools and platforms can help. For detailed work, try manual tools like LabelImg or CVAT. For a smoother process, tools like Labelbox can automate some parts.
- Keep your annotations consistent. This avoids mistakes during training, which can affect the AI's performance.
- Use AI-powered tools when you can. They speed up the work but still keep the quality high, even with a lot of data.
- Join or lead sessions to share and learn about data annotation. This will keep you up to date with new methods and tools.
Also, connect with online groups that focus on easy data annotation for beginners. They can offer tips and help with any problems you face while annotating data.
To be good at data annotation, you need to know the right tools and the best ways to use them. Avoiding common mistakes is also crucial. These tips will help you make a real difference in AI with your accurate and efficient data annotation work.
Summary
Data annotation is vital for AI's growth, helping it understand the world better. You learn more than how to tag images - you learn the importance of being precise and consistent. Annotating data means creating high-quality datasets, crucial for AI's accuracy and reliability.
Your work, be it marking bounding boxes or annotating keypoints, fills AI with knowledge. Understanding tools like Keylabs and managing datasets is key. This guide shows how every step you take helps shape the AI that will change the world.
This guide is a step towards improving AI accuracy and efficiency. Your role is vital, ensuring AI works well by carefully annotating data. Mastering data annotation means you're enhancing AI's future, and in turn, yours.
FAQ
What Is Data Annotation?
Data annotation labels data like images and text. This makes it known to AI. Machines learn from this data to make accurate choices. It's about teaching AI to spot patterns using labels.
Why Is Accurate Data Labeling Important?
Good data labels help AI understand images correctly. But, bad labels can confuse AI, making it predict wrong things. So, precise labeling is key for AI to work well.
What Are Some Common Types of Data Annotations?
There are many ways to label data. These include tags for images, bounding boxes, and more. Each method helps AI see and understand different things in pictures.
What Are Bounding Boxes?
Bounding boxes are rectangles around objects in pictures. They help machines find and recognize these items. This makes them useful for things like spotting cars in photos.
What Is the Difference Between Semantic Segmentation and Instance Segmentation?
Semantic segmentation picks out what each pixel shows. It's like painting each part of a photo with a label to explain what's there. Instance segmentation is more detailed. It tells the AI which separate items it's looking at.
What Challenges Exist in Data Annotation?
Biggest challenges include handling lots of data and keeping it accurate. There's also the issue of different people labeling in different ways. To fight these, making strict rules and checks is important.
How Do Manual and Automated Annotation Compare?
Manual annotation is very accurate but takes a lot of time. Automated tools are faster but can sometimes guess wrong with complex images.
Can You Offer Any Tips for Efficient and Effective Data Annotation?
Choosing the right annotation tools and knowing your project's needs are key. Keep labeling consistent and clear. Learning about different labeling types can also make your work better.
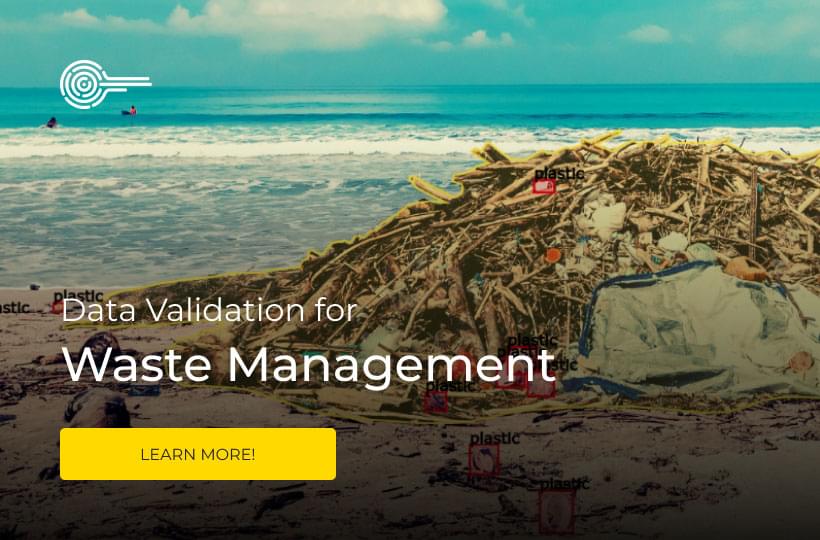